Abstract
Free full text

Neural connectivity predicts spreading of alpha-synuclein pathology in fibril-injected mouse models: Involvement of retrograde and anterograde axonal propagation
Associated Data
Abstract
In Parkinson’s disease, some of the first alpha-synuclein aggregates appear in the olfactory system and the dorsal motor nucleus of the vagus nerve before spreading to connected brain regions. We previously demonstrated that injection of alpha-synuclein fibrils unilaterally into the olfactory bulb of wild type mice leads to widespread synucleinopathy in brain regions directly and indirectly connected to the injection site, consistently, over the course of periods longer than 6 months. Our previously reported observations support the idea that alpha-synuclein inclusions propagates between brain region through neuronal networks. In the present study, we further defined the pattern of propagation of alpha-synuclein inclusions and developed a mathematical model based on known mouse brain connectivity. Using this model, we first predicted the pattern of alpha-synuclein inclusions propagation following an injection of fibrils into the olfactory bulb. We then analyzed the fitting of these predictions to our published histological data. Our results demonstrate that the pattern of propagation we observed in vivo is consistent with axonal transport of alpha-synuclein aggregate seeds, followed by transsynaptic transmission. By contrast, simple diffusion of alpha-synuclein fits very poorly our in vivo data. We also found that the spread of alpha-synuclein inclusions appeared to primarily follow neural connections retrogradely until 9 months after injection into the olfactory bulb. Thereafter, the pattern of spreading was consistent with anterograde propagation mathematical models. Finally, we applied our mathematical model to a different, previously published, dataset involving alpha-synuclein fibril injections into the striatum, instead of the olfactory bulb. We found that the mathematical model accurately predicts the reported progressive increase in alpha-synuclein neuropathology also in that paradigm. In conclusion, our findings support that the progressive spread of alpha-synuclein inclusions after injection of protein fibrils follows neural networks in the mouse connectome.
1. Introduction
Parkinson’s disease is characterized neuropathologically both by death of substantia nigra dopamine neurons and the progressive accumulation of pathological inclusions in widespread parts of the nervous system (Goedert et al., 2017). These inclusions, named Lewy bodies and Lewy neurites, are mainly composed of misfolded alpha-synuclein (αsyn) (Goedert, 2001; Goedert et al., 2017) and they engage an increasing number of brain regions as the disease progresses over several years. This spreading of Lewy pathology in the brain follows a stereotypical pattern and has been suggested to first start in the olfactory system and the dorsal motor nucleus of the vagus (Braak et al., 2003a; Braak et al., 2003b; Braak and Del, 2017; Braak and Del, 2016; Braak et al., 2004; Del Tredici and Braak, 2016). The brain regions that sequentially develop Lewy pathology in PD are all interconnected, an observation that provided some of the scientific premise for the hypothesis that αsyn aggregates can propagate from one neuron to another via neural connections (Angot et al., 2010; Braak et al., 2003a; Brundin et al., 2008; Hawkes et al., 2007).
Extensive work using in vitro models has subsequently demonstrated that misfolded αsyn can act as a template and impose its aberrant conformation to native αsyn, and that the seeds of misfolded αsyn can be released and taken up by neighboring cells that in turn develop αsyn inclusions (Brundin and Melki, 2017; Grozdanov and Danzer, 2018; Steiner et al., 2011; Tyson et al., 2016). Thus, misfolded αsyn itself could be a vector of propagation and could underlie a propagation of synucleinopathy between brain regions in humans (Brundin and Melki, 2017; Rey et al., 2018b). To address this hypothesis, several groups have tested the seeding and prion-like spreading of pathological αsyn in vivo (Dehay and Fernagut, 2016; Dehay et al., 2016; Rey et al., 2018b). Intracerebral or peripheral injection of recombinant αsyn fibrils or αsyn aggregates derived from brain lysates from post-mortem patients with synucleinopathy can induce αsyn aggregation in both animals overexpressing αsyn and in wild type animals (Brundin and Melki, 2017; Dehay et al., 2016; Luk and Lee, 2014; Recasens et al., 2018; Rey et al., 2018b).
Several previously published studies have focused on defining the development of abnormal αsyn inclusions following injections into the striatum or in the substantia nigra, which exhibit synucleinopathy only in advanced PD. By contrast, recent reports from our group have focused on triggering synucleinopathy in the olfactory bulb because it is believed to be one of the first brain regions affected in PD (Rey et al., 2018a, 2013, 2016b, 2018b). By unilateral stereotaxic injection of recombinant αsyn fibrils into the olfactory bulb of wild type mice, we have demonstrated αsyn aggregation is initially triggered at the site of injection and then it progressively spreads sequentially to interconnected brain regions (Rey et al., 2018a, 2016b). Our published observations strongly suggest propagation via a neuronal network, but they do not completely rule out other spreading routes such as local diffusion, or migration of glia containing seeds (Rey et al., 2016a). Indeed, some reviews of the literature to date on αsyn pathology spread question whether the propagate via neural connectivity (Steiner et al., 2011) and suggest that given inconsistencies in the transsynaptic spread literature, perhaps cell-type specific explanations might provide a better explanation (Surmeier et al., 2017b) for observed pathology proliferation patterns.
To further define the anatomical patterns and dynamics of the spread of αsyn inclusions, and thereby gain greater insight into the role of neural connections, here we reanalyze our own data with a specific focus on network transmission of inclusions. For this purpose, we use our recently developed mathematical model called Directional Network Transmission (DNT) (Mezias et al., 2017; Mezias and Raj, 2017), which we have previously demonstrated can predict tau pathology transmission in the mouse brain, but does not well represent aβ progression. In turn, this model was based on our original Network Diffusion model of human degenerative progression (Raj et al., 2012, 2015), which was successful in recapitulating the spatiotemporal pattern of atrophy in human dementia. These mathematical models provide a useful new way of testing hypotheses regarding network transmission since they do not rely on correlative evidence from isolated regions or fiber projections, but can be applied to the entire brain connectome at once. This addresses a gap in the current research regarding the relationship between neural connectivity and αsyn spread, as there is presently a lack of studies elucidating the relationship between the whole brain connectome and αsyn inclusions patterns (Surmeier et al., 2017b). In addition, while both anterograde and retrograde transmission of αsyn have been observed in cell culture models (Freeze et al., 2018; Volpicelli-Daley et al., 2011) the issue of directional bias in αsyn pathology transmission remains controversial (Bieri et al., 2018; Freundt et al., 2012; Uchihara and Giasson, 2016). Accordingly, we undertook the current study to investigate whether the longitudinal evolution of αsyn inclusions and direction of propagation can be predicted by directional trans-neuronal network transmission based on the anatomic network connectivity (or “connectome”) of the mouse.
Using the DNT model and mouse connectome, we studied propagation of αsyn inclusions from the olfactory bulb over time and analyzed the fitting of these predictions to our published in vivo data (Rey et al., 2018a, 2016b). Our work demonstrates that the model of propagation via neuronal networks fits the best with our published in vivo observations. Our work also confirms that a spatial diffusion model fits very poorly with our in vivo data. We also found that a retrograde spreading of inclusions during the first months after injection of αsyn fibrils followed by the involvement of anterograde progression explains with the pattern of inclusions propagation we observe after triggering synucleinopathy in the olfactory bulb. In addition, we applied our DNT model to two additional models of propagation: our dataset based on striatal injections of PFFs (Chatterjee et al., 2019) and a published dataset from a model of intra-nigral injection of alpha-synuclein fibrils (Masuda-Suzukake et al., 2013).
2. Methods
Our previously published work supported the idea that αsyn pathology propagates along axonal pathways, but we could only provide correlative evidence. Therefore, we further analyzed the propagation of αsyn-inclusions from the olfactory bulb in wild type mice. To this end, we developed a model of the theoretical pattern of propagation based on different propagation mechanisms (spatial proximity-based propagation by diffusion; connectivity-based propagation along fiber tracts in anterograde or retrograde directions). We implemented this theoretical model using published data on the mouse connectivity network and compared the fitting of our theoretical models to our in vivo observations.
2.1. Mouse brain connectivity network
We use data from the Allen Institute for Brain Science’s Mouse Connectivity Atlas (MCA) to create the mouse connectivity network. This network is derived from viral tracing studies and contains fully directional connectivity intensity information from 426 regions across both hemispheres; more information on the MCA can be found at the Allen Institute’s website and in the citation (Oh et al., 2014). The network we use here can be obtained either on the Allen Institute’s website in the ‘Mouse Connectivity Atlas’ section or in Supplemental Materials attachment #4 from the above cited paper (Oh et al., 2014).
2.2. Mouse experiments and data collection for original synucleinopathy dataset (propagation of synucleinopathy from the olfactory bulb)
We injected C57/Bl6 wild type mice unilaterally into the olfactory bulb with αsyn pre-formed fibrils (PFFs) made of recombinant wild-type mouse αsyn PFFs (mPFFs) or wild-type human αsyn PFFs (huPFFs). The mice were sacrificed via transcardial perfusion with 4% paraformaldehyde in groups at either 1, 3, 6, 9, 12, or 18 months post-injection. The entire brains were sectioned coronally on a microtome into 30 μm thick sections in 8 series. Free-floating sections were stored in cryoprotectant until use. We stained every 8th section of the entire brain with Ab51253 (Abcam) for αsyn phosphorylated on Serine 129, a marker of pathological αsyn (Beach et al., 2009; Rey et al., 2016a, 2018a, 2016b). Endogenous peroxidase were blocked by incubation with 3% H2O2 for 20 min. Sections were then blocked in 10% normal goat serum, 0.3% triton x100, 0.4% Bovine serum albumin; followed by overnight incubation with rabbit anti pser129 antibody (1/10000 in 2% normal goat serum, 0.3% triton X100). We incubated the sections with secondary antibody goat anti-rabbit biotinylated (1:500, Vector lab) in blocking solution. Sections were then incubated for 1 h in ABC (ABC Kit, Vector lab) and revealed using DAB kit (Vector lab).
These sections were blind coded and were analyzed at x20 using Nikon eclipse NieU microscope. We semi-quantitatively rated αsyn inclusions on a 0–4 scale (one score per brain region) based on relative abundance of pser129 inclusions: 0 = no aggregates; 1 = sparse: very few neurites, max 1 soma; 2 = mild inclusions; 3 = dense inclusions; 4 = very dense inclusions) for 4 mice per group at 1 month post-injection, 4 mPFF and 3 huPFF injected mice at 3 months, 5 mPFF and 4 huPFF injected mice at 6 months, 3 mPFF and 5 huPFF injected mice at 9 months, 7 mPFF and 5 huPFF injected mice at 12 months, and 5 huPFF and 3 mPFF injected mice at 18 months. Mouse brain regions were manually identified using the Paxinos and Franklin mouse brain atlas (Paxinos and Franklin, 2012). The list of the brain regions where αsyn inclusions were detected can be found in the original publications (Rey et al., 2018a; Rey et al., 2016b). Importantly, the staining conditions used would detect only dense pser129-positive inclusions and we have shown previously (Rey et al., 2018a; Rey et al., 2016b) that these inclusions shared the markers for pathological αsyn aggregates (colo-calization with p62, ubiquitin and Thioflavin-S positive). During the scoring, any weak homogenously stained cell bodies were excluded from analysis to semi-quantify only dense inclusions.
Further details on mouse experiment methodology for this data can be found in the same original publications (Rey et al., 2018a; Rey et al., 2016b). It should be noted that data from timepoints up to 12 months post-injection (Rey et al., 2016b) and the dataset obtained at 18 months post-injection (Rey et al., 2018a) were quantified separately and some subregions were grouped differently. Thus, the datasets were not directly comparable, and so r, Δr, and p-values cannot be directly compared. Instead we observe that the pattern of results produced by comparing the 18-month dataset with our modeled predictions remains consistent with the suggested pattern of increasingly heavy involvement of anterograde transit along fiber tracts as a driver of αsyn inclusions propagation. We still include the 18-month data with other quantified timepoints in Fig. 5 as this is meant to be illustrative of the pattern of results we see. Our more in-depth analyses separate the data up to 12-months (Figs. 2 & 3) and at 18-months (Fig. 4) post-injection into different figures.
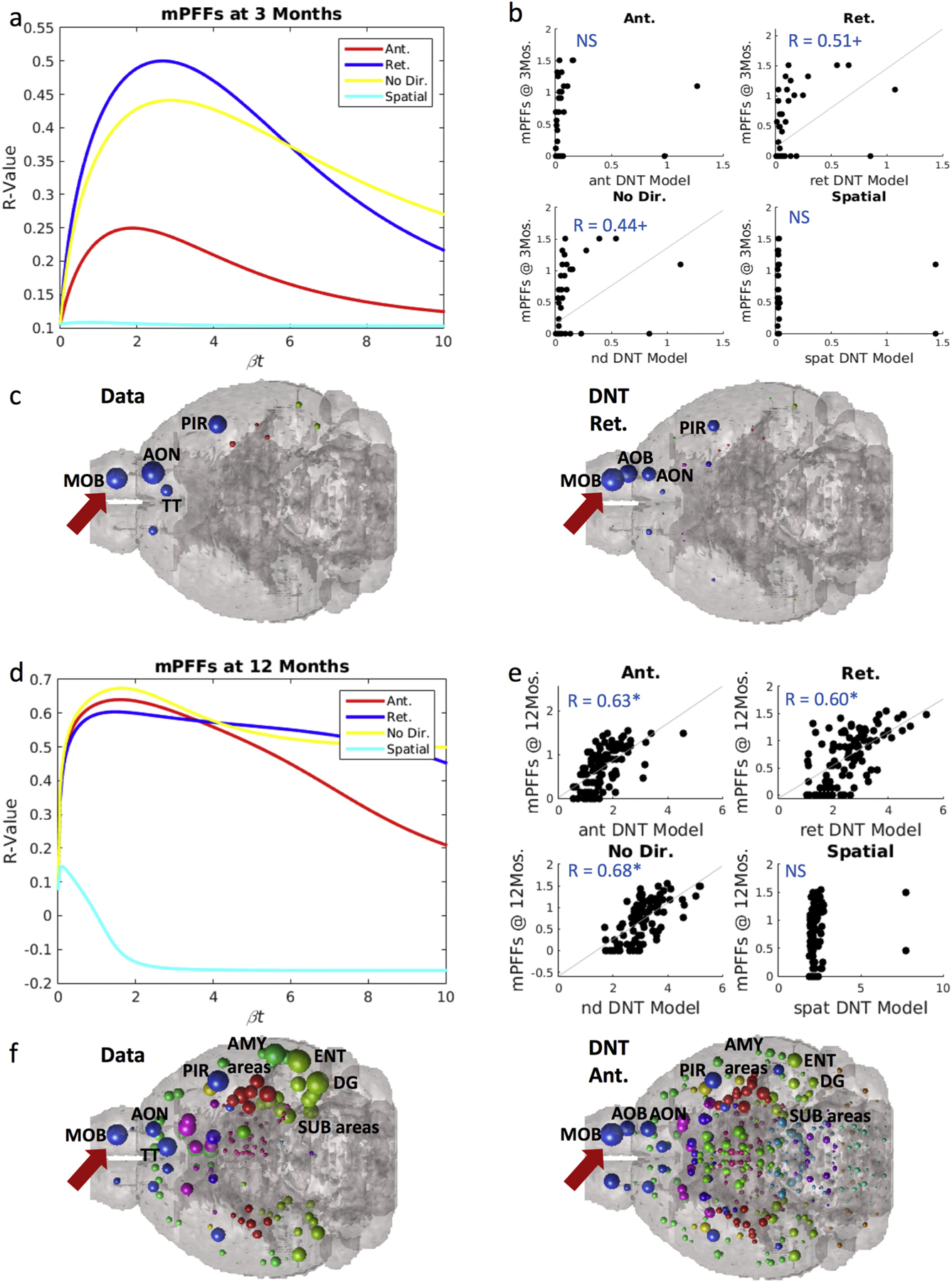
Synuclein inclusion spread is better modeled by retrograde DNT at early timepoints, and anterograde DNT at later timepoints. (a) βt-curve showing NT models’ r-value with mPFF data at 3-months post-injection at different diffusion time constants. (b) Scatterplot of the NT models at their peak β-diffusion time constant, as in (a) to the left, versus data. (c) Anatomical illustrations of mPFF synuclein inclusion data and DNT-retrograde simulation. (d) βt-curve showing NT models’ r-value with mPFF data at 12-months post-injection at different diffusion time constants. (e) Scatterplot of the NT models at their peak β-diffusion time constant, as in (d) to the left, versus data. (f) Anatomical illustrations of mPFF induced synuclein inclusions at 12 months post injection and the corresponding anterograde-DNT simulation. AMY = amygdala, AOB = accessory olfactory bulb, AON = anterior olfactory nucleus, DG = dentate gyrus, ENT = entorhinal cortex, MOB = main olfactory bulb, PIR = piriform cortex, SUB = subiculum, TT = tenia tecta. Please see Fig. 1 for color legend for the major region color scheme of the balls denoting regional αsyn inclusions. The red arrow points toward the MOB where the PFFs were injected. Significance level: + p < .01, * p < .02. (For interpretation of the references to color in this figure legend, the reader is referred to the web version of this article.)
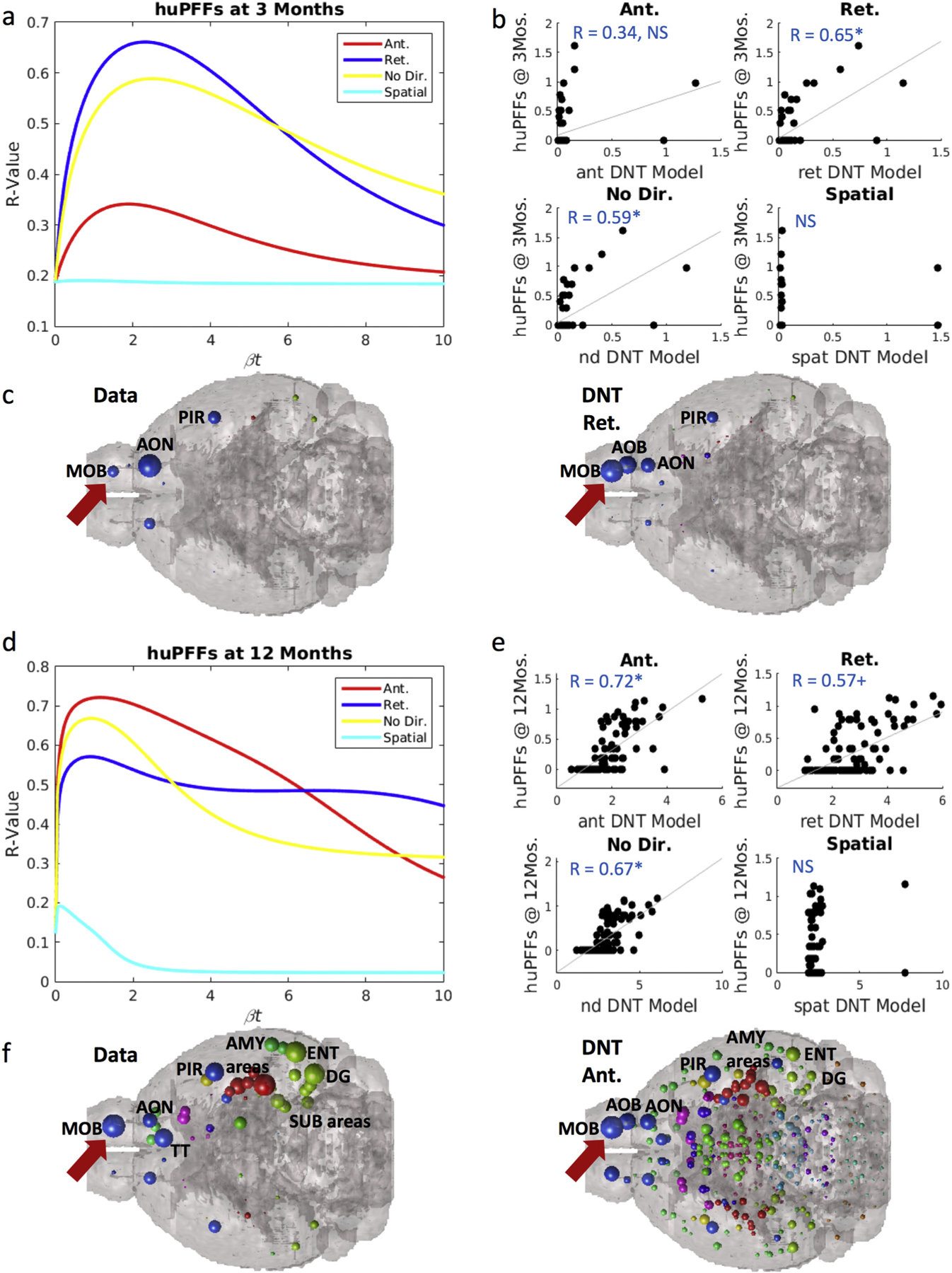
Synuclein inclusion spread is better modeled by retrograde DNT at early timepoints, and anterograde DNT at later timepoints. (a) βt-curve showing NT models’ r-value with huPFF data at 3-months post-injection at different diffusion time constants. (b) Scatterplot of the NT models at their peak β-diffusion time constant, as in (a) to the left, versus data. (c) Anatomical illustrations of huPFF synuclein inclusion data and DNT-retrograde simulation. (d) βt-curve showing NT models’ r-value with huPFF data at 12-months post-injection at different diffusion time constants. (e) Scatterplot of the NT models at their peak β-diffusion time constant, as in (d) to the left, versus data. (f) Anatomical illustrations of huPFF induced synuclein inclusions at 12 months post injection and the corresponding anterograde-DNT simulation. All data and NT simulation values are log-transformed prior to both statistics and anatomical visualizations. AMY = amygdala, AOB = accessory olfactory bulb, AON = anterior olfactory nucleus, DG = dentate gyrus, ENT = entorhinal cortex, MOB = main olfactory bulb, PIR = piriform cortex, SUB = subiculum, TT = tenia tecta. Please see Fig. 1 for color legend for the major region color scheme of the balls denoting regional αsyn inclusions. The red arrow points toward the MOB where the PFFs were injected. + p < .01, * p < .02. (For interpretation of the references to color in this figure legend, the reader is referred to the web version of this article.)
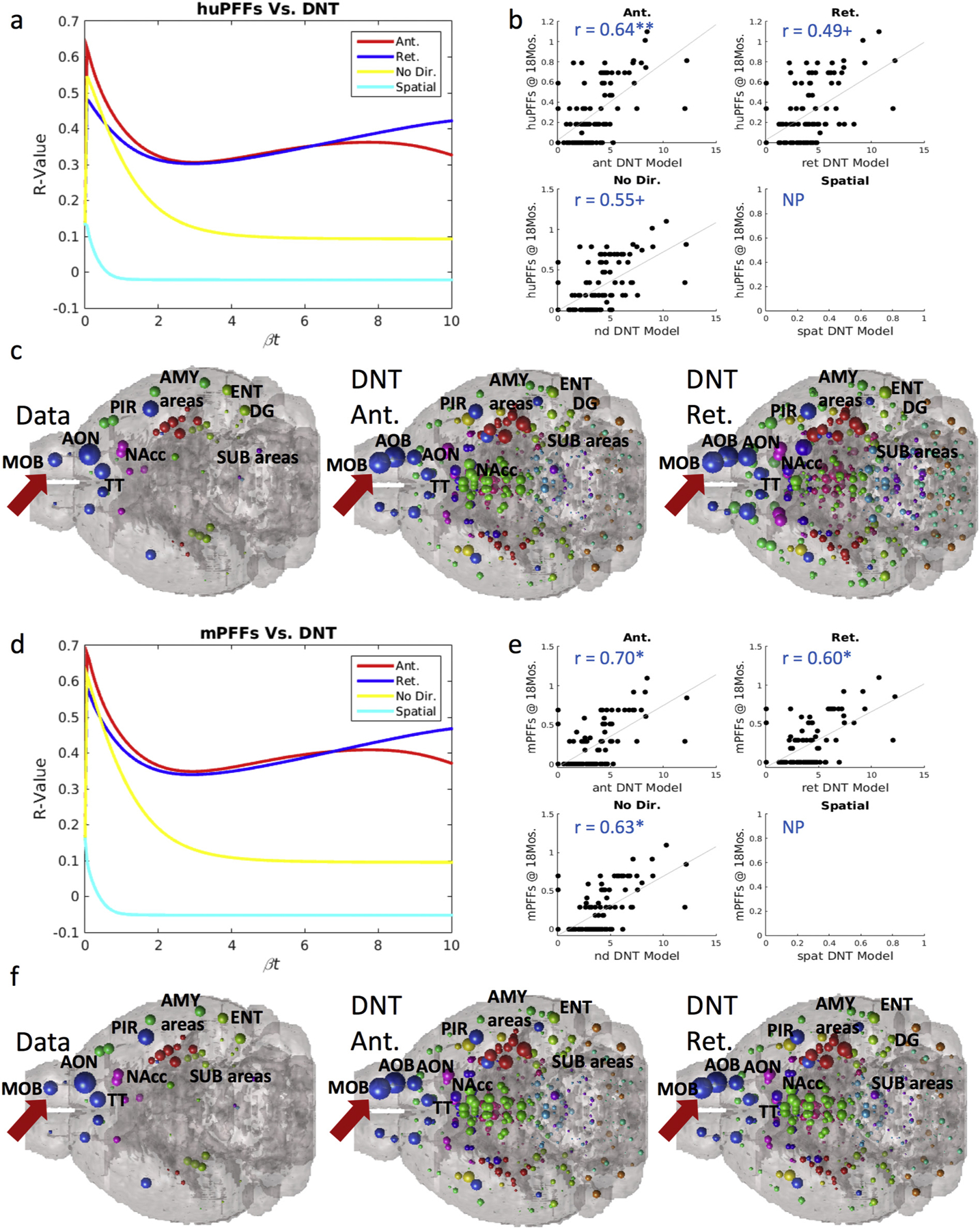
At 18-months post injection, we find the same phenomenon of strong inclusion pattern recreation by our anterograde DNT model at timepoints far from injection, and in contrast with findings at earlier measured timepoints. (a) βt-curve showing NT models’ r-value with huPFF data at 18-months post-injection at different diffusion time constants. (b) Scatterplots for each model’s prediction at the βt diffusion rate constant that maximizes r-values with regional αsyn inclusion data from huPFF injected mice. (c) An anatomical illustration of regional αsyn inclusion pattern data and anterograde and retrograde DNT model predictions for huPFF injected mice. (d) βt-curve showing NT models’ r-value with mPFF data at 18-months post-injection at different diffusion time constants. (e) Scatterplots for each model’s prediction at the βt diffusion rate constant that maximizes r-values with regional αsyn inclusion data from mPFF injected mice. (f) An anatomical illustration of regional αsyn inclusion pattern data and anterograde and retrograde DNT model predictions for mPFF injected mice. AMY = amygdala, AOB = accessory olfactory bulb, AON = anterior olfactory nucleus, DG = dentate gyrus, ENT = entorhinal cortex, MOB = main olfactory bulb, NAcc = nucleus accumbens, PIR = piriform cortex, SUB = subiculum, TT = tenia tecta. Please see Fig. 1 for color legend for the major region color scheme of the balls denoting regional αsyn inclusions. The red arrow points toward the MOB where the PFFs were injected. + p < .01, * p < .02. (For interpretation of the references to color in this figure legend, the reader is referred to the web version of this article.)
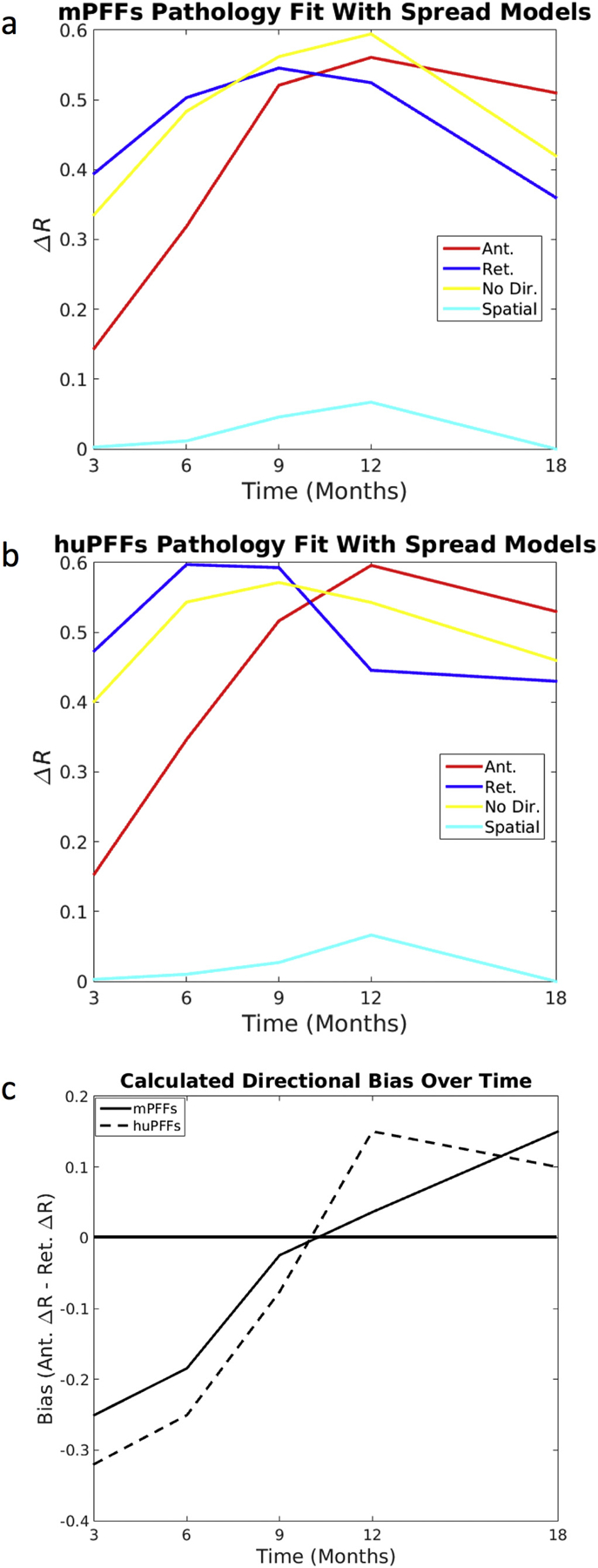
DNT retrograde models are better predictors of synuclein inclusions closer to initial pathological injection, but anterograde DNT is a better predictor of synuclein inclusions as more time elapses following injection. (a) mPFF synuclein injected mice and (b) huPFF synuclein injected mice both show spatiotemporal synucleinopathy development such that at times closer to the initial injection, retrograde DNT is the best predictor, but at times farther from the injection, anterograde DNT better recapitulates αsyn inclusion spread than does retrograde DNT. (c) Calculated directional bias in the fit with data between anterograde and retrograde DNT models shifts toward anterograde DNT over time. A reference line at 0, the value at which there should be no directional bias, is added to the plot in (c). All data and NT simulation values are log-transformed prior to running statistics.
2.3. Mouse experiments and data collection for original dataset from intrastriatal injections of PFFs
For striatal injections, human alpha-synuclein was purified and assembled into PFFs in house following previously published protocols (Luk et al., 2012; Volpicelli-Daley et al., 2015). PFFs were thawed and sonicated as previously published (Rey et al., 2018a; Rey et al., 2016b). 4 week old OF1-Swiss mice were injected stereotactically 2ul of PFFs unilaterally into the dorsal striatum as previously described (striatum coordinates from bregma: AP; +0.2 mm, ML:−2.0 mm, DV: −2.6 mm). Mice were euthanized 8 weeks post injection by deep anesthesia with sodium pentobarbital and perfused transcardially with 0.9% saline, then 4% paraformaldehyde (PFA) in phosphate buffer. We collected the brains and post-fixed them for 24 h in 4% PFA. Brains were then stored in 30% sucrose in phosphate buffer at 4°C until use. Brains were sectioned coronally into 40 um thick sections on a freezing microtome. Free floated sections were stored in cryoprotectant.
The following staining protocol and semiquantitative analyses were employed: Pser129 inclusions was semi-quantified across almost 40 regions, at one timepoint 8 weeks post-injection with in house recombinant human PFFs. The semi-quantification in this study was based on immunohistochemistry and followed the same procedure for converting IHC signal to semi-quants as in (Rey et al., 2018a, 2016b). While this dataset is only cross-sectional rather than longitudinal, as it only contains one timepoint, it contains data from each individual mouse. With this dataset we accordingly assessed whether there was an identifiable directional predilection, according to our DNT modeling, in αsyn spread. The citation for this dataset can be found in (Chatterjee et al., 2019).
2.4. Mouse synucleinopathy datasets obtained from other sources (intranigral injections of fibrils)
To further investigate the propagation of abnormal inclusions in regards to theoretical propagation along axonal network, we chose to apply our connectivity model to another published dataset: Masuda-Suzukake, et al., 2013 (Masuda-Suzukake et al., 2013). Similar to our previous work (Rey et al., 2018a; Rey et al., 2016b), this study used immunohistochemistry as the basis for all quantifications or semi-quantifications and wild-type mice. In the study by Masuda-Suzukake et al., (Masuda-Suzukake et al., 2013) mice were injected into the substantia nigra with recombinant αsyn fibrils, and at least 40 separate brain regions were quantified for αsyn inclusions. The investigators semi-quantitatively assessed αsyn pathology by grading regional severity out to 15 months post-injection using 3 months and 6 months as intermediary timepoints.
2.5. Connectivity metrics, NT, and DNT
Connectivity metrics are calculated based on the topology of the mouse brain network derived from the MCA, where connectivity is represented as “outgoing” along the rows and “incoming” along the columns (Oh et al., 2014). We generated a directed and weighted connectivity measure with seed regions by summing the weighted row-wise or column-wise values in the MCA at the seed nodes. We could then correlate each selected region’s measured αsyn inclusions with its weighted connectivity, in both anterograde and retrograde directions, with the seed nodes in each study. Further information on using the MCA and its connectivity data for graph analyses can be found at the original citation (Oh et al., 2014) and in our prior paper using this atlas (Mezias et al., 2017).
NT and DNT modeling is similarly dependent on the MCA in this study, and on regional graph adjacency to the seed nodes or nodes already containing inclusions. We first calculate the Normalized Adjacency or Laplacian Matrix, L, given by the equation below:
where C is the connectivity matrix from the MCA, I is the identity matrix, and DR and DC are the row and column wise diagonal matrices from the MCA. Previous graph theoretic work has characterized diffusion over a network from defined seed points and has been shown to be predictive for both volumetric loss (Freeze et al., 2018; Raj et al., 2012) and metabolic dysfunction (Raj et al., 2015) in patients with AD or other tauopathic dementia, as well as for mouse models of tauopathy and amyloidopathy (Mezias et al., 2017; Mezias and Raj, 2017):
here L is the Laplacian Matrix, βt is a constant representing diffusion time determined by when it maximizes ΔR, which is the change in R-value from βt = 0 to maximum, X(0) is a vector with n number of nodes length representing seeding, and X(t) is a vector with n number of nodes length representing the final pattern of network diffusion. Further information on the derivation of the above equation and graph diffusion modeling can be found at the aforementioned citations.
In the present study, we use a modified version of the above NT model equation so that we could both capture the directionality information from the MCA allowing us to represent synucleinopathy as not only a process of spread but also of accumulation, analogous to a prion-like disorder. We captured the directionality information from the MCA by calculating different networks for each direction, where C represents the standard connectivity network, CT represents the network in the retrograde direction, and the summed connectivity of both, C + CT, represents the undirected network derived from the MCA. Here we use an integrative or summative approach to capture accumulation and so the equation can be represented in two manners:
More information on the mathematics behind this approach can be found in our previous work (Mezias et al., 2017; Mezias and Raj, 2017; Raj et al., 2015). The Spatial diffusion model (SPD) uses the same Laplacian and model equation, (2), from above; the difference between NT or DNT models and the SPD model is that the SPD model, as its network, instead utilizes the Cartesian distances, in voxels, between the centers of mass of any given region pair, rather than their axonal connectivity.
2.6. Data analyses
All analyses performed were a variant of a standard linear regression using natural log transformed regressions due to empirical pathology and predicted values being best fit by exponential distributions. As in our previous studies (Mezias et al., 2017; Mezias and Raj, 2017), we assessed each model by considering the Pearson’s R-statistic of the correlation between the (D)NT model, evaluated at all model times βt, and the empirical regional distribution of synucleinopathy concentration from the mouse brains. The model time βt is not directly knowable form the model, and hence we chose to record the maximum correlation achieved by the model at all possible model times. We collected two quantities at the peak correlation: the Pearson R-value, as well as the ΔR-value, here defined as the difference between the maximum R-value, and the R-value achieved by the model at βt = 0, i.e. the synuclein inclusions seed pattern. The latter is a relevant measure of model performance, since it indicates how much additional value is added by network transmission beyond the initial seeding pattern. To determine directional bias, we subtracted retrograde DNT ΔR-values from anterograde DNT ΔR-values, as given by Eq. (3):
A calculated bias value of 0 using Eq. (3) would indicate no bias, a value > 0 would indicate a bias in the anterograde direction, and a value < 0 would indicate a bias in the retrograde direction. To test for the effects of progression time on directional bias in αsyn propagation we correlated each measured timepoint’s retrograde and anterograde bias, across all studies, and correlated that with the time of measurement (in months) after study initiation. We also correlated the bias difference scores, as calculated above, across timepoints. Because we present 24 total correlations, when assessing for significance, we corrected the α-value threshold for significance, originally at p < .05, by dividing it by 24 to yield a significance cutoff at p ≤ .002. We analyzed the striatal injection dataset from (Chatterjee et al., 2019) using two-sample t-tests to compare r-values across all data using anterograde versus retrograde biased DNT. All statistical analyses were performed using MatLab unless explicitly stated otherwise.
3. Results
To investigate the propagation of synucleinopathy throughout the brain and define in which direction trans-axonal propagation occurs, we compared the data collected in our in vivo model to the theoretical propagation pattern based on regular axonal transmission (NT), retrograde axonal transmission (DNT-ant), anterograde axonal transmission (DNT-ret) and Spatial Diffusion (SPD), as calculated using Eqs. (1), (2), evaluated on the (426 × 426) connectivity matrix obtained from the Allen Institute for Brain Science’s Mouse Connectivity Atlas (MCA). First, we aimed to go beyond the kind of correlative analysis of isolated or selected brain regions reported in previous studies, and instead wanted to create a model-based mathematical validation of the network transmission hypothesis on the entire brain network. Second, we aimed to quantitatively establish whether directional bias is observed in serial inclusions data and can explain patterns of synucleinopathy propagation. Figs. Figs.1a1a & b illustrate directional spreading of inclusions and the DNT model, and Fig. 1c shows parameter fitting across βt-values using the DNT model, as well as predictive and non-predictive model curves. In Fig. 1d, we provide an example of the anatomical illustrations we use with the DNT model, along with a universal legend for all such figure panels.
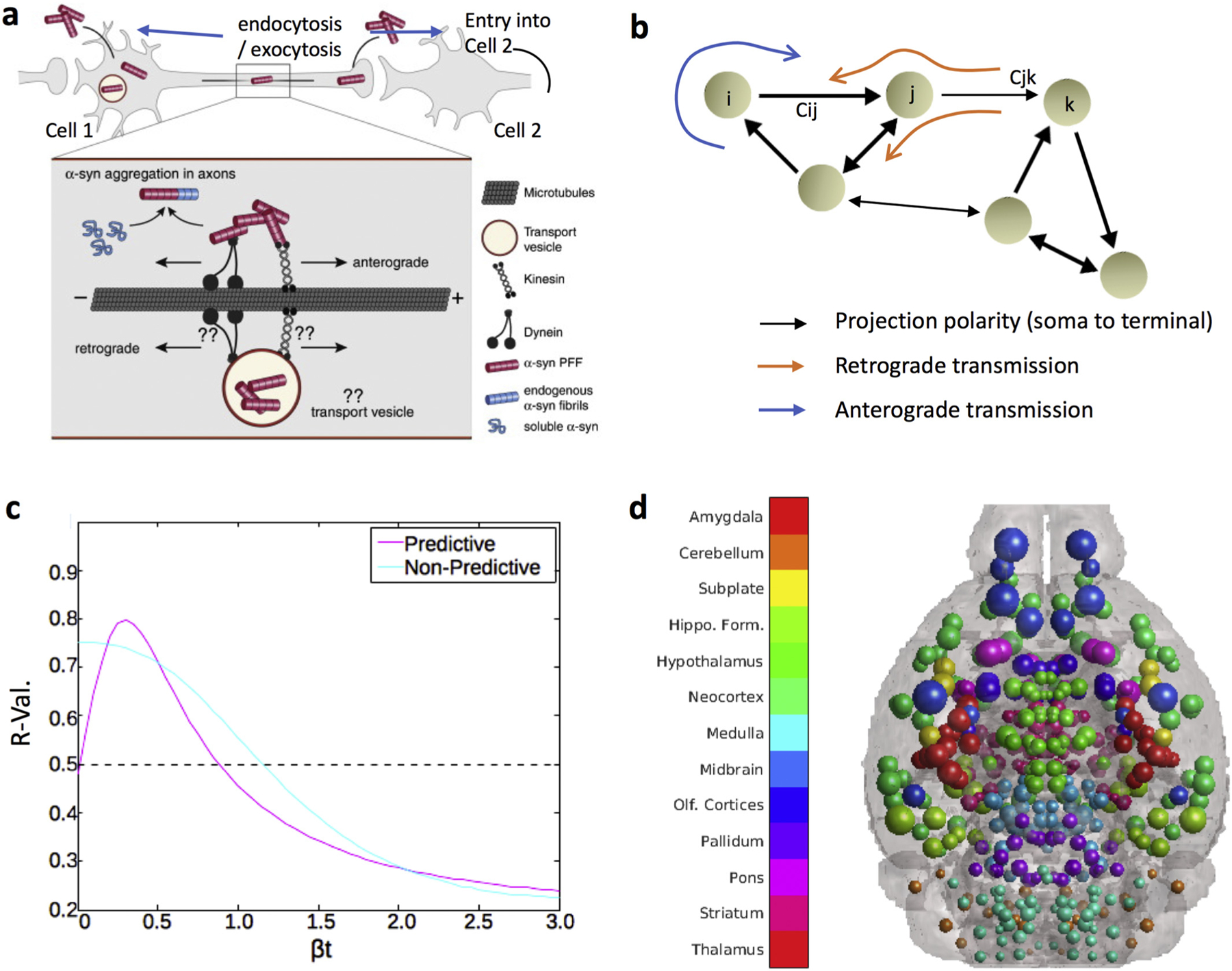
Anatomical example of network transmission, what the DNT model represents, and how to assess it. (a) α-Syn fibrils can be internalized both in the dendrite/cell body compartment and in axons. α-Syn fibrils are actively transported along microtubules both in the anterograde (driven by kinesin) and retrograde (driven by dynein) direction, perhaps directly in the cytoplasm or perhaps in transport vesicles following endocytosis. Aggregation is thought to initially occur in axons, where α-syn fibrils can encounter and template the misfolding of soluble endogenous α-syn proteins that are transported along axons for delivery to synapses. Figure adapted with permission from Bieri, et al., 2018. (b) Graphic illustration of the whole brain directional connectivity network, with nodes representing brain regions, and connection strengths {Cij} representing tracer-derived mesoscale connectivity. Examples of anterograde and retrograde transmission on this network are depicted. This macroscopic network transmission model is driven by the cellular-level transmission and transport processes depicted in panel A. (c) Example of a βt-curve showing r-value across βt parameter values for predictive (peak at βt ≥ 0) and non-predictive models (peak at βt = 0). (d) A color legend and brain showing the color scheme, by major region, for all the balls depicting regions in all anatomical illustrations of mouse brains throughout the rest of the paper. The dot sizes correspond to randomly generated “example“or “pseudo“inclusions amounts, per-region, where each dot represents one region in its center of mass. In anatomic illustrations of this nature with actual results, the inclusions amounts will not be random, but will rather be determined by inclusions severity recorded from data or predicted by a given NT model.
3.1. Interregional α-syn propagation initially mirrors retrograde direction spread along fiber tracts, but increasingly involves anterograde direction transit as time elapses
Using Eq. (2) and a seed signal placed at the injection site in the olfactory bulb, we evolved the DNT model at all model “diffusion times” βt. We first selected 3 and 12 months as timepoints representing respectively short and long amounts of time post-injection, and then assessed interregional αsyn inclusions propagation. The peak R-value between the model evaluated at all model times βt, and empirical regional αsyn inclusions data at 3 months post-injection, both retrograde DNT, R = 0.51, p < .01 and undirected NT, R = 0.44, p < .01, trend toward significantly matching the data of mice injected with mPFFs (Fig. 2a–b). We anatomically illustrate the data and our retrograde DNT (DNT-Ret) model’s predictions in Fig. 2c using a “glass brain” rendering whereby spheres represent brain regions and their size is proportional to the regional value of interest. Anterograde DNT (DNT-Ant) and Spatial diffusion (SPD) models do not significantly recreate empirical inclusion pattern at 3 months post-injection. However, by 12 months following αsyn fibrils injection, anterograde DNT, R = 0.63, p < .002, along with retrograde DNT, R = 0.60, p < .002, and undirected NT, R = 0.68, p < .002 (Fig. 2d–e) all produce significant recreations of empirical regional αsyn inclusions data in mice injected with mPFFs. We anatomically illustrate our anterograde DNT model’s regional inclusions predictions and empirical data in Fig. 2f. The SPD model continues to fail to produce any pattern that significantly matches empirical data.
We observe a remarkably similar pattern in our datasets between injection at 3 and 12-months post injection in mice injected with huPFFs. Retrograde DNT, R = 0.65, p < .002, and undirected NT, R = 0.59, p < .002 significantly recreate empirical regional αsyn inclusions patterns at 3-months post-injection, while anterograde DNT does not (Fig. 3a–b). We anatomically illustrate the strong match between retrograde DNT and empirical data in Fig. 3c. However, by 12-months post-injection anterograde DNT, R = 0.72, p < .002, significantly, and most faithfully, recreates empirical regional patterns of αsyn inclusions (Fig. 3d–e). Retrograde DNT, R = 0.57, p < .01, trends toward, and undirected NT, R = 0.67, p < .002 significantly, recreate empirical inclusions patterns (Fig. 3d–e). We anatomically illustrate the data and the anterograde DNT model’s recreation in Fig. 3f. The SPD model does not recreate spatiotemporal αsyn inclusions patterns in mice injected with huPFFs.
We further analyzed the 18-month post-injection data and observed the same phenomenon of implied heavy involvement of anterograde direction fiber tract transit in mice injected with both mPFFs and huPFFs. As referred to in our note above, the R, ΔR, and p-values at 18 months are not directly comparable with those from the other timepoints in our dataset, so we analyze and present the 18-months data separately. We found that anterograde DNT produces a significant, and the strongest, recreation of regional inclusions patterns in both mice injected with huPFFs, R = 0.64, p < .002 (Fig. 4a–b) and mice injected with mPFFs, R = 0.70, p < .002 (Fig. 4d–e), compared to retrograde DNT, non-directional NT or spatial diffusion. Data at 18-months post-injection from both mice injected with huPFFs and mPFFs also trends toward or does produce significant, albeit not as faithful, recreations by retrograde DNT, R = 0.49, p < .01 (huPFFs), R = 0.60, p < .002 (mPFFs), and undirected NT, R = 0.55, p < .01 (huPFFs), R = 0.63, p < .002 (mPFFs) (Figs. 4a–b & 4d–e). We anatomically illustrate data as well as predictions from our anterograde and retrograde DNT models in Fig. 4c (huPFFs) and Fig. 4f (mPFFs). We again find the consistent pattern, across injectate types, of implied heavy involvement of anterograde direction fiber tract travel as a driver of αsyn pathology spread.
We next computed ΔR-values as described in Data Analysis subsection of Methods. We repeated this for anterograde, retrograde and bidirectional DNT models, as well as the non-network spatial diffusion model. We repeated above analysis by comparing the model against each individual time point of the empirical mouse data. We found that the DNT model of retrograde spread of αsyn along fiber tracts, as modeled by (DNT-Ret), fits better the in vivo αsyn propagation pattern, as measured by ΔR-value against empirical αsyn inclusion data at 3, 6, and 9 months post-injection than does anterograde spread, modeled by (DNT-Ant.). However, by 12 months (the last numerically directly comparable timepoint in these data – please refer to material and methods), this pattern is reversed and anterograde DNT gives a better fit for spatiotemporal αsyn inclusions propagation. Furthermore, despite being slightly different in terms of number and location of regions quantified, the data obtained from 18-months post-injection shows the best fits with modeled synucleinopathy spread proceeding in the anterograde, as opposed to retrograde, direction, consistent with the 12-months results. This result is consistent regardless of whether mice were initially injected with mouse- (Fig. 5a) or human- (Fig. 5b) PFFs. Furthermore, bias toward anterograde synucleinopathy propagation, as measured by anterograde DNT ΔR-value minus retrograde DNT ΔR-value, consistently increases over time in both human and mouse PFF datasets (Fig. 5c).
3.2. Data using other injection sites suggests a similar pattern of early retrograde and later anterograde spread involvement in interregional αsyn propagation
We first directly test whether there might be an initial retrograde bias in early post-injection αsyn inclusions, using the dataset obtained from the Masuda-Suzukake, et al., 2013 (Masuda-Suzukake et al., 2013) publication and another dataset from one of our labs (Chatterjee et al., 2019). We find that in the dataset from (Masuda-Suzukake et al., 2013), at both 3 and 6 months post-injection, akin with similar timepoints post-injection from our own dataset (see above), there is a retrograde bias in how well DNT fits αsyn inclusions data. We further find that, in other data from one of our labs (Chatterjee et al., 2019), the spatial pattern of αsyn inclusions at only 8 weeks post-injection with fibrils, is best fit by retrograde DNT, p < .002, (Fig. 6a) using a two-sample t-test. Akin to results obtained using our own datasets, there is however a switch to a finding of anterograde bias in how well DNT fits αsyn pathology data by 15 months post-injection (Fig. 6b). We subsequently used the data from (Masuda-Suzukake et al., 2013), to create an example illustration of the phenomenon of implied heavy and increasing anterograde direction fiber tract transit involvement in interregional αsyn propagation, as ΔR-values for retrograde DNT are strong by 3 and 6-months post-injection, but anterograde DNT ΔR-values are even higher than those of retrograde DNT by 15 months post-injection (Fig. 6c). We anatomically illustrate our results using data from the Masuda-Suzukake, et al., 2013, study in Fig. 6d. More information on the dataset from (Masuda-Suzukake et al., 2013) and the data from Patrik Brundin’s lab used here can be obtained in the Mouse synucleinopathy dataset obtained from published source subsection of the Methods section. These results and their implications are further explored in the Discussion.
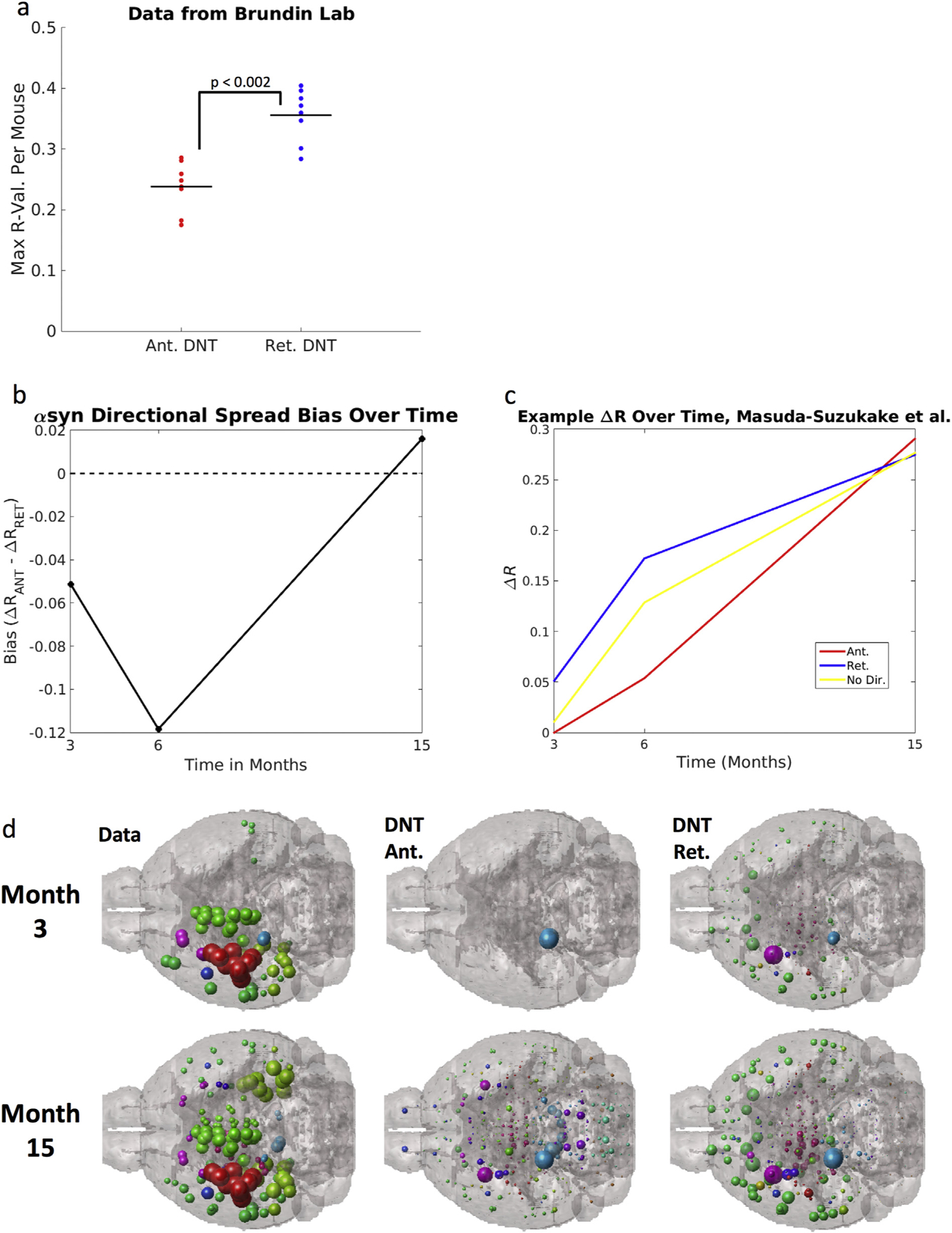
Datasets using other injection sites show the same directional shift as our data: that at timepoints surrounding the injection, synuclein inclusions are better explained by a model positing retrograde spread, but at timepoints farther form the injection, models using anterograde spread perform better. (a) Another dataset from Patrik Brundin’s lab (Chatterjee et al., 2019) using mice injected with fibrils into the striatum with regional quantification at 8-weeks post injection show the same pattern, regardless of genotype, as this early post-injection quantification of αsyn inclusions is best fit by retrograde DNT. The next panels (b,c, d) use data from (Luk and Lee, 2014): (b) Anterograde bias, calculated as in the y-axis legend above is strongest at 15-months post injection, implying that anterograde DNT, relative to retrograde DNT, performs better at later timepoints, across both ours and the Masuda-Suzukake, et al. 2013 study. A reference line at 0, the value at which there should be no directional bias, is added to the plot in (b). (c) An example, from Masuda-Suzukake, et al., 2013, showing the same switch from synuclein inclusions being best recreated by retrograde DNT at early timepoints, to being best recapitulated by anterograde DNT at later timepoints. (d) An anatomic illustration of the data and DNT simulated data from Masuda-Suzukake, et al. 2013, as in (b) & (c) above. All data and NT simulation values are log-transformed prior to running correlations. + p < .01, * p < .02.
4. Discussion
Our results confirm that αsyn inclusions are likely to spread along axons and transsynaptically after triggering synucleinopathy by localized injection of αsyn fibrils. We also observed that both anterograde and retrograde αsyn propagation are possible. Our results suggest that αsyn inclusions spreading is directionally biased with a predominance of retrograde propagation first, and that the directional bias of αsyn pathology propagation changes over time. Specifically, until 9 months post injection, a propagation model involving retrograde transport reproduces the pattern we observed in our animal experiments. In a second phase, 12 months post injection and later, a combination of retrograde and anterograde propagation explains the spatial pattern observed in vivo. A time-dependent directional bias can help explain why in human synucleinopathies some brain regions that are strongly connected to an affected brain area are spared early on, but not later, in the disease course.
We found that our mathematical model of anatomical spreading of αsyn inclusions applied well to both our own data from a mouse model involving αsyn PFFs injection into the olfactory bulb (Rey et al., 2018a, 2016b), as well as in mice where αsyn fibrils or PFFs were injected into either the Substantia nigra (Masuda-Suzukake et al., 2013) or striatum (Chatterjee et al., 2019). While we consistently observed a preferred retrograde spread early and anterograde spread late after injection of fibrils/PFFs, we do imply that the directional switch we find here is a general rule. It is possible that the particular conformation of the initial seed of αsyn aggregates (i.e. specific αsyn “strains”) and the anatomical region where the first seeding event takes place (e.g. olfactory bulb versus substantia nigra) will significantly influence the direction (antero- or retrograde) of spread. We discuss the relevance of this to both αsyn pathology in general and with regards to specific synucleinopathies (e.g. PD, DLB) in subsections below.
4.1. Transsynaptic and fiber tract-based spread model replicates the αsyn inclusions spread observed in vivo
Axonal transport followed by transsynaptic spread have been proposed as the predominant mechanisms underlying αsyn pathology propagation between brain regions (Angot et al., 2010; Brettschneider and Del Tredici, 2015; Brundin et al., 2008; Brundin and Melki, 2017; Dehay et al., 2016; Rey et al., 2016a; Steiner et al., 2011; Ubeda-Bañon et al., 2014). Some studies have utilized cell cultures models (Freundt et al., 2012; Volpicelli-Daley et al., 2011) that do not reflect the complexity of brain connectivity and also lack several of its cellular components (e.g. different glia). Other researchers question the transsynaptic spread hypothesis entirely, arguing that the spread of αsyn pathology does not follow a synaptic connectivity rule (Surmeier et al., 2017a; Surmeier et al., 2017b). We explicitly test the transsynaptic and connectome based spread hypothesis using our current DNT model, with connectome data obtained from the Allen Brain Institute mesoscale mouse connectome [http://connectivity.brain-map.org; 28]. This atlas provides detailed anatomy of 426 different nodes (corresponding to brain regions), and their connections are based on extensive tracing experiments. The connectome takes into account the direction of transport of the tracers and the weight of the different connections. It is, therefore, a very powerful tool to investigate the theoretical patterns of propagation based on anterograde, retrograde or diffuse spreading of pathological seeds. To use this connectome with our DNT modeling, we had to make several assumptions. We assumed that there are no brain-region specific biases in the transfer or uptake of their adenovirus tracer (Oh et al., 2014) and that uptake is therefore even, allowing for some noise, across all parts of the mouse brain. We also assumed that there is a directly proportional relationship between fluorescent signal from the viral tracer and the total number or volume of axonal tracts from the seeded to the signal region. As discussed in the ABI paper introducing the MCA (Oh et al., 2014), these assumptions are unlikely to be true across the board, but are likely accurate enough at the coarse parcellation of a brain into 426 regions. Importantly, making these assumptions allows our model to simulate spread over the entire connectome.
We demonstrate clear correspondence between regions most likely (based on neuronal connections) to exhibit pathology from the region where the initial seeding takes place (the olfactory bulb, substantia nigra, or striatum) and the regions that we found to contain αsyn inclusions in in vivo experiments (Masuda-Suzukake et al., 2013; Rey et al., 2018a; Rey et al., 2016b) (Figs. 2–5). To confirm that the fitting of the theoretical propagation pattern with the empirical in vivo data is not dependent on site of pathology-initiation, we analyzed previously published datasets using two different injection sites (Masuda-Suzukake et al., 2013; Rey et al., 2018a; Rey et al., 2016b). We found the same correspondence between our theoretical modeling of propagation and published data from (Masuda-Suzukake et al., 2013) using with the substantia as initiation site. We further demonstrate retrograde DNT recreates spatial αsyn patterns (Chatterjee et al., 2019) (Fig. 6d). We also tested whether a pattern of spread based on spatial, rather than connectome proximity to the seed region, would recapitulate αsyn patterns, implying potential active or passive extracellular spread of pathogenic protein aggregates. This analysis showed that spatial diffusion is not a predictor of αsyn inclusions localization in the two datasets we examined (Figs. 2–6), and provides further support for the transsynaptic αsyn pathology spread hypothesis.
Importantly, recently published work from Virginia L.Y. Lee’s group investigated also the spread of αsyn pathology through the mouse brain using connectome modeling (Henderson et al., 2019). In this study, the authors investigated the propagation of αsyn inclusions from several injection sites in a time window of 1 to 6 months post injection. In this study, inclusions were also detected with an antibody directed against hyperphosphoraylated alpha-synuclein and stained sections were analyzed quantitatively in an automated way. The authors also implemented in their model the expression level of αsyn at the injection site. Despite different methods used, both our work and Henderson et al.’s demonstrate that early propagation is occurring preferentially in a retrograde direction, and that the preferential retrograde propagation is a generalized mechanism to all the brain regions each lab tested. In addition, the authors observed that the regional level of expression of αsyn influences strongly the vulnerability of a given brain region to develop αsyn inclusions. The study by Henderson et al. explores only short injection delays (up to 6 months), and thus do not investigate the long delays post injections where we observed a switch to a preferential anterograde propagation direction after 9 months, both in our OB-injection dataset and in data from (Masuda-Suzukake et al., 2013).
4.2. Directional switching of αsyn propagation might explain divergent spatiotemporal pathology development patterns between patients
One advantage of our paradigm is the possibility to test transregional spread along fiber tracts in both anterograde (pre-to-postsynaptic) and retrograde (post-to-presynaptic) directions. Interestingly, we found that both anterograde and retrograde directions can successfully recreate transregional αsyn inclusions spread, consistent with prior cell culture (Freundt et al., 2012; Volpicelli-Daley et al., 2011) and in vivo (Rusconi et al., 2018; Ulusoy et al., 2017; Ulusoy et al., 2013) studies showing both anterograde and retrograde transport of αsyn. In fact, our results suggest that the directional bias of αsyn spread may in fact change over time.
What cellular mechanisms might contribute to change biases in directional spread over time? Prior work on transsynaptic αsyn propagation suggests that spread through a neural network in an anterograde direction might involve release of exosomes into the synaptic cleft (Steiner et al., 2011). However, retrograde spread can also occur, via release into the synaptic cleft from dendrites or soma, and possibly enter the presynaptic side via tunneling nanotubes or even receptor mediated internalization (Ubeda-Bañon et al., 2014). For network spread to occur bidirectionally, both transsynaptic propagation and axonal transport/diffusion of αsyn must be bidirectional, assumptions that are supported by cell culture and microfluidic studies (Saha et al., 2004; Tang et al., 2012; Utton et al., 2005). Interestingly, αsyn can interfere with the net directionality of axonal transport by its interaction with kinesin and dynein motors (Saha et al., 2004; Utton et al., 2005), and thereby affect directional bias.
We speculate that biased spread influences spatiotemporal development of αsyn pathology and partly explains why some regions exhibit αsyn inclusions while others do not. The enteric nervous system and/or the olfactory system represent two possible locations where the accumulation of misfolded αsyn species overwhelm the cellular protein degradation mechanisms in PD and DLB. In PD, αsyn inclusions development in the central nervous system is generally first observed in dorsal motor nucleus of the vagus nerve (the origin of enteric nerves) and in olfactory areas such as the olfactory bulb and anterior olfactory nucleus (Braak et al., 2003a; Braak et al., 2003b; Braak and Del, 2017; Braak and Del, 2016; Braak et al., 2004; Del Tredici and Braak, 2016; Rey et al., 2018b; Ubeda-Bañon et al., 2014). In DLB, αsyn aggregates are frequently seen in olfactory structures as well as limbic system (Beach et al., 2009).
Our work posits not only directional biases in αsyn spread, but a directional switch in these biases (Figs. 2–6). αSyn can interact with both anterograde direction kinesin and retrograde direction dynein axonal transport protein families (Bieri et al., 2018). Importantly, αsyn misfolding appears to decrease its anterograde transport by kinesin proteins, and increase its retrograde transport by dynein proteins (Volpicelli-Daley, 2017) by at latest 8 weeks post-aggregation initiation. This could explain an early retrograde bias in αsyn propagation that shifts to either bidirectional or anterograde αsyn spread as αsyn inclusions progress. Because both the substantia nigra and olfactory areas have dense connections with the striatum (Oh et al., 2014), the relative sparsity of αsyn aggregates in the striatum in PD might be viewed as unexpected. However, if transsynaptic αsyn spread is predominantly in the retrograde direction early in disease course (Figs. 2–6), we would indeed not expect early pronounced αsyn inclusions accumulation in the striatum because most of the connections between the injected olfactory bulb and the striatum are afferents to the striatum (Oh et al., 2014).
4.3. Assumptions and limitations
There are limitations of our model and of our datasets. First, our DNT modeling rely on several assumptions so we could model the spread over the entire connectome (we assumed that the uptake of adenovirus tracer is even in the brain, and assume that the tracers fluorescent signal is proportional to the volume of axonal tracts containing fluorescent signal). As mentioned earlier and as discussed in previous work (Oh et al., 2014), our assumptions are likely accurate enough for the parcellation in 426 brain regions we are using here.
In addition, the model does not consider region-specific factors that may influence the susceptibility of regions to become ‘infected’ by pathogenic αsyn. Therefore, our model does not consider region-specific factors which can influence an area’s susceptibility to develop inclusions, regardless of spread, a concern other recent work investigating αsyn inclusion spread notes (Henderson et al., 2019). Our model also does not reflect that neurons might die, due to aggregates forming, in some brain regions, and therefore neither updates the connectome to account for loss of white matter nor updates the model to account for loss of αsyn that dead neurons once contained. Uneven antibody penetrance between mice could potentially confound data. There are also limitations regarding our dataset because it is only semi-quantitative (Rey et al., 2018a; Rey et al., 2016b). However, in our long-term study (Rey et al., 2018a), we performed densitometry analysis of pser129 inclusions on a restricted number of brain regions, in addition to our semi-quantitative analysis, and the results from both method were consistent indicating that our semi-quantitative analysis using scores reflects well the density of inclusions present in a given brain region. Finally, cellular inflammatory and excocytosis responses that occur in response to injection with α-synuclein fibrils could affect spread processes for α-synuclein inclusions at timepoints closest to injection. The fit of the DNT model at 3-months post-injection is worse than at any other timepoint, potentially indicating injection-response cellular processes as a limitation for how well a spread or diffusion model can fit data at timepoints close to injection.
Additionally of note, some technical aspects differs between the different datasets. We performed injections into the olfactory bulb in 7–8 weeks old C57/Bl6 mice, while Masuda-Suzukake and coworkers (Masuda-Suzukake et al., 2013) injected older mice of the same strains. Regarding our intrastriatal injections, we injected 4 week-old mice of a different background strain (OF1mice). The fibrils used for each dataset were different or produced by different labs: for olfactory bulb injections, mPFFs and huPFFs were produced by K. Luk while huPFFs used for striatal injections were produced in our lab (following K Luk’s published protocol) (Volpicelli-Daley et al., 2015); and Masuda-Suzu-kake’s fibrils were produced following a different protocol in their own lab (Masuda-Suzukake et al., 2013). Thus, it is possible that these different assemblies have different seeding and propagation capacities. Despite these differences, our results demonstrate similar propagation characteristics in the three different datasets.
4.4. Concluding remarks
In conclusion, we provide evidence that the propagation of synucleinopathy in fibril-injection mouse models occurs via neural network and transsynaptically. Our findings in mice support the hypothesis by Braak and colleagues of propagation of αsyn pathology via neuronal networks in humans (Braak et al., 2003a; Braak et al., 2003b; Braak and Del, 2017; Braak and Del, 2016; Braak et al., 2004; Del Tredici and Braak, 2016). In addition, we demonstrate that the preferred direction of αsyn inclusions propagation is initially retrograde, and becomes anterograde at several months post injection, indicating that the preferred direction can switch over time.
Acknowledgements
We acknowledge the Van Andel Research Institute and the many individuals and corporations that financially support research into neurodegenerative disease at the Institute. N.L.R.’s work is supported by the Peter C. and Emajean Cook Foundation. P.B. is supported by grants from the National Institutes of Health (1R01DC016519-01, 5R21NS093993-02 and 1R21NS106078-01A1). P.B. reports additional grants from Office of the Assistant Secretary of Defense for Health Affairs (Parkinson’s Research Program, Award No. W81XWH-17-1-0534), The Michael J Fox Foundation, National Institutes of Health, Cure Parkinson’s Trust, which are outside but relevant to the submitted work.
We would also like to acknowledge the Weill Cornell Neuroscience and Radiology Departments, as well as the Feil Family Brain & Mind Research Institute and the many people who generously support Weill Cornell. AR and CM are supported by grants from the National Institutes of Health (R01NS092802). AR and CM would also like to acknowledge our former and present colleagues from the IDEAL lab.
We also thank Luk, KC, Trojanowski, JQ, Lee, VM, George, S, Steiner, JA, Madaj Z, Maroof, N, Chatterjee N, Saiz Sanchez D, Quansah E, Becker K, Ma J, Escobar Galvis ML, Kordower JH for the fruitful collaboration which led us to the three prior publications from which we have extracted data for the current study.
Footnotes
Ethical approval and consent to participate
All mouse-work datasets in the present work was obtained from datasets originally intended and used for other already published work already declaring that all animal work was done under approved protocols and animal care standards (Masuda-Suzukake et al., 2013; Rey et al., 2018a; Rey et al., 2016b).
Data availability statement
All primary data cited in the present manuscript is already available for access from citations (Chatterjee et al., 2019; Masuda-Suzukake et al., 2013; Rey et al., 2016a, Rey et al., 2018a, Rey et al., 2013, Rey et al., 2016b, Rey et al., 2018b).
Declaration of Competing Interest
P.B. has received commercial support as a consultant from Renovo Neural, Inc., Roche, and Teva Inc., Lundbeck A/S, AbbVie, Neuroderm, Fujifilm-Cellular Dynamics, ClearView Healthcare, FCB Health, IOS Press Partners and Capital Technologies, Inc. P.B. has received commercial support for grants/research from Renovo and Teva/Lundbeck. P.B. has ownership interests in Acousort AB and is on the steering committee of the NILO-PD trial.
The authors declare no additional competing financial interests.
References
- Angot E, Steiner JA, Hansen C, Li J, Brundin P, 2010. Are synucleinopathies prion-like disorders? Lancet Neurol. 9, 1128–1138. 10.1016/S1474-4422(10)70213-1. [Abstract] [CrossRef] [Google Scholar]
- Beach TG, Adler CH, Lue L, et al., 2009. Unified staging system for Lewy body disorders: correlation with nigrostriatal degeneration, cognitive impairment and motor dysfunction. Acta Neuropathol. 117, 613–634. 10.1007/s00401-009-0538-8. [Europe PMC free article] [Abstract] [CrossRef] [Google Scholar]
- Bieri G, Gitler AD, Brahic M, 2018. Internalization, axonal transport and release of fibrillar forms of alpha-synuclein. Neurobiol. Dis 109, 219–225. [Europe PMC free article] [Abstract] [Google Scholar]
- Braak H, Del TK, 2016. Potential pathways of abnormal tau and alpha-synuclein dissemination in sporadic Alzheimer’s and Parkinson’s diseases. Cold Spring Harb. Perspect. Biol 1–24. [Europe PMC free article] [Abstract] [Google Scholar]
- Braak H, Del TK, 2017. Neuropathological staging of brain pathology in sporadic Parkinson’s disease: separating the wheat from the chaff. J. Park. Dis 7, 571–585. 10.3233/JPD-179001. [Europe PMC free article] [Abstract] [CrossRef] [Google Scholar]
- Braak H, Rüb U, Gai WP, Del TK, 2003a. Idiopathic Parkinson’s disease: possible routes by which vulnerable neuronal types may be subject to neuroinvasion by an unknown pathogen. J. Neural Transm 110, 517–536. 10.1007/s00702-002-0808-2. [Abstract] [CrossRef] [Google Scholar]
- Braak H, Del K, Rüb U, De VRAI, Jansen ENH, Braak E, 2003b. Staging of brain pathology related to sporadic Parkinson’s disease. Neurobiol. Aging 24, 197–211. [Abstract] [Google Scholar]
- Braak RH, Ghebremedhin E, Rüb U, Bratzke H, Del TK, 2004. Stages in the development of Parkinson’s disease-related pathology. Cell Tissue Res. 318, 121–134. 10.1007/s00441-004-0956-9. [Abstract] [CrossRef] [Google Scholar]
- Brettschneider J, Del Tredici K, 2015. Lee VM-Y, Trojanowski JQ. Spreading of pathology in neurodegenerative diseases: a focus on human studies. Nat. Rev. Neurosci 16 (2), 109–120. 10.1038/nrn3887.Spreading. [Europe PMC free article] [Abstract] [CrossRef] [Google Scholar]
- Brundin P, Melki R, 2017. Prying into the prion hypothesis for Parkinson’s disease. J. Neurosci 37 (41), 9808–9818. 10.1523/JNEUROSCI.1788-16.2017. [Europe PMC free article] [Abstract] [CrossRef] [Google Scholar]
- Brundin P, Li J, Holton JL, Lindvall O, Revesz T, 2008. Research in motion: the enigma of Parkinson’s disease pathology spread. Nat. Rev. Neurosci 9 (October), 741–745. [Abstract] [Google Scholar]
- Chatterjee D, Saiz Sanchez D, Quansah E, et al., 2019. Loss of one Engrailed1 allele enhances induced α-synucleinopathy. J Parkinson’s Dis. 9 (2), 315–326. January (26th). 10.3233/JPD-191590. [Europe PMC free article] [Abstract] [CrossRef] [Google Scholar]
- Dehay B, Fernagut P, 2016. Alpha-synuclein based models of Parkinson’s disease. Rev. Neurol. (Paris) 172 (6–7), 371–378. [Abstract] [Google Scholar]
- Dehay B, Vila M, Bezard E, Brundin P, Kordower J, 2016. Alpha-synuclein propogation: new insights from animal models. Mov. Disord 31 (2), 161–168. [Abstract] [Google Scholar]
- Del Tredici K, Braak H, 2016. Review: sporadic Parkinson’s disease: development and distribution of alpha-synuclein pathology. Neuropathol. Appl. Neurobiol 42, 33–50. 10.1111/nan.12298. [Abstract] [CrossRef] [Google Scholar]
- Freeze B, Acosta D, Pandya S, Zhao Y, Raj A, 2018. Regional expression of genes mediating trans-synaptic alpha-synuclein transfer predicts regional atrophy in Parkinson disease. NeuroImage Clin. 18, 456–466. [Europe PMC free article] [Abstract] [Google Scholar]
- Freundt EC, Maynard N, Clancy EK, et al., 2012. Neuron-to-neuron transmission of alpha-synuclein fibrils through axonal transport. Ann. Neurol 72, 517–524. 10.1002/ana.23747. [Europe PMC free article] [Abstract] [CrossRef] [Google Scholar]
- Goedert M, 2001. Alpha-synuclein and neurodegenerative diseases. Nat. Rev. Neurosci 2 (July), 492–501. [Abstract] [Google Scholar]
- Goedert M, Jakes R, Spillantini MG, 2017. The Synucleinopathies: twenty years on. J. Park. Dis 7, 551–569. 10.3233/JPD-179005. [Europe PMC free article] [Abstract] [CrossRef] [Google Scholar]
- Grozdanov V, Danzer KM, 2018. Release and uptake of pathologic alpha-synuclein. Cell Tissue Res. 373, 175–182. [Abstract] [Google Scholar]
- Hawkes CH, Del TK, Braak H, Wolfgang J, Review MF, 2007. Parkinson’s disease: a dual-hit hypothesis. Neuropathol. Appl. Neurobiol 33, 599–614. 10.1111/j.1365-2990.2007.00874.x. [Europe PMC free article] [Abstract] [CrossRef] [Google Scholar]
- Henderson MX, Cornblath EJ, Darwich A, Zhang B, Brown H, et al., 2019. Spread of alpha-synuclein pathology through the brain connectome is modulated by selective vulnerability and predicted by network analysis. Nat. Neurosci 22, 1248–1257. [Europe PMC free article] [Abstract] [Google Scholar]
- Luk KC, Lee VM, 2014. Modeling Lewy pathology propagation in Parkinson’s disease. Park Relat Disord. 20 (0 1), S85–S87. 10.1016/S1353-8020(13)70022-1. [Europe PMC free article] [Abstract] [CrossRef] [Google Scholar]
- Luk KC, Kehm V, Carroll J, et al., 2012. Pathological alpha-synuclein transmission initiates Parkinson-like neurodegeneration in nontransgenic mice. Science (80-) 338 (16 Novemeber 2012), 949–953. 10.1126/science.1227157. [Europe PMC free article] [Abstract] [CrossRef] [Google Scholar]
- Masuda-Suzukake M, Nonaka T, Hosokawa M, et al., 2013. Prion-like spreading of pathological alpha-synuclein in brain. Brain 136 (July), 1128–1138. 10.1093/brain/awt037. [Europe PMC free article] [Abstract] [CrossRef] [Google Scholar]
- Mezias C, Raj A, 2017. Analysis of amyloid-β pathology spread in mouse models suggests spread is driven by spatial proximity, not connectivity. Front. Neurol 8 (December), 1–12. 10.3389/fneur.2017.00653. [Europe PMC free article] [Abstract] [CrossRef] [Google Scholar]
- Mezias C, LoCastro E, Xia C, Raj A, 2017. Connectivity, not region-intrinsic properties, predicts regional vulnerability to progressive tau pathology in mouse models of disease. Acta Neuropathol Commun. 5 (61), 1–17. 10.1186/s40478-017-0459-z. [Europe PMC free article] [Abstract] [CrossRef] [Google Scholar]
- Oh SW, Harris JA, Ng L, et al., 2014. A mesoscale connectome of the mouse brain. Nature 508 (10 April), 207–214. 10.1038/nature13186. [Europe PMC free article] [Abstract] [CrossRef] [Google Scholar]
- Paxinos G, Franklin K, 2012. Paxinos & Franklin’s: The Mouse Brain in Stereotaxic Coordinates, 4th ed. Academic Press. [Google Scholar]
- Raj A, Kuceyeski A, Weiner M, 2012. A network diffusion model of disease progression in dementia. Neuron 73 (6), 1204–1215. [Europe PMC free article] [Abstract] [Google Scholar]
- Raj A, Locastro E, Kuceyeski A, Tosun D, Relkin N, Weiner M, 2015. Network diffusion model of progression predicts longitudinal patterns of atrophy and metabolism in Alzheimer’s disease. Cell Rep. 10 (January 20), 359–369. [Europe PMC free article] [Abstract] [Google Scholar]
- Recasens A, Ulusoy A, Kahle PJ, Di MDA, Dehay B, 2018. In vivo models of alpha-synuclein transmission and propagation. Cell Tissue Res. 373, 183–193. [Abstract] [Google Scholar]
- Rey NL, Petit GH, Bousset L, Melki R, Brundin P, 2013. Transfer of human α-synuclein from the olfactory bulb to interconnected brain regions in mice. Acta Neuropathol. 126, 555–573. 10.1007/s00401-013-1160-3. [Europe PMC free article] [Abstract] [CrossRef] [Google Scholar]
- Rey NL, George S, Brundin P, 2016a. Review: spreading the word: precise animal models and validated methods are vital when evaluating prion-like behaviour of alpha-synuclein. Neuropathol. Appl. Neurobiol 42, 51–76. 10.1111/nan.12299. [Abstract] [CrossRef] [Google Scholar]
- Rey NL, Steiner JA, Maroof N, et al., 2016b. Widespread transneuronal propagation of α-synucleinopathy triggered in olfactory bulb mimics prodromal Parkinson’s disease. J Exp Med. 8 (August), 1–20. 10.1084/jem.20160368. [Europe PMC free article] [Abstract] [CrossRef] [Google Scholar]
- Rey NL, George S, Steiner JA, et al., 2018a. Spread of aggregates after olfactory bulb injection of α-synuclein fibrils is associated with early neuronal loss and is reduced long term. Acta Neuropathol. 135, 65–83. [Europe PMC free article] [Abstract] [Google Scholar]
- Rey NL, Wesson DW, Brundin P, 2018b. The olfactory bulb as the entry site for prion-like propagation in neurodegenerative diseases. Neurobiol. Dis 109 (January 2018), 226–248. 10.1016/j.nbd.2016.12.013. [Europe PMC free article] [Abstract] [CrossRef] [Google Scholar]
- Rusconi R, Ulusoy A, Aboutalebi H, Di DA, 2018. Long-lasting pathological consequences of overexpression-induced alpha-synuclein spreading in the rat brain. Aging Cell 17, 1–11. 10.1111/acel.12727. [Europe PMC free article] [Abstract] [CrossRef] [Google Scholar]
- Saha AR, Hill J, Utton MA, et al., 2004. Parkinson’s disease α-synuclein mutations exhibit defective axonal transport in cultured neurons. J. Cell Sci 117 (7), 1017–1024. 10.1242/jcs.00967. [Abstract] [CrossRef] [Google Scholar]
- Steiner JA, Angot E, Brundin P, 2011. A deadly spread: cellular mechanisms of alpha-synuclein transfer. Cell Death Differ. 18, 1425–1433. 10.1038/cdd.2011.53. [Europe PMC free article] [Abstract] [CrossRef] [Google Scholar]
- Surmeier DJ, Obeso A, Halliday XGM, 2017a. Parkinson’s disease is not simply a prion disorder. J. Neurosci 37 (41), 9799–9807. 10.1523/JNEUROSCI.1787-16.2017. [Europe PMC free article] [Abstract] [CrossRef] [Google Scholar]
- Surmeier DJ, Obeso JA, Halliday GM, 2017b. Selective neuronal vulnerability in Parkinson disease. Nat. Rev. Neurosci 18 (February), 101–113. 10.1038/nrn.2016.178. [Europe PMC free article] [Abstract] [CrossRef] [Google Scholar]
- Tang Y, Das U, Scott DA, Roy S, 2012. The slow axonal transport of alpha-synuclein—mechanistic commonalities amongst diverse cytosolic cargoes. Cytoskeleton 69 (July), 506–513. 10.1002/cm.21019. [Europe PMC free article] [Abstract] [CrossRef] [Google Scholar]
- Tyson T, Steiner JA, Brundin P, 2016. Sorting out release, uptake and processing of alpha-synuclein during prion-like spread of pathology. J. Neurochem 139, 275–289. 10.1111/jnc.13449. [Europe PMC free article] [Abstract] [CrossRef] [Google Scholar]
- Ubeda-Bañon I, Saiz-Sanchez D, de la Rosa-Prieto C, Martinez-Marcos A, 2014. Alpha-Synuclein in the olfactory system in Parkinson’s disease: role of neural connections on spreading pathology. Brain Struct. Funct 219, 1513–1526. 10.1007/s00429-013-0651-2. [Abstract] [CrossRef] [Google Scholar]
- Uchihara T, Giasson BI, 2016. Propagation of alpha-synuclein pathology: hypotheses, discoveries, and yet unresolved questions from experimental and human brain studies. Acta Neuropathol. 131, 49–73. 10.1007/s00401-015-1485-1. [Europe PMC free article] [Abstract] [CrossRef] [Google Scholar]
- Ulusoy A, Rusconi R, Pérez-Revuelta BI, et al., 2013. Caudo-rostral brain spreading of alpha-synuclein through vagal connections. EMBO Mol Med. 5, 1119–1127. 10.1002/emmm.201302475. [Europe PMC free article] [Abstract] [CrossRef] [Google Scholar]
- Ulusoy A, Phillips RJ, Helwig M, Klinkenberg M, Powley TL, DiMonte DA, 2017. Brain-to-stomach transfer of alpha-synuclein via vagal preganglionic projections. Acta Neuropathol. 133 (3), 381–393. 10.1007/s00401-016-1661-y. [Europe PMC free article] [Abstract] [CrossRef] [Google Scholar]
- Utton MA, Noble WJ, Hill JE, Anderton BH, Hanger DP, 2005. Molecular motors implicated in the axonal transport of tau and alpha-synuclein. J. Cell Sci 118 (20), 4645–4654. 10.1242/jcs.02558. [Abstract] [CrossRef] [Google Scholar]
- Volpicelli-Daley LA, 2017. Effects of α-synuclein on axonal transport. Neurobiol. Dis 105, 321–327. [Abstract] [Google Scholar]
- Volpicelli-Daley LA, Luk KC, Patel TP, et al., 2011. Exogenous alpha-Synuclein fibrils induce Lewy Body pathology leading to synaptic dysfunction and neuron death. Neuron. 72 (1), 57–71. 10.1016/j.neuron.2011.08.033.Exogenous. [Europe PMC free article] [Abstract] [CrossRef] [Google Scholar]
- Volpicelli-Daley L, Luk KC, Lee VM, 2015. Addition of exogenous alpha-synuclein pre-formed fibrils to primary neuronal cultures to seed recruitment of endogenous alpha-synuclein to Lew body and Lewy neurite-like aggregates. Nat. Protoc 9 (9), 2135–2146. 10.1038/nprot.2014.143.Addition. [Europe PMC free article] [Abstract] [CrossRef] [Google Scholar]
Full text links
Read article at publisher's site: https://doi.org/10.1016/j.nbd.2019.104623
HAL Open Archive
https://hal.science/hal-04247276
Citations & impact
Impact metrics
Article citations
Current insights and assumptions on α-synuclein in Lewy body disease.
Acta Neuropathol, 148(1):18, 14 Aug 2024
Cited by: 0 articles | PMID: 39141121 | PMCID: PMC11324801
Review Free full text in Europe PMC
Neuromodulation modifies α-synuclein spreading dynamics in vivo and the pattern is predicted by changes in whole-brain function.
Brain Stimul, 17(4):938-946, 01 Jul 2024
Cited by: 0 articles | PMID: 39096960
Effect of host and strain factors on α-synuclein prion pathogenesis.
Trends Neurosci, 47(7):538-550, 27 May 2024
Cited by: 0 articles | PMID: 38806297
Review
Network models to enhance the translational impact of cross-species studies.
Nat Rev Neurosci, 24(9):575-588, 31 Jul 2023
Cited by: 3 articles | PMID: 37524935 | PMCID: PMC10634203
Review Free full text in Europe PMC
Retina-to-brain spreading of α-synuclein after intravitreal injection of preformed fibrils.
Acta Neuropathol Commun, 11(1):83, 20 May 2023
Cited by: 7 articles | PMID: 37210559 | PMCID: PMC10199563
Go to all (38) article citations
Similar Articles
To arrive at the top five similar articles we use a word-weighted algorithm to compare words from the Title and Abstract of each citation.
Spread of aggregates after olfactory bulb injection of α-synuclein fibrils is associated with early neuronal loss and is reduced long term.
Acta Neuropathol, 135(1):65-83, 05 Dec 2017
Cited by: 104 articles | PMID: 29209768 | PMCID: PMC5756266
Critical appraisal of pathology transmission in the α-synuclein fibril model of Lewy body disorders.
Exp Neurol, 299(pt a):172-196, 19 Oct 2017
Cited by: 24 articles | PMID: 29056362 | PMCID: PMC5736319
Trans-synaptic and retrograde axonal spread of Lewy pathology following pre-formed fibril injection in an in vivo A53T alpha-synuclein mouse model of synucleinopathy.
Acta Neuropathol Commun, 8(1):150, 28 Aug 2020
Cited by: 33 articles | PMID: 32859276 | PMCID: PMC7456087
α-Synuclein in the olfactory system in Parkinson's disease: role of neural connections on spreading pathology.
Brain Struct Funct, 219(5):1513-1526, 18 Oct 2013
Cited by: 35 articles | PMID: 24135772
Review
Funding
Funders who supported this work.
NIA NIH HHS (2)
Grant ID: RF1 AG062196
Grant ID: R56 AG064873
NIBIB NIH HHS (1)
Grant ID: R01 EB022717
NIDCD NIH HHS (1)
Grant ID: R01 DC016519
NINDS NIH HHS (3)
Grant ID: R01 NS092802
Grant ID: R21 NS093993
Grant ID: R21 NS106078
National Institutes of Health (4)
Grant ID: 5R21NS093993-02
Grant ID: 1R21NS106078-01A1
Grant ID: 1R01DC016519-01
Grant ID: R01NS092802
Office of the Assistant Secretary of Defense for Health Affairs (1)
Grant ID: W81XWH-17-1-0534