Abstract
Free full text

Age moderates the association between social integration and diurnal cortisol measures
Abstract
Social integration is defined as the degree to which an individual participates in a broad range of social relationships. Although measures of social integration vary across studies, it is often assessed as the number of social roles (e.g., parent, friend, student, volunteer) that an individual reports actively participating in. More socially integrated individuals tend to be healthier than those less socially integrated, but the biological mechanisms through which this occurs remain unclear. One possibility is that social integration might alter the function of the hypothalamic-pituitary-adrenal axis, of which cortisol is a key product, and in turn influence a broad range of health outcomes. This study examined the association between social integration and two indices of cortisol in a community sample of 680 healthy men and women aged 18–55. Because the social roles held by younger individuals may be more numerous yet superficial than those held by older individuals, this study also tested the hypothesis that these associations could be moderated by age such that lower levels of integration would be associated with cortisol dysregulation for older but not younger individuals in our sample. Participants provided salivary cortisol samples during waking hours on three days that were used to calculate diurnal cortisol levels and slopes. Increased social integration was associated with lower cortisol AUC among older (ages 35–55) but not younger (ages 18–34) individuals in our sample. Moreover, while increased social integration was associated with steeper diurnal cortisol slopes regardless of age, this association was strongest among older individuals. Differences in health behaviors, affect, and psychological stress did not mediate these associations. The results of this study support cortisol as a candidate biological mechanism through which increased social integration is associated with better physical health among older individuals.
1. Introduction
Social integration is the degree to which an individual is included in or connected to a broad range of social relationships (Brissette et al., 2000). Although measures of social integration vary across studies, it is often assessed as the number of social roles (e.g., parent, friend, student, volunteer) that an individual reports actively participating in (e.g., Chang et al., 2017; Cohen et al., 1997). Numerous studies demonstrate an association between social integration and lower rates of morbidity (e.g., Barefoot et al., 2005; Seeman, 1996; Kroenke et al., 2012) and all-cause mortality (reviews by Holt-Lunstad et al., 2010; Shor & Roelfs, 2015). Importantly, social integration appears to be theoretically and empirically distinct from related social interaction measures that assess social contact frequency (Brissette et al., 2000) and has been shown to be more reliably associated with health outcomes (Barefoot et al., 2005; Chang et al., 2017; Cheng et al., 2014; Cohen et al., 1997; Holt-Lunstad et al., 2010; Shor & Roelfs, 2015) than measures of contact frequency.
Despite this compelling evidence, there are few studies of plausible biological mechanisms through which greater social integration may be associated with better long-term health. Previous work has demonstrated that more socially integrated individuals have lower allostatic loads assessed by an undifferentiated aggregation of biomarkers reflecting cardiovascular activity, metabolism and adipose tissue deposition, glucose metabolism, hypothalamic-pituitary-adrenal axis (HPA), and sympathetic nervous system (SNS) activity (Seeman et al., 2002), as well as lower levels of systemic inflammation (Shankar et al., 2011). An alternative possibility is that social integration might influence health outcomes primarily through its impact on the HPA axis, of which cortisol is a key product. Cortisol is a glucocorticoid hormone thought to regulate immunological and metabolic processes relevant to health (Sapolsky et al., 2000). Cortisol follows a diurnal pattern, rising rapidly immediately after waking and then declining steadily throughout the waking day. Flatter diurnal cortisol slopes have been associated with negative physical and mental health outcomes (review by Adam et al., 2017). Heightened total cortisol AUC has similarly been associated with negative outcomes including metabolic syndrome (Anagnostis et al., 2009; Brunner et al., 2002) and coronary atherosclerosis (Dekker et al., 2008). Flatter diurnal cortisol slopes and heightened total cortisol AUC may therefore represent distinct plausible biological pathways through which low levels of social integration are associated with poorer physical health.
There are several potential psychological and behavioral pathways through which social integration may alter the HPA response. For example, those low in social integration (socially isolated) may experience psychological stress which in turn induces HPA axis dysregulation (Berkman et al., 2000; Cohen et al., 1995; Grant et al., 2009). Alternatively, greater social integration may promote positive affect, decrease negative affect, or act as a source of social pressure and motivation to engage in better health practices (Berkman & Breslow, 1983; Berkman & Glass, 2000; Cohen, 1988; Cohen, 2004; Cohen & Lemay, 2007; Umberson, 1987). All of these have been found to aid in the regulation of the HPA response (e.g., Badrick et al., 2008; Badrick et al., 2007).
Finally, the majority of social integration and health research has focused on older adults (ages 55 and above) due to their higher risk for morbidity and mortality. One way to study this association in younger individuals is to focus on how social integration relates to biological markers with implications for long-term health. However, few studies thus far have pursued this strategy in younger participants. One notable exception is work by Yang et al. (2016), which found that lower levels of social integration were associated with greater inflammation and increased hypertensive risk among older adults (two samples ages 50–98 and 57–91), but not among younger adults (ages 24–32) or adolescents (ages 12–18). The social motivations and social roles held by younger individuals are likely to be quite different than those of older individuals (Carstensen, 1992). Younger individuals may possess more numerous yet more superficial connections, while older individuals may possess fewer but more meaningful connections. Moreover, the more superficial connections among younger individuals may not be sufficient to drive affective response or produce the social pressures and motivation to alter health practices. Consistent with previous research (Yang et al., 2016), we hypothesized that lower levels of social integration would be associated with greater average cortisol AUC and flatter diurnal slopes for individuals in their mid- to late-30s and older, but that social integration would not be associated with cortisol dysregulation for individuals in their early-30s and younger.
In the current study, we collected measures of social integration, social contact frequency, and salivary cortisol from 680 healthy volunteers between the ages of 18–55. Social integration was assessed as the number of social roles an individual participates in; and social contact frequency as the average number of people one interacts with daily. We expected social integration to be a more potent predictor of diurnal cortisol AUC and slopes than related measures of social contact frequency (Cohen et al., 1997; Shor & Roelfs, 2015). Salivary cortisol was collected across the waking period of three separate days. We hypothesized an association of greater social integration with lower cortisol AUC and steeper diurnal slopes in older but not younger individuals in our sample. Moreover, we expected that social integration would be associated with more positive affect, less negative affect, less psychological stress, and better health practices among older individuals, which would in turn mediate the association between social integration and diurnal cortisol (e.g., Cohen, 2004). Consistent with earlier research suggesting that social integration is a more potent predictor of morbidity and mortality than measures of social contact frequency, we expected social integration to account for more variance than contact frequency, and predict cortisol outcomes independently of frequency.
2. Methods
2.1 Participants
We examined aggregated archival data from three viral-challenge studies conducted by our laboratory between 1997 and 2011: Pittsburgh Cold Study 2 (PCS2), Pittsburgh Cold Study 3 (PCS3), and Pittsburgh Mind-Body Center Study (PMBC). Complete procedures and data from each study can be found at www.commoncoldproject.com. Prior to the beginning of all three studies, participants completed health screenings on the telephone, as well as an in-person medical examination administered by a study physician to determine that they were in good health and did not meet any study exclusion criteria: history of chronic illness, previous nasal/otologic surgery, regular use of prohibited medication, recent psychiatric diagnosis or hospitalization, HIV seropositivity, abnormal clinical profiles as determined by urinalysis, complete blood count, and analysis of blood chemistry, currently pregnant, lactating, or planning to become pregnant, recently participated in another psychology study, having cold or flu-like symptoms within the previous 30 days, previous hospitalization due to flu-like illness, use of steroids or immunosuppressants within previous 3 months, or allergies to egg or egg-products.
In total, 740 participants completed the three studies. We excluded participants from analyses if they did not provide information about their social interactions during the interview period (n=1), or were missing either relevant covariates (n=1) or primary outcome data (n=58). The final sample consisted of 680 healthy adults between the ages of 18 and 55 years (M=31.39, SD=10.8) recruited from the Pittsburgh, PA area. The sample was 49.7% female, 65.7% white, 30.0% African-American, and 4.3% other ethnicities. Participants provided informed consent prior to study enrollment and received financial compensation following their participation. The institutional review boards of both Carnegie Mellon University and the University of Pittsburgh School of Medicine approved each study.
2.2 Procedures
As part of the parent studies, participants were then exposed to a virus known to cause the common cold, then followed in quarantine and monitored for the development of infection. All data reported here were collected during the baseline period of the parent studies prior to viral-exposure. Eligible participants completed baseline assessments at home, during in-person laboratory visits, and during an initial day of quarantine prior to viral-exposure.
2.3 Measures
2.3.1 Salivary cortisol
Salivary cortisol samples were collected across the waking period of three separate non-consecutive days (see suggestions by Kraemer et al., 2006; Saxbe, 2008). Participants were provided with rolls of cotton inside of a plastic collection tube used to collect each sample (Salivettes®; Sarstedt AG & Co, Nümbrecht, Germany), as well as detailed written instructions and either a pre-programmed wristwatch (PCS2) or handheld computer (PCS3, PMBC) that signaled participants at each collection time. For each collection, participants were instructed to place the cotton in their mouth, allow it to become saturated with saliva, spit it back into the tube, and then reseal the tube. At each collection time, the signaling device provided a unique alphanumeric code. Participants were instructed to write this code as well as the time and date of collection on each tube. Finally, participants stored completed samples in their refrigerator and then brought the tubes with them to their baseline study session where they were collected by staff and frozen for storage.
Two of the three sets of cortisol samples were collected on separate non-consecutive days in the participant’s natural environment 1–6 weeks prior to viral challenge. The third set of samples was collected on the baseline day of quarantine prior to viral-challenge. In two of the studies (PMBC, PCS3), cortisol was collected seven times daily (1, 2, 4, 6, 8, 12, and 14 h post-waking) on each pre-quarantine day and eight times (0, 1, 2, 4, 5, 7, 9, and 14 h post-waking) on the day of quarantine. In the third study (PCS2), cortisol was collected eleven times daily on each pre-quarantine day and fourteen times on the day of quarantine. In order to establish approximate equivalency across the three studies while still capturing the diurnal rhythm of cortisol, we used seven samples from pre-quarantine days (assessed at 1, 2, 4, 7, 9, 11, and 14 h post-waking) and the eight closest samples from the day of quarantine (0, 1, 4.25, 6.25, 7.25, 9.25, 12.75, and 16.75 h post-waking) when analyzing participants from PCS2 (Chin et al. 2017; Janicki-Deverts et al., 2016; also see www.commoncoldproject.com). Note that cortisol samples necessary to compute the cortisol awakening response (CAR; 30 minutes post-waking) were not collected in two of the three studies we aggregated data from, and the CAR was therefore not assessed as an outcome here.
For two of the three studies (PCS2, PCS3), cortisol assays were performed by the laboratory of Dr. Clemens Kirschbaum in Dresden, Germany. Cortisol concentrations were determined using time-resolved fluorescence immunoassays with a cortisol-biotin conjugate as a tracer (Dressendörfer et al., 1992). Intra- and inter-assay variability were both less than 12%. For the third study (PMBC), assays were performed by the Immunological Monitoring and Cellular Products Laboratory at the University of Pittsburgh Cancer Institute. Cortisol concentrations were determined using enzyme-linked immunosorbent assays (Salimetrics, State College, PA). The average deviation between individual replicate pairs was 4%. Previous research has shown that the two assays used across the three studies provide very similar results (r=0.97; Dressendörfer et al., 1992). However to control for any potential differences in assays across studies, as well as any other potentially relevant study differences, we included two dummy variables representing the three studies covariates in all analyses (PCS3 was the reference category).
For all studies, samples were only included for analysis if they were collected ±45 min of scheduled collection time. Samples collected outside of this range were considered missing. This was done based on previous work demonstrating that this cutoff range would allow us to maintain at least 95% of our data while also accurately capturing cortisol’s diurnal rhythm (e.g., Chin et al., 2017; Janicki-Deverts et al., 2016; also see www.commoncoldproject.com). The actual time of collection (rather than the expected time of collection) was used in all analyses.
To calculate daily cortisol AUC, the area under the curve with respect to ground (AUCG) was computed for each day among participants with sufficient data (Pruessner et al., 2003). Sufficient data was defined as not missing any of the first three samples of the day or more than two of the day’s remaining samples. Next, average daily cortisol AUC was computed for participants who had sufficient data for at least two of three days. This was done by averaging the total adjusted concentrations from all days with available data. Finally, average daily cortisol AUC was log-10 transformed due to the non-normal distribution of data. To calculate average diurnal cortisol slopes, multilevel modeling methods were used (see Data Analysis below). Multilevel models are advantageous because they accommodate missing data and hence allow for the use of all available data.
2.3.2 Social integration
We assessed social integration in two different ways. First, daily (evening) telephone interviews were conducted during the baseline period of the study to assess participants’ daily social activities. Prior to the start of the interview period, participants were asked to provide information about the composition of their social networks. Specifically, participants identified members of their social network that they were most likely to interact with during the interview period and classified these individuals into one of 20 possible social roles: spouse/partner, boyfriend/girlfriend, ex-partner, child, parent, parent-in-law, close relative, non-close relative, close-friend, non-close friend, roommate, neighbor, acquaintance, religious group member, volunteer group member, other group member, person at work, person at school, service/professional, other. In PCS2, participants were interviewed on 3 evenings a week (2 weekdays, 1 weekend day) for 2 weeks for a total of six interviews. As part of a larger battery of interview items, participants were asked to indicate each social interaction they had that day and with whom they had interacted. For the purpose of this interview, interactions were defined as spending time with one or more persons for at least ten minutes (Reis & Wheeler, 1991). In PCS3 and PMBC, participants were interviewed every evening for 2 weeks for a total of fourteen interviews. As part of a larger series of interview questions, participants were asked to indicate with whom they had completed six types of activities during the past 24 hours: (a) meals; (b) leisure activity at home; (c) leisure activity away from home; (d) work around the house; (e) family/personal errands away from home; and (f) any other type of activity. Interview responses were used to compute the total number of social roles participated in during the interview period. When calculating total number of social roles, we sought to establish equivalency across the three studies to control for the number of interviews conducted. To do this, only interviews from the first four weekdays and first two weekends for PCS3 and PMBC participants were included when calculating the total number of social roles.
Second, the Social Network Index (SNI; Cohen et al., 1997) questionnaire was administered at baseline to retrospectively assess participation in 12 types of social roles: spouse, parent, child, child-in-law, close relative, close friend, church or religious group member, student, employee/coworker, neighbor, volunteer, or other group member. Social integration was defined as the total number of social roles participated in at least once every two weeks.
Finally, we created a composite measure of social integration by standardizing the measures of total social roles assessed via daily interviews and of total social roles assessed using the SNI, then averaging the standardized scores. This composite measure was used in all analyses reported below. See Table 1 for the exact items used to assess social integration.
Table 1
Summary of social integration and social contact frequency variables.
Variable | Description |
---|---|
Social integration | |
1. Total Roles (Daily Interviews) | Total number of social roles individuals reported participating in across the six days of interviews. |
2. Total Social Roles (SNI Questionnaire) | Total number of social roles individuals reported participating in at least once every two weeks. |
Social contact frequency | |
1. Average Partners (Daily Interviews) | Average number of people participants reported interacting with each day. |
2. Total Network Members (SNI Questionnaire) | Total number of people participants reported being in contact with at least once every two weeks. |
2.3.3 Social contact frequency
Interview responses were also used to compute average number of people interacted with each day. Averages were computed by dividing the total number of people throughout the interview period by the number of interviews completed. Total number of people interacted with was also assessed by the SNI questionnaire as the number of people respondents reported being in contact with in their social roles at least once every two weeks (for example: how many friends did you talk to in person or on the phone during the last two weeks?). To prevent artificial inflation of total network members contacted in a single role, participants could only receive credit for up to 7 network members per social role. Finally, we created a composite measure of social contact frequency by standardizing the measures of average number of social partners assessed via daily interviews, and of total network members assessed using the SNI, then averaging the two standardized scores. This composite measure was used in all analyses reported below. See Table 1 for the exact items used to assess social contact frequency.
2.3.4 Perceived stress
Perceived stress was measured during baseline prior to quarantine using the 10-item Perceived Stress Scale (PSS-10; Cohen & Williamson, 1988). The PSS-10 asks participants to indicate how often they found their lives to be unpredictable, uncontrollable, and overloaded throughout the past month using a five-point Likert scale ranging from 0 (“Never”) to 4 (“Very Often”). Responses to all items are summed to create an index of total perceived stress (α=.872).
2.3.5 Health behaviors
Three health behaviors (smoking, drinking, physical activity) were measured during baseline prior to quarantine via self-report. Smoking was assessed as the average number of cigarettes participants reported smoking per day. Drinking was assessed as the average number of alcoholic drinks participants reported consuming per week. Physical activity was assessed as the number of minutes that participants reported exercising per week.
2.3.6 Positive affect
Positive affect was assessed at baseline as part of the daily telephone interviews using adjectives derived from a factor analysis of the Profile of Mood States (Usala & Hertzog, 1989). In PCS2, participants were asked to indicate how accurately from 0 (“Not at all accurate”) to 4 (“Extremely accurate”) each of nine positive mood adjectives described how they felt in the previous 24 hours: lively, full-of-pep, energetic, happy, pleased, cheerful, at ease, calm, and relaxed. In PCS3 and PMBC, participants were asked to indicate how accurately each of six positive mood adjectives described how they felt in the previous 24 hours: lively, full-of-pep, happy, cheerful, at ease, calm. For all studies, daily positive affect was calculated by averaging the responses to all items. The internal reliabilities (α) for each day ranged from .89–.93 in PCS2, .83–.89 for PCS3, and .82–.90 for PMBC. Finally, trait positive affect was determined by computing average daily positive affect across all interview days (e.g., Polk et al., 2005).
2.3.7 Negative affect
Negative affect was assessed at baseline as part of the daily telephone interviews using adjectives derived from a factor analysis of the Profile of Mood States (Usala & Hertzog, 1989). In PCS2, participants were asked to indicate how accurately from 0 (“Not at all accurate”) to 4 (“Extremely accurate”) each of nine negative mood adjectives described how they felt in the previous 24 hours: hostile, angry, resentful, on edge, tense, nervous, sad, unhappy, depressed. In PCS3 and PMBC, participants were asked to indicate how accurately each of six negative mood adjectives described how they felt in the previous 24 hours: hostile, angry, on edge, tense, sad, and unhappy. For all studies, daily negative affect was calculated by averaging the responses to all items. The internal reliabilities (α) for each day ranged from .87–.92 in PCS2, .83–.91 for PCS3, and .83–.91 for PMBC. Finally, trait negative affect was determined by computing average daily negative affect across all interview days (e.g., Cohen et al., 2003).
2.3.8 Primary control variables
The primary control variables were selected a priori because of their potential associations with social integration, social contact frequency, and cortisol (e.g., Berkman et al., 2000; Berkman et al., 2004; Champaneri et al., 2013; Cohen et al., 2006; Van Cauter et al., 1996). Five of the control variables were assessed at screening: sex (male or female), race (categorized as white, black, or other), education (years), body mass index (BMI; weight in kilograms/height in meters2), and season of the year (spring, summer, fall, or winter). To adjust for slight differences across studies, two dummy variables indicating which of the three studies the participant had been in (PCS2, PMBC, PCS3) were included as covariates. All six of these covariates were included in all models and analyses. (As discussed later, age was entered as a main effect and in interaction with the social variables).
Finally, because earlier analysis of this data found an association between cortisol measures and marital status (Chin et al., 2017), marital status was included as an additional covariate in secondary analyses to demonstrate that any associations between social integration, social contact frequency, and cortisol were not simply driven by marital status (see earlier description). In addition, two dummy coded interaction terms were also included in secondary analyses in order control for the interaction between marital status and age (Never Married × Age, Previously Married × Age).
Each covariate was mean-centered prior to analysis. Although mean-centering binary variables such as sex or race may seem unintuitive, this is an accepted statistical practice that allows for the interpretation of multilevel regression intercepts and slopes regardless of individual differences in various binary covariates (for discussions regarding the logic of centering binary variables, see Bryk & Raudenbush, 2002; Enders & Tofighi, 2007; Hox, 2010).
2.4 Data Analysis
2.4.1 Average cortisol AUC
Initial analyses were performed using SPSS (Version 21; IBM Corporation). Hierarchical multiple linear regression was used to examine the association of social integration and social contact frequency with average cortisol AUC. Social integration and social contact frequency were initially tested individually in separate models, then tested in a single model with all variables entered together. In the first step, we entered the six primary control variables. In the second step, we entered age and social integration or social contact frequency. In models used to test the moderating effect of age, we added a third step to each model that included social integration or social contact frequency by age interaction term. In other words, models testing for the main effect of social integration or social contact frequency did not include interaction terms but models testing for interactions included both main effects as well as the interaction term (Cohen et al., 2013). We report standardized regression coefficients (β), standard errors (SE), and p-values.
Significant interactions were probed using the PROCESS macro for SPSS (Version 2.16.1; Hayes, 2013) by testing the conditional effects of social integration or social contact frequency at three levels of age: one standard deviation below the mean (20.6 years), the mean (31.4 years), and one standard deviation above the mean (42.2 years). Additionally, the Johnson-Neyman technique was used to identify regions of significance. Finally, we also tested whether psychological stress, positive affect, or better health practices mediated the association between social integration or social contact frequency and cortisol at each level of age (i.e., moderated mediation). We report unstandardized regression coefficients (b) and 95% CIs for the b-values, as well as p-values for the mediation analyses.
2.4.2 Diurnal cortisol slopes
We used multilevel modeling to test whether diurnal cortisol slopes differed as a function of social integration or social contact frequency, as well as whether age moderated these associations. We used the software HLM (Version 6.08; Raudenbush et al., 2004) to test 3-level models. At Level-1, log-transformed cortisol values were predicted as a function of time (in hours) and time-squared since the first sample of the day. At Level-2, the Level-1 intercept and slope were modeled by intercept terms for the three sampling days. At Level-3, the Level-2 intercepts were predicted as a function of individual differences in age and social integration or social contact frequency. In models testing for statistical interactions between social integration or social contact frequency and age, the interaction term was added at Level-3 as well. Models were estimated using an unstructured covariance matrix. Random effects were modeled for all Level-1 and Level-2 intercept and slope terms. Finally, we adjusted for all primary control variables at Level-3 (sex, race, education, BMI, season of the year, study). Model equations are presented in the Online Supplementary Material.
3. Results
3.1 Cortisol AUC
Adjusting for all primary control variables, there were no main effects of either social integration or social contact frequency on average cortisol AUC; there was also no main effect of age on cortisol AUC, β=.045, SE=.001, p=.211. In separate models adjusted for all primary control variables, there was a Social Integration × Age interaction on average cortisol AUC and a marginal Social Contact Frequency × Age interaction on average cortisol AUC (see Table 2). To determine whether these effects were independent, both interactions were entered simultaneously into a single model adjusted for all primary control variables. In this model, only the Social Integration × Age interaction remained marginally associated with cortisol AUC (see Table 2).
Table 2
Fully adjusted associations between social integration, social contact frequency, and average cortisol AUC moderated by age
Separate Regressions | Entered Simultaneously | |||||
---|---|---|---|---|---|---|
| ||||||
β | SE | p | β | SE | p | |
Social Integration × Age | −.085 | .000 | .009 | −.081 | .001 | .066 |
Social Contact Frequency × Age | −.061 | .000 | .062 | −.005 | .001 | .913 |
We probed the interaction between Social Integration × Age in order to explore the nature of this ceffect. The PROCESS macro for SPSS was used to test the conditional effects of social integration on cortisol at three levels of age: one standard deviation below the mean (20.6 years), the mean (31.4 years), and one standard deviation above the mean (42.2 years). There were no associations of social integration and cortisol at 1 SD below the mean age or the mean age. However at 1 SD above the mean age, greater social integration was associated with lower total cortisol AUC, b=−.0166, 95% CI [−.0281, −.0050], p=.005. The Johnson-Neyman technique shows that the relationship between social integration and age is significant when age is greater than 34.92 years.
3.2 Diurnal cortisol slopes
We used multilevel modeling to test whether diurnal cortisol slopes differed as a function of social integration or social contact frequency. All models included both a linear and quadratic term for time, but only results from the higher-order term (i.e., the quadratic term) were interpreted and reported. First, in models without social integration or the interaction between social integration and age, there was an interaction between age and the linear term for time such that older individuals had steeper diurnal declines than younger individuals in this sample, γ=−0.002, p<.001, 95% CI [−0.0020, −0.0011]. There was a significant three-way interaction between age, social integration, and the linear term for time in predicting diurnal cortisol, γ=0.0003, p=.037, 95% CI [0.00002,0.001] (see Figure 1, left panel). Simple slopes analyses revealed that while more integrated individuals showed steeper cortisol slopes than less integrated individuals, those who were one standard deviation above the means for both age and social integration had the steepest diurnal cortisol slopes, b=−0.061, p<.001, 95% CI [−0.069, −0.053], whereas individuals who were one standard deviation below the means for both age and social integration had the least steep cortisol slopes, b=−0.014, p=.011, 95% CI [−0.024, −0.003].
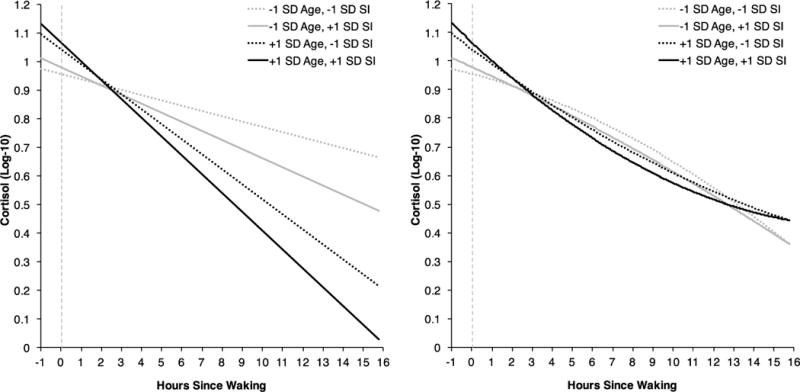
Multilevel model results predicting diurnal cortisol slopes as a function of the interaction between age, social integration (SI), and the linear term for time (left panel) or the quadratic term for time (right panel), adjusted for the control variables. The intercept value of 0 (vertical dashed line) represents the cortisol sample taken at 1-hour post waking.
There was also a significant three-way interaction between age, social integration, and the quadratic term for time in predicting diurnal cortisol, γ=−0.00002, p=.031, 95% CI [−0.00004, −0.000001] (see Figure 1, right panel). Simple slopes analyses revealed that individuals who were one standard deviation above the means for both age and social integration had the fastest acceleration in diurnal cortisol decline, translating to lower exposure to cortisol during the afternoon and evening in particular, b=0.001, p<.001, 95% CI [0.001,0.002]. Conversely, individuals who were one standard deviation below the means for both age and social integration showed evidence of deceleration in diurnal cortisol decline, translating to higher exposure to cortisol during the afternoon and evening in particular, b=−0.002, p<.001, 95% CI [−0.002, −0.001]. Model results are presented in Table 3.
Table 3
Multilevel model results for predicting cortisol slopes as a function of the interaction between social integration and age
Fixed Effects | |||
---|---|---|---|
Parameter | γ | p-value | 95% Confidence Interval |
Intercept | 1.011 | < .001 | [0.993, 1.028] |
Sex | −0.037 | .008 | [–0.064, −0.010] |
Black | –0.030 | .078 | [–0.064, 0.003] |
Other Race | –0.067 | .025 | [–0.126, −0.008] |
Education | –0.001 | .759 | [–0.009, 0.007] |
Body Mass Index | –0.001 | .393 | [–0.003, 0.001] |
Winter | –0.003 | .886 | [–0.040, 0.034] |
Summer | 0.045 | .029 | [0.005, 0.085] |
Fall | 0.015 | .468 | [–0.025, 0.054] |
Pittsburgh Cold Study 2 | 0.164 | < .001 | [0.132, 0.195] |
Pittsburgh Mind Body Center Study | –0.157 | < .001 | [–0.206, −0.107] |
Age | 0.004 | < .001 | [0.002, 0.006] |
Social Integration | 0.007 | .182 | [–0.003, 0.018] |
Social Integration × Age | –0.002 | .002 | [–0.002, −0.001] |
Time | –0.042 | < .001 | [–0.047, −0.037] |
Time × Age | –0.002 | < .001 | [–0.002, −0.001] |
Time × Social Integration | –0.004 | .009 | [–0.007, −0.001] |
Time × Social Integration × Age | < .001 | .037 | [0.000, 0.001] |
Time2 | < .001 | .162 | [0.000, 0.001] |
Time2 × Age | < .001 | < .001 | [0.000, 0.000] |
Time2 × Social Integration | < .001 | .019 | [0.000, 0.000] |
Time2 × Social Integration × Age | < .001 | .032 | [0.000, 0.000] |
| |||
Random Effects | |||
| |||
Parameter | Variance | χ2(df) | p-value |
Level-1/Level-2 | |||
![]() | 0.039 | 2722.59(1343) | < .001 |
![]() | 0.002 | 1767.42(1343) | < .001 |
![]() | 0.000 | 1754.52(1343) | < .001 |
![]() | 0.079 | ||
Level-3 | |||
![]() | 0.166 | 1405.71(666) | < .001 |
![]() | 0.042 | 1223.37(676) | < .001 |
![]() | 0.002 | 959.46(676) | < .001 |
There was no three-way interaction between age, social contact frequency, and the linear term for time, γ=−0.0001, p=.601, or the quadratic term for time, γ<.0001, p=.987, in predicting diurnal cortisol slopes.
3.3 Mediation analyses
In the PROCESS macro for SPSS, 50,000 bootstrapped samples were used to test proposed mediators of the association of social integration with average cortisol AUC for older individuals in our sample. Models used all standard covariates except for those testing for a moderated mediating effect of health behaviors, which used all standard covariates except for BMI which was likely to be confounded with these behaviors. One participant was missing drinking data (n=1) and was excluded from that moderated mediation analysis; two participants were missing exercise data (n=2) and were excluded from that analysis. In separate moderated mediation models, none of the proposed variables (psychological stress, b=.0001, 95% CI [−.0012,.0008], trait positive affect, b=.0004, 95% CI [−.0003,.0015], trait negative affect, b<.0001, 95% CI [−.0006,.0006], smoking, b=−.0008, 95% CI [−.0025,.0002], drinking, b=−.0010, 95% CI [−.0027, .0000], or exercise, b=−.0002, 95% CI [−.0018,.0006]), mediated the association between social integration and cortisol AUC for individuals 1 SD above the mean age (42.2 years).
In separate regular multiple linear regression models, there was a Social Integration × Age interaction in predicting cigarettes smoked per day, β=.077, SE=.017, p=.036, but not perceived stress, positive affect, negative affect, number of drinks per week, or minutes of exercise per week, (all ps>.39). In the multilevel models predicting cortisol, we found no evidence that either the Social Integration × Age × Time or the Social Integration × Age × Time-Squared interactions were at all attenuated when additionally adjusting the model for any proposed mediator. As such, there was no evidence that any of the proposed mediators mediated the association between Social Integration × Age and diurnal cortisol slopes.
3.4 Controlling for marital status
Because an earlier analysis of this data found an association of cortisol AUC and diurnal slopes with marital status (Chin et al., 2017), we conducted secondary analyses including marital status as a covariate in order to demonstrate that the association between social integration and cortisol were not driven only by marital status. In addition, these analyses also controlled for the interaction between marital status and age. Controlling for both marital status and the interaction between marital status and age did not meaningfully affect the Social Integration × Age interaction on average cortisol AUC, β=−.084, SE<.001, p=.012, the three-way interaction between age, social integration, and the linear term for time in predicting diurnal cortisol, γ=0.0003, p=.037, 95% CI [0.00002,0.001], or the three-way interaction between age, social integration, and the quadratic term for time in predicting diurnal cortisol, γ=−0.00002, p=.032, 95% CI [−0.00004, −0.000001].
4. Discussion
Previous research has demonstrated that increased social integration is associated with lower rates of morbidity (e.g., Barefoot et al., 2005; Kroenke et al., 2012; Pinquart & Duberstein, 2010; Seeman, 1996) and mortality (Holt-Lunstad et al., 2010; Shor & Roelfs, 2015). However, the biological mechanisms through which these associations occur remain understudied. One possibility is that social integration might influence health outcomes by altering the function of the HPA axis. Those lacking social integration may experience psychological stress which in turn induces HPA axis dysregulation. Alternatively, social integration may improve health by increasing positive affect, decreasing negative affect, or promoting better health practices, all of which aid in the regulation of the HPA response. Here we tested whether social integration, measured as the number of social roles in which an individual reports actively participating, was associated with differences in diurnal cortisol AUC and slopes. We found that greater social integration was associated with lower cortisol AUC in older (ages 35–55) but not younger (ages 18–34) individuals in our sample. Additionally, while social integration predicted steeper diurnal slopes regardless of age, the association was strongest among older individuals in our sample. However, the association between greater social integration and lower daily cortisol levels among older adults was not mediated by differences in positive affect, negative affect, psychological stress, or health behaviors.
While the majority of social integration and health research has focused on individuals older than those in our study (ages 55 and above), this study provides some of the first evidence that social integration is associated with biological markers with implications for long-term health in a mid-life sample (mid 30s to 55 years). Previous work by Yang et al. (2016) found that lower levels of social integration were associated with greater inflammation and increased hypertensive risk among older adults (two samples ages 50–98 and 57–91), but not among younger adults (ages 24–32) or adolescents (ages 12–18). Consistent with these earlier findings, our results demonstrate that lower levels of social integration are associated with elevated daily cortisol AUC among older but not younger adults. Additionally, while lower levels of social integration were associated with less steep diurnal decline in cortisol regardless of age, this association was strongest among older compared to younger individuals in our sample.
Although the primary aim of this study was to examine the association whether age moderated the association between social integration and diurnal cortisol, we were also able to test the main effect of age among individuals aged 18–55. Greater age was associated with steeper diurnal cortisol declines but not AUC. Although previous research suggests that older age is associated with increased cortisol AUC and flatter diurnal slopes (review by Gaffey et al., 2016), all studies examining these associations included individuals considerably older than those in the current sample (ages 18–55).
There are several possible explanations for why higher levels of social integration were more strongly associated with cortisol outcomes among older compared to younger individuals in our sample. One possibility is that the social motivations and social contexts of younger individuals are likely to differ substantially from those of older individuals (Carstensen, 1992). Younger individuals may possess more superficial connections that do not confer the same health benefits as the more meaningful connections held by older individuals. Alternatively, it is possible that we did not find stronger associations between social integration and cortisol outcomes for younger individuals because our assessments failed to measure some of the social roles that are meaningful for this group. For example, a more effective assessment of social integration in college-aged populations may need to ask about roles more relevant to these individuals such as sorority/fraternity membership, casual dating partners, or online contacts. Although differences in health behaviors did not mediate the association between social integration and lower cortisol AUC in older individuals, it is possible that we did not find evidence for mediation because health behaviors were only assessed on a single occasion that did not coincide with the specific days of cortisol collection. Another possibility still is that the effects of social integration on health accumulate over time and less socially integrated younger individuals will eventually show the elevations in cortisol seen in their older less-integrated counterparts. These results suggest the importance of taking into account the context of aging when examining the effects of social interactions on health.
One strength of the current study is how social interactions were measured. Here we disaggregated measurement of two related but distinct concepts: social integration and social contact frequency. This allowed us to examine the unique associations of both constructs with diurnal cortisol. Consistent with research on social factors predicting mortality (e.g., Holt-Lunstad et al., 2010; Shor & Roelfs, 2015), we found that social integration was the dominant predictor of cortisol AUC and diurnal slopes in those in their mid 30s and older, with a greater effect size than social contact frequency. While greater social contact frequency was also associated with lower cortisol AUC in older individuals, this effect did not persist when controlling for total number of social roles. This suggests that the degree to which individuals have diverse social connections is more important than the actual frequency of interactions. This study highlights the need for future research to carefully consider the ways in which social interaction variables are operationalized and measured.
There are several limitations to the current study that warrant consideration. First, the cross-sectional design of this study prevents us from drawing causal inference about the direction of the association between social integration and cortisol. However it seems unlikely that differences in total cortisol AUC or diurnal slopes influence levels of social integration, especially in a sample selected for being in good health. Although we controlled for a large number of potential confounding factors, it is also possible that an unmeasured third variable may account for the association between social integration and cortisol for older individuals in our sample. It is also possible that the use of three days of cortisol collection was insufficient to optimize the reliability of cortisol measures (Segerstrom et al., 2014 but see dissenting opinions by Kraemer et al., 2006; Saxbe, 2008). If so, we may have underestimated the effect sizes in our study. Finally, an implicit assumption of this study is that cortisol may mediate the association between social integration and health outcomes. However, total social roles were unrelated to risk of objectively catching a cold in analyses not reported here, and we were therefore unable to test cortisol as a mediator of the association between total social roles and disease.
Acknowledgments
Preparation of this paper was supported by a grant from the National Center for Complementary and Integrative Health (AT006694); the conduct of the studies was supported by grants from the National Institute of Mental Health (MH50429), National Heart, Lung, and Blood Institute (HL65111; HL65112), and National Institute for Allergy and Infectious Diseases (R01 AI066367); and secondary support was provided by a grant from the National Institutes of Health to the University of Pittsburgh Medical Center General Clinical Research Center (NCRR/GCRC 5M01 RR00056) and from the National Institutes of Health to the University of Pittsburgh Clinical and Translational Science Institute (UL1 RR024153 and UL1 RT000005); and supplemental support was provided by John D. and Catherine T. MacArthur Foundation Research Network on Socioeconomic Status & Health. We thank David Creswell and Brooke Feeney for their feedback on an earlier version of this manuscript.
Role of the funding source
The funding sources had no further role in the writing of this article.
Footnotes
Publisher's Disclaimer: This is a PDF file of an unedited manuscript that has been accepted for publication. As a service to our customers we are providing this early version of the manuscript. The manuscript will undergo copyediting, typesetting, and review of the resulting proof before it is published in its final citable form. Please note that during the production process errors may be discovered which could affect the content, and all legal disclaimers that apply to the journal pertain.
Conflict of interest statement
All authors declare there are no conflicts of interest.
References
- Adam EK, Quinn ME, Tavernier R, McQuillan MT, Dahlke KA, Gilbert KE. Diurnal cortisol slopes and mental and physical health outcomes: A systematic review and meta-analysis. Psychoneuroendocrinology. 2017;83:25–41. [Europe PMC free article] [Abstract] [Google Scholar]
- Anagnostis P, Athyros VG, Tziomalos K, Karagiannis A, Mikhailidis DP. The pathogenetic role of cortisol in the metabolic syndrome: a hypothesis. J Clin Endocrinol Metab. 2009;94(8):2692–2701. [Abstract] [Google Scholar]
- Badrick E, Bobak M, Britton A, Kirschbaum C, Marmot M, Kumari M. The relationship between alcohol consumption and cortisol secretion in an aging cohort. J Clin Endocrinol Metab. 2008;93(3):750–757. [Europe PMC free article] [Abstract] [Google Scholar]
- Badrick E, Kirschbaum C, Kumari M. The relationship between smoking status and cortisol secretion. J Clin Endocrinol Metab. 2007;92(3):819–824. [Abstract] [Google Scholar]
- Barefoot JC, Grønbæk M, Jensen G, Schnohr P, Prescott E. Social network diversity and risks of ischemic heart disease and total mortality: findings from the Copenhagen City Heart Study. Am J Epidemiol. 2005;161(10):960–967. [Abstract] [Google Scholar]
- Berkman LF, Breslow L. Health and ways of living: The Alameda County study. New York: Oxford University Press; 1983. [Google Scholar]
- Berkman LF, Glass T. Social integration, social networks, social support, and health. In: Berkman LF, Kawachi I, editors. Social epidemiology. New York: Oxford University Press; 2000. pp. 137–173. [Google Scholar]
- Berkman LF, Glass T, Brissette I, Seeman TE. From social integration to health: Durkheim in the new millennium. Soc Sci Med. 2000;51(6):843–857. [Abstract] [Google Scholar]
- Berkman LF, Melchior M, Chastang JF, Niedhammer I, Leclerc A, Goldberg M. Social integration and mortality: a prospective study of French employees of Electricity of France–Gas of France: the GAZEL Cohort. Am J Epidemiol. 2004;159(2):167–174. [Abstract] [Google Scholar]
- Brissette I, Cohen S, Seeman TE. Measuring social integration and social networks. In: Cohen S, Underwood L, Gottlieb B, editors. Support measurements and interventions: A guide for social and health scientists. New York: Oxford University Press; 2000. [Google Scholar]
- Brunner EJ, Hemingway H, Walker BR, Page M, Clarke P, Juneja M, Papadopoulos A. Adrenocortical, autonomic, and inflammatory causes of the metabolic syndrome nested case-control study. Circulation. 2002;106(21):2659–2665. [Abstract] [Google Scholar]
- Bryk AS, Raudenbush SW. Hierarchical linear models: Applications and data analysis methods. 2nd. Thousand Oaks, CA: Sage Publications, Inc; 2002. [Google Scholar]
- Carstensen LL. Social and emotional patterns in adulthood: support for socioemotional selectivity theory. Psychol Aging. 1992;7(3):331–338. [Abstract] [Google Scholar]
- Champaneri S, Xu X, Carnethon MR, Bertoni AG, Seeman T, DeSantis AS, Golden SH. Diurnal salivary cortisol is associated with body mass index and waist circumference: the Multiethnic Study of Atherosclerosis. Obesity. 2013;21(1):E56–E63. [Europe PMC free article] [Abstract] [Google Scholar]
- Chang SC, Glymour M, Cornelis M, Walter S, Rimm EB, Tchetgen ET, Kubzansky LD. Social Integration and Reduced Risk of Coronary Heart Disease in Women. Circ Res. 2017;120(12):1927–1937. [Europe PMC free article] [Abstract] [Google Scholar]
- Cheng ST, Leung EM, Chan TWS. Physical and social activities mediate the associations between social network types and ventilatory function in Chinese older adults. Health Psychol. 2014;33(6):524–534. [Abstract] [Google Scholar]
- Chin B, Murphy ML, Janicki-Deverts D, Cohen S. Marital status as a predictor of diurnal salivary cortisol levels and slopes in a community sample of healthy adults. Psychoneuroendocrinology. 2017;78:68–75. [Europe PMC free article] [Abstract] [Google Scholar]
- Cohen J, Cohen P, West SG, Aiken LS. Applied multiple regression/correlation analysis for the behavioral sciences. 3rd. Mahwah, NJ: Erlbaum; 2013. [Google Scholar]
- Cohen S. Psychosocial models of the role of social support in the etiology of physical disease. Health Psychol. 1988;7(3):269–297. [Abstract] [Google Scholar]
- Cohen S. Social relationships and health. Am Psychol. 2004;59(8):676–684. [Abstract] [Google Scholar]
- Cohen S, Doyle WJ, Skoner DP, Rabin BS, Gwaltney JM. Social ties and susceptibility to the common cold. JAMA. 1997;277(24):1940–1944. [Abstract] [Google Scholar]
- Cohen S, Doyle WJ, Turner RB, Alper CM, Skoner DP. Emotional style and susceptibility to the common cold. Psychosom Med. 2003;65(4):652–657. [Abstract] [Google Scholar]
- Cohen S, Kessler R, Gordon L. Strategies for measuring stress in studies of psychiatric and physical disorders. In: Cohen S, Kessler R, Gordon L, editors. Measuring Stress. New York, NY: Oxford University Press; 1995. pp. 3–26. [Google Scholar]
- Cohen S, Lemay EP. Why would social networks be linked to affect and health practices? Health Psychol. 2007;26(4):410–417. [Abstract] [Google Scholar]
- Cohen S, Schwartz JE, Epel E, Kirschbaum C, Sidney S, Seeman T. Socioeconomic status, race, and diurnal cortisol decline in the Coronary Artery Risk Development in Young Adults (CARDIA) Study. Psychosom Med. 2006;68(1):41–50. [Abstract] [Google Scholar]
- Cohen S, Williamson G. Perceived stress in a probability sample of the US. In: Spacapam S, Oskamp S, editors. The social psychology of health: Claremont symposium on applied social psychology. Newbury Park, CA: Sage; 1988. pp. 31–67. [Google Scholar]
- Dekker MJHJ, Koper JW, Van Aken MO, Pols HAP, Hofman A, de Jong FH, Tiemeier H. Salivary cortisol is related to atherosclerosis of carotid arteries. J Clin Endocrinol Metab. 2008;93(10):3741–3747. [Abstract] [Google Scholar]
- Dressendörfer RA, Kirschbaum C, Rohde W, Stahl F, Strasburger CJ. Synthesis of a cortisol-biotin conjugate and evaluation as a tracer in an immunoassay for salivary cortisol measurement. J Steroid Biochem Mol Biol. 1992;43(7):683–692. [Abstract] [Google Scholar]
- Enders CK, Tofighi D. Centering predictor variables in cross-sectional multilevel models: A new look at an old issue. Psychological Methods. 2007;12:121–138. 10.1037/1082-989X.12.2.121. [Abstract] [CrossRef] [Google Scholar]
- Gaffey AE, Bergeman CS, Clark LA, Wirth MM. Aging and the HPA axis: Stress and resilience in older adults. Neuroscience & Biobehavioral Reviews. 2016;68:928–945. [Europe PMC free article] [Abstract] [Google Scholar]
- Grant N, Hamer M, Steptoe A. Social isolation and stress-related cardiovascular, lipid, and cortisol responses. Ann Behav Med. 2009;37(1):29–37. [Abstract] [Google Scholar]
- Hayes AF. Introduction to mediation, moderation, and conditional process analysis: A regression-based approach. New York, NY: Guilford Press; 2013. [Google Scholar]
- Holt-Lunstad J, Smith TB, Layton JB. Social relationships and mortality risk: a meta-analytic review. PLoS Med. 2010;7(7):e1000316. [Europe PMC free article] [Abstract] [Google Scholar]
- Hox JJ. Multilevel Analysis: Techniques and Applications. 2nd. New York, NY: Routledge; 2010. [Google Scholar]
- Janicki-Deverts D, Cohen S, Turner RB, Doyle WJ. Basal salivary cortisol secretion and susceptibility to upper respiratory infection. Brain Behav Immun. 2016;53:255–261. [Europe PMC free article] [Abstract] [Google Scholar]
- Kraemer HC, Giese-Davis J, Yutsis M, O’Hara R, Neri E, Gallagher-Thompson D, Spiegel D. Design decisions to optimize reliability of daytime cortisol slopes in an older population. Am J Geriatr Psychiatry. 2006;14(4):325–333. [Abstract] [Google Scholar]
- Kroenke CH, Michael Y, Tindle H, Gage E, Chlebowski R, Garcia L, Caan BJ. Social networks, social support and burden in relationships, and mortality after breast cancer diagnosis. Breast Cancer Res Treat. 2012;133(1):375–385. [Europe PMC free article] [Abstract] [Google Scholar]
- Pinquart M, Duberstein PR. Associations of social networks with cancer mortality: a meta-analysis. Crit Rev Oncol Hematol. 2010;75(2):122–137. [Europe PMC free article] [Abstract] [Google Scholar]
- Polk DE, Cohen S, Doyle WJ, Skoner DP, Kirschbaum C. State and trait affect as predictors of salivary cortisol in healthy adults. Psychoneuroendocrinology. 2005;30(3):261–272. [Abstract] [Google Scholar]
- Pruessner JC, Kirschbaum C, Meinlschmid G, Hellhammer DH. Two formulas for computation of the area under the curve represent measures of total hormone concentration versus time-dependent change. Psychoneuroendocrinology. 2003;28(7):916–931. [Abstract] [Google Scholar]
- Raudenbush SW, Bryk AS, Congdon R. HLM 6 for Windows [Computer software] Lincolnwood, IL: Scientific Software International; 2004. [Google Scholar]
- Reis HT, Wheeler L. Studying social interaction with the Rochester Interaction Record. Adv Exp Soc Psychol. 1991;24:269–318. [Google Scholar]
- Sapolsky RM, Romero LM, Munck AU. How do glucocorticoids influence stress responses? Integrating permissive, suppressive, stimulatory, and preparative actions. Endocr Rev. 2000;21(1):55–89. [Abstract] [Google Scholar]
- Saxbe DE. A field (researcher’s) guide to cortisol: tracking HPA axis functioning in everyday life. Health Psychol Rev. 2008;2(2):163–190. [Google Scholar]
- Seeman TE. Social ties and health: The benefits of social integration. Ann Epidemiol. 1996;6(5):442–451. [Abstract] [Google Scholar]
- Seeman TE, Singer BH, Ryff CD, Love GD, Levy-Storms L. Social relationships, gender, and allostatic load across two age cohorts. Psychosom Med. 2002;64(3):395–406. [Abstract] [Google Scholar]
- Segerstrom SC, Boggero IA, Smith GT, Sephton SE. Variability and reliability of diurnal cortisol in younger and older adults: implications for design decisions. Psychoneuroendocrinology. 2014;49:299–309. [Europe PMC free article] [Abstract] [Google Scholar]
- Shankar A, McMunn A, Banks J, Steptoe A. Loneliness, social isolation, and behavioral and biological health indicators in older adults. Health Psychol. 2011;30(4):377. [Abstract] [Google Scholar]
- Shor E, Roelfs DJ. Social contact frequency and all-cause mortality: A meta-analysis and meta-regression. Soc Sci Med. 2015;128:76–86. [Abstract] [Google Scholar]
- Umberson D. Family status and health behaviors: Social control as a dimension of social integration. J Health Soc Behav. 1987:306–319. [Abstract] [Google Scholar]
- Usala PD, Hertzog C. Measurement of affective states in adults: Evaluation of an adjective rating scale instrument. Res Aging. 1989;11(4):403–426. [Abstract] [Google Scholar]
- Van Cauter E, Leproult R, Kupfer DJ. Effects of gender and age on the levels and circadian rhythmicity of plasma cortisol. J Clin Endocrinol Metab. 1996;81(7):2468–2473. [Abstract] [Google Scholar]
- Yang YC, Boen C, Gerken K, Li T, Schorpp K, Harris KM. Social relationships and physiological determinants of longevity across the human life span. Proc Natl Acad Sci USA. 2016;113(3):578–583. [Europe PMC free article] [Abstract] [Google Scholar]
Full text links
Read article at publisher's site: https://doi.org/10.1016/j.psyneuen.2018.02.008
Read article for free, from open access legal sources, via Unpaywall:
http://manuscript.elsevier.com/S0306453017313653/pdf/S0306453017313653.pdf
Citations & impact
Impact metrics
Citations of article over time
Alternative metrics
Smart citations by scite.ai
Explore citation contexts and check if this article has been
supported or disputed.
https://scite.ai/reports/10.1016/j.psyneuen.2018.02.008
Article citations
Stay social, stay young: a bioanthropological outlook on the processes linking sociality and ageing.
Geroscience, 11 Nov 2024
Cited by: 0 articles | PMID: 39527178
Review
The biology of aging in a social world: Insights from free-ranging rhesus macaques.
Neurosci Biobehav Rev, 154:105424, 11 Oct 2023
Cited by: 7 articles | PMID: 37827475 | PMCID: PMC10872885
Review Free full text in Europe PMC
Demographic factors, partial social belonging and psychological resources associated with coping.
Front Psychol, 14:1154659, 03 Apr 2023
Cited by: 0 articles | PMID: 37077847 | PMCID: PMC10106560
Perceived partial social integration, levels of distress and resilience, and COVID-19 vaccine rejection of Jewish and Arab citizens of Israel.
Front Public Health, 10:1021015, 22 Nov 2022
Cited by: 1 article | PMID: 36483251 | PMCID: PMC9723345
Coping strategies moderate the effect of perceived stress on sleep and health in older adults during the COVID-19 pandemic.
Stress Health, 38(4):708-721, 13 Jan 2022
Cited by: 5 articles | PMID: 34951930 | PMCID: PMC10124294
Go to all (7) article citations
Data
Data behind the article
This data has been text mined from the article, or deposited into data resources.
BioStudies: supplemental material and supporting data
Similar Articles
To arrive at the top five similar articles we use a word-weighted algorithm to compare words from the Title and Abstract of each citation.
Marital status as a predictor of diurnal salivary cortisol levels and slopes in a community sample of healthy adults.
Psychoneuroendocrinology, 78:68-75, 19 Jan 2017
Cited by: 31 articles | PMID: 28171850 | PMCID: PMC5365082
Chronic stress exposure, diurnal cortisol slope, and implications for mood and fatigue: Moderation by multilocus HPA-Axis genetic variation.
Psychoneuroendocrinology, 100:156-163, 06 Oct 2018
Cited by: 25 articles | PMID: 30340064
Diurnal cortisol rhythms in Tsimane' Amazonian foragers: new insights into ecological HPA axis research.
Psychoneuroendocrinology, 37(2):178-190, 29 Jun 2011
Cited by: 17 articles | PMID: 21719201
Diurnal cortisol slopes and mental and physical health outcomes: A systematic review and meta-analysis.
Psychoneuroendocrinology, 83:25-41, 24 May 2017
Cited by: 321 articles | PMID: 28578301 | PMCID: PMC5568897
Review Free full text in Europe PMC
Funding
Funders who supported this work.
NCATS NIH HHS (1)
Grant ID: UL1 TR000005
NCCIH NIH HHS (1)
Grant ID: R01 AT006694
NCRR NIH HHS (2)
Grant ID: M01 RR000056
Grant ID: UL1 RR024153
NHLBI NIH HHS (2)
Grant ID: P50 HL065112
Grant ID: P50 HL065111
NIAID NIH HHS (1)
Grant ID: R01 AI066367
National Center for Complementary and Integrative Health (1)
Grant ID: AT006694
National Heart, Lung, and Blood Institute (2)
Grant ID: HL65112HL65111
Grant ID: HL65111
National Institute for Allergy and Infectious Diseases (1)
Grant ID: R01 AI066367
National Institute of Mental Health (1)
Grant ID: MH50429
National Institutes of Health to the University of Pittsburgh Clinical and Translational Science Institute (2)
Grant ID: UL1 RR024153
Grant ID: UL1 RT000005
National Institutes of Health to the University of Pittsburgh Medical Center General Clinical Research Center (1)
Grant ID: NCRR/GCRC 5M01 RR00056