Abstract
Purpose
Here we aim to review the association between mammographic density, collagen structure and breast cancer risk.Findings
While mammographic density is a strong predictor of breast cancer risk in populations, studies by Boyd show that mammographic density does not predict breast cancer risk in individuals. Mammographic density is affected by age, parity, menopausal status, race/ethnicity, and body mass index (BMI).New studies normalize mammographic density to BMI may provide a more accurate way to compare mammographic density in women of diverse race and ethnicity. Preclinical and tissue-based studies have investigated the role collagen composition and structure in predicting breast cancer risk. There is emerging evidence that collagen structure may activate signaling pathways associated with aggressive breast cancer biology.Summary
Measurement of film mammographic density does not adequately capture the complex signaling that occurs in women with at-risk collagen. New ways to measure at-risk collagen potentially can provide a more accurate view of risk.Free full text

Mammographic density: intersection of advocacy, science, and clinical practice
Abstract
Purpose:
Here we aim to review the association between mammographic density, collagen structure and breast cancer risk.
Findings:
While mammographic density is a strong predictor of breast cancer risk in populations, studies by Boyd show that mammographic density does not predict breast cancer risk in individuals. Mammographic density is affected by age, parity, menopausal status, race/ethnicity, and body mass index (BMI).
New studies normalize mammographic density to BMI may provide a more accurate way to compare mammographic density in women of diverse race and ethnicity. Preclinical and tissue-based studies have investigated the role collagen composition and structure in predicting breast cancer risk. There is emerging evidence that collagen structure may activate signaling pathways associated with aggressive breast cancer biology.
Summary:
Measurement of film mammographic density does not adequately capture the complex signaling that occurs in women with at-risk collagen. New ways to measure at-risk collagen potentially can provide a more accurate view of risk.
Introduction
Population-based studies consistently show that high mammographic breast density is associated with a 2 to 6-fold increased risk of breast cancer [1]. The biological basis, however, for why high density is associated with increased breast cancer-risk is not well understood. Studies by Norman Boyd’s team of postmenopausal women in the NSABP Study of Tamoxifen and Raloxifene (STAR) showed that high breast density was significantly associated with increased breast cancer risk [2].
Mammographic density, while relatively easy to measure, is the sum of complex variables that change during a woman’s lifetime. Low mammographic density is associated with increasing age and body mass index (BMI); high mammographic density is associated with young age, low BMI, and with hormone use; mammographic density also varies between racial and ethnic groups. For example, an athletic 20-year-old woman with a BMI of 20 may have high mammographic density but this does not reflect increased risk, but rather that the woman is young and has a low BMI. Conversely, mammographic density in an obese woman may be low, but the actual breast collagen structure may be associated with high- rather than low-risk for breast cancer.
High mammographic density on film-based mammographic screening has been consistently associated with the risk of “missed” or interval breast cancer. While, high mammographic density can mask the presence of a breast cancer. Alternatively, the majority of biologically aggressive triple-negative breast cancers (TNBC) are not associated with mammographic calcifications; in the absence of a breast mass, TNBC are not easily detected by mammogram, regardless of low or high mammographic density.
Mammographic density has been the subject of intense lobbying and advocacy. A majority of states have passed laws (or in the process of passing laws), requiring physicians to tell a woman whether she has mammographically dense tissue [3]. These laws state that women should be informed that dense breast tissue can 1) hide tumors on a mammogram, 2) increase the risk of breast cancer, and 3) require additional screening tests, such as ultrasound and breast Magnetic Resonance Imaging (MRI) [4, 3].
These new laws are not without controversy. Some professional groups argue that the measurement of mammographic density is subjective and not highly reproducible in a community setting [4]. To further complicate issues, there are no standardize methods to standardize mammographic density measurements for variables that affect density including age, BMI, race, ethnicity, and breast size [1]. While breast MRI can be recommended, the American Cancer Society Breast MRI guidelines do not recommend breast MRI screening for women with high breast density in the absence of established clinical risk factors (> 20% lifetime risk) [5].
Here our goal is to provide a review of the published literature on mammographic density and breast cancer risk, the biological basis of mammographic density, and review tissue-based and preclinical studies linking collagen structure with activation of signaling events that promote aggressive breast cancer. biology
Measurement of breast density.
Breast density reflects breast composition, particularly, the relative ratio of fibroglandular to adipose (fat) tissue (Fig. 1 A,,B).B). Fibroglandular tissue includes the 1) ducts and glands of the breast and 2) connective tissue between ducts and glands (Fig. 1 A,,B).B). Adipose tissue appears dark on a mammogram and is considered “low” density. Areas of brightness on mammogram are considered to be “dense” and have high fibroglandular tissue content.
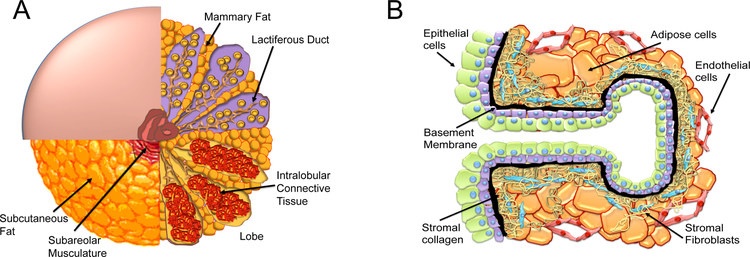
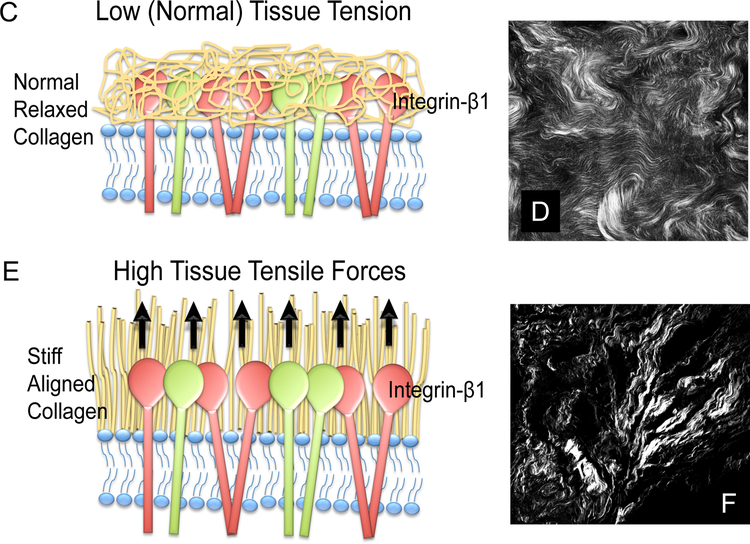
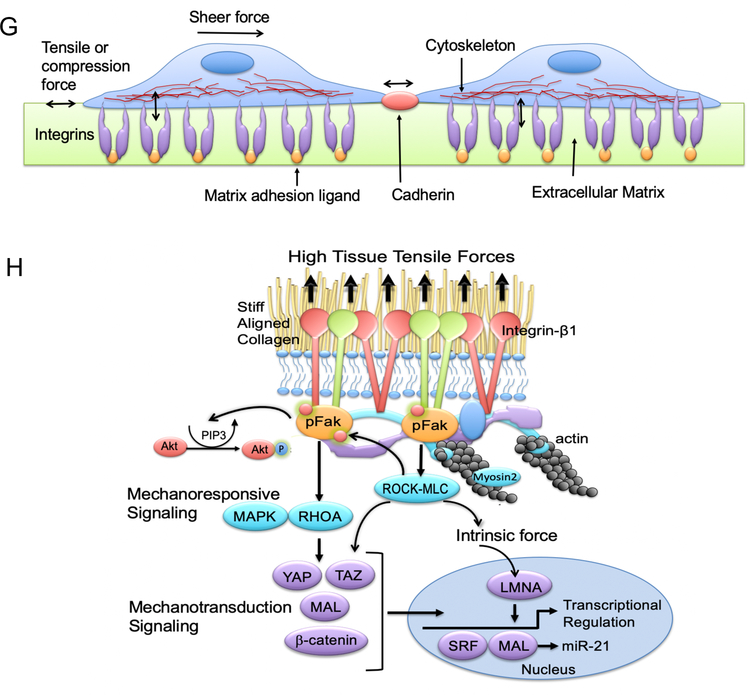
A,B The human breast composition is a complex structure. A. Progressive quadrant dissection (counter clockwise) of the human breast reveals subcutaneous fat, breast lobes containing intralobular connective tissue, and the lactiferous ducts and mammary fat. B. The terminal ductal lobular unit of the human breast contains epithelial cells, basement membrane, stromal fibroblasts, adipose cells, endothelial cells, and immune cells. Each cell type contributes to the breast density of an individual woman.
C-F Recent research provides evidence that collagen structure may play a key role in breast cancer survival and perhaps breast cancer risk. C,D. Normal collagen is curly. E,F. High risk collagen is aligned, stiff, and exerts tension (high tissue tensile forces) on adjacent epithelial cells. Studies by Patricia Keely, Ph.D. and her collaborators provide evidence that aligned collagen (tumor associated collagen E,F. Second harmonic generation imaging of collagen structure in human breast biopsies containing in invasive breast cancer. Image in D depicts curly normal collagen. Image in F depicts high-risk aligned TASK-3 collagen.
G. Tensile or compression forces on epithelial cells, cell-cell junctions, and basement membrane. H. High tissue tensile forces result in mechanoresponsive signaling, mechanotransduction signaling, and alterations in intrinsic forces.
In 1976, Wolfe first demonstrate the association between mammographic density and breast cancer risk [6]. Breast density can be measured by a number of techniques including film mammogram, digital mammography, and MRI. However, most studies predicting breast cancer risk have utilized mammography [7]. Density is typical reported using 1) the original Wolfe grading system, 2) percentage of mammographic density tissue, and/or 3) BIRADS assessment of density. Percentage density that is determined subjectively by a radiologist is shown the strongest predictor of breast cancer-risk [8]. The most widely used density reporting method in the United States today is the BIRADS classification: 1) BIRADS-1; predominately fatty, 2) BIRADS-2; scattered density, 3) BIRADS 3; heterogeneous density and 4) BIRADS 4; extremely dense.
For women with BIRADS 4 mammographic density (extreme density) the sensitivity and specificity of mammography is 63 and 89% respectively. This compares to 87 and 97% sensitivity and specificity for women with BIRADS1 (predominately fatty) breasts [9]. While MRI can be used to screen for breast cancer in women with high breast density, American Cancer Society finds insufficient evidence for (or against) the use of MRI in women with high breast density alone (and no additional risk factors [5].
Breast composition and biological determinants of mammographic density.
Mammographic density is determined by the differential ability of x-rays to penetrate human breast tissue. While breasts can feel dense or not density on clinical examination, there is no direct correlation between breast density as assessed by clinical breast exam and mammographic breast density [1].
The human breast is composed of epithelial cells, connective tissue/fibroblasts, adipose cells, endothelial cells, and immune cells (Fig. 1 A,,B).B). X-rays readily penetrate adipose tissue; a high volume of adipose tissue correlates with low mammographic density; adipose tissue appears “black” on mammography (low density) [1]. In contrast, epithelial and stromal tissues, are radio-opaque compared to adipose tissue, and appear “white” on a mammogram (high density) [1].
While the majority of breast cancers are epithelial in origin, the relative volume of breast collagen to adipose cell is the major predictor of mammographic density. Recent studies show that during breast cancer initiation there is a desmoplastic reaction consisting of 1) activation and recruitment of stromal cells, and 2) collagen deposition. Regions of the breast undergoing desmoplastic reaction demonstrate increased mammographic density.
Modifiers of mammographic density.
Mammographic density decreases with increasing age and increasing BMI. High mammographic density is associated with hormone use, low BMI, and young age [1]. The majority of White women less than 25 years old have high mammographic density (BIRADS 3,4) [10]. For women less than 40; 81% have high mammographic density (BIRADS 3,4) [10]. As women age, there is a decrease in mammographic density but an increase in breast cancer risk and incidence [10], underscoring the importance of correcting for both age and BMI in breast cancer risk assessment studies [10].
Combination hormone replacement therapy (estrogen and progesterone) is consistently associated with an increase in mammographic density [11]. High mammographic breast cancer density predicts breast cancer risk in women with a first-degree relative who has had breast cancer [12]. in BRCA1 or BRCA2 mutation-carriers, however, the association between high mammographic density and breast cancer risk risk was less strong [12].
Population-based studies of mammographic density and increased breast cancer risk.
Over 40 population-based studies demonstrate an 2 to 6-fold increased risk of breast cancer in women high mammographic density (Table 1) [15]. Originally, this association was thought to be due to the difficulty of detecting breast cancer in mammograms with extremely or heterogeneously density, termed “masking bias” [13]. Subsequent studies shown that masking bias is not the only factor that accounts for the higher breast cancer incidence observed in mammographically dense breast tissue [14, 1]..
Table 1.
Mammographic Density, Breast Cancer Risk, Modulation of Density and Risk
Study Author | Sample / Trial Size | Age of Subjects (years) | Study: Follow up Trial: Duration (years) | MD Classification | Study: Risk associated with MD^ Trial: Variable# and effect on MD and risk^ |
---|---|---|---|---|---|
Byrne [88] | 1,880:2,125 | 35–74 | 10 | Percent | OR for >75% versus 0%. PrM and PoM. 4.5 (3.2–6.4) |
Vacek [89] | 61,844 | <40 - >75 | 3.1 | BIRADS | RR for extremely dense versus entirely fatty PrM: 4.6 (1.7 – 12.6) and PoM: 3.9 (2.6 – 5.8) |
Ziv [24] | 44,811 | Mean: 54 | 3.8 | BIRADS | HR for BIRADS 4: 2.09 (1.6–2.75) |
Boyd [1] | 1,114 | 40–70 | 8 | Percent | OR for <10% versus >75% 4.7 (3.0 – 7.4) |
Eng-Wong [90] | 27 | Mean: 43 | 1 – 3 | Percent | Raloxifene: No change in MD. Risk not evaluated. |
Conroy [32] | 722 | Median: 46 | Mean: 5.6 | Percent | Physical Activity: Annual decline of 1.1% in density (decrease in non-dense breast area and no change in dense breast area). Risk not evaluated. |
Woolcott [33] | 320 | 50 – 74 | 1 | Percent and Volume | Aerobic exercise: No change in percent dense area (following adjustment changes in % body fat). Risk not evaluated. |
Martin [34] | 4,690 | 30 – 65 | Mean: 10 | Percent (all patients in trial had ≥ 50% MD) | Reduced fat diet: No effect on risk |
Cuzick [39] | 123:942 | <45 - >60 | 1 – 2 | Percent | Tamoxifen: 46% of patients had a ≥ 10% reduction in MD. Those patients had a 63% reduction in risk. |
MD, mammographic density; OR, odds ratio; RR, relative risk; HR, hazard ratio; PrM, premenopausal, PoM, postmenopausal.
In additional population-based studies, the association between mammographic density and breast cancer risk appears to hold across ethnic groups. However, the relationship appears to be weaker in Asian women versus non-Hispanic Whites or Black/African American women [15]. Asian women have relatively higher mammographic density thannon-Hispanic Whites or Black/African American women [15]. However, when adjustments are made for age and BMI, absolute breast density in Asian women is significantly lower than the age/BMI corrected mammographic density of non-Hispanic Whites and Black/African American women [16, 17]. In one study, mammographic density associated risk was greatest in patients with BMI >30 [17]. These observations underscore the importance of correcting for both age and BMI when assessing breast density [15].
High-mammographic density, masking, and biology.
It is well established that breast cancer screening is complicated by the presence of increased mammographic density. Using film mammography from the 1970’s, Whitehead et al., first demonstrated that high mammographic density had a “masking” effect [18, 6]. This “masking” effect was hypothesized to obscure the early detection; dense mammograms are more radio-opaque (“white”); many cancers also are radio-opaque and appear “white: on mammograms; therefore, the greater the mammographic density (increased “whiteness”), the more difficult it would be to detect a “white” cancer lesion.
Interval cancers are cancers diagnosed within 12 months of a normal screening radiological study. In a landmark study, Boyd et al., investigated the relationship between mammographic density and the diagnosis of an interval cancer [14]. This study was carried out three nested case–control studies in screened populations with 1112 matched case– control pairs. The association between measured percentage of density in the baseline mammogram and risk of breast cancer was investigated versus 1) method of cancer detection, 2) time since the initiation of screening, and 3) age. As compared with women with low mammographic density (<10%), women with high-mammographic density (>75%) had an increased risk of breast cancer (odds ratio, 4.7; 95% confidence interval [CI], 3.0 to 7.4), For women <56 years of age, 26% of all breast cancers and 50% of cancers detected <12 months after a negative screening mammogram test were attributable to density in 50% or more of the mammogram. This study provides evidence that high mammographic density is strongly associated with the risk for subsequent diagnosis of an interval cancer.
While studies by Boyd et al. demonstrated in women <56 years of age an association between high mammographic density and interval cancers, the study did not consider the ability of mammography to accurately diagnose specific breast cancer subtypes, particularly triple-negative breast cancer (TNBC). TNBC breast cancers are biologically aggressive and present more frequently as interval breast cancers than do other breast cancer subtypes. There is emerging evidence that in the absence of a palpable breast mass, mammograms are less readily able to diagnose TNBC than other breast cancer subtypes. Yang et al. investigated the relationship between mammographic features and breast cancer subtypes [19]. In this study, investigators observed that TNBC were less frequently associated with microcalcifications (5/33 [15%]) than were HER2-overexpressing breast cancers (HER2+) (43/64 [67%]) and ER+ breast cancers (53/87 [61%]) (p < 0.0001) [19]. In contrast, TNBC were more frequently associated with a mass (33/33 [100%]) than were HER2+ (35/64 [55%]) and ER+ cancers (42/87 [48%]) (p< 0.0001) [19]. This study provides evidence that additional imaging studies, beyond mammography, may be required for effective screening and early detection of TNBC. Findings also suggest that the potential “masking” effect of breast density may be lessened due to the low frequency of microcalicficaitons observed in TNBC.
Studies in individuals:
Studies by Norman Boyd’s team of 13,409 postmenopausal participants in the NSABP Study of Tamoxifen and Raloxifene in post-menopausal women showed that high BI-RADS breast density was significantly associated with increased breast cancer risk when considered in conjunction with Gail score but provided only slight improvement to the Gail score for predicting the incidence of invasive breast cancer [2]. This study calculated the receiver operator curve (ROC) and area under the curve (AUC) for the ability of mammographic density to predict breast cancer risk. The ROC curve is a plot of the test true-positive rate (y-axis) against the corresponding false-positive rate (x-axis); i.e., sensitivity against 1-specificity. The curve is built from test performance at different “diagnostic thresholds.” While mammographic density can be high/not-high, mammographic density has range of normal values. If the diagnosis of breast cancer increasingly likely with higher values, the proportion of patients ultimately diagnosed depends on applying a diagnostic threshold that denotes a positive test. In such analysis, an AUC of 0.5 (0.5 slope = line of identify) means that a positive test result will have an equal chance of being predictive vs. not predictive (true-positive vs. false-positive). In the studies by Boyd’s group, while mammographic density was positively associated with breast cancer risk, the AUC was 0.55, only slightly above the line of identity (AUC=0.5). Gail score had an AUC=0.63 and Gail Score + Density had an AUC=0.64. These studies highlight the weak predictive power mammographic density has in predicting breast cancer risk in post-menopausal women.
Breast cancer density and breast cancer subtypes.
High breast density is positively associated with nulliparity [1, 20], absence of breast feeding among parous women [10], and with increasing exposure to hormone replacement therapy [10, 11]. Given the association between high breast density and hormonally modulated factors, it would be logical for the tumors developed in women with high breast density to be estrogen receptor- and progesterone receptor-positive (ER+/PR+). However, findings in the existing literature are somewhat mixed. With regards to breast cancer subtype, a number of studies summarized in a meta-analysis by Antoni, et al. suggested an association between estrogen receptor positive (ER+) breast cancers and high breast density [21]. A study by Bertrand, et al. suggested that the effect of high breast density on breast cancer risk defined by subtype might be modified by age, with a stronger association between high breast density (PMD>51%) and ER- tumors among women <55 years (ORHBD=2.84, 95% CI [1.83, 4.40]) versus ER+ tumors among women <55 years (ORHBD=1.96, 95% CI[1.56, 2.45]) and no association indicated for women 55 and older [22]. Others found either no difference in the association between high breast density and breast cancer subtype and receptor status [23–25]. Therefore, there seems to be no consistency in the association between breast density and breast cancer subtype. Once again however, the variability in the breast density measurements and categorizations, the varied study designs, populations and covariate adjustments make it difficult to truly compare across studies and draw conclusions on whether breast density may be differentially association with breast cancer subtypes.
High mammographic breast density and breast cancer survival.
The association between high breast density and breast cancer survival remains a debated issue. Gierach et al., prospectively investigated the potential relationship between breast density and breast cancer death in the Breast Cancer Surveillance Consortium cohorts; there was no observed increased risk of death in women with high breast density; conversely, there was an increased risk of death among obese women with very low breast density (HR 2.02, 95%CI 1.4, 3.0) [26]. Zhang, et al. showed no association between breast density and breast cancer-related death, even after adjusting for the effects of age, race, and cancer stage [27]. Eriksson, et al. concluded that breast density was not associated with survival [28]. However, Maskarinec, et al. reported an adverse effect of high breast density on breast cancer survival among patients who did not receive radiation treatment (HR = 1.46; 95% CI: 1.00–2.14; p = 0.05), while those that received radiation had better survival (HR = 0.77; 95%CI: 0.60–0.99; p = 0.04) [29]. Olsson, et al. also found increased risk of breast cancer death among women with high breast density compared to women with fatty breasts, robust to adjustments (HR 2.56, 95%CI 1.07, 6.11), with a significant trend over increasing density categories and modified by mode of detection, with a less pronounced effect for asymptomatic (HR 2.04, 95%CI 0.49, 8.49) compared to symptomatic women (HR 3.40, 95%CI 1.06, 10.90) [30]. Finally, a recent (2015) study by Masarwah, et al. found overall worse breast cancer survival among patients with low (<25%) and very low (<10%) breast density, compared to those with higher breast density (75.3% v. 90.2, p=0.003), even after adjusting for possible confounders (HRvery low BD 3.3, 95%CI 1.8, 6.1) [31]. These studies, summarized in Table 2, highlight the lack of consensus with regard to the association between high breast density and breast cancer mortality.
Table 2.
Published Literature on the Association of Breast Density and Breast Cancer Survival
Author | Year | Country | Study Design | Outcome | Sample Size | Years Follow-up | HBD associated w/ worse survival? (Y/N) |
---|---|---|---|---|---|---|---|
Gierach [26] | 2012 | US | Prospective Cohort | BC death & all cause death | 9232 | 6.6 mean | N |
Zhang [27] | 2013 | US | Prospective Cohort | BC death & all cause death | 22,597 | 13 complete f/u | N |
Eriksson [28] | 2013 | Sweden | Case only (post-menopausal) | BC death (5 & 10 yr survival) | 1774 | 10 complete f/u | N |
Maskarinec [29] | 2013 | US | Nested Case/Control | BC death | 607 cases/980 controls | 12.9 mean | Y (for women who received radiation) |
Olsson [30] | 2014 | Sweden | Prospective Cohort | BC death | 619 | 7.8 median | Y |
Masarwah [31] | 2015 | Finland | Case only | BC death | 270 | 6.4 mean | N |
Clinical Guidelines for MRI Screening in women with high breast density.
High mammographic density is associated with increased breast cancer risk however, the biological mechanism is not well understood. Currently, outside of a research setting, we lack the ability to account for many of the confounding factors that modify mammographic density such as age, menopausal status, hormone use, BMI, and parity. Clinical guidelines suggest that breast MRI should be added to breast cancer screening in women with greater than 20% lifetime risk of breast cancer [5]. However, the current guidelines do not support the use of breast MRI in women with less than 20% lifetime risk of breast cancer [5]. Women with high mammographic density should be encouraged to enroll in clinical trials.
Can density be modified?
Mammographic density is inversely related to age [1]. This age-related decrease in mammographic density with reflects a simultaneous decrease in glandular breast tissue and an increase in adipose tissue. However, mammographic density declines with age and breast cancer risk increases with age. This paradox has led some to hypothesize that a cumulative exposure to high breast density may be the driving factor for increased [1]. Consistent with this hypothesis is that the risk of breast cancer associated with high mammographic density is stronger for women over 50 than those less than 50 years old [32].
It is well established that physical activity reduces breast cancer risk independent of impact on BMI. This BMI-independent reduction in risk is thought to mediated by a reduction in circulating breast proliferative hormones. While the impact of exercise on breast cancer risk is well established, the impact of physical activity on mammographic density is less well established. A recent trial evaluating 722 multi-ethnic women with a mean age of 46 years found no association between physical activity and reduction in mammographic density [32]. A second trial evaluating the effect of exercise on mammographic density in postmenopausal women found that weight loss was associated with an increase in mammographic density, however the total volume of breast density did not change significantly [33, 34]. In contrast, a Spanish study including over 3,500 women, has shown that weight gain in adulthood has a significant association with high mammographic density [35]. These observations further raise the question of whether weight loss, might be a means of modifying mammographic density.
The relationship between mammographic density and hormones is well established. Mammographic density declines after menopause supporting the role of female hormones promoting mammographic density. Numerous studies shown that combined estrogen-progesterone containing hormone replacement therapy increases mammographic density. The impact of estrogen therapy alone on breast density is less clear [36]; variables including age and BMI may modify these effects. The impact of hormone replacement therapy on mammographic density is weakest in younger women and women with an elevated BMI.
Pregnancy is another modifier of mammographic density. High mammographic density is inversely associated with parity and decreases 16% with each additional birth [37]. High mammographic density is positively associated with older maternal age at first birth and inversely associated with lactation-duration.
The association between hormone exposure and pregnancy and mammographic density led to studies testing whether anti-hormone-based chemoprevention (e.g. tamoxifen) could reduce both breast cancer risk and breast density. The most consistent data are studies testing for the relationship between tamoxifen-chemoprevention, breast cancer risk, and breast density. Well powered clinical trials that include both pre- and post-menopausal women show that tamoxifen significantly reduces breast density [38, 39]. Furthermore, there appears to be a direct relationship between the reduction in mammographic density and the breast cancer risk-reduction associated with tamoxifen. In the International Breast Cancer Intervention Study; Cuzick et al, showed that 46% of women in the tamoxifen treatment arm experienced a 10% or greater reduction in breast density following a year of therapy [39]. For those women on tamoxifen with a significant reduction in breast density, there was a 63% reduction in breast cancer risk. However, women on tamoxifen with less than 10% reduction in breast density had no reduction in breast cancer risk. This study has provided support for the hypothesis that a decrease in breast density can serve as a surrogate maker for breast cancer risk reduction. While the use of mammographic density as a surrogate endpoint for chemoprevention trials has gained acceptance, the molecular mechanism underlying the reduction in breast cancer risk is not understood. This lack of underlying mechanism highlights the need for molecular studies to evaluate the effect of breast density on breast cancer initiation and risk.
Collagen structure, breast cancer risk, and mammographic density.
The biological mechanisms by which breast density may increase breast cancer risk are only just beginning to be understood. High mammographic density is associated with increased collagen deposition. Up until recently, collagen was felt to be a relatively inert structural protein that did not contribute substantially to cancer initiation and progression. Recent studies, however, provide evidence that collagen fibers may act as a conduit or “super highway” that traffic breast cancer cells within the mammary gland [40, 41]. These studies raise the question of whether mammary collagen structure (as opposed to mammographic density) may be a more accurate predictor of breast cancer risk.
Increased mammographic density is characterized by an increased in X-ray absorbance throughout the entire breast [40]. Generalized high mammographic density is distinct from the focal events that accompany breast cancer initiation and progression. During breast cancer initiation, there is a focal stromal response (desmoplastic reaction) characterized by 1) increased collagen matrix deposition and 2) stromal cell recruitment and activation [42, 43]. Increased cell density/numbers and increased collagen deposition both increase mammographic density. Mammographic imaging and ultrasound are inadequate to distinguish whether a focal increase in mammographic is due to an increase in cell number and/or an increase in collagen deposition. Since tumor cells invade in collagen-rich stroma it is important to develop molecular tools that can detect early interactions between pre-malignant and malignant breast epithelial cells and collagen matrix [44–47]. Therefore, techniques that identify and characterize features of the epithelial-stromal interaction at the single cell level are of great diagnostic potential.
Studies in mouse models first identified and classified sequential collagen alterations as potential markers of mammary carcinoma initiation and progression. Collagen alterations that were associated with carcinogenesis are called tumor-associated collagen signatures (TACS) [47, 48] (Fig. 1 C–F). During cancer initiation, mouse mammary tumors first exhibit a localized increase in the deposition of collagen near the tumor lesion (TACS-1) [49, 50]. As tumors increase in size, there is a straightening of collagen fibers aligned parallel to the tumor boundary (TACS-2) [47]. During the final stage of carcinogenesis, there is remodeling and reorientation of collagen such that multiple collagen fibers are bundled and aligned perpendicular to the tumor boundary (TACS-3) [47]. Studies by the late Patricia Keely, Matthew Conkin, and Kevin Eliceri show that TACS-3 collagen fiber alignment is significant; regions containing TACS-3 correspond to sites of focal invasion into the stroma [47, 48]. This group and others have shown that tumor cells preferentially invade along straightened, aligned collagen fibers [47, 51–53, 41].
Collagen alignment can now be detected through second harmonic generation (SHG) imaging. During imaging two photons of incident light interact with the non-centrosymmetric structure of collagen fibers such that the resulting photons are half the wavelength of the incident photons [54] (Fig. 1 D,,F).F). This nonlinear coherent process is non-fluorescent and will specifically image collagen; whereby the SHG signal can be separated from endogenous fluorescence through the use of narrow bandpass filters centered at one-half the laser wavelength [40]. Thus, SHG does not requires labeling or staining; SHG imaging can be used in lieu of stains for collagen, such as Masson’s trichrome or picrosirius red stain [40]. SHG generates contrast in an image based solely on the presence of collagen and is influenced by the properties of collagen itself such as the degree of cross linking, fiber thickness, and alignment of overlapping fibers (ordered versus disordered) [40, 55–61]. Keely, Conklin and Eliceri used SHG to test for TACS-3 in breast cancer biopsy samples [40]. Their studies show that TACS-3 collagen is an independent predictor of poor survival in non-Hispanic White women with breast cancer and provide evidence that collagen structure is associated with breast cancer initiation and poor survival [40].
Syndecan-1 interacts with constituents in the extracellular matrix, including collagen fibers [62]. A recent study tested whether 1) stromal syndecan-1 expression and 2) orientation of collagen fibers surrounding ductal carcinoma in situ (DCIS) predicts local DCIS recurrence [62]. The study evaluated collagen fiber alignment and syndecan-1 expression in 227 women diagnosed with DCIS in 1995 to 2006 followed through 2014 (median, 14.5 years; range, 0.7– 17.6) [62]. Stromal collagen alignment was evaluated from diagnostic tissue slides using SHG microscopy [62]. Greater fiber angles surrounding DCIS lesions, but not syndecan-1 staining intensity, were related to poor prognostic features including 1) positive HER2 (p=0.002) amplification, 2) comedo necrosis (p=0.03), and 3) ER- (p=0.002) and PR- (p=.0.02) status [62]. Although collagen alignment and stromal syndecan-1 expression did not predict recurrence, collagen fibers perpendicular to the duct perimeter were more frequent in DCIS lesions with features typical of poor prognosis [62].
Mammographic density is correlated with a relative abundance of collagen-rich fibroglandular tissue, the structural, molecular, and mechanical consequences of increased mammographic density remain poorly defined [63]. In a limited study of 22 subjects, McConnell et al. developed a bedside-to-bench workflow to determine the relationship between mammographic density, collagen abundance and alignment, tissue stiffness and the expression of extracellular matrix organizing proteins [63]. Collagen abundance was determined by both Masson’s trichrome and Picrosirius red staining; localized collagen fibril organization and stiffness was evaluated in tissue sections by atomic force microscopy/spectroscopy and the abundance of key extracellular proteins was assessed using mass spectrometry [63]. This study showed that mammographic density positively correlated with the abundance of aligned periductal fibrils rather than with the abundance of amorphous collagen [63]. Compared with matched tissue resected from the breasts of low mammographic density patients, the highly birefringent tissue in mammographically dense breasts was both significantly stiffer and characterized by large (>80 μm long) fibrillar collagen bundles [63]. Subsequent proteomic analyses identified the up-regulation of periostin and collagen XVI (regulators of collagen fibril structure and architecture) as potential mediators of localized mechanical stiffness [63]. These studies provide interesting evidence that remodeling, and hence stiffening, of the existing stromal collagen microarchitecture may promotes high mammographic density within the breast [63]. In turn, this aberrant mechanical environment may trigger neoplasia-associated mechano-transduction pathways in mammary epithelial cells [63].
Experimental assessment of collagen, tensile force, and molecular-signaling.
Studies by Valerie Weaver, Ph.D. show that aligned stromal collagen increases the stiffness of the extracellular matrix (ECM), adjacent to the basement membrane of epithelial cells [64]. Increased stromal collagen stiffness increases the “pull” or “stretch” that the stroma exerts on mammary epithelial cells (increased tissue tensile forces) [64]. When the cells sense these mechanical cues, they activate integrins; the interface that mediates cell-ECM interaction. Activation of integrins promote their clustering, oligomerization and maturation into focal adhesions [65–69]. Focal adhesions are composed of mechanosensor proteins such as talin and vinculin, in addition to signal transduction proteins such as focal adhesion kinase (FAK), SRC, PI3K, adapter proteins and actin linker proteins such as filamin and alpha-actin. The later proteins physically link integrins to the cytoskeleton [70, 71]. Upon integrin oligomerization, talin and vinculin association is fostered and FAK and SRC are activated [65, 72, 73]. Downstream signaling of FAK and SRC stimulate Rho proteins, which in turn activate Rho-associated protein kinases (ROCK) that subsequently lead to cytoskeletal reinforcement and acto-myosin contractility [65]. Rho-GTPase dependent myosin contractility is mediated by phosphorylation of myosin light chain (MLC) of myosin II. The cytoskeletal response of acto-myosin contractility is the intrinsic force generated inside the cells in response to increased stiffness (exogenous force) (Fig. 1 G,,H)H) [65, 70, 64].
Simultaneously, focal adhesion formation and signaling of FAK and SRC via interaction of growth factor receptors (GFRs) and G-protein coupled receptors (GPCRs) lead to MAPK or ERC activation (Fig. 1 G,,H)H) [65, 73, 74]. ERC activation in response to stiff microenvironment leads to enhanced growth of mammary epithelial cells in vivo and in vitro and activation of proliferation signature genes [73, 75]. Rho GTPase activation in response to stiff mechanical forces is known to activate other pathways that are involved in breast cancer and aggressive biology [64]. For instance, Rho GTPases also signal to phosphoinositide 3-kinase (PI3K) which promotes cellular proliferation via Akt signaling [76]. In response to force, ERK and Jun N-terminal kinase (JNK) activate transcription factors that include p53, STAT1, STAT3, MYC, cAMP response element-binding protein (CREB) and nuclear factor–KB (NF-KB) [64]. Moreover, transcriptional regulators YAP and TAZ are activated in response to stiff mechanical cues in response to RhoGTPases and acto-myosin cytoskeletal tension [77]. Activation of beta-catenin in response to stiff ECM is also reported to be dependent on integrin/FAK stimulation (Fig. 1 H) [78]. Most of these pathways and transcription factors are involved in initiation/progression of aggressive forms of breast cancer such as triple negative breast cancer.
Inside the nucleus, a mesh of intermediate filaments called laminins interact with both the cytoskeleton and the chromatin [79]. These laminins respond to mechanical cues sensed by the cells at focal adhesions and transmitted through the cytoskeleton [80]. The cytoskeleton is physically linked to the nucleus via LINC (linker of nucleoskeleton and cytoskeleton) complexes [80]. In response to ECM stiffness, LINC complexes are reinforced and type-A laminins (LMNA) are stabilized, increased and assembled to counteract the external mechanical force [80, 81]. Upon actin polymerization, MAL is shuttled from the cytoplasm and accumulates in the nucleus [82]. MAL, a coactivator of the transcription factor serum response factor (SRF) activates SRF to turn on genes regulating cellular motility and contractility such as vinculin, actin and SRF itself [83], contributing to a tension-induced “inside-out signaling”. Thus, the nucleus feeds back to the cytoskeleton to balance the extrinsic and intrinsic forces (Fig. 1 H) [80].
Another factor that is activated in response to mechanical force is secreted TGFβ [84]. Activated TGFβ stimulates matrix modifying proteins such matrix metalloproteinases (MMPs) and thus drastically change the characteristics of the extracellular matrix. In sever scenarios, chronic activation of TGFβ was shown to induce tissue fibrosis in the liver and kidney [85]. TGFβ inhibition by FDA-approved Pirfenidone is used clinically to treat idiopathic pulmonary fibrosis [86, 87], but its use in treating other fibrotic diseases is not yet established.
Conclusions:
The relative ratio of breast collagen to adipose cell volume roughly correlates with mammographic density. However, collagen and adipose cells are not the only contributors to mammographic density. Currently, we do not have the clinical ability to sort out the relative contributions of epithelial versus fibroblasts/stromal cells in determining mammographic density. Mammographic density inversely correlates with age and body mass index (BMI), is positively associated with hormone use, and varies in different racial and ethnic groups. In population-based studies increased breast density is associated with a 2 to 6-fold increased risk of developing breast cancer, however, the biological mechanism for this increased breast cancer-risk is not well understood. While mammographic density correlates with breast cancer-risk in populations, mammographic density does not accurately predict breast cancer risk in individuals. There is evidence that a decrease in breast density in women taking tamoxifen is associated with a reduction in breast cancer risk reduction. However, is not known whether the reduction in breast density specifically reduced the breast cancer risk.
Acknowledgments
Supported by:
National Institutes of Health/National Cancer Institute (NIH/NCI) grants R01CA155664, R01CA158668, R01CA170851, R01CA192914, and U01CA189283 (all to VLS) and P30CA033572. The funders had no role in the manuscript design, data analysis, decision to publish, or preparation of the manuscript.
References
Full text links
Read article at publisher's site: https://doi.org/10.1007/s12609-019-00316-4
Read article for free, from open access legal sources, via Unpaywall:
https://www.ncbi.nlm.nih.gov/pmc/articles/PMC7728377
Citations & impact
Impact metrics
Alternative metrics

Discover the attention surrounding your research
https://www.altmetric.com/details/136787175
Article citations
Mammographic Density Laws and Inclusion-Time for Change.
JAMA Oncol, 8(1):39-40, 01 Jan 2022
Cited by: 4 articles | PMID: 34817549 | PMCID: PMC8926005
Similar Articles
To arrive at the top five similar articles we use a word-weighted algorithm to compare words from the Title and Abstract of each citation.
Mammographic density: intersection of science, the law, and clinical practice.
Am Soc Clin Oncol Educ Book, 01 Jan 2013
Cited by: 0 articles | PMID: 23714458
Mammographic density, parity and age at first birth, and risk of breast cancer: an analysis of four case-control studies.
Breast Cancer Res Treat, 132(3):1163-1171, 06 Jan 2012
Cited by: 35 articles | PMID: 22222356 | PMCID: PMC3336030
Mammographic density and estrogen receptor status of breast cancer.
Cancer Epidemiol Biomarkers Prev, 13(12):2090-2095, 01 Dec 2004
Cited by: 66 articles | PMID: 15598766
Clinical and epidemiological issues in mammographic density.
Nat Rev Clin Oncol, 9(1):33-40, 06 Dec 2011
Cited by: 61 articles | PMID: 22143145
Review
Funding
Funders who supported this work.
NCI NIH HHS (11)
Grant ID: R01 CA192914
Grant ID: U01 CA189283
Grant ID: R01 CA114068
Grant ID: P30 CA033572
Grant ID: R01 CA098441
Grant ID: R01 CA155664
Grant ID: R01 CA170851
Grant ID: U54 CA285116
Grant ID: R01 CA088799
Grant ID: R01 CA158668
Grant ID: R01 CA220693