Abstract
Free full text

Functional Genomic Landscape of Acute Myeloid Leukemia
Abstract
The implementation of targeted therapies for acute myeloid leukemia has been challenged by complex mutational patterns within and across patients as well as a dearth of pharmacologic agents for most mutational events. Here, we report initial findings from the Beat AML program on a cohort of 672 tumor specimens collected from 562 patients. We assessed these specimens using whole exome sequencing, RNA-sequencing, and ex vivo drug sensitivity analyses. Our data reveal novel mutational events not previously detected in AML. We show association of drug response with mutational status, including instances of drug sensitivity that are specific to combinatorial mutational events. Integration with RNA-sequencing also revealed gene expression signatures, which predict a role of specific gene networks in drug response. Collectively, this report offers a dataset, accessible by the Beat AML data viewer (www.vizome.org), that can be leveraged to address clinical, genomic, transcriptomic, and functional inquiries into the biology of AML.
INTRODUCTION
Approximately 21,000 people are diagnosed with acute myeloid leukemia (AML) with over 10,000 AML-related deaths annually in the United States1,2. Cytogenetic and sequencing analyses have revealed at least 11 genetic classes of AML3 and over 20 subsets can be assigned when also considering cell differentiation states of the leukemic blasts4,5. Deep sequencing of AML by The Cancer Genome Atlas (TCGA) revealed a heterogeneous disease with nearly 2,000 somatically mutated genes observed across 200 patients 6. Many of the recurrent cytogenetic events and somatic mutations have been shown to carry prognostic significance3,7,8. Some of the most frequent somatic variants can also be observed in myelodysplastic syndromes (MDS) and myeloproliferative neoplasms (MPN)9–11 that can transform into AML. These same mutations have also been observed in healthy individuals exhibiting age-related clonal hematopoiesis, which carries significant risk for the development of MDS, MPN, and AML12–15.
A small number of therapies targeted to mutational events have been developed for AML patients, with the current standard of care largely unchanged over the past 30–40 years. The first targeted therapy for AML involved use of all-trans retinoic acid (ATRA) in combination with arsenic trioxide for patients with rearrangement of the retinoic acid receptor16,17. More recently, fms related tyrosine kinase 3 (FLT3) inhibitors have been developed for FLT3 mutational events that occur in ~20–30% of AML patients18–21. FLT3 inhibitors deployed as single agents yielded responses of only 2–6 months22–25. Midostaurin, a broad-spectrum FLT3 inhibitor, was recently approved for use in newly diagnosed, FLT3-mutated AML patients in combination with standard of care chemotherapy 26; however, relapse was still prevalent in this setting. Targeting of mutant isocitrate dehydrogenase (NADP(+)) 1, cytosolic 1/2 (IDH1/2)27, has shown clinical benefit leading to approval of the IDH2 inhibitor, enasidenib, and the IDH1 inhibitor, ivosidenib28,29. Additional proposed strategies have included inhibition of epigenetic modifiers such as enhancer of zeste 2 polycomb repressive complex 2 subunit (EZH2)30, LSD1 zinc finger family protein (LSD1)31, and DOT1L like histone lysine methyltransferase (DOT1L)32 based on direct mutation of these targets or synthetic lethality in the context of drug combinations (ATRA and LSD1 inhibitors) or specific genetic features (lysine methyltransferase 2A (KMT2A)-gene rearrangement for DOT1L inhibitors). Hypomethylating agents have been employed in AML patients with better responses reported for certain genetic subsets, such as those with mutation of TET233 or tumor protein 53 (TP53)34. Most recently, the BCL2 apoptosis regulator (BCL2) inhibitor, venetoclax, showed a ~20% response rate when used as a single agent in relapsed patients35 with higher response rates (~60%) reported in combination with hypomethylating agents in newly diagnosed, elderly AML patients36.
RESULTS
To better understand genetic or transcriptional markers and mechanisms of drug sensitivity and resistance in AML, we developed a cohort of 672 primary specimens from 562 unique AML patients on which extensive functional and genomic interrogations were undertaken. Detailed clinical annotations, including diagnostic information, clinical laboratory values, treatments, responses and outcomes were curated from electronic medical records and are reported in Supplementary Information (Tables S1–5).
Comparative Genomic Landscape of AML
We performed exome sequencing on 622 of the specimens from the cohort representing 531 unique patients. The final, high-confidence variant list (Supplementary Information, Table S7) revealed a range of 1–80 somatic variants per patient (cohort median of 13 somatic variants) (Extended Data Fig. 1). Comparison of the top 33 most commonly mutated genes across Beat AML and TCGA6 showed generally similar frequencies. Higher frequency of mutations in serine and arginine rich splicing factor 2 (SRSF2) were seen in Beat AML than in TCGA, and this difference was conserved comparing only the de novo cases in Beat AML with TCGA (Fig. 1A). In contrast, mutational events that were seen at less than 2% frequency across Beat AML and TCGA were much more divergent with variants in 221 mutant genes called in common, 939 mutant genes called only in TCGA, and ~1,500 mutant genes called only in Beat AML, irrespective of whether we compared only de novo or non-de novo cases (Fig. 1B,,C).C). Most of these divergent mutational events were observed in only single patients, however, there were mutations in 11 genes that were called in 1% or more of patients in Beat AML, but were not observed in prior AML sequencing studies (Extended Data Fig. 1). Finally, co-occurrence and exclusivity of the most frequent variants were computed revealing significant patterns of mutational co-segregation suggesting biological cooperativity of certain mutational events (Fig. 1D).
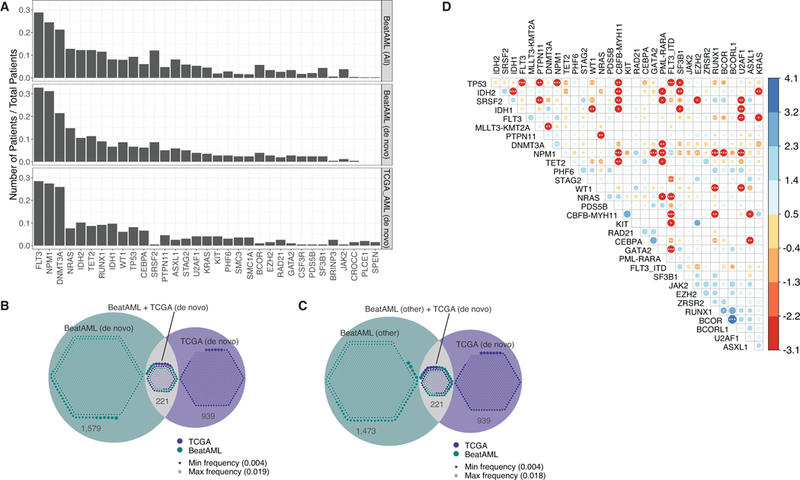
(A) Frequency of the 33 mutational events that were cumulatively most frequent in Beat AML (n=531 patients) and TCGA (n=200 patients). Top row represents the full Beat AML cohort and the middle bar represents only the de novo Beat AML cases. Mutations were summarized by gene as was done by TCGA whereas the FLT3-ITD mutations were kept separate in the rest of this manuscript.
(B) Mutational events at 2% frequency or less in the de novo cases of Beat AML and TCGA were compared for overlap. Venn diagram displays the overlap with the small circles within each compartment representing a size-scaled frequency of each mutational event.
(C) Analysis as in panel B with only the non-de novo Beat AML cases versus TCGA.
(D) Co-occurrence or exclusivity of the most recurrent mutational events in the Beat AML cohort (n=531 patients) were assessed using the DISCOVER40 method with a dot plot indicating the odds ratio of co-occurrence (blue) or exclusivity (red) using color-coding and circle size as well as asterisks that indicate FDR-corrected statistical significance.
Drug Response and Gene Expression
RNA sequencing was performed on 451 specimens from 411 patients. Clustering of the 2,000 most variably expressed genes across the cohort revealed gene expression signatures that associated with many of the prominent genetic and cytogenetic disease groups (Extended Data Fig. 2). To understand the profile of sensitivity and resistance to a variety of small-molecule inhibitors, we profiled primary tumor cells from 409 specimens derived from 363 patients against a panel of 122 small-molecule inhibitors using an ex vivo drug sensitivity assay37. Drug sensitivity patterns were analyzed with respect to clinical and genetic features of tumors (Extended Data Fig. 3). We compared the average area-under-the curve (AUC) values for each drug between the de novo and transformed samples via a series of single factor analysis of variance (ANOVA) tests. Generally, transformed cases showed less sensitivity to most drugs than de novo cases. Of the 122 drugs tested, 64 were significantly (false discovery rate (FDR) < .1) more sensitive in the de novo samples, while only 1 drug Panobinostat (HDAC inhibitor) was significantly more sensitive in the transformed cases (Extended Data Fig. 4). In addition, we analyzed the concordance of drug sensitivity patterns with respect to predicted target gene, gene family, or pathway for each drug (drug assignments to target families found in Supplementary Information, Table S11). This analysis revealed drug targets/families that were highly concordant amongst constituent members as well as drug families that were quite discordant (Extended Data Fig. 5). To create a global view of overall sensitivity or resistance of each case, we generated a heatmap of binary sensitive/resistant calls for each sample to each drug. We then annotated the sensitivity or resistance fraction of each case against European Leukemia Net (ELN) 2017 (Extended Data Fig. 6A) and World Health Organization (WHO) 2016 (Extended Data Fig. 6B) classifications.
Gene Signatures of Drug Response
We performed a cohort analysis to assess correlation between drug sensitivity patterns and mutational events or gene expression levels. Correlation of drug sensitivity with mutational events was performed by assessing the range of sensitivity of cases with mutation of an individual gene (as well as co-occurring mutational events) versus cases with wild type sequence for that same gene. Broad summaries of full cohort results are displayed in Circos and Manhattan plots (Extended Data Fig. 7A, ,8).8). Individual drug/mutation associations are displayed on a Volcano plot with the horizontal axis showing difference in drug sensitivity between mutant versus wild type and FDR-corrected significance values38 plotted on the vertical axis (Fig. 2A). Some of the associations showing highest levels of statistical significance involved FLT3-ITD exhibiting sensitivity to FLT3 pathway inhibitors, which served as a proof-of-principle since FLT3 inhibitors are known to be more effective against FLT3-ITD AML. However, to reveal drug/mutation associations that were not biased by co-occurrence with FLT3-ITD, we also plotted the same analysis using only cases that were wild type for FLT3 (Extended Data Fig. 7B). We also created Volcano plots that were specific to each individual drug (versus all mutational events) and for each individual gene (versus all drugs tested). All Volcano plots can be found with interactive features in our online data browser (www.vizome.org) and (http://vizome.org/additional_figures_BeatAML.html).
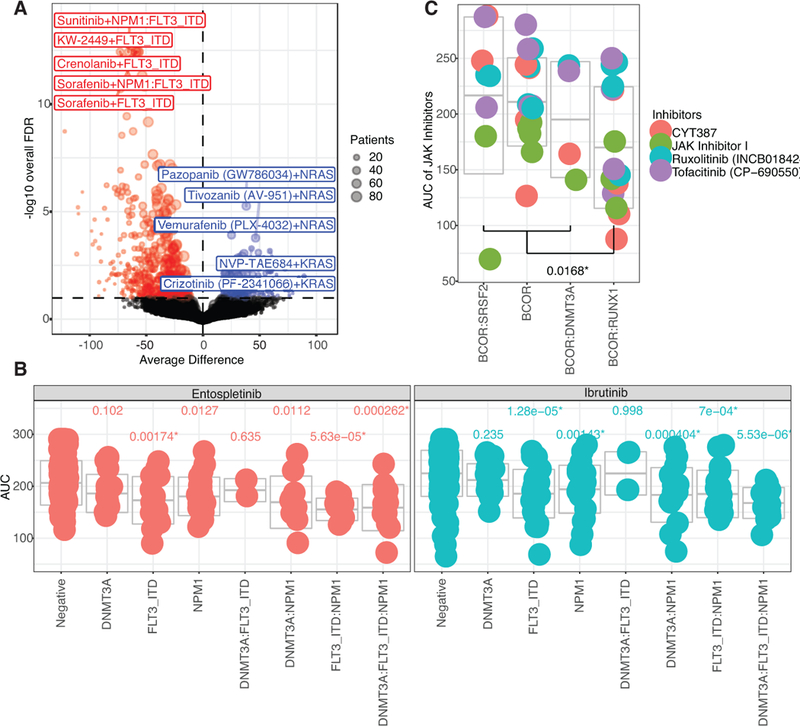
(A) Average difference in AUC drug response between mutant and wild type cases was determined using a two-sided t-test from a linear model fit (plotted on the horizontal axis and FDR-corrected q-value is plotted on the vertical axis). FDR was computed using the Benjamini-Hochberg (BH) over all the drugs. The n used to correlate each mutational event with drug sensitivity is reported in the Supplementary Information (Table S17). Expanded and interactive plots are available in our online data browser (www.vizome.org) and (http://vizome.org/additional_figures_BeatAML.html).
(B)Area under the curve for ibrutinib or entospletinib (n=277 or 168 patient samples, respectively) was plotted for cases with single, double, or triple mutation of FLT3-ITD, NPM1, and DNMT3A with the mean and one s.d. indicated by center and outer gray bars, respectively. An ANOVA was conducted using the Bonferroni approach (statistical results and sample size for all groups reported in Supplementary Information, Tables S18,19).
(C) Inhibitors of JAK family kinases were assessed for activity against cases with BCOR mutation alone or BCOR in combination with SRSF2, RUNX1, or DNMT3A. The AUC values are plotted per case with the mean and one standard deviation indicated by center and outer gray bars, respectively. There was a significant difference in AUC by two-sided t-test (t(42)=−2.489, p=0.0168, 95% CI [−73.018, −7.643]) between BCOR and RUNX1 (n=16) versus the average of BCOR alone (n=16), BCOR and SRSF2 (n=8), and BCOR and DNMT3A (n=4).
Mutation of several genes, most notably TP53 or ASXL1 transcriptional regulator 1 (ASXL1), were shown to cause a broad pattern of drug resistance. Interestingly, a few drugs trended more sensitive to TP53 mutant cases, such as elesclemol, or ASXL1, such as panobinostat, hinting at candidates to explore for AML cases with these poor prognostic features. Mutation of NRAS proto-oncogene, GTPase (NRAS) or KRAS proto-oncogene, GTPase) KRAS also correlated largely with resistance to most drugs, though did show the predicted sensitivity to MAPK inhibitors. It was interesting to note a stronger association of NRAS than KRAS mutation with MAPK sensitivity. IDH2 mutation conferred sensitivity to a broad spectrum of drugs, while mutation of IDH1 conferred resistance to most drugs. Mutation of RUNX1 correlated with sensitivity to PIK3C/MTOR inhibitors, such as BEZ235, and to the multi-kinase vascular endothelial growth factor receptor (VEGFR) inhibitor, cediranib. Mutation of splicesome components U2 small nuclear RNA auxiliary factor 1 (U2AF1) and zinc finger CCCH-type, RNA binding motif and serine/arginine rich 2 (ZRSR2) correlated with novel sensitivity to several drugs. The mechanisms underlying these latter sensitivity correlations (and many others in the dataset) merit further investigation. A significant association was seen between mutation of FLT3, NPM1, and DNMT3A with sensitivity to the FDA approved drug, ibrutinib. Since these mutations exhibit a significant pattern of co-occurrence, we next examined every combination of single, double, or triple mutation of these genes with respect to ibrutinib. We observed that DNMT3A alone or DNMT3A/FLT3 double mutant cases were not significantly different than wild type, while cases with FLT3-ITD alone or any variation of NPM1 mutation (including cases with all three genes mutated) were significantly more sensitive than wild type (Fig. 2B). Ibrutinib is an inhibitor of BTK and TEC family kinases, although it can exhibit broad off-target effects when maintained in continuous culture with target cells. We noted that another kinase inhibitor with high specificity for spleen associated tyrosine kinase (SYK), entospletinib, showed a similarly significant pattern of sensitivity for cases with FLT3-ITD and NPM1 (Fig. 2B), potentially pointing to an operationally important target for this disease subset. Indeed, prior work has suggested SYK as an interacting target of FLT3-ITD in AML39. Finally, we wanted to perform an additional analysis that leveraged multiple inhibitors with common targets to see whether this approach could identify additional associations. We focused on correlation of the four selective Janus kinase (JAK) inhibitors on our drug panel (momelotinib, ruxolitinib, tofacitinib, and JAK Inhibitor I) with mutation of BCL6 corepressor (BCOR) alone or co-mutation of BCOR with DNMT3A, RUNX1, or SRSF2. By plotting the average difference of each JAK inhibitor between mutant and wild type groups of these four mutational categories and performing a one-way ANOVA analysis of the four groups, we found that co-mutation of BCOR with RUNX1 correlated with increased sensitivity to all four JAK kinase inhibitors, while BCOR mutation alone or co-mutation of BCOR with DNMT3A or SRSF2 showed no difference in sensitivity to the JAK kinase inhibitors, although BCOR mutation alone did show sensitivity to alternative drugs, such as the tankyrase/WNT inhibitor, XAV-939, and the multi-kinase inhibitor, crizotinib (Fig. 2C). Collectively, these data suggest that JAK pathway dysregulation may represent a vulnerability within certain, specific combinatorial mutation settings and not in others.
We also performed an integration of drug sensitivity data with respect to patterns of gene expression, comparing the 20% of samples with lowest AUC versus the 20% with highest AUC and assessing the most differentially expressed genes between those sample sets. This analysis revealed significant (FDR < .05) expression signatures for 78 testable drugs on the panel (78/119 65.5%). As an example, the 20% most and least sensitive cases to ibrutinib could be clearly distinguished by an expression signature of 17 genes (Fig. 3; Extended Data Fig. 9). Expression signature heatmaps for each drug can be found in our online data browser (http://vizome.org/additional_figures_BeatAML.html).
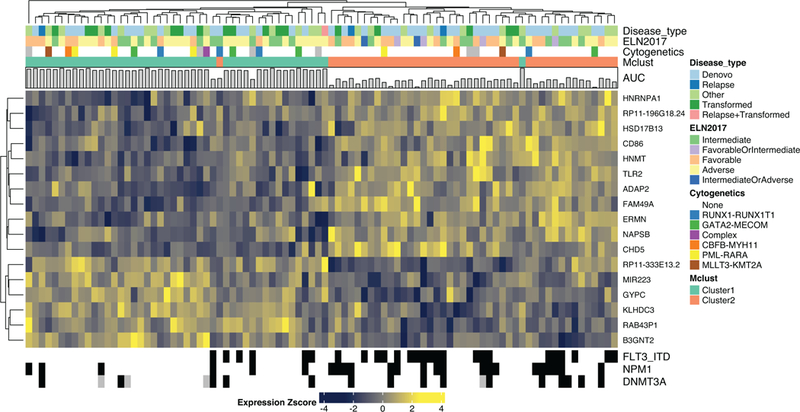
Differential gene expression signature distinguishing the 20% most ibrutinib sensitive (n=46) from 20% most resistant (n=44) specimens. Heatmaps for all other drugs are available in our online data browser (http://vizome.org/additional_figures_BeatAML.html). For the n used to correlate each drug with gene expression, please see Supplementary Information (Table S17).
Finally, to assess the joint contributions of both mutation and system-level co-expression patterns (based on de-novo network inference) in predicting drug response, multivariate modeling was performed. This integrated analysis allows us to move beyond the significant associations of single mutations (such as FLT3-ITD and NPM1). We performed a weighted correlation network analysis (WGCNA) analysis of RNA-sequencing data that identified 14 sets of genes where gene expression patterns showed significant clustering across the cohort (clusters contained both elevated and decreased gene expression events). We performed iterative regression modeling (lasso) to understand how strongly any mutational event or any of these 14 gene expression clusters correlated with sensitivity or resistance to any of the drugs on the panel. We identified numerous, novel co-occurrences of mutations and expression clusters that were associated with drug sensitivity or resistance, with co-occurrences to ibrutinib shown as an example (Extended Data Fig. 9). For ibrutinib, these co-occurrences included a co-expression cluster of 345 genes (using a color labeling scheme and indicated as “turquoise”) that correlated with drug sensitivity and frequently co-occurred with FLT3-ITD, which also correlated with drug sensitivity. There was significant overlap between this “turquoise” gene expression cluster and the 17-gene signature in Figure 3 (indicated by the ratio of observed overlap to expected or representation factor: 13.6; P<1.734e-04). It is important to note that network analysis of this gene expression cluster highlighted enrichment for a number of immune-related pathways, which was not detected within the 17-gene signature (displayed at http://vizome.org/additional_figures_BeatAML.html). We also identified a 110-gene subnetwork (labeled as “magenta” in Figure 6C), which was associated with resistance to ibrutinib and was significantly associated with adverse ELN 2017 risk. To look more broadly at associations between mutations or gene expression clusters, we summarized drugs at family level to assess the frequency by which mutations and gene expression clusters were selected in iterative regression modeling (displayed at http://vizome.org/additional_figures_BeatAML.html)
DISCUSSION
In sum, here we report the largest functional genomic dataset of primary tumor biopsies to date. We present a cohort of AML patient specimens for which we have performed detailed clinical annotations, genomic and transcriptomic analyses, and ex vivo drug sensitivity studies, and we provide analytical approaches for data integration. Each of these datasets alone has revealed new information about the biology and potential translational approaches in AML, and the integration of these datasets has been revealing of new markers and mechanisms of drug sensitivity and resistance that merit further study. These data have all been made publicly available through the NIH/NCI dbGaP and Genomic Data Commons (GDC) resources, and we have developed tools to facilitate user interface with the dataset (www.vizome.org). We hope and expect that this public data release will stimulate further use of the data such that novel findings can be derived and parlayed into new clinical approaches for AML.
METHODS
Patient Samples
All patients gave informed consent to participate in this study, which had the approval and guidance of the institutional review boards at Oregon Health & Science University (OHSU), University of Utah, University of Texas Medical Center (UT Southwestern), Stanford University, University of Miami, University of Colorado, University of Florida, National Institutes of Health (NIH), Fox Chase Cancer Center and University of Kansas (KUMC). Samples were sent to the coordinating center (OHSU; IRB#9570; #4422; NCT01728402) where they were coded and processed. Specific names of centers associated with each specimen were coded and centers providing less than 5 samples were aggregated together and given one center identifier. Mononuclear cells were isolated by Ficoll gradient centrifugation from freshly obtained bone marrow aspirates or peripheral blood draws. Cell pellets were snap frozen in liquid nitrogen for subsequent DNA isolation (Qiagen, DNeasy Blood & Tissue Kit), freshly pelleted cells were lysed immediately in guanidinium thiocyanate (GTC) lysate for subsequent RNA isolation (Qiagen, RNeasy Mini Kit), and freshly isolated mononuclear cells were plated into an ex vivo drug sensitivity assays within 24 hours (described in detail below). Skin punch biopsies were collected at the site of Jamshidi needle insertion for subsequent bone marrow biopsies and genomic DNA was isolated for use as matched normal controls for exome sequencing (Qiagen, DNeasy Blood & Tissue Kit). Clinical, prognostic, genetic, cytogenetic, and pathologic lab values as well as treatment and outcome data were manually curated from patient electronic medical records. Patients were assigned a specific diagnosis based on the prioritization of genetic and clinical factors as determined by WHO guidelines. To prevent re-identification, any patient over the age of 90 was placed into a >90 aggregated age bracket. Genetic characterization of the leukemia samples included results of a clinical deep-sequencing panel of genes commonly mutated in hematologic malignancies (Sequenome and GeneTrails (OHSU); Foundation Medicine (UTSW); Genoptix; and Illumina).
Whole Exome and Custom Capture Validation Sequencing
For exome sequencing, Illumina Nextera RapidCapture Exome capture probes and protocol were utilized, which provided coverage of 37 Mb of genomic DNA coding regions. Briefly, following initial QC on a TapeStation (Agilent), 50 ng of intact genomic DNA was fragmented and tagged (tagmentation) in a single step. Following clean-up, the tagmented DNA was amplified by 10 cycles of PCR, which added the indexed adaptors for clustering and sequencing. Libraries were hybridized to capture pools in 12 sample sets with two rounds of hybridization performed to increase specificity. Libraries recovered with streptavidin magnetic beads were amplified by 10 cycles of PCR, unincorporated reagents were removed with AMPure beads (Agencourt), and validated on the TapeStation. Quantification of capture pools was done using real time PCR (Kapa). Libraries were denatured, flow cells set up using the cBot (Illumina), and run on a HiSeq 2500 using paired end 100 cycle protocols. For the AML tumor samples, 5 or 6 lanes were run per capture group. For the matched skin biopsy samples, 3 lanes (or equivalent) were run per capture group. The instrument and chemistry for all capture groups are provided in the Supplementary dashboard tables (Supplementary Information, Table S12,13).
For validation of sequencing results, an 11.9 megabase custom capture library was developed that provided coverage of all variants previously reported in AML as well as all new variants detected from exome sequencing in this study (Genes, Variants, and Capture Regions for this custom library are found in Supplementary Information, Table S14). This capture library was then applied to sequence 96 specimens that had previously been subjected to whole exome sequencing for validation of variant calls.
Whole Exome Sequencing Data Processing
We developed customized analytical pipelines that combined published algorithms with novel filtering, curation, and quality control steps. Detailed depictions of analytical workflows are found in Supplementary Information (Table S6) and on our online browser (http://vizome.org/additional_figures_BeatAML.html). Initial data processing and alignments were performed with commonly used analytical tools. For each flowcell and each sample, the FASTQ files were aggregated into single files for read 1 and 2. During this process these reads were trimmed by 3 on the 5’ end and 5 on the 3’ end. BWA MEM version 0.7.10-r78941 was used to align the read pairs for each sample-lane FASTQ file. As part of this process, the flowcell and lane information was kept as part of the read group of the resulting SAM file. The Genome Analysis Toolkit (v3.3) and the bundled Picard (v1.120.1579) were used42 for alignment post-processing. The files contained within the Broad’s bundle 2.8 were used including their version of the build 37 human genome (These files were downloaded from: ftp://ftp.broadinstitute.org/bundle/2.8/b37/). The following steps were performed per sample-lane SAM file generated for each CaptureGroup:
The SAM files were sorted and converted to BAM via SortSam
MarkDuplicates was run, marking both lane level standard and optical duplicates
The reads were realigned around indels from the reads--RealignerTargetCreator/IndelRealigner.
Base Quality Score Recalibration
The resulting BAM files were then aggregated by sample and an additional round of MarkDuplicates was carried out at the sample level. Quality control reports were generated using the ReportingTools43 and qrqc44 Bioconductor R packages along with sequencing core and alignment output files. Each AML sample BAM was paired with its skin biopsy pair and an additional round of indel realignment was carried out to ensure consistency of genotypes between the two samples. If an AML sample did not have a pair, the indel realignment was instead done at the sample level.
Whole Exome Sequencing Variant Detection
For genotyping, each AML/skin biopsy pair was realigned at the sample level and then genotyped for single nucleotide variations using Mutect v1.1.745 and Varscan2 v2.4.146. Indels were produced using Varscan2. Each variant call format (VCF) file was annotated using the Variant Effect Predictor v83 against GRCh3747. The resulting VCF files were filtered to include only those annotated to a gene and were converted to mutation annotation format (MAF) format using the vcf2maf v1.6.6 tool48.
Mutect v1.1.745 was run using default parameters except that no limit was placed on the number or frequency of the alternative allele frequency in the normal to help address normal contamination.
Varscan2 v2.4.146 was run in somatic mode with the recommended filtering scheme49 except as shown in Supplementary Information, Table S23.
Indels and single nucleotide variants (SNVs) were produced for the tumor-only samples again using Mutect without a specified normal for consistency and VarScan2 in mpileup2indel or mpileup2snp mode respectively.
These variants were assigned to their most deleterious effect on Ensembl transcripts using Ensembl VEP v83 on GRCh37. This assignment was done using the same VEP parameters as the vcf2maf (v1.5.0) program.
The TCGA AML variants6 in MAF form were downloaded from the GDC archive site: https://portal.gdc.cancer.gov/legacy-archive/files/c410d927-d49c-4d4f-8356–601bee563ebe. The MAF was converted to VCF files using the vcf2maf suite50. The resulting VCF files were lifted over from NCBI36 to GRCh37 of the human genome using CrossMap51. Only those variants that successfully lifted over were kept. Variants from Table S2 in Jaiswal et al 201414 were extracted and further processed removing variants that were ambiguous in terms of external sources and could not be found in their whole exome sequencing (WES) variants. The unique set of BeatAML variants was annotated relative to RefSeq transcripts using Ensembl VEP similar to above and all consequences were kept. This set of variants and consequences was searched against the set of processed Jaiswal variants.
Using the runs from MuTect and VarScan, these data were next filtered to keep only the protein impacting SNVs and indels from Mutect and VarScan2 and filtered requiring that the variants had at least 5 reads and either not be seen in the exome aggregation consortium (ExAC)52 or be seen at a frequency < .01. These data present several additional challenges. First somatic calls cannot be obtained directly from the tumor-only samples, second there is always a possibility of tumor contamination of the skin samples for those samples that were paired. To address these issues and maximize comparability we used an iterative approach. The following was done separately for the two genotypers:
An initial set of higher confidence somatic mutations were retrieved from the paired tumor/normal samples requiring tumor variant allele frequency (VAF) >= 8% and normal VAF <= 5% in addition to the significance tests already performed by the programs.
A list of all candidate mutations was collated requiring that a mutation was either seen in the high confidence somatic set, the set of variants from Jaiswal et al or from the lifted over set of variants from the TCGA AML paper.
Mutations from the overall set were kept if:
○ The overall number of calls in the paired samples was not more than twice the number of high confidence somatic calls
○ The tumor-only frequency for the calls was less than 50% greater than the number of calls in the paired samples
○ The mutation was seen in Jaiswal/TCGA list
High Confidence somatic mutations were kept regardless
The data from the two genotypers were combined along with FLT3-ITD calls from Pindel53. Comparing our variant lists from whole exome sequencing versus our custom capture validation sequencing, we noticed, similar to others54, that low allele frequency C->A variants (< 15%) tended to have poor concordance (7.7%; data not shown) between the initial run and the technical validation run. These variants were removed in these data and along with a curated ‘blacklist’ (Supplementary Information, Table S15) of known problematic variants/genes including mitochondrial DNA variants. In addition, all variants that were seen in a cumulative list of BeatAML normal samples at a frequency greater than 1% were removed. Cumulatively, of this set, 94% of covered single-nucleotide variants were validated with 82% of insertion/deletion calls also being confirmed with validation sequencing.
Manual review was then carried out in the following steps:
- a.)
The addition back of all Jaiswal flagged rows.
- b.)
Reviewed all TCGA flagged rows for VAF pattern that matched or did not match with known drivers in same specimen. Some TCGA variants were added back based on convincing VAF pattern and known pathogenic role, other TCGA variants were kept excluded based on VAF pattern unlike known drivers in same specimens.
- c.)
Other variants were added back based on other specimens that had the same variant that was still on the include list and VAF pattern looked convincing for inclusion.
- d.)
All Jaiswal genes with only frameshift/nonsense variants were manually reviewed and missense mutations were manually removed.
- e.)
Genes/variants that were on both the include and exclude lists were manually reviewed and were removed if c to a with over 15% VAF, did not validate, and/or VAF pattern unlike known drivers in same specimen
- f.)
Further review of all genes in summary sheet with cohort frequency of 8 or more (1% of more). Any that were not familiar from knowledge of AML literature were manually reviewed for VAF patterns that did or did not match known drivers within same specimens. Those that did not match were manually removed.
After this manual review, additional curated mutations from the UnifiedGenotyper run were added back in along with a curated set of variants from tumor-only patients in Jaiswal et al genes. The tumor-only AML samples utilized in Zhang et al (submitted) were removed and utilized for that study.
Internal FLT3-ITD and NPM1 mutation detection
A subset of samples was tested for FLT3-ITD and NPM1 mutation status using an internally run PCR assay and capillary electrophoresis. Genomic DNA was extracted from fresh blood or bone marrow aspirates of AML patients was used to detect the presence or absence of FLT3-ITD and NPM1 4 bp insertion mutations55,56. Primers for FLT3 spanned approximately 330 bp to include the common internal duplication site55. Primers for NPM1 spanned approximately 170 bp to cover the clustered multiple insertional or insertion/deletion sites56,57. Primers were HPLC-purified by the manufacturer. The multiplex PCR reaction solution consisted of 100 ng gDNA, 10 pmol of the respective forward and reverse primers for FLT3 and NPM1, 25 mmol/l MgCl2, 2·5 mmol/l dNTP, 5 μl 10 × PCR buffer, 0·2 μl AccuTaq DNA polymerase and water in a total volume of 50ul58. The PCR conditions were: initial denaturing for 5 min at 94°C, followed by 30 cycles at 94°C for 30 s, 56°C for 45 s and 72°C for 30 s with a final cycle of 10 min at 72°C. The PCR products were diluted 1:10 and analyzed by capillary electrophoresis on a QIAxcel high resolution DNA cartridge according to the manufacturer’s protocol.
Forward Primer FLT3: 5′ - AGCA ATT TAG GTA TGA AAG CCA GCTA - 3′
Reverse Primer FLT3: 5′ - CTT TCA GCA TTT TGA CGG CAA CC - 3′
Forward Primer NPM1: 5′ - GTT TCT TTT TTT TTT TTT CCA GGC TAT TCA AG - 3′
Reverse Primer NPM1: 5′ - CAC GGT AGG GAA AGT TCT CAC TCT GC - 3′
Derivation of FLT3-ITD and NPM1 consensus calls
Consensus FLT3-ITD and NPM1 mutation calls found in the clinical summary table (Supplementary Information, Table S5) were determined by comparing the internal capillary PCR test (internal; method described above), the Clinical Laboratory Improvement Amendments/College of American Pathology (CLIA/CAP) laboratory run test (Sequenome, GeneTrails, Foundation Medicine, Genoptix, Illumina). The internal test was used for the sample consensus call when available as it was performed on the exact sample that was used for further ex vivo drug sensitivity assays. Where discordance existed between the internal test versus the CLIA lab test results, the sample was flagged for manual review. The trace file for the internal test was visually inspected and if discordance with the CLIA/CAP test results persisted, the whole exome sequencing data was then used to help determine the consensus call.
Derivation of CCAAT enhancer binding protein alpha (CEBPA) biallelic consensus calls
N-terminal and C-terminal mutations have been described to occur on opposing alleles and patients harboring CEBPA biallelic mutations have been shown to fall into a favorable risk category59. Patients were scored positive for biallelic CEBPA mutation if described in the clinical notes as biallelic or double positive. Patients were also scored as CEBPA biallelic if both N-terminal and C-terminal mutations were identified in the whole exome sequencing data.
RNA-Sequencing and Data Processing
All samples were sequenced using the Agilent SureSelect Strand-Specific RNA Library Preparation Kit on the Bravo robot (Agilent). Briefly, poly(A)+ RNA was chemically fragmented. Double stranded cDNAs were synthesized using random hexamer priming with 3’ ends of the cDNA adenylated then indexed adaptors were ligated. Library amplification was performed using three-primer PCR using a uracil DNA glycosylase addition for strandedness. Libraries were validated with the Bioanalyzer (Agilent) and combined to run 4 samples per lane, with a targeted yield of 200 million clusters. Combined libraries were denatured, clustered with the cBot (Illumina), and sequenced on the HiSeq 2500 using a 100-cycle paired end protocol. In addition to the AML samples, there was also a sample of purified CD34 molecule (CD34)+ cells from healthy control bone marrow, which was included in each sample group (for a total of 12 times sequencing this control RNA). This control served as both a healthy comparator and a quality check on inter-group batch effects. 21 additional, individual healthy bone marrow samples were also included, two of which were CD34-selected (17–00053 and 17–00056) with the other 19 being whole mononuclear bone marrow cells from healthy donors.
Workflows for processing and analysis of RNA sequencing data to generate gene counts and gene fusions for each sample are shown on our online browser (http://vizome.org/additional_figures_BeatAML.html) with processed gene expression values for each specimen listed in Supplementary Information (Table S8,9). For each flowcell and each sample, the FASTQ files were aggregated into single files for read 1 and read 2 (if not already done by the sequencing core). During this process these reads were trimmed by 3 on the 5’ end and 5 on the 3’ end. Alignments of reads was performed using the subjunc aligner (1.5.0-p2)60. BAM files obtained from subjunc were used as inputs into featureCounts (1.5.0-p2)61 and gene-level read counts were produced. For a reference genome, the GRCh37 build provided by the Broad as part of the GATK bundle was used. Gene assignments were based on the Ensembl build 75 gene models on GRCh37. See the parameters below for software usage.
subjunc -i /path/to/reference/ -u -r fastq1 -R fastq2 -o outputBAMFilename -I 5 -T 7 -d 50 -D 600 -S fr
featureCounts -a Homo_sapiens.GRCh37.75.gtf -o output -F GTF -t exon -g gene_id -s 2 -C -T 10 -p -P -d 50 -D 600 -B BAM_files
The data was collated from featureCounts matrices and all genes with no counts across the samples were excluded. Genes with duplicate gene symbols and those where the counts were < 10 for 90% or more of the samples were additionally removed prior to normalization similar to the approach suggested for weighted gene correlation network analysis (WGNCA)62. Samples for which their median expression was less than 2 standard deviations below the average were removed from the dataset (N=10). Normalization was performed using the conditional quantile normalization procedure63, which produced GC-content corrected log2 reads per kilobase per million mapped reads (RPKM) values. This procedure produces both offsets to be used in conjunction with edgeR as well as a matrix of log2 normalized RPKM values for clustering.
In addition, the subjunc BAM files were processed using the RNA-sequencing genotyping protocol (as of GATK v3.3) which was similar to the WES protocol described in the ‘Whole Exome Sequencing’ section including for each sample:
MarkDuplicates
SplitNCigarReads
RealignerTargetCreator/IndelRealigner.
Base Quality Score Recalibration
The resulting BAM files were used to produce RNA genotypes using the UnifiedGenotyper for the purposes of QC and ethnicity estimation.
Gene fusion data was additionally generated using the TopHat-Fusion (v2.0.14) program using default parameters64.
Coexpression network formation
We formed coexpression modules using the WGCNA procedure on the RNA-sequencing data from the ‘RNA-Sequencing and Data Processing’ section. All RNA-sequencing samples were used to form the set of modules. Due to the heterogeneity of clinical expression data we generated ‘signed hybrid’ networks using ‘bicor’ correlation setting the max proportion of outliers to .165. We ran the procedure multiple times, varying several parameters to choose the most relevant set for further analysis. The WGCNA procedure was run on datasets formed from the top 2,000 and 5,000 most variable genes. For each dataset we set the ‘power’ variable to either 2 or 3. For each of these runs we varied the module detection parameters of dynamicTreeCut62, namely the deepSplit parameter was set to 0 or 2 and the pamStage parameter was set to TRUE or FALSE. For each of these sets of modules we computed a series of module quality statistics66, mean correlation, mean adjacency, mean MAR, mean KME, proportion of variability explained and the mean cluster coefficient. Significance of modules was determined by computing a Zscore of each of these values relative to the mean and standard deviation of those from 100 random assignment of modules. We chose the set of modules to use in our analyses as those that were most correlated with the ‘specimentSpecificDx’ using the module quality as a tie-breaker. The analysis set of modules was chosen to be the version using the 5,000 most variable genes, power set to 2 and modules formed using deepSplit=2 and pamStage=F. Of this set of modules only the grey module didn’t have a summary Z statistic (median across the 4 density measures) of at least 2. Additionally after correcting the data using the estimated principal components67, the module structure didn’t change appreciably (data not shown).
Quality Control
The UnifiedGenotyper runs for both the WES and RNA-sequencing were combined into a single VCF file using the GATK CombineVCFs functionality. This combined VCF file was converted to a GDS file using SNPRelate (1.12.2)68. Note the version is an upper bound as several versions were used across the entire project). The overall similarity of the genotypes of each pair of samples were computed, termed identity by state (IBS) and a hierarchical clustering was performed using one minus this similarity. From this clustering and visualization we had devised hard cutoffs for further inspection based on the types of data being compared. For instance samples not meeting the specified IBS thresholds (DNA-DNA=.9; RNA-RNA=.83; DNA-RNA=.89) were subject to manual review. Based on the dendrogram structure as well as the clinical/lab information, samples were either excluded, assigned to a different patient ID or in rare cases assigned to a different sample. It was observed that bone marrow transplants between sample collections produced a noticeable but milder effect in these dendrograms and such samples were flagged for removal in RNA-sequencing analysis and for treatment as tumor-only samples in the WES analysis as is described in the ‘WES Variant Detection’ section.
Fusion annotation for analysis
Fusions calls were determined from a consensus of three datatypes, a specific diagnosis categorization at the time of sample acquisition, current set of clinical karyotypes and fusions detected in RNA-sequencing data by TopHat-Fusion. All sources were limited to the same set of known fusions: RUNX1-RUNX1T1, CBFB-MYH11, MLLT3-KMT2A, DEK-NUP214, GATA2-MECOM and PML-RARA. It was determined that the RNA-sequencing calls did not provide additional resolution in detecting these known fusions and was not performed on all the samples so the consensus was limited to the clinical karyotype calls as well as the specific diagnosis categorization (which was determined based on karyotype and other cytogenetic clinical tests). Overall the calls were based on the karyotype data except in 10 cases, 3 where the karyotype and diagnosis was sufficiently complex to warrant a separate ‘Complex’ categorization. The remaining 7 of these cases were set to the specific diagnosis classification. It should be noted there was additional support from the RNA-sequencing data for several of these cases.
Ethnicity
The combined RNA and WES VCF from the ‘Quality Control’ step was merged with a set of Hapmap genotypes69 lifted over to build 37. The SNPRelate package was used to convert the VCF to GDS, perform LD pruning using an LD threshold of .2, MAF cutoff of .05 and allowing a missing rate of .3 and calculation of the principal components. The methodology of Zheng and Weir 201570 was used to assign admixture proportions relative to the HapMap samples using the principal components. Each sample was assigned to an ethnicity group based on the group with the maximum admixture proportion. If the maximum was 50% or less, we labeled it Admixed. As we have observed previously that the clustering of ethnicities for RNA-sequencing samples are more diffuse than exome sequencing, we assigned the final inferred ethnicity to each patient based on the distinct WES calls if available, deferring to RNA-sequencing only if not available. If multiple exome sequencing samples were present with discrepant calls, the patient was subject to manual review. The only patient where this occurred was patient 4043, a self-identified Hispanic who had 2 RNA samples and an exome sequencing sample inferred as White and one exome sample inferred to be HispNative. Only the White call for the exome sequencing had an admixture proportion over .5. The patient was kept consistent with the self-identification and labeled as HispNative.
Sex
For DNA, coverage is first computed over the Y chromosome and the counts for each sample are added up and log10 transformed (after adding 1 to all the counts). K-means clustering is used to assign samples to 2 clusters with the cluster with the lower mean labeled as the Female cluster.
For RNA-sequencing, counts were converted to counts per million (CPM) after applying the Trimmed Mean of M scaling normalization71. A set of 28 genes chosen to successfully discriminate the genders via DE analysis over multiple studies (data not shown) were used in conjunction with Kmeans clustering to form two clusters. The Female cluster was labeled based on high XIST expression.
ELN 2017 Classification
This procedure is based off of the categorization in Table 5 of the 2017 ELN update paper5
Karyotypes in the clinical file were first cleaned and parsed into clones/subclones and distinct abnormalities using standard conventions72. The current representation was corrected for nomenclature type (e.g. idem vs sl) in a basic manner. For instance, ambiguous events such as chromosomal loss (e.g. −15) was not corrected for whether the preceding clone had a counteracting gain. Also, additional +/− symbols in conjunction with valid karyotype operators in a separate clone (e.g. +del(12)(q?15) or -del(12)(q?15)) ) were treated separately with gains (+) being kept in the unique count of events and losses (−) being removed.
Abnormalities were first checked for the following categories:
RUNX1-RUNX1T1
CBFB-MYH11
MLLT3-KMT2A
DEK-NUP214
KMT2A-*
BCR-ABL1
GATA2-MECOM
−5/del(5q) (termed minus_5)
−7 (termed minus_7)
−17 (termed minus_17)
Note the bold were further considered to be WHO recurrent fusions.
The number of unique abnormalities (across clones) was then computed.
Whether or not a karyotype was considered to be polyploid was also recorded (at least 60 chrs or ‘(>=3)n’ or label).
NPM1, FLT3-ITD, and biallelic CEBPA were derived from consensus calls. FLT3-ITD allelic ratios were determined solely for the samples with an internal assay. The MAF values of the internal assay were converted to a ratio using the formula MAF/(1-MAF).
RUNX1, ASXL1 and TP53 were derived from the clinical genotypes.
Abn_17 calls were manually curated from the karyotype data and clinical genotype calls respectively.
The determination of ELN 2017 categories proceeds by assigning TRUE/FALSE/NA values to one or more of the 5 columns (3 ELN and 2 ambiguous) in the following way:
isFavorable is considered TRUE if a sample has at least one of the following:
RUNX1-RUNX1T1
CBFB-MYH11
Positive NPM1 and negative FLT3-ITD
Positive NPM1 and positive FLT3-ITD with allelic ratio < .5
Biallelic CEBPA
isFavorableOrIntermediate
NPM1 is positive and FLT3-ITD is positive but the allelic ratio is not available
isAdverse is considered TRUE if a sample has at least one of the following:
DEK-NUP214
KMT2A-*
BCR-ABL1
GATA2-MECOM
minus_5
minus_7
minus_17
abn_17
3 or more abnormalities and not a WHO recurrent fusion
One monosomy (autosomal) and at least one additional abnormality except for CBFB-MYH11
Positive RUNX1 or ASXL1 and not considered to be isFavorable or isFavorableOrIntermediate
Positive TP53
isIntermediate is considered TRUE if a sample has at least one of the following:
MLLT3-KMT2A
NPM1 positive and positive FLT3-ITD with allelic ratio >= .5
NPM1 negative and negative or low allelic ratio (<.5) FLT3-ITD
At least one abnormality and is not considered isFavorable or isAdverse
isIntermediateOrAdverse
NPM1 negative and FLT3-ITD positive without an allelic ratio
NAs occur in the absence of FLT3-ITD or NPM1 calls.
Samples where the specific diagnosis at inclusion indicated ‘Acute promyelocytic leukaemia with t(15;17)(q22;q12)’ were automatically set to ‘Favorable’.
Any overlaps of the categories were resolved based on manual expert review.
Ex vivo Functional Drug Screens
Ex vivo functional drug screens were performed on freshly isolated mononuclear cells from AML samples. Briefly, 10,000 cells per well were arrayed into three, 384-well plates containing 122 small-molecule inhibitors. This panel contained graded concentrations of a drugs with activity against two-thirds of the tyrosine kinome as well as other non-tyrosine kinase pathways, including mitogen activated protein kinases (MAPKs), phosphatidylinositol-4,5-bisphosphate 3-kinase/AKT serine/threonine kinase 1/mechanistic target of rapamycin kinase (PIK3C/AKT/MTOR), protein kinase AMP-activated (AMPK/PRKAA), ATM serine/threonine kinase (ATM), Aurora kinases, calcium/calmodulin dependent protein kinases (CAMKs), cyclin-dependent kinases (CDKs), serine/threonine protein kinase 3 (GSK3), I-kappaB kinase (IKK), cAMP dependent protein kinase (PKA), protein kinase C (PKC), polo-like kinase 1 (PLK1), and RAF proto-oncogene serine/threonine kinase (RAF). In addition, the library contained small molecule inhibitors with activity against the BCL2 family, bromodomain containing 4 (BRD4), Hedgehog, heat shock protein 90 (HSP90), NOTCH/gamma-secretase, proteasome, survivin, signal transducer and activator of transcription 3 (STAT3), histone deacetylase (HDAC), and WNT/beta-catenin. Drug plates were created using inhibitors purchased from LC Laboratories and Selleck Chemicals and master stocks were reconstituted in dimethyl sulfoxide (DMSO) and stored at −80 °C. Master plates were created by distributing a single agent per well in a seven-point concentration series, created from three-fold dilutions of the most concentrated stock resulting in a range pf 10 μM to 0.0137 μM for each drug (except dasatinib, ponatinib, sunitinib, and YM-155 which were plated at a concentration range of 1 μM to 0.00137 μM). DMSO control wells and positive control wells containing a drug combination of Flavopiridol, Staurosporine and Velcade were placed on each plate, with the final concentration of DMSO ≤0.1% in all wells. Daughter plates were created using a V&P Scientific 384-well pin tool head operated by the Caliper Sciclone ALH 3000 and equipped with 0.457mm diameter, 30 nanoliter, slotted stainless steel pins (cat num: FP1NS30). Daughter and destination plates were sealed with pealable thermal seals using a PlateLoc thermal sealer. Destination plates were stored at −20 °C for no more than three months and thawed immediately before use. Primary mononuclear cells were plated across single-agent inhibitor panels within 24 h of collection. Cells were seeded into 384-well assay plates at 10,000 cells per well in Roswell Park Memorial Institute (RPMI) 1640 media supplemented with fetal bovine serum (FBS) (10%), l-glutamine, penicillin/streptomycin, and β-mercaptoethanol (10−4 M). After 3 d of culture at 37 °C in 5% CO2, MTS reagent (CellTiter96 AQueous One; Promega) was added, optical density was measured at 490 nm, and raw absorbance values were adjusted to a reference blank value and then used to determine cell viability (normalized to untreated control wells).
Ex vivo Functional Drug Screen Data Processing
A workflow summarizing data normalization, curve fit parameters, and quality assurance/quality control (QA/QC) steps is found on our online browser (http://vizome.org/additional_figures_BeatAML.html) with processed drug response data for each specimen listed in Supplementary Information (Table S10). A given sample was run on one or more panels and within each panel, the majority of drugs were run without within-panel replicates. Two steps were performed to harmonize these data prior to model fitting:
A ‘curve-free’ AUC (integration based on fine linear interpolation between the 7 data points themselves) was calculated for those runs with within-panel replicates after applying a ceiling of 100 and a floor of 0 for the normalized viability. The maximum change in AUC amongst the replicates was noted and those runs with differences > 100 were removed.
Remaining within-plate replicates had their normalized viability averaged and subject to a ceiling of 100 and floor of 0. An additional set of ‘curve-free’ AUCs was computed for sample-inhibitor pairs run on multiple panels. The maximum change in AUC amongst the across-panel replicates was noted and those runs with differences > 75 were removed.
At this point, the within and across plate replicates for the normalized viability were averaged together and a ceiling of 100 was applied. From the steps above, the floor was already at 0.
Based on the methodology used in our prior drug combination study73, a probit regression was fit to all possible run groups using the model:
Where for all groups there were N=7 dose-response measurements.
The summary measures of curve fit were inspected and cutoffs were devised removing all runs with an AIC > 12 and deviance > 2. For inhibitors that were run using multiple concentration ranges, only the latest concentration range was kept. Finally, these data were compared to the AUC values from third order polynomial fits. Those runs that were discrepant in terms of sensitive/resistant calls were manually reviewed as subject to removal.
Ex vivo Functional Drug Screen Analysis
For all drug analyses requiring a call of sensitivity or resistance (e.g. the gene expression signatures), sensitivity/resistance was determined by the lowest and highest 20% of the AUC values for each drug.
Correlations between drugs in families
For each inhibitor in the study, available data on targets of the inhibitors were pulled from a variety of online resources and published studies, many of which were aggregated in the Cancer-Targetome74,75. Activity of each inhibitor for targets was then distilled into a 5-tier system to afford comparison across drugs with differing degrees of potency and/or for which differing assays were used to measure drug/target activity. Well-represented genes, gene families, and pathways were then filtered for drugs having activity in the top 3 tiers for one or more member of the gene family or pathway. These lists were then manually curated to arrive at a final list of high-confidence drug target families (shown in Supplementary Information, Table S11). For each inhibitor assigned to at least one drug target family, the Pearson’s correlation was computed against all other drugs assigned to at least one drug target family for the AUC values of all available samples shared between the two drugs.
Correlations between drugs/samples
Drugs were first filtered requiring greater than two hundred samples per drug. Additional samples were removed accordingly to allow correlations to be computed between all present samples using available AUC data and between all drugs.
Summary Drug Response Scores
For each patient sample, a binary encoding (1/0) was used for each drug based on same threshold as for the gene signatures (e.g., sensitivity/resistance was determined by the lowest and highest 20% of the AUC values for each drug). Individual scores were computed for resistance and sensitivity separately and represented as the proportion over all drugs screened for each patient sample.
Expression Analysis and Integration with Ex Vivo Functional Drug Screen
For all the below analyses the earliest sample was chosen for each patient.
Expression heatmap
The top 2,000 most variable genes were extracted. The expression values were centered and scaled across patients and complete-linkage hierarchical clustering was performed using the ComplexHeatmap R package76.
Sensitive/Resistant differential expression
For each drug, it was required that at least 3 sensitive and 3 resistant samples using the 20%/20% criteria outlined in the ‘Drug Analysis’ section. Patient samples were limited to those labeled as sensitive or resistant. Next, genes were limited based on their expression, where at least half the patients used for analysis had to have greater than one counts per million (an approach suggested in the limma users manual77. The normalized expression as in the ‘RNA-Seq Processing’ section data with the chosen samples and genes was used for differential expression analysis. As the data had not been batch corrected at this point, surrogate variable analysis (SVA)78 was used to infer covariates for correcting out technical confounders. Next the linear model fitting for each gene was performed using the limma-trend approach79 testing whether the average expression was different between resistant – sensitive correcting for the SVA covariates. Genes with Benjamini-Hochberg (BH)38 FDR values of less than .05 were kept for the cluster analysis. The expression matrix was corrected with respect to the estimated surrogate variables for consistency with the differential expression procedure using fSVA80 and Mclust81 was used to determine optimal number of clusters and parameterization. The results were then visualized using a clusplot82 which displays the clustering results with respect to the first two principal components of the gene expression for the kept genes.
Mutation Analysis and Integration with Ex vivo Functional Drug Screen
For all the below analyses where groups of samples were compared, the earliest sample was chosen for each patient.
TCGA comparison
The lifted over TCGA variants from the ‘WES Variant Detection’ section were annotated using the VEP from Ensembl build 83, filtered for protein-altering and splice site variants and our ‘blacklist’ was applied to ensure the variant sets were comparable.
Co-occurrence/mutual exclusivity
Only mutations seen in at least 10 patients were kept. The DISCOVER40 method was used to determine significant mutual exclusivity and co-occurrence. A plot of the co-occurrences was generated using corrplot83 with the odds ratio of the pairwise co-occurrence used to color and scale the circle sizes.
Mutation/drug association
For each mutated gene in the exome sequencing samples and each recurrent fusion (counting FLT3-ITD as a distinct entity from other FLT3 mutants) we determined all available (at least 5 patients) pairwise and three-way co-occurrence sets. For each drug and each valid set of genes (from one to three genes) we fit a linear model with AUC as the response and examined the linear contrast (i.e.. two-sided t-test) comparing the AUC of the gene(s) to the appropriate negative. For example the average AUC of the FLT3:DNMT3A:NPM1 mutants would be compared to average AUC of the samples negative for all three genes. FDR was computed using the BH over all the drugs.
For the Ibrutinib and entospletinib comparisons, the presence and absence of the 3 genes/mutations: NPM1, FLT3_ITD and DNMT3A was collapsed into levels of a single factor. The corresponding single factor ANOVA was carried out with the ‘triple negative’ category set as the reference. Significance of the p-values of each coefficient were compared to the Bonferroni corrected .05 level.
For the JAK-family analysis, the AUC values were pooled for the four JAK inhibitors (CYT387, Tofacitinib (CP-690550), JAK Inhibitor I, Ruxolitinib (INCB018424)) for each gene mutation set (BCOR, BCOR:DNMT3A, BCOR:RUNX1, BCOR:SRSF2). The contrast of the difference between BCOR:RUNX1 samples and the average of the other three mutation groups was tested.
Integration of both mutation and RNA-Sequencing with Ex vivo Functional Drug Screen
Mutations (0/1 encoding) and the module eigengenes from the WGCNA analysis were used separately and combined together in regression models with coefficients selected using the lasso approach84 as implemented in glmnet85. For each datatype and the combination, only drugs with at least 200 patients samples were tested. The 3 datasets were initially randomly separated into training (75%) and test (25%) sets. Similar to a previous approach86, a bootstrap aggregation approach was used where the 1,000 bootstraps of the training dataset was generated and for each one, the lasso trained using 10 fold cross-validation. Predictions were formed for the test dataset over these bootstrap models and the predicted AUC was averaged. R2 values were computed for these aggregated predictions relative to the test AUC values. As performance was seen to be dependent on the initial test/training split, we repeated the entire process 100 times, recording the mean and standard deviation of the R2 value as well as the count non-zero coefficients.
Extended Data
Extended Data Fig. 1.

622 specimens from 531 unique patients were subjected to whole exome sequencing. Automated and manual curation steps (described in the Methods, Supplementary Information, and at http://vizome.org/additional_figures_BeatAML.html) were used to arrive at a final set of high confidence variants (annotated in Supplementary Information, Table S7) and the earliest sample for each unique patient was used in this analysis. Clinical cytogenetics and gene fusion calls from RNA-sequencing were used to curate recurrent gene rearrangements (Supplementary Information). The mutational profile for each unique patient is shown with frequency ranked mutational events in the upper portion and frequency ranked gene rearrangements in the lower portion. The mosaic plot is annotated with clinical features of each case, such as diagnosis/relapse and de novo/transformed disease states and the first bar chart to the right summarizes the cohort frequencies of mutational and gene rearrangement events. The last bar chart on the right summarizes the frequency of significant drug/mutation associations for the given gene across the cohort with drug sensitivity displayed in red and drug resistance displayed in blue. Eleven genes not previously reported to be somatically mutated in cancer were observed with mutations at ~1% cohort frequency: CUB and Sushi multiple domains 2 (CSMD2), NAC alpha domain containing (NACAD), teneurin transmembrane protein 2 (TENM2), aggrecan (ACAN), ADAM metallopeptidase with thrombospondin type 1 motif 7 (ADAMTS7), immunoglobulin-like and fibronectin type III domain containing 1 (IGFN1), neurobeachin like 2 (NBEAL2), poly(U) binding splicing factor 60 (PUF60), zinc finger protein 687 (ZNF687), cadherin EGF LAG seven-pass G-type receptor 2 (CELSR2), and glutamate ionotropic receptor NMDA type subunit 2B (GRIN2B). For the n used to correlate each drug with mutations, please see Supplementary Information (Table S17).
Extended Data Fig. 2.
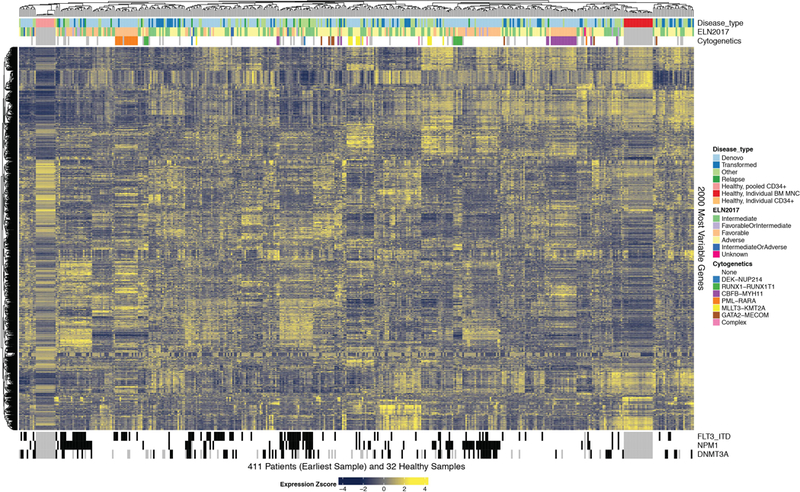
451 specimens from 411 unique AML patients were subjected to RNA-sequencing. The 2,000 genes with greatest differential expression across these unique AML patients are displayed on the heatmap. The heatmap is annotated with disease type, ELN risk stratification groups, genetic, and cytogenetic features of disease as noted in the figure legend.
Extended Data Fig. 3.

409 specimens from 363 unique AML patients were subjected to an ex vivo drug sensitivity assay whereby freshly isolated mononuclear cells from blood or bone marrow of patient specimens were incubated with graded concentrations of 122 small-molecule inhibitors (7 dose points in addition to the no drug control). Probit curve fits were used to compute drug response metrics, and the z-score of area under the dose response curve is plotted for each unique patient specimen against each drug. Drug sensitivity (blue) and resistance (red) are annotated by a color gradient, with grey indicating no drug data available. The heatmap is annotated at the top and bottom with major clinical, cytogenetic, and genetic features of disease as noted in the figure legend.
Extended Data Fig. 4.

The average inhibitor response AUCs for all cases that were de novo (n=288) versus all cases that transformed from a background of MDS (n=111) were compared for every inhibitor having at least 3 cases with evaluable data in each group. The middle point represents the average difference in AUC between the two groups with the bars representing the 95% confidence interval. For the sample size and statistical results of each drug/sample group correlation please see Supplementary Information (Table S20).
Extended Data Fig. 5.

To understand patterns of small-molecule sensitivity against prior annotation of each drug’s gene and pathway targets, drugs were placed into drug families according to target genes and/or pathways and the Pearson’s correlation value of each drug was plotted onto a clustered heatmap showing drugs with similar or dissimilar patterns of sensitivity across the patient cohort. Prior knowledge annotation of the drug families to which each drug could be assigned is shown to the right of the heatmap with alternating black and grey boxes/labels used to aid in tracking. Descriptions of each drug family as well as the n used to calculate each pairwise drug correlation are found in Supplementary Information (Table S11,21).
Extended Data Fig. 6
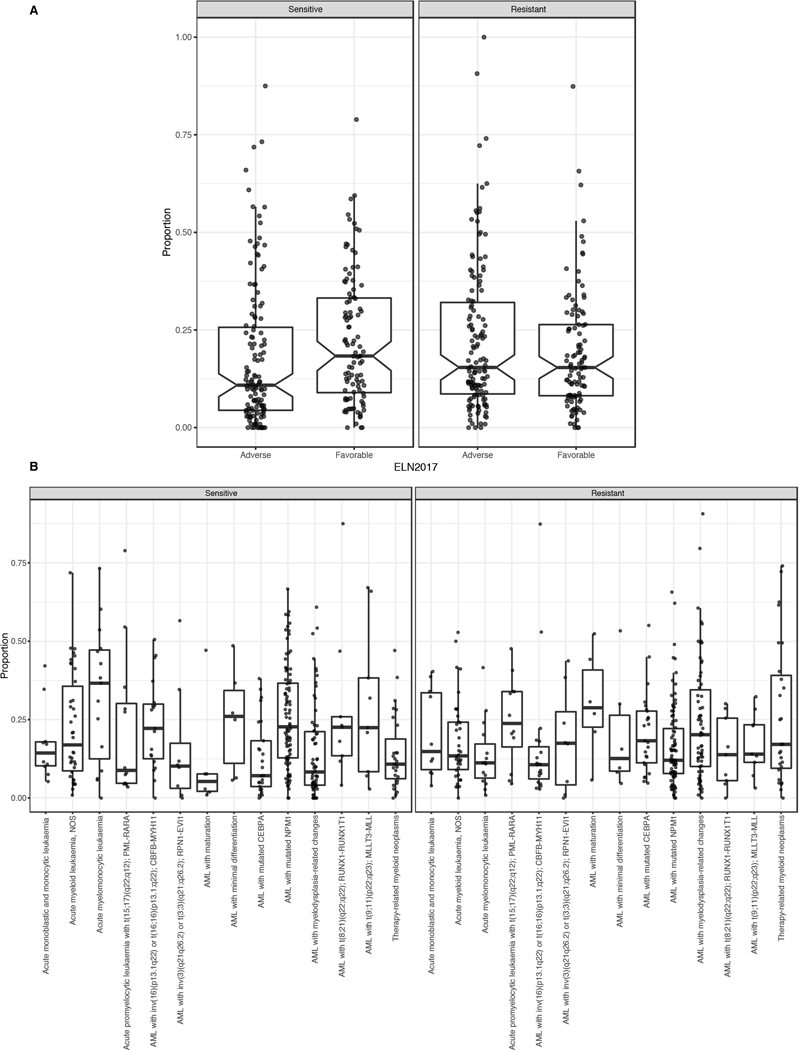
A. For the intersect of every specimen with evaluable response data for each inhibitor, we created a threshold for binary sensitive/resistant calls based on whether the individual specimen response fell within the most sensitive 20% of all specimens tested against that drug. A matrix plot showing the unsupervised clustering of the binary calls can be found at http://vizome.org/additional_figures_BeatAML.html. The binary drug resistance calls for each specimen were rolled up into a single value representing the proportion of drugs to which an individual specimen was sensitive (left) or resistant (right). Specimens were divided into Favorable and Adverse groups based on ELN 2017 criteria to determine whether overall drug sensitivity/resistance correlated with prognostic features of disease (n=233 patients).
B. The binary drug resistance calls for each specimen as in panel B. Specimens were divided into diagnostic groups based on WHO 2016 categories to determine whether overall drug sensitivity/resistance correlated with cytogenetic or morphologic features of disease (n=340 patients). For both A and B the upper and lower points of the boxplots show 1.5 times the interquartile range (IQR) from the upper/lower lines while the upper, middle and bottom lines indicate the 75th, median and 25th percentile of the data with the notches extending 1.58 * IQR / sqrt(n). Specific sample sizes of each group are reported in Supplementary Information (Table S22).
Extended Data Fig. 7.
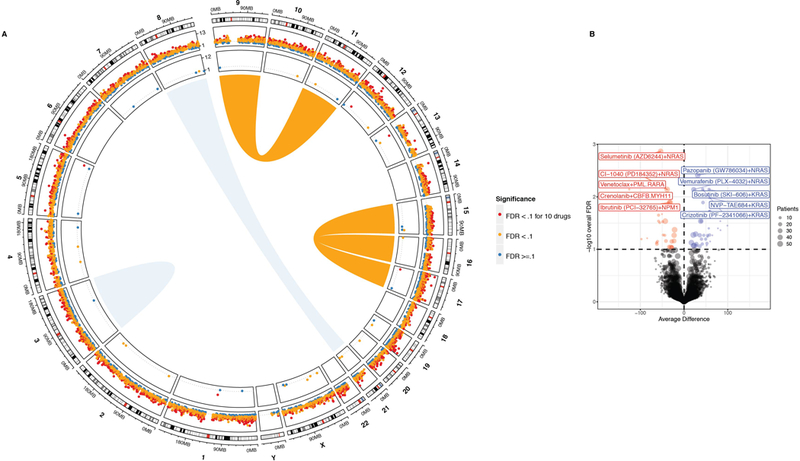
(A) Circos plot showing AML rearrangements in the center, mutational events in the next concentric ring, and gene expression events in the outer ring. The size/width indicates frequency of the event and FDR-corrected q-value of association with drug sensitivity is color-coded (sensitivity (red); resistance (blue)). For each gene, tests involving expression were two-sided t-tests (linear model) of the differences between sensitive and resistant samples. For mutational events, the average difference in AUC between mutant/wild type samples was determined using two-sided t-tests from a linear model as shown in Fig 2A. FDR was computed using the Benjamini-Hochberg over all the drugs. The n used to correlate each mutational event with drug sensitivity is reported in the Supplementary Information (Table S17).
(B) As in Fig. 2A, the average difference in AUC drug response between mutant and wild type cases was determined using a two-sided t-test from a linear model fit (plotted on the horizontal axis and FDR-corrected q-value is plotted on the vertical axis). The analysis here represents only FLT3-ITD negative cases. FDR was computed using the Benjamini-Hochberg (BH) over all the drugs. The n used to correlate each mutational event with drug sensitivity is reported in the Supplementary Information (Table S17). Expanded and interactive plots are available in our online data browser (www.vizome.org) and (http://vizome.org/additional_figures_BeatAML.html).
Extended Data Fig. 8.
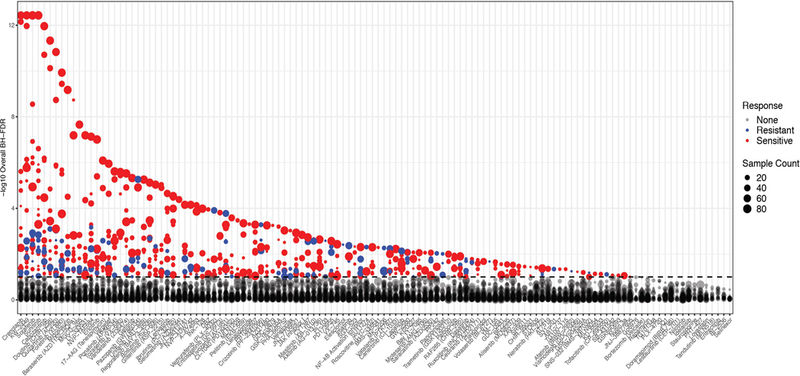
Correlation of drug sensitivity with mutational events. The average difference in AUC drug response between mutant and wild type cases was determined using a two-sided t-test from a linear model fit. FDR was computed using the BH over all the drugs. The degree of significance is represented on the vertical axis (sensitivity (red); resistance (blue)). The n used to correlate each mutational event with drug sensitivity is reported in the Supplementary Information (Table S17).
Extended Data Fig. 9.

A. Co-occurrences with regard to WGCNA gene expression clusters and/or mutational events (coefficients) were detected by multivariate modeling with respect to ibrutinib response (resistance (blue); sensitivity (red)) with degree of correlation quantified in top track stacked barplot. All coefficients that appear in 25% of the bootstrap sample sets are shown as segments of the circle. Segment width (the colored ring) corresponds to the percentage of bootstrap samples in which that coefficient appears (quantified above the dotted line). The variables appear in descending order clockwise starting at 12 o’clock. Each link indicates pairwise co-occurrence of mutational events and gene expression clusters (width represents frequency of the co-occurrence). The largest co-occurrence for each coefficient is quantified.
B. The capacity of differential gene expression to distinguish the 20% most ibrutinib sensitive (n=46) from 20% most resistant (n=44) specimens is shown on a principle component plot (n=239 patient samples total tested for ibrutinib sensitivity and RNA-Sequencing).
For the n used to correlate each drug with gene expression and perform Lasso regression, please see Supplementary Information (Table S17).
Supplementary Material
2
SI Guide
Supplementary Tables S1-S22
Acknowledgements
We thank all of our patients at all sites for donating precious time and tissue. DNA and RNA quality assessments, library creation, and short read sequencing assays were performed by the OHSU Massively Parallel Sequencing Shared Resource. Sheenu Sheela, Catherine Lai, Katherine Lindblad and Kary Oetjen assisted in study coordination at NIH. Barry Sawicki and Christina Cline assisted in study coordination at the University of Florida. S. Ravencroft assisted with patient sample shipping and data entry and K. Schorno provided project management and support of activities at the University of Kansas Cancer Center. Jack Taw assisted with patient sample shipping and Shyam Patel assisted with data entry at Stanford University.
Funding Funding for this project was provided in part by a Leukemia Lymphoma Therapy Acceleration Grant to B.J.D. and J.W.T. and by support provided by the Knight Cancer Research Institute (Oregon Health & Science University, OHSU). Supported by grants from the National Cancer Institute (1U01CA217862, 1U54CA224019, 3P30CA069533–18S5) and NIH/NCATS CTSA UL1TR002369 (SKM, BW). ASB was supported by the National Library of Medicine Informatics Training Grant (T15LM007088). JWT received grants from the V Foundation for Cancer Research, the Gabrielle’s Angel Foundation for Cancer Research, and the National Cancer Institute (1R01CA183947). CRC received a Scholar in Clinical Research Award from The Leukemia & Lymphoma Society (2400–13), was distinguished with a Pierre Chagnon Professorship in Stem Cell Biology and Blood & Marrow Transplant and a UF Research Foundation Professorship. This work was supported in part by the Intramural Research Program of the National Heart, Lung, and Blood Institute of the National Institutes of Health.
The authors declare competing financial interests detailed below.
Competing Interests JWT receives research support from Agios, Aptose, Array, AstraZeneca, Constellation, Genentech, Gilead, Incyte, Janssen, Seattle Genetics, Syros, Takeda; JWT is a co-founder of Vivid Biosciences. MWD serves on the advisory boards and/or as a consultant for Novartis, Incyte, and BMS and receives research funding from BMS and Gilead. CSH receives research funding from Merck. TLL consults for Jazz Pharmaceuticals and receives research funding from Tolero, Gilead, Precient, Ono, Bio-Path, Mateon, Genentech/Roche, Trovagene, Abbvie, Pfizer, Celgene, Imago, Astellas, Karyopharm, Seattle Genetics, and Incyte. DAP receives research funding from Pfizer and Agios and served on advisory boards for Pfizer, Celyad, Agios, Celgene, AbbVie, Argenx, Takeda and Servier. BJD serves on the advisory boards for Gilead, Aptose, and Blueprint Medicines. BJD is principal investigator or coinvestigator on Novartis and BMS clinical trials. His institution, Oregon Health & Science University, has contracts with these companies to pay for patient costs, nurse and data manager salaries, and institutional overhead. He does not derive salary, nor does his laboratory receive funds from these contracts. The authors certify that all compounds tested in this study were chosen without input from any of our industry partners.
Footnotes
Data Availability Statement
All raw and processed sequencing data, along with relevant clinical annotations have been submitted to dbGaP and Genomic Data Commons. The dbGaP study ID is 30641 and accession ID is phs001657.v1.p1. The raw data for clinical annotations, variant calls, gene expression counts, and drug sensitivity that underlie all figures in this manuscript are found in the Supplementary Information. In addition, all data can be accessed and queried through our online, interactive user interface, Vizome, at www.vizome.org.
Supplementary Information is available in the online version of the paper.
See also Supplementary Information for listing of authors, affiliations, and contributions
REFERENCES
Full text links
Read article at publisher's site: https://doi.org/10.1038/s41586-018-0623-z
Read article for free, from open access legal sources, via Unpaywall:
https://europepmc.org/articles/pmc6280667?pdf=render
Citations & impact
Impact metrics
Article citations
Lymphoma and Leukemia Cell Vulnerabilities and Resistance Identified by Compound Library Screens.
Methods Mol Biol, 2865:259-272, 01 Jan 2025
Cited by: 0 articles | PMID: 39424728
In vivo models of subclonal oncogenesis and dependency in hematopoietic malignancy.
Cancer Cell, 42(11):1955-1969.e7, 01 Nov 2024
Cited by: 0 articles | PMID: 39532065
Single-cell landscape of innate and acquired drug resistance in acute myeloid leukemia.
Nat Commun, 15(1):9402, 30 Oct 2024
Cited by: 0 articles | PMID: 39477946 | PMCID: PMC11525670
The present and future of the Cancer Dependency Map.
Nat Rev Cancer, 28 Oct 2024
Cited by: 0 articles | PMID: 39468210
Review
A View of Myeloid Transformation through the Hallmarks of Cancer.
Blood Cancer Discov, 5(6):377-387, 01 Nov 2024
Cited by: 0 articles | PMID: 39422551
Review
Go to all (674) article citations
Data
Data behind the article
This data has been text mined from the article, or deposited into data resources.
BioStudies: supplemental material and supporting data
dbGaP - The database of Genotypes and Phenotypes
- (1 citation) dbGaP - phs001657
Similar Articles
To arrive at the top five similar articles we use a word-weighted algorithm to compare words from the Title and Abstract of each citation.
Whole-exome sequencing identifies somatic mutations of BCOR in acute myeloid leukemia with normal karyotype.
Blood, 118(23):6153-6163, 19 Oct 2011
Cited by: 163 articles | PMID: 22012066
Genomics in acute myeloid leukemia: from identification to personalization.
R I Med J (2013), 98(11):27-30, 02 Nov 2015
Cited by: 1 article | PMID: 26517252
DNMT3A mutations are over-represented in young adults with NPM1 mutated AML and prompt a distinct co-mutational pattern.
Leukemia, 33(11):2741-2746, 17 Jun 2019
Cited by: 14 articles | PMID: 31209279
Perspectives for therapeutic targeting of gene mutations in acute myeloid leukaemia with normal cytogenetics.
Br J Haematol, 170(3):305-322, 19 Apr 2015
Cited by: 24 articles | PMID: 25891481
Review
Funding
Funders who supported this work.
Howard Hughes Medical Institute
NCATS NIH HHS (4)
Grant ID: KL2 TR000152
Grant ID: UL1 TR002369
Grant ID: KL2 TR002370
Grant ID: UL1 TR002366
NCI NIH HHS (6)
Grant ID: U54 CA224019
Grant ID: R01 CA214428
Grant ID: K99 CA237630
Grant ID: P30 CA069533
Grant ID: R01 CA183974
Grant ID: U01 CA217862
NIAID NIH HHS (1)
Grant ID: R37 AI110985
NLM NIH HHS (1)
Grant ID: T15 LM007088