Abstract
Free full text

Single-cell repertoire tracing identifies rituximab-resistant B cells during myasthenia gravis relapses
Abstract
Rituximab, a B cell–depleting therapy, is indicated for treating a growing number of autoantibody-mediated autoimmune disorders. However, relapses can occur after treatment, and autoantibody-producing B cell subsets may be found during relapses. It is not understood whether these autoantibody-producing B cell subsets emerge from the failed depletion of preexisting B cells or are generated de novo. To further define the mechanisms that cause postrituximab relapse, we studied patients with autoantibody-mediated muscle-specific kinase (MuSK) myasthenia gravis (MG) who relapsed after treatment. We carried out single-cell transcriptional and B cell receptor profiling on longitudinal B cell samples. We identified clones present before therapy that persisted during relapse. Persistent B cell clones included both antibody-secreting cells and memory B cells characterized by gene expression signatures associated with B cell survival. A subset of persistent antibody-secreting cells and memory B cells were specific for the MuSK autoantigen. These results demonstrate that rituximab is not fully effective at eliminating autoantibody-producing B cells and provide a mechanistic understanding of postrituximab relapse in MuSK MG.
Abstract
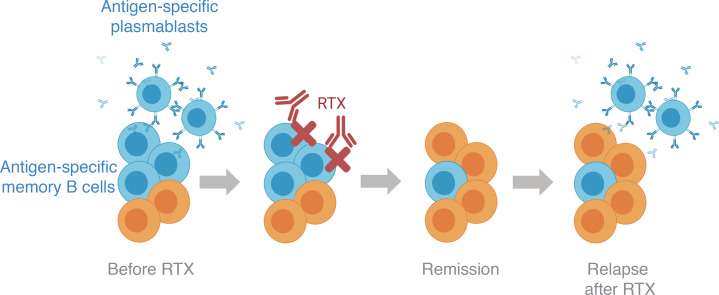
Introduction
Acquired myasthenia gravis (MG) is an autoimmune disorder caused by pathogenic autoantibodies that bind to membrane proteins at the neuromuscular junction, leading to muscle weakness (1). While the majority of MG patients have serum autoantibodies against the acetylcholine receptor (AChR), a subset of patients have autoantibodies against muscle-specific tyrosine kinase (MuSK) (2, 3). MuSK is essential for AChR clustering and synaptic differentiation (4). Through binding MuSK, serum-derived autoantibodies impair clustering of AChRs, and neuromuscular transmission, thereby causing disease (5).
Anti-CD20–mediated B cell depletion therapy (BCDT) is indicated for the treatment of multiple autoimmune disorders (6–8). The conspicuous clinical benefit of BCDT in patients with MuSK MG was recently demonstrated (9–11). However, a fraction of patients treated with the anti-CD20 BCDT agent rituximab (RTX) experienced postrituximab (post-RTX) relapses despite initial symptomatic responses and a fall in MuSK autoantibody titer (9, 12–14). Post-RTX relapse in MuSK MG is associated with the presence of circulating plasmablast expansions, which include those that secrete MuSK-specific autoantibodies (15, 16). Previous studies have shown that antigen-experienced plasma cells or plasmablasts are resistant to BCDT owing to their low CD20 expression (17–20). Moreover, memory B cells, which express CD20, can also be abundant after BCDT, and their increased frequency is associated with post-RTX relapses (21, 22). Whether or not plasmablasts, plasma cells, or memory B cells emerge from inadequately depleted circulating B cells (versus emerging entirely de novo) is not known. The characteristics of antigen-experienced B cells that resist depletion also remain unclear.
Understanding the mechanisms underlying post-RTX relapse is important for the development of new modalities for monitoring depletion and more effective therapy combinations for treating MG and other autoimmune disorders. Accordingly, we sought to further understand whether post-RTX relapse in MuSK MG is associated with the failed depletion of preexisting B cells, including autoantibody-producing B cell subsets. In addition, we investigated the phenotype of the B cells that persist throughout the course of treatment and relapse. To this end, we integrated data from multiple profiling methods on the same patients, including (a) adaptive immune receptor repertoire sequencing (AIRR-Seq) to generate high-depth B cell receptor (BCR) libraries from unselected PBMCs collected at pre-RTX treatment time points and during episodes of post-RTX relapse; (b) previously published sequences of human monoclonal autoantibodies, with known specificity for the MuSK autoantigen, that emerged during post-RTX relapses; and (c) single-cell gene expression analysis with paired BCR repertoire sequencing, to trace B cell populations temporally and to identify the phenotype of B cells that emerge during post-RTX relapse. In comparison with the examination of clonal overlap between bulk BCR repertoires alone, this approach, which we refer to as Single-cell Tracing of Adaptive Immune Receptor (STAIR) repertoires, allows for the unbiased characterization of the gene expression profile of persistent, disease-relevant B cells (including those specific for the autoantigen) at single-cell resolution. By using this approach, we offer new insights into the mechanism of post-RTX relapse in patients with MuSK MG and potential avenues for improving the treatment of MG and other autoantibody-driven autoimmune diseases.
Results
Experimental design.
We used the following experimental approach (Figure 1A) to identify and characterize B cell clones that resist RTX depletion in 3 patients with MuSK MG who experienced relapses. We first tested whether B cell clones persisted across pre- and post-RTX repertoires by sequencing the BCR repertoire of unselected PBMCs at high depth using a bulk approach to capture a large number of sequences for identifying persistent clones. Second, we tested whether MuSK autoantibody-producing B cells were among these persistent clones. In previous studies of these same patients, we produced MuSK-specific recombinant human monoclonal antibodies (15, 16) from single B cells isolated during post-RTX relapses. Here, though, we sought to determine whether those specific BCR clones were present in pre-RTX repertoires. Third, we identified the phenotype of both persistent and nonpersistent B cell clones. This was achieved through paired transcriptional profiling and BCR sequencing of single cells. These BCR sequences were traced to high-depth bulk sequencing repertoires derived from pre-RTX time points. The transcriptional profiles of persistent and nonpersistent B cell clones were then compared.
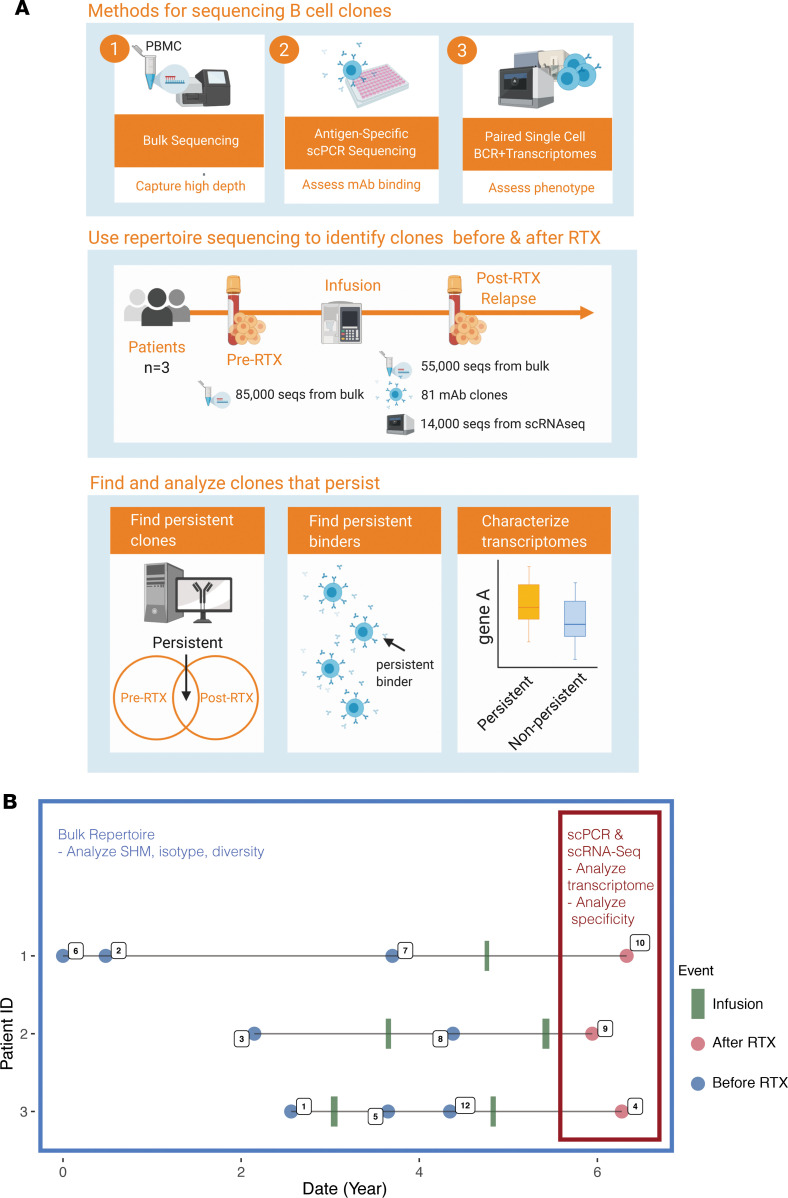
(A) Three approaches were used for sequencing. First, bulk repertoires using next-generation sequencing by isolating unpaired IGH V(D)J sequences directly from RNA were generated. Second, paired heavy and light chain V(D)J sequences from circulating B cells expressed as recombinant antibodies and tested for antigen specificity were traced to pre-RTX repertoires. Finally, paired transcriptome and heavy and light chain V(D)J sequence repertoires from high-throughput emulsion-based single-cell sequencing was performed. These 3 types of repertoires were analyzed together in our analysis workflow for each patient across the sampled time points. (B) Timeline of clinical events, specimen collection, and B cell analysis for 3 patients with MG who experienced post-RTX relapses. Infusion describes a time point associated with a recorded infusion of RTX. Post-RTX describes an event associated with a disease exacerbation during which B cells were observed to secrete MuSK-specific autoantibodies. Pre-RTX describes any collection time point preceding post-RTX by an intervening infusion event. Dates of events are approximations.
A subset of B cell clones are refractory to depletion.
We first sought to identify clones that escape RTX depletion and contribute to relapses when the B cell compartment repopulates after treatment. To that end, we sequenced the B cell repertoire, using our high-depth bulk approach, from 3 patients with MG before and after treatment (Figure 1B, Supplemental Table 1, and Supplemental Table 2; supplemental material available online with this article; https://doi.org/10.1172/jci.insight.136471DS1). The number of V(D)J sequences isolated for patient 1 was 639,715; 82,720 from patient 2; and 107,504 from patient 3. Grouping the sequences into clones — those with a common ancestor based on their V, J gene usage and junction sequences (23) — generated 187,195; 34,719; and 14,579 clones for patients 1, 2, and 3, respectively (Supplemental Figure 1). A total of 2462 clones were found with members that were present in both the pre-RTX and post-RTX relapse repertoire (termed persistent clones) in patient 1 (or 1.6% of pre-RTX clones); 902 (4.9%) and 345 (7.6%) were found in patients 2 and 3, respectively (Figure 2A). Given that clonal relationships are inferred computationally, we next sought to substantiate that these persistent clones were not the result of sequence similarity that happened by chance. To estimate the frequency at which clones are spuriously observed to be shared between the post-RTX and pre-RTX repertoires, the average background sharing between the post-RTX circulation and the pre-RTX circulation of unrelated individuals was calculated (e.g., post-RTX patient 1 was compared with pre-RTX patient 2). This rate was used as a null hypothesis to establish the significance of the observed clonal sharing between post-RTX and pre-RTX repertoires in the same patient. Patient 1 shared an average of 0.4% clones in comparison with other patients, patient 2 shared an average of 1.4%, and patient 3 shared an average of 0.9%. Quantification using a clonal overlap metric demonstrated that the observed overlaps between pre-RTX and post-RTX repertoires were significantly higher relative to the background (t test P = 0.037) (Figure 2B). These collective data demonstrate that circulating B cell clones persist despite RTX-mediated depletion of the repertoire.

(A) Venn diagrams show counts of clones present at pre-RTX and post-RTX time points and those that overlap both time points for all study subjects (the relative area of each circle corresponds to the proportion of clones found at different time points for each patient). (B) Shared B cell clones between pre-RTX and post-RTX time points quantified as Bray-Curtis overlap for the same patient (intrapatient) or across different patients (interpatient). Horizontal bars show the mean overlap of each comparison. A 1-tailed t test was used to assess the significance of the null hypothesis that intrapatient overlap was not higher than interpatient overlap. Overall distribution of frequencies of different isotypes (C) and average somatic hypermutation frequencies (D) among the set of sequences belonging to persistent and nonpersistent clones during post-RTX relapse for study subjects. Two-way ANOVA was performed to assess significance for an overall somatic hypermutation frequency difference and isotype usage frequency difference between nonpersistent compared with persistent clones across isotypes; specifically the effect of isotype, persistence, and interaction between the 2 was assessed for significance. Post hoc 2-tailed t tests were also performed, although no significant differences were observed in C and D. Data for the same n = 3 patients are shown for all panels. Violin plots are used in place of error bars to show the full range of values. Statistical differences are shown only when significant (***P < 0.001; **P < 0.01; *P < 0.05).
Persistent clones are antigen experienced.
Next we compared the V(D)J properties of nonpersistent clones with persistent clones that emerge post-RTX to investigate whether the latter have distinct characteristics that might contribute to their persistence (Figure 2, C and D). Given the possible role of persistent B cells in recognizing antigen, we characterized features of the repertoire associated with antigen experience, namely isotype switching and elevated somatic hypermutation (SHM) frequency. High-depth bulk repertoires were first examined to quantify the difference in isotype frequency among V(D)J sequences from persistent and nonpersistent post-RTX clones. Only B cells displaying an antigen-experienced phenotype were found to be persistent; when the approach shown in Figure 2B was applied to only B cells displaying a naive phenotype (unmutated IgM and IgD B cells), no clonal sharing was observed (1-tailed t test P = 0.317, data not shown). Furthermore, nonpersistent sequences were 43.2% IgM, 6.4% IgD, 24.7% IgG, and 25.5% IgA of the repertoire on average (Figure 2C), and persistent ones were disproportionately switched (15.0% IgM, 2.2% IgD, 27.4% IgG, and 55.0% IgA) (ANOVA P = 0.027). In addition, the overall SHM frequency of persistent clones was significantly elevated (Figure 2D) (ANOVA P = 0.014), and this difference in SHM frequency did not depend on the isotype of the sequence (ANOVA P = 0.287). Therefore, persistent clones are preferentially isotype switched with elevated SHM, reflecting a more antigen-experienced phenotype.
Persistent clones do not consistently expand or gain SHM.
We next sought to determine whether antigen experience (in terms of clonal expansion and SHM) was gained by persistent clones during reconstitution of the B cell compartment after RTX. To that end, the high-depth bulk repertoire was first examined to test whether persistent clones expanded between pre-RTX and post-RTX time points. Clonal expansion was assessed by computing the change in size of persistent clones between pre-RTX and post-RTX time points. Average decreases of –11% (95% CI –93% to +1313%), –85% (95% CI –98% to +439%), and –7% (95% CI –99% to +40.5%) in clonal size were observed across patients 1, 2, and 3, respectively (1-sample t test P = 0.37) (Supplemental Figure 2, A–C). Thus, decreases in clonal size were observed but these decreases were not significant. Given that persistent clones have elevated frequencies of SHM, we tested whether individual clones acquire additional SHM by quantifying the average change in SHM frequency of persistent clones between pre-RTX and post-RTX time points. The median change in SHM frequency was –0.2% (95% CI –89% to +1132%), –2.4% (95% CI –74% to 187%), and +4.5% (95% CI –41% to +67%) for patients 1, 2, and 3, respectively (Supplemental Figure 2, D–F); no consistent change in the SHM frequency of persistent clones between pre-RTX and post-RTX samples was detected (1-sample t test P = 0.807). These collective findings suggest that persistent clones are enriched for features of antigen experience pre-RTX but do not acquire further antigen experience between pre-RTX and post-RTX time points.
Some persistent clones are disease relevant and bind MuSK.
We then sought to investigate whether persistent B cell clones were autoantigen specific. In 2 previous studies, using the same 3 patients described here, we identified B cell clones during post-RTX relapse that were specific for autoantigen (Supplemental Table 3). In the first study, 43 unique B cell clones were used to generate recombinant monoclonal antibodies, all of which were derived from circulating plasmablasts because this cell type was demonstrated to produce MuSK-specific antibodies when cultured in vitro (15). In the second, a fluorescent MuSK antigen bait was used to both identify and isolate MuSK-specific B cell clones, from which an additional 38 recombinant monoclonal antibodies were produced (16). In total, 11 members of these collective 81 clones bound surface-expressed MuSK across a range of binding strengths when tested as monoclonal antibodies with a cell-based assay (15, 16). The BCR sequences of these clones were compared with the sequences in high-depth bulk repertoires to identify clonal relatives present both before and after treatment. Of these 81 clones, 27 were members of clones found in the bulk post-RTX BCR repertoires. This finding demonstrates that these clones were expanded because they were found in unique, independent sample aliquots. We tested whether members of these 81 clones were present in pre-RTX repertoires; members belonging to 74 clones were not observed. However, we observed that 7 clones could be found in pre-RTX repertoires (Figure 3 and Supplemental Figures 3–5). One of these clones was among the 11 previously demonstrated to specifically bind MuSK (Figure 3) (15). Thus, disease-relevant and MuSK autoantigen-specific B cells present during relapse can derive from the failed depletion of B cell clones that existed before therapy.
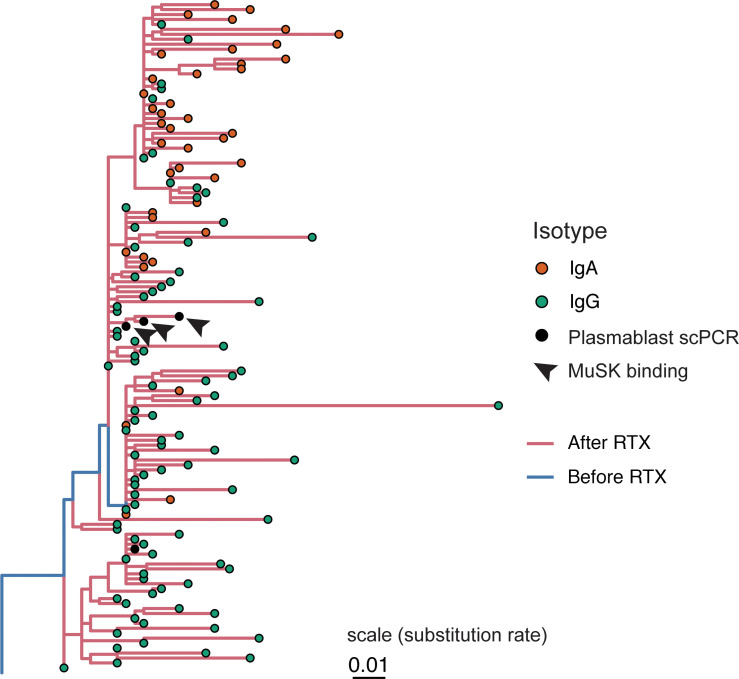
Sequences isolated from plasmablasts using single-cell PCR (scPCR) sequencing-based approaches and tested as monoclonal antibodies are denoted as “Plasmablast scPCR” (black dots); 3 of these bind MuSK and are denoted as “MuSK binding” (black arrowheads). Lineage tree topology and branch lengths were estimated using maximum parsimony, with edge lengths representing the expected number of nucleotide substitutions per site (see scale) as estimated using dnapars v3.967 (89). Tips are colored by antibody isotype, and each internal branch is colored by whether its descendant node was determined to have occurred in the pre- or post-RTX repertoire using a constrained maximum parsimony algorithm (see Methods).
ASC-like B cells are more persistent than memory B cells.
We next sought to characterize the transcriptional state of persistent B cells to determine their phenotype in an unbiased manner and quantify how different B cell subsets are represented among persistent populations. To accomplish this, we carried out single-cell transcriptome and paired BCR repertoire profiling of circulating post-RTX B cells. Owing to the high frequency of transitional B cells during post-RTX reconstitution, IgDlo B cells were selected by sorting before single-cell analysis (Supplemental Figure 6). Further, the analysis was limited to the switched IgG and IgA repertoire by computationally filtering out cells with V(D)Js paired with an IgD or IgM constant region. Clones were identified in the single-cell data sets that were related to clones present in pre-RTX high-depth bulk BCR repertoires. This allowed for direct interrogation of the transcriptomes of persistent B cells. That is, we leveraged the resolution offered by high-throughput single-cell transcriptomes and high depth offered by bulk BCR analysis to perform STAIR. From single-cell sequencing, a total of 18,133 cells associated with 14,671 IGH V(D)J sequences were identified (Supplemental Table 4) from the same 3 patients with post-RTX relapse and an additional asymptomatic AChR MG patient used as a control. Gene expression pattern and repertoire characteristics were used to partition the cells into 11 distinct clusters (Supplemental Figure 7, A–G, and Supplemental Table 5). Clusters of antibody-secreting cells (ASCs), memory B cells, mature naive B cells, and transitional B cells were assigned by analysis of the gene expression of these clusters (Figure 4A). Because B cells from the single-cell expression analysis could not be experimentally isolated to assess for antibody secretion or other functional features associated with plasmablasts/plasma cells, we chose to label the cluster most associated with a plasmablast/plasma cell signature as ASCs. By combining single-cell repertoires with high-depth bulk pre-RTX BCR repertoires, 820 persistent clones with members in the single-cell profiling data were identified. Persistent clones could be identified in both the ASC as well as memory B cell clusters. The ASC cluster had a higher frequency (27.9%) of persistent clones in comparison with the memory cluster (14.6%) (Figure 4, B and C) (t test P = 0.045). As further confirmation that some ASC and memory B cell clones were persistent, IGH sequences were also identified from single cells in the ASC and memory B cell cluster with identical V(D)J sequences as pre-RTX members (Figure 4B). Thus, these data suggest that while both ASCs and memory B cells can persist, members of the ASC subset are more likely than memory B cells to persist after RTX.
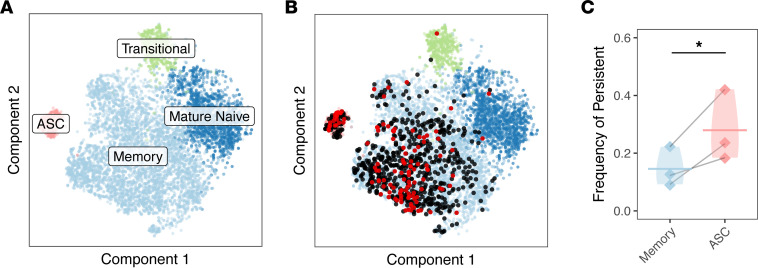
(A) Unbiased clustering of single-cell transcriptomes from all study subjects is visualized by t-distributed stochastic neighbor embedding (t-SNE) and colored based on B cell subset assignment. (B) V(D)J sequences associated with persistent B cell clones are visualized over the t-SNE plot labeled in A. B cells are colored black or red if the heavy chain V(D)J sequence paired with the cell is part of a persistent B cell clone. B cells are colored red if the heavy chain V(D)J sequence paired with the cell has the same V(D)J sequence as one present in a pre-RTX bulk repertoire and black if the clonal relative differs by 1 or more SHM. (C) The relative fractions of persistent compared with nonpersistent B cells for the memory B cell cluster compared with ASC cluster. A 1-tailed t test was used to assess the significance of the null hypothesis that ASCs would not be enriched compared with memory B cells. Horizontal bars show the average frequency of a given B cell subset across patients. Frequencies belonging to the same patient are paired with a gray line. Data for the same n = 3 patients are shown for all panels. Violin plots are used in place of error bars to show the full range of values. Statistical differences are shown only when significant (***P < 0.001; **P < 0.01; *P < 0.05).
ASCs have persistence-associated features compared with memory B cells.
We next sought to investigate phenotypic characteristics of ASCs compared with memory B cells during post-RTX relapse to further understand their mechanism of persistence and identify possible subsets of persistent ASCs. The expression of CD20, the target of rituximab, was first investigated. To test the association between the expression of CD20 and persistence of ASCs, CD20 expression was examined among B cell subsets. The ASC cluster was observed to express lower CD20 levels when compared with memory B cells (t test P = 0.021) (Figure 5A). However, when we examined the expression of CD20 on persistent ASCs compared with nonpersistent ASCs, a decrease was observed, but this trend did not reach significance (t test P = 0.15) (Figure 5B).

(A) Expression of log-normalized CD20 expression (Z score) visualized by intensity over t-SNE plot. (B) The expression of CD20 (the receptor target of RTX, gene symbol MS4A1) and BAFF or APRIL receptors (TACI or TNFRSF13B, BAFF-R or TNFRSF13C, BCMA or TNFRSF17) for persistent and nonpersistent members of the ASC cluster. Normalized gene expression values are computed from counts of gene expression transcripts. Paired 2-tailed t tests were used to test for the significant differential expression of each gene. Horizontal bars show the average expression of ASC cluster members for each patient for cells of a given status. (C) Clonal expansion expressed as Simpson’s diversity for ASC and memory B cell clusters. A 1-tailed t test was used to assess the significance of the null hypothesis that ASCs would not be less diverse than memory B cell clones. Horizontal bars show the average diversity of cluster members for each patient of memory B cells or ASCs. (D) Simpson’s diversity for persistent and nonpersistent members of the ASC cluster. A 1-tailed t test was used to assess the significance of the null hypothesis that persistent clones would not be less diverse than nonpersistent clones. Horizontal bars show the average diversity of ASC cluster members for each patient for cells of a given status. (E) The frequency of ASCs in post-RTX MuSK MG patients and a control AChR MG patient. The fraction of ASC cluster members is quantified as a ratio of the number of cells in the ASC cluster divided by the number of total B cells for each patient. A 1-sample t test was used to assess the significance of the null hypothesis that ASCs would not be more abundant in post-RTX samples compared with the asymptomatic AChR MG patient sample. Horizontal bars show the average frequency of ASC cluster members for each patient of a given status. Values belonging to the same patient are paired with a gray line for all graphs. Data for the same n = 3 patients are shown for all panels except panel E, which includes an asymptomatic patient sample (n = 4). Violin plots are used in place of error bars to show the full range of values. Statistical differences are shown only when significant (***P < 0.001; **P < 0.01; *P < 0.05).
BAFF (also known as B cell activating factor, B lymphocyte stimulator, or BlyS) is a TNF superfamily member produced almost exclusively by myeloid cells (24, 25). BAFF (and another TNF superfamily ligand, APRIL) can bind a family of B cell–specific receptors to promote B cell survival. Given that APRIL/BAFF receptor genes have been observed to play a role in mediating B cell survival after RTX depletion, we investigated the differential expression of APRIL/BAFF receptor genes (26–28). Persistent ASCs were not observed to differentially express APRIL/BAFF receptor genes (TACI, BAFF-R, and BCMA) (P = 0.085, 0.555, and 0.288 for TACI, BAFF-R, and BCMA, respectively) (Figure 5B).
To investigate other features that might distinguish persistent and nonpersistent ASCs, the relative usage of different isotypes and average SHM frequencies were examined. Persistent ASCs were also not observed to differ in terms of isotype usage (ANOVA P = 0.194) or frequency of SHM (ANOVA P = 0.101) compared with their nonpersistent counterparts (Supplemental Figure 8, A and B). The clonal diversity (more clonal expansions) of the ASC cluster was lower compared with memory B cells (t test P = 0.011) (Figure 5C). However, when we examined clonal diversity within the ASC cluster, a consistent difference between persistent ASCs compared with nonpersistent ASCs was not observed (t test P = 0.079) (Figure 5D). Finally, the fraction of cells in the ASC cluster was 10.0% of the total B cell repertoire in the post-RTX cohort (Figure 5E). This was significantly elevated compared with the 0.5% observed in the control asymptomatic AChR MG sample (1-sample t test P = 0.044). However, when we examined whether clonal expansion of persistent B cells between the pre-RTX and post-RTX time points could explain the abundance of ASC B cells that we observed post-RTX relapse, ASC B cell clones were not consistently found to expand (or acquire SHM); between pre-RTX and post-RTX time points, approximately half expanded and the other half shrunk. The average change in clonal size was therefore not consistent across patients (t test P = 0.099) (Supplemental Figure 9, A–F).
To summarize, persistent ASC cluster members were not observed to differ from nonpersistent members in terms of their expression of CD20 and receptors for BAFF/APRIL, clonal diversity, isotype usage, or SHM frequency. However, ASC cluster members expressed lower CD20 and were more clonally expanded than memory B cells overall; these data identify features of the ASC cluster as a whole that may explain their higher frequency among persistent clones.
Persistent memory B cell clones are expanded and express low levels of CD20.
While ASC clones were observed to be more persistent than memory cells, ASC members of the MuSK-specific clone identified previously (Figure 3) were also observed to be related to 2 members of the memory B cell cluster. Moreover, given the high frequency of persistent memory B cells observed overall, we next sought to explore whether persistent memory B cells had distinct features compared with nonpersistent memory B cells. We tested CD20 expression, expression of APRIL/BAFF receptors, SHM, isotype usage, and clonal diversity (Figure 6). Persistent memory B cells were observed to have significantly lower CD20 expression (t test P = 0.022). To investigate whether this difference in CD20 mRNA would be expected to correlate with surface CD20 expression, we examined B cells from a published data set generated using CITE-Seq (29, 30). CD20 surface expression was found to be significantly elevated in cells with higher CD20 mRNA expression across subjects (t test P = 5.1e-5, Supplemental Figure 10). Other features of persistent memory B cells included higher expression of TACI (t test P = 0.037) but lower expression of BAFF-R (t test P = 0.037) (Figure 6A). Analysis of SHM and isotype usage revealed that persistent memory B cells also had higher frequencies of SHM across isotypes (ANOVA P = 0.037) (Figure 6B); the fraction of persistent memory B cells expressing IgG1 (t test P = 0.022) and IgG3 (t test P = 0.025) (ANOVA P = 0.007) was less than that of their nonpersistent counterparts (Figure 6C). We next examined whether persistent memory B cells were clonally expanded compared with their nonpersistent counterparts. They were observed to have lower clonal diversity post-RTX, suggesting that they were clonally expanded (t test P = 0.030) (Figure 6D). Initial clustering of cells based on single-cell gene expression identified 6 distinct subclusters of memory B cells. To better characterize the gene expression features of persistent memory B cells, we examined the most abundant subcluster among persistent memory B cells. This cluster (cluster 3) (Figure 7, A and B) represented 36.7% of all persistent memory B cells but only 25.0% of all memory B cells (not the most prevalent overall, which was cluster 2) (Figure 7, B and C). Analysis of gene expression showed that cluster 3 was specifically characterized by lower CD20 expression (t test P = 0.005), higher TACI expression (t test P = 0.008), and lower BAFF-R expression (t test P = 0.014) compared with other memory B cell subclusters (Figure 7D and Supplemental Figure 11A). This cluster also displayed a higher frequency of SHM across all isotypes (ANOVA P = 1.46e-6) (Supplemental Figure 11B) and demonstrated increased usage of IgA compared with IgG isotypes (ANOVA P = 0.0103) (Supplemental Figure 11C) but was not more clonally expanded compared with other memory B cells (t test P = 0.169) (Supplemental Figure 11D).
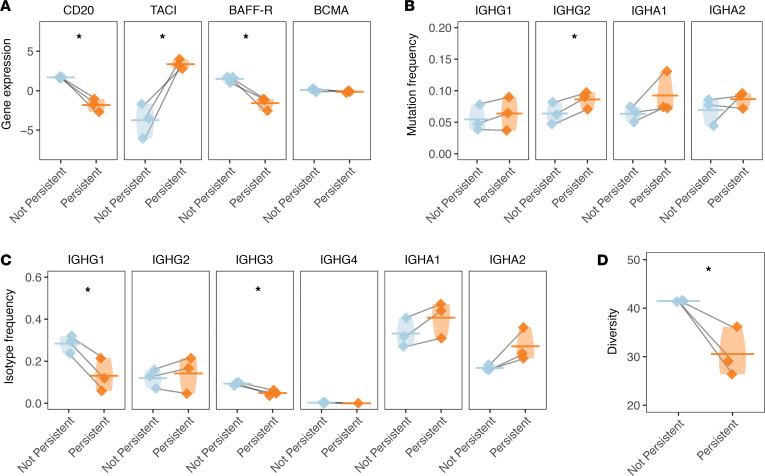
(A) The normalized gene expression of CD20 (the receptor target of RTX, gene symbol MS4A1) and BAFF or APRIL receptors (TACI or TNFRSF13B, BAFF-R or TNFRSF13C, BCMA or TNFRSF17) is presented for persistent and nonpersistent members of the memory B cell cluster for each patient for cells of a given status. Normalized gene expression values are computed from counts of gene expression transcripts. Paired 2-tailed t tests were used to test for the significant differential expression of each gene. (B) Individual SHM frequencies for persistent compared with nonpersistent cells are presented for memory B cell cluster members. Only mean SHM frequencies are computed for isotypes with more than 3 V(D)J sequences. Horizontal bars show the average SHM frequency for a given cluster. Paired 2-tailed t tests were used to assess the significance of differences in mutation frequency. (C) Overall constant region usage frequencies are quantified for persistent compared with nonpersistent memory B cell cluster members per patient. Horizontal bars show the average frequency of constant region usage across patients. Two-way ANOVA was performed to assess significance for an overall isotype usage difference between nonpersistent compared with persistent clones across isotype. Paired 2-tailed t tests were used to assess the significance for the differential usage of each constant region. (D) Clonal expansion expressed as Simpson’s diversity of persistent compared with nonpersistent memory B cell cluster members. A 1-tailed t test was used to assess the significance of the null hypothesis that persistent clones would not be less diverse than nonpersistent clones. Horizontal bars show the average diversity of memory B cell cluster members for each patient of a given status. Values belonging to the same patient are paired with a gray line for all graphs in this panel. Data for the same n = 3 patients are shown for all panels. Violin plots are used in place of error bars to show the full range of values. Statistical differences are shown only when significant (***P < 0.001; **P < 0.01; *P < 0.05).
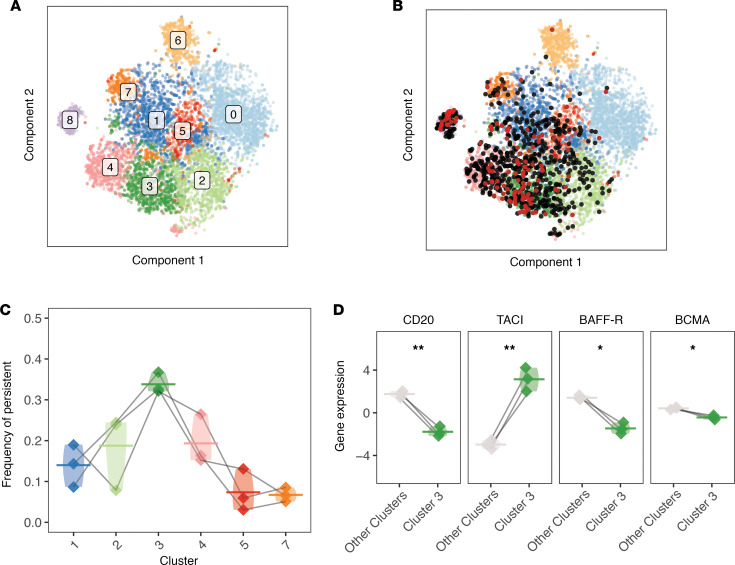
(A) Visualization of initial shared nearest-neighbor clustering of circulating B cells post-RTX by t-SNE with labels over clusters. (B) B cells that were clonally related to cells in the pre-RTX repertoire are indicated (red dot if the pre- and post-RTX V(D)J sequence is identical at the nucleotide level, and black dot otherwise). (C) The frequency of each cluster (by cell) among the set of persistent B cells is shown. (D) The expression of the receptor target of RTX, CD20 (MS4A1), is presented for members of cluster 3 and members of other memory B cell clusters. The expression of known receptors for BAFF and APRIL (TACI or TNFRSF13B, BAFF-R or TNFRSF13C, BCMA or TNFRSF17) is also presented. Differential expression of each gene was assessed using paired 2-tailed t tests. Horizontal bars show averages, with values belonging to the same patient paired with a gray line. Data for the same n = 3 patients are shown for all panels. Violin plots are used in place of error bars to show the full range of values. Statistical differences are shown in D (**P < 0.01; *P < 0.05).
Unbiased differential gene expression identified 1282 differentially expressed genes between memory cluster 3 (enriched for persistent clones) and all other memory B cell subclusters (Supplemental Figure 12 and Supplemental Data) (FDR < 0.05). The upregulated subset of these genes was enriched for a MYC signature (or c-Myc, a transcription factor that enhances B cell survival) and BRCA1/TP53 signature (transcription factors that regulate cell senescence and cell cycle) (Supplemental Figures 13 and 14) (31–33). The expression of 2 tissue homing genes that are the targets of the immunotherapy agents natalizumab and fingolimod (ITGA4 and S1PR1, respectively) was also observed as upregulated among cluster 3 memory B cells (P = 0.049 for ITGA4, and P = 0.039 for S1PR1) (Supplemental Data) (34). Thus, persistent memory B cells are clonally expanded and are characterized by a subset of B cells with elevated SHM, less IgG1 and IgG3 usage, high expression of TACI, low expression of CD20, and a gene expression pattern associated with transcription factors that control cell survival, proliferation, and senescence.
Discussion
Recent studies have demonstrated the remarkable benefits of RTX-mediated BCDT in MuSK MG; however, relapses have been observed in some patients and are associated with the presence of circulating plasmablasts that express MuSK-specific autoantibodies (9, 10, 15, 16, 35, 36). Through investigating the characteristics of B cells that emerge post-RTX, we sought to determine whether these disease-relevant B cells derive either from the failed depletion of preexisting B cell clones or from de novo generation.
We show that B cell clones, including MuSK-specific autoantibody-secreting B cells, emerge from the failed depletion of preexisting clones and that memory B cells serve as a reservoir for these cells, including antigen-specific members (Supplemental Figure 15). A potentially novel approach was used that combined multiple methods of sequencing the BCR repertoire with single-cell gene expression (STAIR) to demonstrate that persistent B cell clones are composed of expanded ASCs and memory B cell populations expressing low levels of CD20 associated with a unique gene expression profile. Although the persistence of B cell clones after RTX is not specific to MuSK MG relapse (37–39), MuSK-specific ASCs are found during MuSK MG relapse; we show here that these ASCs and their memory B cell precursors are incompletely depleted by RTX in a subset of patients with MG who experienced post-RTX relapses.
That many ASCs present after BCDT are related to preexisting populations is consistent with a large body of evidence showing that ASCs resist depletion owing to their low levels of CD20 expression and tissue localization (17, 19, 20, 40); their frequency predicts poor treatment response in patients with systemic lupus erythematosus (SLE) and rheumatoid arthritis (RA) (41–44). The finding that circulating memory B cell clones are also persistent is consistent with data showing that the frequency of memory B cells also predicts poor responses to RTX in SLE and RA (21, 22, 45). While these previous studies were important for identifying predictors of outcome, they were not designed to highly resolve B cell subsets because of limitations inherent with flow cytometry. By comparison, here, the definition of memory using single-cell transcriptomes does not rely on flow cytometry markers, but rather hundreds of markers that allow for accurate identification of common gene expression patterns associated with memory B cells. Furthermore, by applying STAIR, we were able to profile persistent memory B cell subsets in detail. A recent study using bulk AIRR-Seq to investigate the use of RTX for treating ANCA-associated vasculitis demonstrated that persistent clonotypes are isotype switched, which we also observed in our study (38). Here, persistence also correlated with a specific gene expression signature, that is, lower CD20 expression and differences in APRIL/BAFF receptor expression relative to other memory B cells.
Studies of RTX responses in IgM anti-myelin-associated glycoprotein peripheral neuropathy and pemphigus vulgaris (45–47) demonstrated that poor responses to RTX are associated with increased repertoire clonality post-RTX. Our results show that persistent memory B cells are more clonally expanded than nonpersistent memory B cells and suggest that the association between increased repertoire clonality and poor responses to RTX may result from the persistence of clonally expanded memory B cells.
Our work is congruent with studies that have shown that BAFF and the differential expression of receptors for APRIL/BAFF (TACI, BAFF-R, BCMA) on antigen-experienced B cells may lead to poor outcomes after BCDT (26–28, 48). BAFF (also known as B cell activating factor, B lymphocyte stimulator, or BlyS) is a TNF superfamily member produced almost exclusively by myeloid cells (24, 25). BAFF (and APRIL) can bind a family of B cell–specific receptors to promote B cell survival. A higher frequency of memory B cells expressing lower BAFF-R can be detected in patients with RA relapsing after RTX (28) as well as in patients with graft-versus-host disease who respond poorly to RTX (27). This is consistent with our observation that persistent memory B cells express lower BAFF-R levels. Further, BAFF levels have been shown to negatively correlate with BAFF-R expression after RTX therapy in primary Sjögren’s syndrome and SLE (48), and exposure of B cells to soluble BAFF decreases BAFF-R in vitro. By comparison, exposure to anti-BAFF increases the expression of BAFF-R on B cells (49). By directly characterizing B cell clones that resist RTX depletion, we are able to show that memory B cell subsets that persist after RTX express surface markers associated with poor responses to RTX during relapse.
Our analysis showed that ASCs and cluster 3 memory B cells during relapse were predominantly IgA switched. We speculate that the abundance of IgA-switched B cells could be related to BAFF expression given the known role of BAFF in class switch recombination, particularly in the gut (50–52). The presence of IgA-switched circulating ASCs has been shown to depend on BAFF (53). Moreover, IgA-secreting plasma cells reduce disease severity in an experimental autoimmune encephalomyelitis model, owing to their production of IL-10 (54). Thus, further characterization of how the induction of IgA-producing B cell subsets by RTX occurs is needed to investigate alternative mechanisms of BCDT-mediated therapeutic benefit.
The conclusions of this study offer insights into therapeutic and diagnostic avenues for patients who experience relapses after RTX. Our findings regarding the BAFF/APRIL system suggest that combining RTX with BAFF pathway inhibitors (e.g., belimumab) could offer ways to deplete B cells that persist after RTX, including autoantibody producers. Combination strategies involving both belimumab and RTX were successful in a phase IIa randomized clinical trial in SLE, now followed by an ongoing phase III trial (55, 56). A combination approach using belimumab and RTX has also shown promise for treating Sjögren’s syndrome (57). This study by no means validates the use of combination therapy targeting BAFF as standard of care, nor do we argue that this is the only approach to combination therapy; further investigations are warranted to better understand such strategies. Mechanisms underlying RTX resistance are complex and may not be limited to B cell persistence. For example, a suggested role for variable complement activity could alter RTX effectiveness (58). Furthermore, concomitant statin treatment may introduce conformational changes in the CD20 receptor that impair RTX binding or disturb the integrity of RTX-induced translocation of CD20 into lipid rafts, both of which are important for cytotoxicity (59, 60).
We also observed that upregulated genes associated with the subset of memory B cells most resistant to depletion (cluster 3) included those involved in tissue homing (ITGA4, S1PR1). S1P receptor is upregulated in lymphocytes localized to lymph nodes and inflamed tissues and a target of the immunotherapeutic fingolimod. ITGA4 is a component of VLA-4, an integrin dimer also responsible for the localization of leukocytes to inflamed tissue, and target of natalizumab (34). We speculate this could reflect the tissue-associated origin for this subset. This is consistent with evidence showing that lymph nodes, spleen, bone marrow, and tonsils harbor RTX-resistant memory B cells (21, 61–63). Therapies such as engineered T cell technology, including chimeric-antigen receptor or chimeric-autoantibody receptor T cell therapy (64, 65), and other methods for eliminating tissue-localized subsets may be well suited for preventing and treating relapses.
Existing AIRR-Seq approaches for generating immune repertoires commonly begin with genomic DNA or mRNA and produce high-depth bulk repertoires of unpaired immunoglobulin or T cell receptor V(D)J sequences (66–68). More recent approaches use single-cell isolation techniques with high-throughput sequencing to construct repertoires, sometimes with paired gene expression (69–72). The use of these single-cell-based approaches was included in studies on tumor-infiltrating lymphocytes from cancer biopsies, infiltrates from the brains of mice, and B cells from the spleens of mice after vaccination in mice (70–72). No single-cell repertoire studies have investigated the B cell repertoire in autoimmune disease thus far to our knowledge. Our ability to extract biologically meaningful results from STAIR analysis suggests that tracing individual BCR sequences associated with relapse could provide a powerful tool for monitoring patient responses to immunotherapies that deplete B and T cells. Moreover, in comparison with techniques that use bulk BCR repertoire sequencing to monitor therapy progress, this approach allows for the individual characterization of lymphocytes that resist therapy based on gene expression, which may suggest alternative avenues for treatment (73).
Several limitations of this study should be considered. First, the single-cell gene expression of B cells was characterized only after RTX depletion. Determining the phenotype of B cells that resist RTX depletion before therapy may be of interest to identify resistant subsets for targeting before relapse. A recent study demonstrated that not only do B cell clones move between peripheral blood and cerebrospinal fluid over the course of immunotherapy in some patients with multiple sclerosis (MS), but also many have both memory and plasma cell members (74). Similar studies using single-cell transcriptomics could identify memory B cell subsets that give rise to autoantibody-producing plasmablasts during relapse. Second, our results rely on tracing B cell clones by analyzing their IGH V(D)J sequences alone. While we (and others) have demonstrated that clonal relationships can be reliably inferred given the diversity of IGH V(D)J sequences, false positives may occur (23, 75, 76). Third, owing to the abundance of transitional B cells that repopulate the repertoire during RTX relapse and our interest in studying antigen-experienced B cell subsets, we excluded IgD+ B cells from our single-cell analysis. Nevertheless, a small fraction of BCRs paired with an IGHD constant region (3.2%–11.4%) was found in our single-cell data. This is likely because flow-based sorting is not absolute in eliminating gated populations. Fourth, the expressed mRNA transcriptome may not always reflect the expression of surface markers used for sorting. Nevertheless, in the specific case of CD20, we showed a consistent correlation between CD20 mRNA expression and CD20 surface expression; low expression of surface CD20 has been associated with resistance to RTX depletion in murine models (17, 20). Fifth, we were limited to a cohort of 3 patients owing to the rarity of MuSK MG relapse after RTX. This limitation was weighed against the benefits of studying a disease directly caused by antigen-specific antibodies, permitting more definitive statements about the contribution of persistent antigen-specific B cells to clinical relapse. Nevertheless, because of the small cohort size, caution should be taken when generalizing the results of this study to patients with MuSK MG. Finally, although we previously generated human recombinant MuSK-specific monoclonal antibodies from B cells present during post-RTX relapse, these monoclonal antibodies recognized MuSK with different binding capacities ranging from weak to very strong (15, 16). The one we were successful in tracing to the pre-RTX repertoire in the present study was among those showing weaker binding capacity. Accordingly, we can only speculate on its pathogenic capacity in vivo. That it was a member of a large expanded clone with multiple clonal variants may suggest that this particular clonal member represented an intermediate step toward a higher binding disease-causing relative.
The reported results motivate future investigations. By combining the depth offered by bulk repertoire sequencing with the resolution of single-cell repertoire and gene expression, STAIR analysis allows for the characterization of B cells present at multiple time points in detail. Extending these observations to studies where anti-CD20 therapy has strong evidence for efficacy, such as MS, may be particularly worthwhile. Applying STAIR analysis to the simultaneous investigation of other therapies, such as anti-CD19 therapy (inebilizumab) — recently shown to be efficacious for the treatment of neuromyelitis optica — could allow for the optimal selection of B cell–depleting agents for preventing disease relapse (77). While RTX is a reliable treatment modality for MuSK MG, data for AChR MG has been mixed and patient relapses do occur (12). An investigation of factors that contribute to relapse after RTX in the context of AChR MG using similar approaches could lead to the development of improved therapeutics for diagnosing and treating patients with MG regardless of disease subtype.
Methods
Patient-derived specimens.
Peripheral blood was collected from subjects with MG (Yale Myasthenia Gravis Clinic, New Haven, Connecticut, USA). A MuSK MG cohort (n = 3) was identified for investigation of post-RTX relapses with these characteristics that we have described previously: (a) an RTX-induced complete stable remission, minimal manifestation (CSR/MM) clinical status (>1 year); (b) repopulation of the B cell compartment after RTX; and (c) disease relapse following sustained CSR/MM after RTX (15, 16). Selected patients may have been treated with RTX before study entry. All pre-RTX sample collection time points precede post-RTX time points by at least 1 infusion of RTX. An additional asymptomatic (by Myasthenia Gravis Foundation of America classification score) AChR MG control subject was also included.
Bulk library preparation.
RNA was prepared from frozen PBMCs using the RNeasy Mini Kit (QIAGEN) per the manufacturer’s instructions. Cells were pelleted and lysed in freezing medium without washing to preserve cell populations sensitive to cryopreservation. RNA libraries were made using reagents supplied by New England BioLabs (a gift from Eileen Dimalanta and Chen Song, Ipswich, Massachusetts, USA) as part of the NEBNext Immune Sequencing Kit. IGH library preparation and sequencing were performed in the same manner as previously published using only primers targeting IGHA, IGHD, IGHE, IGHG, and IGHM regions (78).
Raw read quality control and assembly for bulk libraries.
Bulk repertoire data processing and analysis was carried out using tools in the Immcantation framework (http://immcantation.org). Preprocessing was carried out using pRESTO v0.5.10 (79) as follows: (a) Reads with a mean Phred quality score below 20 were removed. (b) Reads were aligned against constant region primer and template switch sequences, with a maximum mismatch rate of 0.2 and 0.5, respectively. Reads failing to match both a constant region primer and template switch sequence were removed. (c) Remaining reads were grouped based on unique molecular identifier (UMI) sequences determined by the 17 nucleotides preceding the template switch site. (d) Separate consensus sequences for the forward and reverse reads within each UMI group were constructed with a maximum error score of 0.1 and minimum constant region primer frequency of 0.6. If multiple constant region primers were associated with a particular UMI group, the majority primer was used. (e) Forward and reverse consensus sequence pairs were aligned to each other with a minimum overlap of 8 nucleotides and a maximum mismatch rate of 0.3 and assembled into full-length sequences. Sequence pairs that failed to align to each other were assembled by alignment against the ImMunoGeneTics (IMGT) human germline IGHV reference database (IMGT/GENE-DB v3.1.19; retrieved June 21, 2018; http://www.imgt.org/genedb/) with a minimum overlap of 0.5 and an E value threshold of 1 × 105. (f) Isotypes were assigned by local alignment of the 3′ end of each consensus sequence to isotype-specific constant region sequences with a maximum mismatch rate of 0.4. (g) Duplicate sequences were removed, except those spanning multiple biological samples or with different isotype assignments. Constant region primers, isotype-specific internal constant region sequences, and template switch sequences used are available at https://bitbucket.org/kleinstein/immcantation
Single-cell library preparation and gene expression analysis.
B cells were isolated from PBMCs by EasySep Human Pan-B cell Enrichment Kit (immunomagnetic negative selection kit, STEMCELL Technologies) per the manufacturer’s instructions. Additional fluorescence-activated cell sorting was then performed using a FACSAria flow cytometer (Becton Dickinson, Mountain View, California, USA) and visualized using the python package FlowCytometryTools v0.5.0; B cells labeled with 7AAD Viability Staining Solution (BioLegend) and FITC-labeled anti-IgD antibody (mouse anti-human monoclonal antibody, BioLegend clone IA6-2) were removed to exclude nonviable cells and all naive B cell populations. Sorted B cells were then loaded into the Chromium Controller (10x Genomics). Single-cell gene expression libraries were prepared using the Chromium Single-cell 5′ Reagent Kit (10x Genomics) for version 1 chemistry per the manufacturer’s protocol. Samples were sequenced on the NovaSeq 6000 Sequencing System (Illumina) with 100 × 100 or 150 × 150 paired-end reads for gene expression and BCR libraries, respectively. Base calls were converted to FASTQ sequences, demultiplexed using the cellranger mkfastq function from 10x Genomics 2.2.0, and aligned to the GCRhg38 genome supplied by 10x Genomics. Sparse count matrices, bar code assignments, and feature calls were prepared using the cellranger count function. Seurat v.2.3.4 was used for gene expression analysis. Cells with fewer than 50 genes detected, with mitochondrial content above 0.2 of all transcripts, or expressing detectable CST3, CD3E, KLRB1, NKG7, and GATA2 transcripts (to remove non-B cell populations like T cells and myeloid cells) were excluded. Single-cell gene expression values were computed from log-normalized count values (80). A total of 12,534 cells were identified after filtering. t-SNE was computed after identifying highly variable genes using FindVariableGenes and correcting for batch effects using the first 20 dimensions from canonical correlation analysis after visualization of components with MetageneBicorPlot. The first 10 dimensions were used for principal components analysis based on the location of the inflection point from PCElbowPlot. FindClusters was then used to identify clusters in Seurat v.2, which uses shared nearest-neighbor clustering (80).
Cell subsets were identified using a “basis set” of published marker genes called immunoStates (81). The mean expression of each gene was computed for each cluster from the scaled log-normalized expression values; each cluster was then assigned to the immunoStates subset with the highest Pearson correlation coefficient. The absence of transitional B cells in the immunoStates data set meant this cluster was identified manually using marker genes alone. Small clusters with elevated expression of mitochondria-associated genes were also excluded from further analysis. We confirmed our assignments by visualizing the expression of known gene expression markers, for instance, CD27 (for memory B cells), MS4A1 (CD20, for B cells in general except plasma cells), MME (CD10, for transitional B cells), and PRDM1 (BLIMP1 for plasma cells) (Supplemental Figure 7, A–C). Overall marker expression was also visualized based on the percentage of cells in each cluster expressing the gene and the overall mean level of gene expression in each cluster (Supplemental Figure 7, D and E). To further confirm the identities of these single-cell clusters, we assessed their paired IGH repertoire features for mean SHM frequency and isotype usage, which were consistent with expected values (Supplemental Figure 7, F and G).
Bulk BCR repertoire V(D)J gene annotation, additional sequence filtering, clonal assignment, and germline reconstruction for STAIR.
Reconstructed V(D)J sequences from single-cell sequencing were extracted using the cellranger vdj function from FASTQ reads. Single-cell V(D)J BCR repertoires as well as previously published scPCR-derived V(D)J sequences were pooled with bulk V(D)J BCR repertoires (15, 16). V(D)J germline assignments were made with IgBLAST v1.7.0 (82) using the June 21, 2018, IMGT reference gene database from all repertoires. Cells with multiple IGH V(D)J sequences were assigned to the most abundant IGH V(D)J sequence by UMI count. Only functional V(D)J sequences were assigned into clonal groups using Change-O v0.3.4 (23). Sequences were first partitioned based on common IGHV and IGHJ gene annotations and junction lengths. Within groups, sequences differing by a length-normalized Hamming distance of 0.126 were defined as clones by single-linkage clustering. This distance threshold was determined based on the average local minima between the 2 modes of the within-sample bimodal distance-to-nearest histogram for the 3 patients across multiple time points; thresholds were determined using a kernel density estimate in the same manner described previously (23). Germline sequences were then reconstructed for each clonal cluster (VH) with D segment and N/P regions masked (replaced with Ns) using the CreateGermlines.py function within Change-O v0.3.4.
Differential gene expression analysis.
Single-cell expression values were imputed using the ALRA algorithm to account for dropping out (83). Any gene with a mean expression value less than 0.1 across all cells after imputation was excluded. The gene expression of each gene per cell was subtracted by the mean expression of the gene for that sample; the average expression of each gene was then calculated for all cells of a given status (e.g., persistent vs. nonpersistent) to generate a matrix of pseudobulk expression values. This matrix was further Z score normalized by status. Paired 2-tailed t tests were performed to assign P values. FDR correction was performed using Storey’s method implemented within the qvalue package v2.10.0 in R from Bioconductor (for 12,066 P values from cluster 3–associated genes) (84). The enrichr package v1.0 in R was used for gene ontology analysis, which implements Wilcoxon’s signed rank test for significance (85). A q value (corrected P value) threshold of 0.05 was used for assigning significance.
CITE-Seq data used to investigate the association between CD20 mRNA (MS4A1) and surface protein expression levels were downloaded from the source publication (patients were healthy, between 18 and 45, and derived from a study on influenza vaccination, and samples were collected as frozen PBMCs; 10 patient samples were examined) (30). A cluster corresponding to non-ASC B cells (cluster K1) was extracted from the data object (cells from cluster C3); mRNA was normalized for each sample by Z score (as above). Cells were identified as CD20 mRNA high or low based on whether they fell above or below the 50th percentile in terms of CD20 mRNA expression, respectively.
Analysis of SHM, diversity, and clonal overlap from BCR repertoires.
Mutations relative to the germline sequence were quantified using ShazaM v0.1.8 in R v3.4.2 (86). Diversity analysis was performed using Alakazam v0.2.11 and assessed using the generalized Hill index, with uniform downsampling [to the number of V(D)J from the sample with the fewest sequences to account for different sequencing depth] and 2000 replicates of bootstrapping from the inferred complete clonal abundance (87). Clonal overlap was computed using a Bray-Curtis metric implemented by the function scipy.spatial.distance.braycurtis in scipy v1.1.0 (88).
Inference of B cell lineage trees and prediction of intermediate time points.
B cell lineage tree topologies and branch lengths were estimated using the dnapars program distributed as part of PHYLIP (v3.697) (89). A maximum parsimony algorithm was used to label the internal nodes of each lineage tree as either pre-RTX or post-RTX, given the date of sampling of each sequence at the tree’s tips. This was accomplished using an implementation of the Sankoff parsimony algorithm (90) in which switches from post-RTX to pre-RTX are forbidden using a cost matrix that weights post-RTX to pre-RTX switches as 1000 times more costly than switches in the opposite direction. Clusters of internal nodes separated by 0-length branches (polytomies) were reordered using nearest-neighbor interchange moves to minimize the number of label switches along the tree. These analyses were performed using IgPhyML v1.1.1 (91). Trees were visualized using the R packages ape v5.3 (92) and ggtree v2.0.4 (93).
Data availability.
Gene expression and repertoire data in this study are available in the National Center for Biotechnology Information’s Gene Expression Omnibus (GEO) database through GEO series accession number GSE149133.
Statistics.
R v3.4.2 (86) and Python 3.5.4 were used for all statistical analysis (whereas phylogenetic analysis was performed using R v3.6.3). Venn diagram plotting was performed using the R package VennDiagram v1.6.20. Data frame handling and plotting were performed using functions from the tidyverse 1.2.1 in R and pandas 0.24.2, scipy 1.1.0, and matplotlib 2.2.2 in Python. All parametric statistical testing aside from that used for differential gene expression analysis (described previously) was performed in R using the aov function for 2-way ANOVA or t.test functions for paired 2-tailed t tests (or 1-tailed when appropriate). A significance threshold of P < 0.05 was shown on plots with a single asterisk; double asterisks correspond to P < 0.01, and triple asterisks correspond to P < 0.001. P values less than 0.05 were considered significant. Error bars are not presented in our analysis; violin plots are shown to highlight the full range of the distribution for all figures instead.
Study approval.
This study was approved by Yale University’s Institutional Review Board. Written informed consent was received from all participating patients before inclusion in this study.
Author contributions
RJ, PS, SHK, and KCO designed the study. RJ, MCP, MLF, and PS performed all the experiments. RJ and KBH analyzed the data. RJ, MLF, KBH, RJN, PS, SHK, and KCO wrote the manuscript. RJN, SHK, and KCO provided resources, reagents, and funding.
Supplementary Material
Acknowledgments
This project was supported by the National Institute of Allergy and Infectious Diseases of the NIH through grant awards to KCO, under award numbers R01-AI114780 and R21-AI142198, and to SHK, under award number R01-AI104739; by a Neuromuscular Disease Research program award from the Muscular Dystrophy Association to KCO under award number MDA575198; and by the James Hudson Brown - Alexander Brown Coxe Postdoctoral Fellowship in the Medical Sciences (to MLF). KBH was supported through a PhRMA Foundation Postdoctoral Fellowship in informatics. PS was partly supported by the Onassis Foundation under the Special Grant and Support Program for Scholars’ Association members (R ZO 006/2018-2019). We thank Jason Vander Heiden for assistance with developing methods for processing V(D)J sequences from 10x Genomics sequencing. We thank Karen Boss and Abeer Obaid for helpful feedback on the text. We thank Eileen Dimalanta and Chen Song from New England BioLabs for providing reagents for the preparation of bulk BCR libraries.
Version Changes
Version 1. 06/23/2020
In-Press Preview
Version 2. 07/23/2020
Electronic publication
Footnotes
Conflict of interest: KCO has received research support from Ra Pharmaceuticals, Inc. (now part of UCB), and is a consultant and equity shareholder of Cabaletta Bio. KCO is the recipient of a sponsored research subaward from the University of Pennsylvania, the primary financial sponsor of which is Cabaletta Bio. SHK receives consulting fees from Northrop Grumman. RJN has received research support from Alexion Pharmaceuticals, Genentech, Grifols, and Ra Pharmaceuticals, Inc., and has served as a paid consultant for Alexion Pharmaceuticals; Momenta; Ra Pharmaceuticals, Inc.; Roivant Sciences; Shire (now part of Takeda); and Grifols. MLF has received research support from Grifols.
Copyright: © 2020, American Society for Clinical Investigation.
Reference information: JCI Insight. 2020;5(14):e136471.https://doi.org/10.1172/jci.insight.136471.
References
Articles from JCI Insight are provided here courtesy of American Society for Clinical Investigation
Full text links
Read article at publisher's site: https://doi.org/10.1172/jci.insight.136471
Read article for free, from open access legal sources, via Unpaywall:
http://insight.jci.org/articles/view/136471/files/pdf
Citations & impact
Impact metrics
Citations of article over time
Alternative metrics
Smart citations by scite.ai
Explore citation contexts and check if this article has been
supported or disputed.
https://scite.ai/reports/10.1172/jci.insight.136471
Article citations
The Type 1 Diabetes T Cell Receptor and B Cell Receptor Repository in the AIRR Data Commons: a practical guide for access, use and contributions through the Type 1 Diabetes AIRR Consortium.
Diabetologia, 29 Oct 2024
Cited by: 0 articles | PMID: 39467874
Different Patterns of Autoantibody Secretion by Peripheral Blood Mononuclear Cells in Autoimmune Nodopathies.
Neurol Neuroimmunol Neuroinflamm, 11(5):e200295, 22 Aug 2024
Cited by: 1 article | PMID: 39173087 | PMCID: PMC11379437
nf-core/airrflow: An adaptive immune receptor repertoire analysis workflow employing the Immcantation framework.
PLoS Comput Biol, 20(7):e1012265, 26 Jul 2024
Cited by: 1 article | PMID: 39058741 | PMCID: PMC11305553
Effectiveness and safety of telitacicept for refractory generalized myasthenia gravis: a retrospective study.
Ther Adv Neurol Disord, 17:17562864241251476, 14 May 2024
Cited by: 0 articles | PMID: 38751755 | PMCID: PMC11095194
B-cell immune dysregulation with low soluble CD22 levels in refractory seronegative myasthenia gravis.
Front Immunol, 15:1382320, 22 Apr 2024
Cited by: 0 articles | PMID: 38711503 | PMCID: PMC11071663
Go to all (34) article citations
Data
Data behind the article
This data has been text mined from the article, or deposited into data resources.
BioStudies: supplemental material and supporting data
GEO - Gene Expression Omnibus
- (1 citation) GEO - GSE149133
Similar Articles
To arrive at the top five similar articles we use a word-weighted algorithm to compare words from the Title and Abstract of each citation.
Autoantibody-producing plasmablasts after B cell depletion identified in muscle-specific kinase myasthenia gravis.
JCI Insight, 2(17):94263, 07 Sep 2017
Cited by: 49 articles | PMID: 28878127 | PMCID: PMC5621905
Mechanisms underlying B cell immune dysregulation and autoantibody production in MuSK myasthenia gravis.
Ann N Y Acad Sci, 1412(1):154-165, 01 Jan 2018
Cited by: 27 articles | PMID: 29381221 | PMCID: PMC5793885
Review Free full text in Europe PMC
Reemergence of pathogenic, autoantibody-producing B cell clones in myasthenia gravis following B cell depletion therapy.
Acta Neuropathol Commun, 10(1):154, 28 Oct 2022
Cited by: 10 articles | PMID: 36307868 | PMCID: PMC9617453
Short- and Long-Lived Autoantibody-Secreting Cells in Autoimmune Neurological Disorders.
Front Immunol, 12:686466, 17 Jun 2021
Cited by: 20 articles | PMID: 34220839 | PMCID: PMC8248361
Review Free full text in Europe PMC
Funding
Funders who supported this work.
Muscular Dystrophy Association (1)
Grant ID: MDA575198
NCATS NIH HHS (1)
Grant ID: UL1 TR001863
NIAID NIH HHS (3)
Grant ID: R01 AI104739
Grant ID: R01 AI114780
Grant ID: R21 AI142198
NIGMS NIH HHS (1)
Grant ID: T32 GM007205
National Institute of Allergy and Infectious Diseases (1)
Grant ID: R01-AI114780,R21-AI142198,R01-AI104739