Abstract
Background
It was demonstrated in our previous research that trypsin scavenges superoxide anions. In this study, the mechanisms of storage quality improvement by trypsin were evaluated in H. undatus.Results
Trypsin significantly delayed the weight loss and decreased the levels of ROS and membrane lipid peroxidation. Transcriptome profiles of H. undatus treated with trypsin revealed the pathways and regulatory mechanisms of ROS genes that were up- or downregulated following trypsin treatment by gene ontology (GO) and Kyoto Encyclopedia of Genes and Genomes pathway (KEGG) enrichment analyses. The current results showed that through the regulation of the expression of hub redox enzymes, especially thioredoxin-related proteins, trypsin can maintain low levels of endogenous active oxygen species, reduce malondialdehyde content and delay fruit aging. In addition, the results of protein-protein interaction networks suggested that the downregulated NAD(P) H and lignin pathways might be the key regulatory mechanisms governed by trypsin.Conclusions
Trypsin significantly prolonged the storage life of H. undatus through regulatory on the endogenous ROS metabolism. As a new biopreservative, trypsin is highly efficient, safe and economical. Therefore, trypsin possesses technical feasibility for the quality control of fruit storage.Free full text

Transcriptomic analysis reveals hub genes and subnetworks related to ROS metabolism in Hylocereus undatus through novel superoxide scavenger trypsin treatment during storage
Abstract
Background
It was demonstrated in our previous research that trypsin scavenges superoxide anions. In this study, the mechanisms of storage quality improvement by trypsin were evaluated in H. undatus.
Results
Trypsin significantly delayed the weight loss and decreased the levels of ROS and membrane lipid peroxidation. Transcriptome profiles of H. undatus treated with trypsin revealed the pathways and regulatory mechanisms of ROS genes that were up- or downregulated following trypsin treatment by gene ontology (GO) and Kyoto Encyclopedia of Genes and Genomes pathway (KEGG) enrichment analyses. The current results showed that through the regulation of the expression of hub redox enzymes, especially thioredoxin-related proteins, trypsin can maintain low levels of endogenous active oxygen species, reduce malondialdehyde content and delay fruit aging. In addition, the results of protein-protein interaction networks suggested that the downregulated NAD(P) H and lignin pathways might be the key regulatory mechanisms governed by trypsin.
Conclusions
Trypsin significantly prolonged the storage life of H. undatus through regulatory on the endogenous ROS metabolism. As a new biopreservative, trypsin is highly efficient, safe and economical. Therefore, trypsin possesses technical feasibility for the quality control of fruit storage.
Graphical abstract

Background
Hylocereus undatus (H. undatus) is a perennial climbing cactus plant that is native to tropical areas of Mexico and Central and South America [1]. H. undatus is a nonclimacteric fruit that reaches its best edible quality when harvested ripe, and its quality decreases during storage. The shelf life of fresh H. undatus is short. As a newly cultivated crop, few studies have aimed to extend the postharvest quality of this fruit [2].
Disorder of reactive oxygen species (ROS) metabolism and excessive accumulation of ROS causes an increase of membrane lipid peroxidation and leads to fruit spoilage during the process of fruit ripening and decay [3, 4]. Trypsin is a serine protease and is used as a proteolytic enzyme. It was shown that the presence of trypsin significantly affects the activity of flavonoids in scavenging 2,2-diphenyl-1- picrylhydrazyl (DPPH), 2, 2-azinobis (3-ethylbenzo- thiazoline-6-sulphonic acid; ABTS) and hydroxyl radicals [5]. We also reported that trypsin can protect cells by scavenging superoxide anions (O2−) [6]. The function and mechanisms of the impact of trypsin on the quality of H. undatus during storage have not been determined to date.
RNA-seq is a highly efficient technology for gene expression analysis [7, 8]. For plants, RNA-seq has been widely used to identify regulatory mechanisms and screen target genes in the area of phytopathology [9, 10]. However, few such studies have been performed with respect to gene expression related to postharvest technology of fruits and vegetables. Although the hub genes involved in the biosynthesis of betalain have been identified in H. undatus [11], the regulatory mechanisms of postharvest quality of fruits and vegetables have not been elucidated to date.
The analysis of protein-protein interactions (PPI) enables us to further elucidate the biological processes, organization, and action mechanisms of proteins [12]. Cytoscape is an open platform with a series of plugins to make it more visualized and able to perform deep network analysis. A number of the plugins of Cytoscape, such as NetworkAnalyzer, MCODE, or cytoHubba, could be employed to score and rank the nodes or screen modules in the PPI network [13].
In the current study, we investigated the impact of trypsin on the quality and shelf life of H. undatus by regulating active oxygen metabolism. The differentially expressed ROS genes (DERGs) of H. undatus peel samples were obtained. GO and KEGG enrichment analyses of DERGs were applied, and the PPI network of ROS related genes and subnetwork of DERGs were constructed. The hub genes related to ROS mechanisms of fruit quality improvement by trypsin during storage were further analyzed by Cytoscape with such plugins as cytoHubba and MCODE.
Results
Effect on storage quality of H. undatus
The H. undatus fruits of both groups were in excellent condition at the beginning of storage (Fig. 1). After 159h of storage, the squamas of the fruits in the control group were completely dry and brittle, the color was dim and the fruit bodies were significantly corrupted with plaque and were inedible (Fig. (Fig.1c).1c). In the trypsin group, the squamas were partly dry; however, the peel was bright and clean, and the flesh was edible (Fig. (Fig.11d).

Effect of trypsin treatment on the storage quality or ROS levels of H. undatus. Values are the mean±
SE of triplicate samples. Symbols “**” represent p
<
0.01. a-d, Fruits of H. undatus stored at 25
°C for 159
h with and without trypsin treatment. a and c, Control fruits; b and d, trypsin-treated fruits; 0
h or 159
h represent that fruits were stored for 0 or 159
h, respectively. e, Weight loss of H. undatus fruit stored with or without trypsin for 9
days; f, MDA contents of H. undatus fruit stored with or without trypsin for 7
days; g or h, Superoxide anion production rates, or H2O2 concentrations in H. undatus peel with or without trypsin for 7
days
The weight loss rate of each group showed a significant upward trend with increasing storage time (Fig. (Fig.1e).1e). The fruit in the control group exhibited a relatively large mean decrease in fresh weight of 1.15% weight loss per day, while the trypsin group showed only a 0.81% (trypsin) weight loss per day (Fig. 1e). There was a significant difference between the trypsin and control groups (p<
0.01).
Impact on the level of cell injury
To further investigate the preservation mechanism of trypsin, the difference of injury on cells between control and trypsin group was evaluated in H. undatus. Figure 1f showed that the membrane lipid peroxidation in the control group sharply increased by fourfold after 7days of storage. The increase of malondialdehyde (MDA) was fully impeded by trypsin. There was a highly significant difference between the two groups by the end of the storage period (Day 7) (p
<
0.01).
Impact on the ROS metabolisms of H. undatus
Excess ROS was the major source of cell injury during storage of fruit. Results showed that the levels of O2− and H2O2 in the fruits of the control group increased with storage (Fig. (Fig.11 g and h), exhibiting similar trends as did the MDA content. Trypsin entirely inhibited the accumulation of ROS, especially O2− (Fig. (Fig.1g1g and h).
Transcriptomic analysis
Sequencing and de novo assembly of Transcriptome
To further reveal the hub genes and key pathway of ROS regulation by trypsin, transcriptomic data was analyzed in H. undatus. The two libraries of the control and trypsin groups produced 50,236,685 and 44,897,144 raw reads, respectively (Table 1). The length of a single read was 150bp. From control and trypsin group libraries, 48,702,393 and 43,456,887 high quality reads were obtained, respectively. Q20 values were 98.31 and 98.33%, respectively. Q30 values were 94.80 and 94.90%, respectively. Also, 224,395 transcripts were composed by high-quality reads using Trinity software. The average length was 1086
bp. N50 value was 1982. The length of transcripts ranged from 201 to 15,462
bp. BUSCO score was 64.8%. Figure S1 showed the length distribution of these transcripts. In fact, 78.63 and 72.92% of transcripts have been mapped, respectively.
Table 1
Summary of the sequencing data of H. undatus
Sample | Raw reads | Raw bases | Clean reads | Clean bases | Error rate (%) | Q20 (%) | Q30 (%) | GC content (%) | Mapped ratio (%) |
---|---|---|---|---|---|---|---|---|---|
Control | 50,236,685 | 7,535,502,700 | 48,702,393 | 6,604,345,574 | 0.013 | 98.31 | 94.80 | 46.87 | 78.63 |
Trypsin | 44,897,144 | 6,734,571,600 | 43,456,887 | 5,841,023,572 | 0.012 | 98.33 | 94.90 | 45.19 | 72.92 |
Functional annotation and analyses
Since there was still no reference genome for H. undatus, a total of 131,559 transcripts and 86,808 unigenes were blasted against six public databases (Swiss-Prot, NR, COG, Pfam, GO and KEGG) (E value <1e-4). 67,506 (51.31% of all) transcripts and 31,756 (36.58% of all) unigenes were annotated using these databases (Fig. S2, Table S1 and S2). Based on COG and NOG classifications, 3191 or 2755 unigenes and 8288 or 6677 transcripts were assigned into 24 functional groups, respectively (Fig. S3 and Table S3). The number of transcripts was much higher than that of unigenes. Each unigene was spliced by one or more transcripts. All of these unigenes belonging to three different categories, including biological process (BP), molecular function (MF), or cellular component (CC), have been classified (Fig. S4a).
The main functions were gathered in “binding” and “catalytic activity” on level 2 of molecular function classification (Fig. S4b). In the category of biological processes, they were focused on “organic substance metabolic process” (7758 unigenes, 15.98%), “primary metabolic process” (5442 unigenes, 15.12%) and “cellular metabolic process” (5247 unigenes, 14.54%) (Fig. S4c). In the cellular component, cell part (13.01%) and membrane part (12.35%) were the major parts (Fig. S4d). 7943 unigenes were assigned to 20s categories belonging to 6 first categories of KEGG pathways (E-value: 1e-4; Identity: 0; Similarity: 0) (Fig. S5 and Table S4).
Analysis of differentially expressed genes with trypsin treatment
A total of 31,756 unigenes were identified and quantified from 86,808 genes (Table S1). The expression levels of these genes have been concluded by using a volcano plot (Fig. 2a and Table S5). The total number of different unigenes identified was 1703, including 934 upregulated unigenes (red points) and 769 downregulated unigenes (green points), (p<
0.05, fold change (FC)
>
1) in the control and treatment groups (Table S5). On the other hand, a total of 1117 ROS related genes (orange circle) were determined, including 434 upregulated unigenes (yellow circle) and 465 downregulated unigenes (purple circle). Among the 1117 ROS related genes, 85 genes (green circle) involved in 1703 genes (blue circle) were expressed to a significantly different extent (Fig. (Fig.2b2b and Table S6).
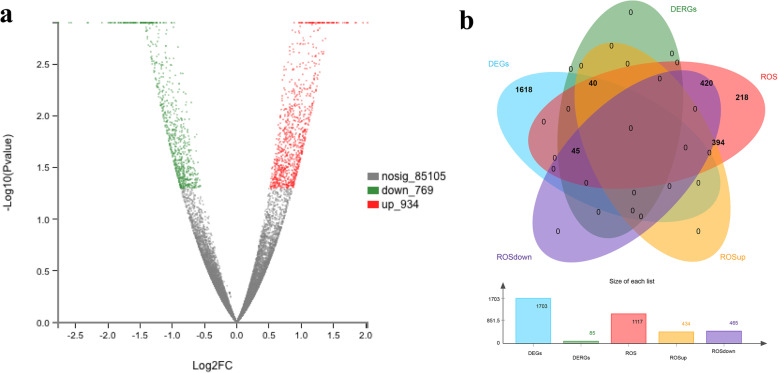
Analysis of differentially expressed genes with trypsin. a Volcano plot of significant differences in gene expression between control and trypsin groups; b Venn diagram representation of all 1703 differentially expressed genes (DEGs), upregulated genes, downregulated genes, and all of the 1117 ROS related genes (ROSup, ROSdown, and ROS), and 85 DERGs identified in the trypsin treatment group
GO enrichment analyses
The biological functions of the patterns up- or downregulated by trypsin treatment were analyzed by gene ontology (GO)-based enrichment. The top 10 GO terms in the two expression patterns were shown in Table 2 (FDR <10−
6). The upregulated enriched GO terms were “oxidoreductase activity, acting on single donors with incorporation of molecular oxygen (GO: 0016701)” and “oxidoreductase activity (GO:0016491).” On the other hand, besides more oxidation/reduction GO terms, “hydrogen peroxide catabolic process (GO: 0042744),” “peroxidase activity (GO: 0004601),” “oxidoreductase activity, acting on NAD(P) H, oxygen as acceptor (GO: 0050664),” and so on, antioxidant or catabolic GO terms, including “peroxidase activity,” “antioxidant activity” or “catalytic activity” were presented in the downregulated pattern (Table S7).
Table 2
Top 10 GO terms related to ROS enriched (FDR<
10−6) by trypsin
Pattern | Number | GO ID | Term Type | Description | p-value* | FDRa |
---|---|---|---|---|---|---|
Upregulation | 8 | GO:0016701 | MF | oxidoreductase activity, acting on single donors with incorporation of molecular oxygen | 2.01E-12 | 1.11E-08 |
25 | GO:0016491 | MF | oxidoreductase activity | 3.79E-11 | 1.05E-07 | |
Downregulation | 6 | GO:0042744 | BP | hydrogen peroxide catabolic process | 7.85E-10 | 6.07E-07 |
6 | GO:0042743 | BP | hydrogen peroxide metabolic process | 8.80E-10 | 6.07E-07 | |
11 | GO:0004601 | MF | peroxidase activity | 4.05E-12 | 1.77E-08 | |
11 | GO:0016684 | MF | oxidoreductase activity, acting on peroxide as acceptor | 6.40E-12 | 1.77E-08 | |
11 | GO:0016209 | MF | antioxidant activity | 2.75E-11 | 5.07E-08 | |
5 | GO:0050664 | MF | oxidoreductase activity, acting on NAD(P) H, oxygen as acceptor | 5.88E-11 | 8.06E-08 | |
28 | GO:0016491 | MF | oxidoreductase activity | 7.30E-11 | 8.06E-08 | |
31 | GO:0003824 | MF | catalytic activity | 1.80E-10 | 1.65E-07 |
*p-values were calculated using Fisher’s test
aFDR corrections were calculated using the Benjamini-Hochberg procedure
The major ROS related pathways involved in trypsin regulation can be summarized into a schematic representation (Fig. 3). GO terms are related to one another in a directed acyclic graph (DAG), where more detailed terms are described as children of more general terms. For example, the GO biological process “hydrogen peroxide catabolic process (GO:0042744)” is a child of four terms: “single-organism cellular process (GO:0044763),” “hydrogen peroxide metabolic process (GO:0042743),” “cellular catabolic process (GO:0044248),” and “single-organism catabolic process (GO:0044712).” The GO biological process “oxylipin biosynthetic process (GO:0031408)” is a child of two terms: “oxylipin metabolic process (GO:0031407)” and “fatty acid biosynthetic process (GO:0006633).” These in turn have parent terms, as shown in Figure 3, tracing back to the ultimate ancestor, biological process (GO:0008150), the root of the molecular function ontology. In addition, H2O2 catabolic metabolism was only downregulated (Fig. S6). Cell redox homeostasis was shown to be an upregulated GO process (Fig. S7).

Relationships between GO terms in a Directed Acyclic Graph (DAG). Red to white colors represent decreasing significance levels (Red is the most, while white is the least significant). The figure illustrates a subset of the molecular function DAGs for the oxylipin biosynthetic process (GO:0031408), hydrogen peroxide catabolic process (GO:0042744) and response to oxidative stress (GO:0006979). Arrows indicate relationships of the is_a type. The ancestors of GO:0031408, GO:0042744 or GO:0006979 are highlighted back to the root of the biological process ontology via arrows
KEGG pathway analyses
The top 9 enriched pathways were shown in Table 3 (FDR <0.05). With trypsin, downregulated ROS related genes were enriched in several pathways, including the “phenylpropanoid biosynthesis pathway (map 00940),” which is associated with fatty acid biosynthesis, as shown in the GO analysis section, “MAPK signaling pathway - plant (map 04016),” which involves a series of defense responses induced by ROS, and “Plant-pathogen interaction (map 04626),” which highly focus on the hypersensitive response (HR) induced by ROS. On the other hand, “Linoleic acid metabolism (map 00591),” “Photosynthesis (map 00195),” “Ascorbate and aldarate metabolism (map 00053),” and “Porphyrin and chlorophyll metabolism (map 00860)” were significantly upregulated.
Table 3
Pathways related to ROS enriched (FDR<
0.05) by trypsin
Pattern | Number | KO ID | Term | p-value* | FDRa |
---|---|---|---|---|---|
Upregulation | 3 | map 00591 | Linoleic acid metabolism | 1.56E-05 | 0.00034 |
3 | map 00195 | Photosynthesis | 0.00017 | 0.0018 | |
2 | map 00053 | Ascorbate and aldarate metabolism | 0.0084 | 0.046 | |
2 | map 00860 | Porphyrin and chlorophyll metabolism | 0.0065 | 0.047 | |
Downregulation | 9 | map 00940 | Phenylpropanoid biosynthesis | 1.29E-10 | 2.06E-09 |
6 | map 04016 | MAPK signaling pathway - plant | 5.52E-06 | 4.41E-05 | |
6 | map 04626 | Plant-pathogen interaction | 1.34E-05 | 7.15E-05 | |
2 | map 00073 | Cutin, suberin and wax biosynthesis | 0.0020 | 0.0080 | |
2 | map 00592 | alpha-Linolenic acid metabolism | 0.010 | 0.033 |
*p-values were calculated using Fisher’s test
aFDR corrections were calculated using the Benjamini-Hochberg procedure
PPI networks of DERGs
In total, we obtained 85 DERGs (FDR <0.01, 40 upregulated and 45 downregulated) among 1117 ROS related genes, including 434 upregulated genes and 465 downregulated genes (Table S8).
The PPI subnetwork of total ROS genes was composed of 404 nodes and the first 2000 edges, containing 40 DERGs (Fig. 4 and Table S9). The upregulated ROS gene PPI subnetwork contained 278 nodes and the first 2000 edges, including 29 upregulated DERGs (Fig. S8a and Table S10). The downregulated antioxidant gene PPI subnetwork contained 288 nodes and the first 2000 edges (interactions), including 10 downregulated DERGs (Fig. S8b and Table S11).
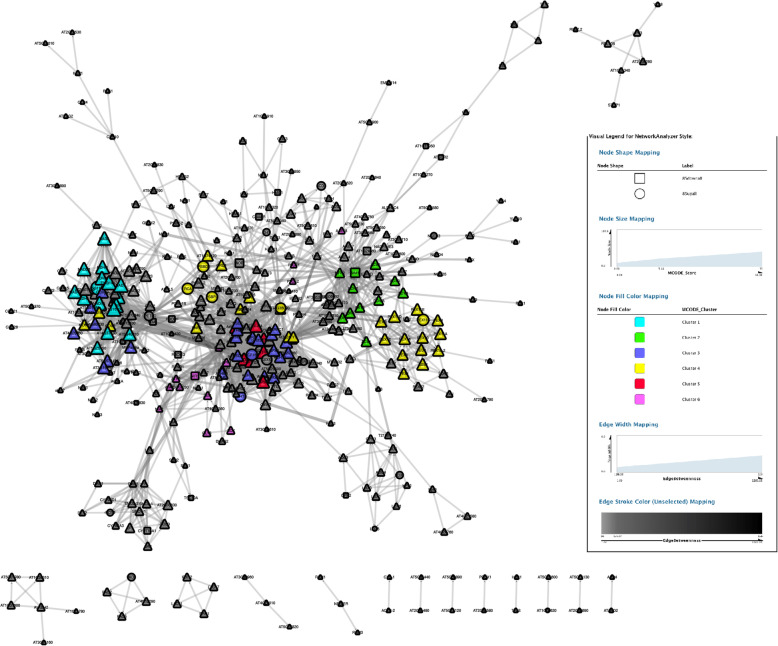
ROS related PPI networks induced by trypsin were constructed by Cytoscape software. Rectangle nodes represent proteins encoded by downregulated genes; Round nodes represent proteins encoded by upregulated genes. Triangle-shaped nodes represent the last interacting proteins without significantly different expression. The top 6 clusters calculated by MCODE were colored as shown in the legend
Further, the Cytoscape plugin “MCODE” was layered on the PPI network. Twenty clusters were obtained (Table S12). Nodes belonging to the top 6 clusters were labeled by different colors in the PPI network (Fig. (Fig.4).4). The top 6 clusters analyzed by the CytoHubba plugin of Cytoscape were then shown in Figure 5. The central nodes of each cluster were shown in Table 4.
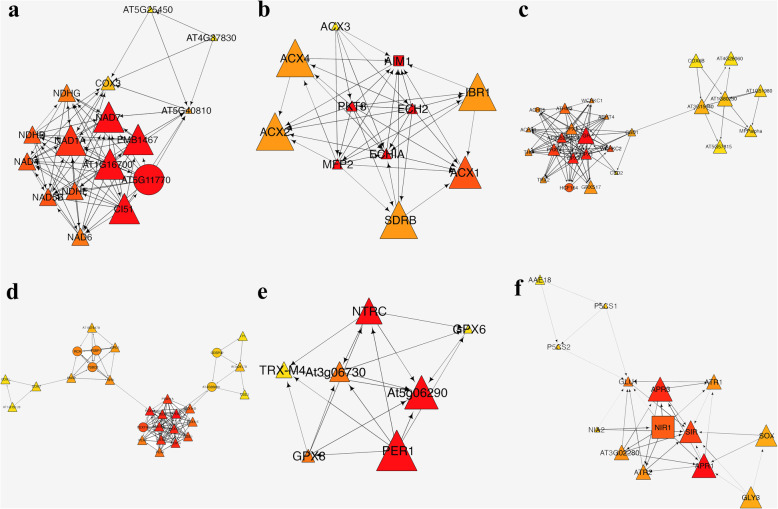
Top 6 clusters of ROS related PPI network calculated by MCODE. a-f represent clusters 1–6, respectively
Table 4
Central nodes of top 6 clusters of ROS related PPI subnetwork of H. undatus
Cluster | Central nodes | Score of clusters | Score of each node |
---|---|---|---|
1 | AT1G16700; NAD7; EMB1467; AT5G11770; CI51 | 10.533 | 322,680 |
2 | PKT3; ECHIA; MFP2; AIM1; ECH2 | 8.600 | 2280 |
3 | GR | 7.750 | 344 |
4 | PEX5 | 7.571 | 171,362 |
5 | At5g06290; PER1; NTRC | 6.000 | 72 |
6 | APR1 | 5.385 | 42 |
Furthermore, all of the DERGs were selected to construct 3 new PPI networks, including total (60 nodes, 255 edges) (Fig. 6a), upregulated (30 nodes, 81 edges) (Fig. S9a), and downregulated (28 nodes, 62 edges) DERGs subnetworks (Fig. S9b). Three clusters were constructed by MCODE as shown in Figure 6 c, d and e.
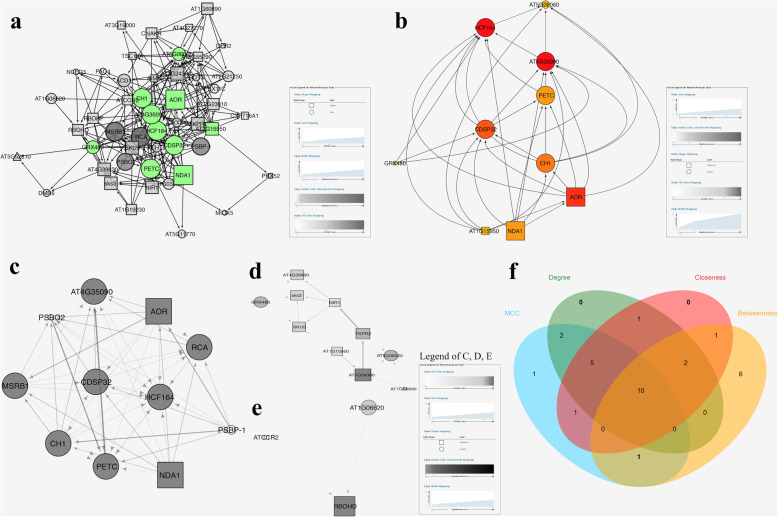
Analysis of hub genes of DERGs. The shape setting of nodes was the same as in Figure 4. a, Subnetwork of DERGs. The top 10 nodes of cytoHubba were colored; b, Hierarchical layout of 10 hubs; c-e, Clusters 1–3 of DERGs by MCODE; f, Overlapping DERGs among the four methods according to cytoHubba
Based on the calculation of CytoHubba plugin, because the screen results of either Density of Maximum Neighborhood Component (DMNC) or Maximum Neighborhood Component (MNC) did not match well to the other methods, hub genes were determined by overlapping the genes according to 4 ranked methods, including Maximal Clique Centrality (MCC), Degree, Closeness and Betweenness in cytoHubba (Table S13). Ten hub genes were discovered, including 7 upregulated and 3 downregulated genes (Fig. (Fig.66 b and f).
Topological properties of networks
The node degree distributions of the total, upregulated, and downregulated ROS related gene subnetworks followed power law fit distributions (R2=
0.798, 0.765, and 0.762, respectively) (Fig. S10). The subnetwork topological parameters, including network centralization clustering coefficient, and so on, were shown in Table 5.
Table 5
Topological parameters of the ROS related genes, DERGs and Hub genes of the PPI subnetwork of H. undatus impacted by trypsin
PPI subnetwork | y![]() ![]() | R2 | Correlation | Clustering coefficient | Network centralization | Network density | Num. of nodes | Characteristic path length |
---|---|---|---|---|---|---|---|---|
ROS related genes network | ||||||||
![]() | y![]() ![]() | 0.798 | 0.891 | 0.381 | 0.150 | 0.025 | 404 | 3.920 |
![]() | y![]() ![]() | 0.765 | 0.853 | 0.413 | 0.286 | 0.052 | 278 | 2.839 |
![]() | y![]() ![]() | 0.762 | 0.733 | 0.353 | 0.299 | 0.048 | 288 | 2.748 |
DERGs of ROS subnetwork | ||||||||
![]() | y![]() ![]() | 0.412 | 0.582 | 0.361 | 0.395 | 0.144 | 60 | 2.397 |
![]() | y![]() ![]() | 0.422 | 0.807 | 0.354 | 0.355 | 0.186 | 30 | 2.448 |
![]() | y![]() ![]() | 0.001 | −0.021 | 0.239 | 0.222 | 0.164 | 28 | 2.437 |
Hubs subnetwork | ||||||||
![]() | y![]() ![]() ![]() ![]() | 0.268 | 0.499 | 0.849 | 0.333 | 0.733 | 10 | 1.267 |
The subnetworks of DERGs, except the downregulated DERGs subnetwork (R2=
0.001), were characterized as scale-free networks, even though they were much weaker than ROS related genes networks, which approximately followed power law fit distributions, with R2
=
0.412 and 0.422, respectively (Fig. S10 and Table Table5).5). Since the DERGs were chosen from the ROS related genes network, either the centralization or the density of the total DERGs network was much higher (0.395 and 0.144, respectively) than that of the total ROS related genes network (0.150 and 0.025, respectively). Finally, the correlation of hub network was decreased to 0.499 (R2
=
0.268).
Accuracy of RNA-Seq data verification by RT-qPCR
The accuracy of the RNA-Seq data of ten hub genes of DERGs involved in ROS metabolism were verified by reverse transcription quantitative PCR (RT-qPCR) (Fig. S11). The IDs, NR description, Log2FC(E/C), p value, FDR, and primers of the 10 hub genes are shown in Table 6 and Table S16.
Table 6
Primer sequences used in RT-qPCR
Name | Gene ID | Primer |
---|---|---|
β-actin | Forward: 5′-TCTGCTGAGCGAGAAAT-3′ | |
Reward: 5′-AGCCACCACTAAGAACAAT-3′ | ||
HCF164 | TRINITY_DN39264_c0_g2 | Forward: 5′-GCGAATGATAATCCAAGCCAAGC −3′ |
Reward: 5′-TATGATACCGCCAAGGCAGACAG −3′ | ||
CDSP32 | TRINITY_DN42075_c0_g1 | Forward: 5′-TTCGCTTCTCCTTTCTCCCTTGC-3′ |
Reward: 5′-TGCTGTGGACTTTCTGGACCCTC-3′ | ||
AOR | TRINITY_DN44827_c0_g2 | Forward: 5′-GAGTACACGGCTGCTGAAGAAAG-3′ |
Reward: 5′-GGCACCACCAAGAATAAGGATAG-3′ | ||
CH1 | TRINITY_DN40044_c0_g1 | Forward: 5′-AGGTTAGAGGCAACATTGGAGTC-3′ |
Reward: 5′-ATGGAGCATCATTATGGTGAGAA-3′ | ||
PETC | TRINITY_DN41555_c0_g1 | Forward: 5′-CCCATCAACAGCGGTGGCTAAAC-3′ |
Reward: 5′-GGGACGAAGAAGGCAGCGTAAGG-3′ | ||
AT4G35090 | TRINITY_DN52071_c2_g1 | Forward: 5′-GCAGCCCTGAAACCATCCGTGAC-3′ |
Reward: 5′-CCCACATCGTCGAAGAGCCAACC-3′ | ||
NDA1 | TRINITY_DN41480_c0_g1 | Forward: 5′-GAGAGAGAGCCAATATCCTATGAAGCATCC-3′ |
Reward: 5′-GGATGCTTCATAGGATATTGGCTCTCTCTC-3′ | ||
AT5G06060 | TRINITY_DN39500_c0_g1 | Forward: 5′-GGGTCTCAGGAAAGAAACAGTAA-3′ |
Reward: 5′-GCTCCATAAATAGGACCAGCATTA-3′ | ||
AT1G15950 | TRINITY_DN6609_c0_g1 | Forward: 5′-AAAGAATGCCCATTTGAGGGAGC-3′ |
Reward: 5′-TTTGTGCCGTTTACTGCTGGTTC-3′ | ||
GRX480 | TRINITY_DN13090_c0_g1 | Forward: 5′-AACCACCTTACCAAGAGCTTCCC-3′ |
Reward: 5′-CCAATAACAAACGCCTGACAACAT-3′ |
Discussion
Trypsin treatment alone can already significantly reduce the loss of water, impede the dehydration and improve the fruit quality. MDA is considered to represent the degree of cell membrane lipid peroxidation, since it is a marker of membrane lipid peroxidation [14]. The result of MDA indicates that trypsin can significantly decrease the MDA content, which represents the lipid peroxidation of the cell membrane, thereby significantly slowing the cell damage.
In the process of maturity or decline, the disruption of balance between the generation and scavenging of ROS causes the accumulation of ROS. A high concentration of ROS such as O2− and H2O2 can cause lipid peroxidation, which is the main cause of membrane damage [15]. As expected, the novel superoxide scavenger trypsin entirely inhibited the accumulation of ROS, especially O2−. However, how can trypsin perform ROS regulation? Which genes were impacted by trypsin during storage? The transcriptomic analysis was used to reveal the hub genes regulating ROS metabolism through trypsin treatment.
Analyzed from transcriptomic profile, a total of 1117 ROS related genes were determined, including 434 upregulated unigenes and 465 downregulated unigenes. Among the 1117 ROS related genes, 85 genes were expressed to a significantly different extent.
The biological functions of the patterns up- or downregulated by trypsin treatment were analyzed by gene ontology (GO)-based enrichment. Lots of oxidation/reduction GO terms were enriched. The major ROS related pathways involved in trypsin regulation were summarized by DAGs (Fig. (Fig.3).3). The DAGs indicated that the H2O2 catabolic metabolism and oxylipin biosynthesis are key processes of trypsin regulatory mechanisms during H. undatus storage.
Next, to illustrate the pathways involved in the trypsin responsive patterns, the KEGG pathways were enriched. Important physiological metabolic processes, such as photosynthesis, porphyrin and chlorophyll metabolism, were induced, while defense responses were impeded by trypsin. In the top 2 pathways regulated by trypsin, 11 and 7 genes were involved in “Phenylpropanoid biosynthesis (map 00940)” and “MAPK signaling pathway- plant (map 04016)”. As we know, peroxidase (POD) is the key enzyme of the last process of lignin synthesis [16, 17]. In map 00940, Guaiacyl lignin, 5-hydroxy-gualacyl lignin, syringyl lignin and p-hydroxy-phenyl lignin were regulated by POD (Fig. S12). In map 04016, results showed that respiratory burst oxidase (RbohD) was regulated to maintenance the homeostacis of ROS, and stress-tolerant response was also induced which due to the significantly activated catalase (CAT) (Fig. S13). These observations were consistent with our previous results [18]. The results of KEGG indicated that, as a novel superoxide scavenger, trypsin regulated antioxidant system of pitaya and exhibited protection of pitaya during storage.
It is essential to explore the potential ROS regulatory mechanisms of trypsin by the exposition of the DERGs; the investigation on the PPI networks would promote the functional research on DERGs induced by trypsin. The PPI network of total, upregulated, or downregulated ROS genes were constructed. These three subnetworks indicated that trypsin treatment greatly disturbed the PPI network in H. undatus. The biological consequences were magnified by hundreds of proteins interacting with DERGs.
Further, the Cytoscape plugin “MCODE” and “CytoHubba” were layered on the PPI network to illustrate subnetworks and screen hub genes. Among the obtained 10 hub nodes, 7 nodes, including HCF164 (Thioredoxin-like protein), AT4G35090 (Catalase), PETC, and so on, were clustered in module 1 of the DERGs PPI network; the other 3 nodes including AT5G06060 (NAD(P)-binding Rossmann-fold superfamily protein), GRX480 (Thioredoxin superfamily protein), and AT1G15950 (Cinnamoyl-CoA reductase) were clustered in module 2 of the DERGs PPI network (Table S14 and S15). In addition, only HCF164 was clustered in the top 6 modules (cluster 3) of all ROS related PPI networks (Figs. 5 and and6).6). Most of the hubs were thioredoxin related proteins. This result indicated that the mechanisms of trypsin regulation of ROS are closely associated with sulfur metabolism.
Furthermore, the hub of AT1G15950 (Cinnamoyl-CoA reductase) suggested that the quality of H. undatus storage was associated with lignin metabolism, which was also shown in the DAG of GO analysis (Fig. (Fig.3).3). From the information of the arrow directions, either NDA1 (Internal NAD(P) H dehydrogenase in mitochondria) or AOR (NADPH-dependent alkenal/one oxidoreductase, chloroplastic) is the upstream gene of AT5G06060 (Fig. (Fig.6).6). This pathway is dependent on the redox of NAD(P) H, which is the main source of O2−. These results strongly indicated that the downregulated NAD(P) H and lignin pathways might be the key regulatory mechanisms of trypsin, the superoxide scavenger, on the quality improvement of H. undatus during storage. While, there still lots of question unclear. For example, who is the transcriptional regulation factor primarily induced by trypsin? Further works are needed to investigate the regulatory mechanisms of trypsin on lignin synthesis.
The correlation of the distribution of node degrees of a PPI network to the power law distribution is a judgment standard for scale-free networks. This property distinguishes the PPI network from random networks [19]. The node degree distributions of the total, upregulated, and downregulated ROS related gene subnetworks followed power law fit distributions. This suggested that these three PPI subnetworks were true cellular complex biological scale-free networks. These results also showed that a few protein nodes serve as hubs with links to other protein nodes [19].
As expected, the correlation of hub network was decreased with the decrease of nodes of total ROS, DERGs, and hub networks (Table (Table5).5). This indicates that the hub genes are highlighted in a larger network and that our PPI networks are reliable.
The accuracy of the RNA-Seq data of these hub genes were verified by RT-qPCR. Expression changes of these 10 genes were consistent with the RNA-Seq data. This showed that RNA-Seq data are credible.
Conclusions
Trypsin treatment significantly reduced the accumulation of ROS, including O2− and H2O2, in H. undatus during storage, impeded membrane lipid peroxidation, and prolonged the storage life of H. undatus. Transcriptomic analysis revealed 10 hub genes regulated by trypsin involved in H. undatus quality improvement during storage. PPI network analysis suggested that the downregulated NAD(P) H and lignin pathways might represent the key regulatory mechanisms of trypsin. As a new biopreservative, trypsin is highly efficient, safe and economical. Therefore, trypsin possesses technical feasibility for the quality control of fruit storage.
Methods
Main materials
H. undatus (Vietnam No. 1 cultivar, white pulp) was harvested from Ruyang county in Henan Province, China. The plant was identified by Prof. Zhaoyong Shi (College of Agriculture, Henan University of Science and Technology, Luoyang, China) and the voucher specimens (No. Hu-20,160,923) have been deposited in our laboratory. Fruits without mechanical damage and with uniform color, size and number of scales were chosen for the study. Trypsin (bovine, 500units/mg, crystalline) was purchased from Ameresco (Solon, OH, USA).
H. undatus treatment methods
Trypsin was brushed evenly for 80s onto the peels of 15 H. undatus fruits as the trypsin group. The fruits of H. undatus of the control group were treated in the same conditions with PBS buffer. The fruits were then placed in an incubator (25
°C, 85% relative humidity), and their physical and chemical indices (weight loss, MDA, etc.) were periodically measured. The optimal concentration of trypsin (2.41
×
10−
6
mol/L) was determined in our pre-experiment and used for the current study.
Library construction and Illumina RNA-sequencing
Total RNA was extracted from two groups of H. undatus peels (with or without trypsin). The peels of each group were taken from 15 fruits. Transcriptome libraries for RNA-seq were constructed from 5μg samples of RNA (≥ 100
ng/μL) using the Truseq™ RNA sample preparation kit from Illumina (San Diego, CA). Libraries were constructed and sequenced with the Illumina HiSeq xten (2
×
150
bp read length) by the Tolo Biotech company of China. All data were uploaded to the I-Sanger cloud platform and analyzed as described below.
De novo assembly and annotation
The raw paired end reads were trimmed and quality controlled by SeqPrep (https://github.com/jstjohn/SeqPrep) and Sickle (https://github.com/najoshi/sickle) with default parameters. Then clean data from the samples were used to do de novo assembly with Trinity (http://trinityrnaseq.sourceforge.net/) [20]. All the assembled transcripts were searched against the NCBI protein nonredundant (NR), String, and KEGG databases using BLASTX to identify the proteins that had the highest sequence similarity with the given transcripts to retrieve their function annotations and a typical cut-off E-values less than 1.0×
10−
5 was set. BLAST2GO (http://www.blast2go.com/b2ghome) program was used to get GO annotations of unique assembled transcripts for describing biological processes, molecular functions and cellular components. Metabolic pathway analysis was performed using the Kyoto Encyclopedia of Genes and Genomes (KEGG, http://www.genome.jp/kegg/).
Identification of differentially expressed genes
To identify differentially expressed ROS genes (DERGs) between control and samples treated with trypsin, the expression level of each transcript was calculated according to the fragments per kilobase of exon per million mapped reads (FRKM) method. Differential expression analysis was performed by EdgeR software from the R statistical package. The false discovery rate (FDR) was used to adjust the resulting P-values using the Benjamini and Hochberg approach.
GO and KEGG enrichment for differentially expressed genes
Functional-enrichment analyses including GO and KEGG were performed as reported by Candar-Cakir et al. [21]. The enriched GO terms were shown with DAGs (directed acyclic hierarchical graph) and bar charts [21].
Gene expression analysis by reverse transcription-qPCR
Total RNA was extracted as described above. Reverse Transcription-qPCR was performed as reported by Yang et al. [22]. The information of primers is listed in Table Table6.6. β-actin was used as internal control. The relative copy numbers of the genes were obtained by the 2−Ct method [10, 22].
Protein-protein interaction (PPI) analyses
PPI network generation
The ROS related proteins were screened from the I-Sanger cloud platform and imported into Cytoscape software. The relationship between ROS related proteins and their putative targets was visualized through PPI networks of ROS, DERGs, or Hubs of H. undatus induced by trypsin using Cytoscape [19].
Network topological parameters
Several network topological parameters could compare and characterize the complex networks. The primary topological parameters of networks were calculated by NetworkAnalyzer [19]. Here, the edges were considered as undirected. The equation y=
βxα and parameters R2 and correlation described the fit to the power line [23].
Module
A Molecular Complex Detection (MCODE) analysis was performed to identify the clusters in the entire ROS related network (Degree cutoff: 2; Node score cutoff: 0.2; K-Core: 2; Max depth: 100) [24]. Here, the edges were treated as directed for MCODE or next hub node analysis.
Analysis of hubs
The key genes in the PPI network were investigated topologically by NetworkAnalyzer. The cytoHubba plugin of Cytoscape further analyzed the network, and the high degree nodes were identified [25]. Through the cytoHubba plugin, 11 topological analysis methods were obtained. To increase the sensitivity and specificity, besides three centrality methods including degree, closeness, and betweenness centrality, the top 20 hub-forming proteins were also identified based on local method, including MCC, MNC and DMNC, respectively. Then, the overlapping proteins were considered as the hubs. Finally, we found the common nodes using Venn diagrams [26].
Determination of the weight loss rate
The weight loss rates for each group were determined using 3 replicates (n=
6) and were recorded at the same time every day for 9
days as the fruit was stored at 25
°C. The replicate information, including recording time point or temperature, among others, was identical to that of other index determinations, including browning index and electrical conductivity. The percentage of weight loss was calculated after days of storage.
Quantification of lipid peroxides in H. undatus peel
MDA contents were measured using the thiobarbituric acid reactive substrates (TBARS) assay as reported by Zhou et al. (2014) [27].
Determination of superoxide and hydrogen peroxide content of H. undatus peel
First, 2g of H. undatus peel was ground with 6
ml 50
mM PBS (pH
=
7.8) and 1% (w/v) PVP at 0
°C. The sample was obtained by 12,000
g centrifugation (4
°C, 15
min). The production of O2− and hydrogen peroxide (H2O2) was as described by Schneider et al. [28] or Li and Imlay in 2018 [29], respectively.
Statistical analyses
The SPSS statistical software package (11.0.1) (15 November 2001, SPSS Inc., Chicago, IL) was used for data analyses. Our results were obtained by 3 independent experiments. A paired sample t-test was used to analyze the differences between samples. Significant difference was estimated by p<
0.05. Highly significant means p
<
0.01.
Supplementary information

Acknowledgements
We are grateful for the free online Majorbio I-Sanger Cloud Platform (www.i-sanger.com). We would also like to thank English Editing by Elsevier Language Editing Services (Registration No. 331566771).
Authors’ contributions
XL and YY conceived the projects. XL and XYP designed and executed the experiments. HCY and YXY contributed expertise in the H. undatus system, XRL and BRL detected the activities of enzymes, and XYP and BRL detected the browning indices and analyzed the data. All authors discussed the results. XL and XYP cowrote the paper. All authors have read and approved the manuscript.
Funding
This work has been supported by the National Key Research and Development Program of China (2017YFC1600802). XL is supported by the State Key Laboratory of Cotton Biology Open Fund (CB2018A22), and the Natural Science Foundation of Henan Province of China (182300410083). XYP is supported by the Natural Science Program of the Educational Commission of Henan Province of China (18A310013). Publication costs for this work were funded the Science and Technique Foundation of Henan Province (182102310644).
Availability of data and materials
All data and materials used in this research are publicly available. Raw sequence data from this study have been submitted to the NCBI sequence read archive under the BioProject accession [PRJNA509494] and are available at the following link: https://trace.ncbi.nlm.nih.gov/Traces/sra_sub/sub.cgi?acc=SRP173572. Other supporting data are included as additional files listed below and are submitted with the manuscript.
Competing interests
None of the authors have potential financial or ethical conflicts of interest with the contents of this submission.
Footnotes
Publisher’s Note
Springer Nature remains neutral with regard to jurisdictional claims in published maps and institutional affiliations.
Contributor Information
Xin Li, Email: nc.ude.tsuah@nixil.
Xueru Liu, Email: nc.ude.tsuah.uts@753090813081.
Xinyue Pang, Email: nc.ude.tsuah@87yxgnap.
Yong Yin, Email: nc.ude.tsuah@gnoyniy.
Huichun Yu, Email: nc.ude.tsuah@nuhciuhuy.
Yunxia Yuan, Email: nc.ude.tsuah@nauyxy.
Bairu Li, Email: nc.ude.tsuah.uts@802021614171.
Supplementary information
Supplementary information accompanies this paper at 10.1186/s12864-020-06850-1.
References
Articles from BMC Genomics are provided here courtesy of BMC
Full text links
Read article at publisher's site: https://doi.org/10.1186/s12864-020-06850-1
Read article for free, from open access legal sources, via Unpaywall:
https://bmcgenomics.biomedcentral.com/track/pdf/10.1186/s12864-020-06850-1
Citations & impact
Impact metrics
Article citations
Transcriptomic Analysis of Liver Tissue of Black Sea Bass (Centropristis striata) Exposed to High Nitrogen Environment.
Genes (Basel), 14(7):1440, 13 Jul 2023
Cited by: 0 articles | PMID: 37510344 | PMCID: PMC10378819
Stem transcriptome screen for selection in wild and cultivated pitahaya (Selenicereus undatus): an epiphytic cactus with edible fruit.
PeerJ, 11:e14581, 06 Jan 2023
Cited by: 1 article | PMID: 36632141 | PMCID: PMC9828283
Genomic Approaches for Improvement of Tropical Fruits: Fruit Quality, Shelf Life and Nutrient Content.
Genes (Basel), 12(12):1881, 25 Nov 2021
Cited by: 10 articles | PMID: 34946829 | PMCID: PMC8701245
Review Free full text in Europe PMC
When anaerobes encounter oxygen: mechanisms of oxygen toxicity, tolerance and defence.
Nat Rev Microbiol, 19(12):774-785, 28 Jun 2021
Cited by: 63 articles | PMID: 34183820 | PMCID: PMC9191689
Review Free full text in Europe PMC
Data
Data behind the article
This data has been text mined from the article, or deposited into data resources.
BioStudies: supplemental material and supporting data
Quick GO (Showing 15 of 15)
- (4 citations) GO - GO0042744
- (3 citations) GO - GO0016491
- (2 citations) GO - GO0042743
- (2 citations) GO - GO0006979
- (1 citation) GO - GO0016701
- (1 citation) GO - GO0008150
- (1 citation) GO - GO0016209
- (1 citation) GO - GO0006633
- (1 citation) GO - GO0044248
- (1 citation) GO - GO0004601
- (1 citation) GO - GO0050664
- (1 citation) GO - GO0031407
- (1 citation) GO - GO0003824
- (1 citation) GO - GO0031408
- (1 citation) GO - GO0016684
Show less
Similar Articles
To arrive at the top five similar articles we use a word-weighted algorithm to compare words from the Title and Abstract of each citation.
Transcriptomic analysis reveals key genes related to antioxidant mechanisms of Hylocereus undatus quality improvement by trypsin during storage.
Food Funct, 10(12):8116-8128, 01 Dec 2019
Cited by: 5 articles | PMID: 31746888
Hub genes and sub-networks of stoma-related genes in Hylocereus undatus through trypsin treatment during storage revealed by transcriptomic analysis.
J Food Biochem, 45(1):e13538, 05 Nov 2020
Cited by: 1 article | PMID: 33152799
Catechin gallate acts as a key metabolite induced by trypsin in Hylocereus undatus during storage indicated by omics.
Plant Physiol Biochem, 158:497-507, 25 Nov 2020
Cited by: 2 articles | PMID: 33257230
Transcriptomic Analysis Reveals Cu/Zn SODs Acting as Hub Genes of SODs in Hylocereus undatus Induced by Trypsin during Storage.
Antioxidants (Basel), 9(2):E162, 17 Feb 2020
Cited by: 3 articles | PMID: 32079316 | PMCID: PMC7070240
Funding
Funders who supported this work.
National Key Research and Development Program of China (1)
Grant ID: 2017YFC1600802
Natural Science Foundation of Henan Province (1)
Grant ID: 182300410083
Natural Science Program of the Educational Commission of Henan Province of China (1)
Grant ID: 18A310013
Science and Technique Foundation of Henan Province (1)
Grant ID: 182102310644
State Key Laboratory of Cotton Biology Open Fund (1)
Grant ID: CB2018A22