Abstract
Background and objectives
To assess the molecular landscape of B-cell subpopulations across different compartments in patients with neuromyelitis optica spectrum disorder (NMOSD).Methods
We performed B-cell transcriptomic profiles via single-cell RNA sequencing across CSF, blood, and bone marrow in patients with NMOSD.Results
Across the tissue types tested, 4 major subpopulations of B cells with distinct signatures were identified: naive B cells, memory B cells, age-associated B cells, and antibody-secreting cells (ASCs). NMOSD B cells show proinflammatory activity and increased expression of chemokine receptor genes (CXCR3 and CXCR4). Circulating B cells display an increase of antigen presentation markers (CD40 and CD83), as well as activation signatures (FOS, CD69, and JUN). In contrast, the bone marrow B-cell population contains a large ASC fraction with increased oxidative and metabolic activity reflected by COX genes and ATP synthase genes. Typically, NMOSD B cells become hyperresponsive to type I interferon, which facilitates B-cell maturation and anti-aquaporin-4 autoantibody production. The pool of ASCs in blood and CSF were significantly elevated in NMOSD. Both CD19- and CD19+ ASCs could be ablated by tocilizumab, but not rituximab treatment in NMOSD.Discussion
B cells are compartmentally fine tuned toward autoreactivity in NMOSD and become hyperreactive to type I interferon. Inhibition of type I interferon pathway may provide a new therapeutic avenue for NMOSD.Free full text

B-Cell Compartmental Features and Molecular Basis for Therapy in Autoimmune Disease
Abstract
Background and Objectives
To assess the molecular landscape of B-cell subpopulations across different compartments in patients with neuromyelitis optica spectrum disorder (NMOSD).
Methods
We performed B-cell transcriptomic profiles via single-cell RNA sequencing across CSF, blood, and bone marrow in patients with NMOSD.
Results
Across the tissue types tested, 4 major subpopulations of B cells with distinct signatures were identified: naive B cells, memory B cells, age-associated B cells, and antibody-secreting cells (ASCs). NMOSD B cells show proinflammatory activity and increased expression of chemokine receptor genes (CXCR3 and CXCR4). Circulating B cells display an increase of antigen presentation markers (CD40 and CD83), as well as activation signatures (FOS, CD69, and JUN). In contrast, the bone marrow B-cell population contains a large ASC fraction with increased oxidative and metabolic activity reflected by COX genes and ATP synthase genes. Typically, NMOSD B cells become hyperresponsive to type I interferon, which facilitates B-cell maturation and anti–aquaporin-4 autoantibody production. The pool of ASCs in blood and CSF were significantly elevated in NMOSD. Both CD19− and CD19+ ASCs could be ablated by tocilizumab, but not rituximab treatment in NMOSD.
Discussion
B cells are compartmentally fine tuned toward autoreactivity in NMOSD and become hyperreactive to type I interferon. Inhibition of type I interferon pathway may provide a new therapeutic avenue for NMOSD.
Neuromyelitis optica spectrum disorder (NMOSD) is a devastating inflammatory disease of the CNS, characterized by relapsing optic neuritis and longitudinal extensive transverse myelitis. NMOSD was recognized as a new disease entity, distinct from multiple sclerosis (MS), on the discovery of autoantibodies against water channel aquaporin-4 (AQP4-IgG) on astrocytes.1,2 B cells are involved in the production of AQP4-IgG and neuronal damage. Although a multitude of B-cell aberrances have been proposed to contribute to astrocytic damage and demyelination in NMOSD,3-5 the triggers for pathogenic B-cell activation remain elusive. Because of the scarcity of B cells in CSF, a comprehensive analysis of B cells has not been performed in numerous inflammatory neurologic diseases.6 Therefore, the anatomic site for persistent B-cell activation remains debated. The efficacy of B-cell depletion monoclonal antibodies (mAbs) with anti-CD20 rituximab, and recently with anti-CD19 inebilizumab, in reducing relapses in NMOSD further reveals the deleterious effects exerted by B cells in NMOSD.7-9 However, heterogeneous responsiveness to rituximab leaves 10%–25% of patients who experience relapses after B-cell depletion treatment.10,11 In addition, sera AQP4-IgG levels do not always directly correspond with B-cell depletion in patients with NMOSD,12 indicating heterogeneous properties of B-cell populations.13
A critical assessment of the B-cell landscape across different organ systems would advance understanding in the immune mechanisms of diverse B cells, which drive NMOSD relapses. Here, we performed high-dimensional single-cell sequencing to comprehensively characterize B-cell signatures across CSF, blood, and bone marrow in NMOSD. Analysis at the single-cell resolution reveals a major desynchronization of B-cell subsets with specific gene expression programs across different compartments in NMOSD, and the characterization of the molecular spectrum underlines potential new therapeutic targets and differential responsiveness to major NMOSD therapies.
Methods
Study Design and Patient Enrollment
To identify the B-cell landscape across CSF, blood, and bone marrow from patients with NMOSD, 11 patients with NMOSD with positive AQP4-IgG were enrolled for single-cell sequencing. Inclusion criteria for patients with NMOSD were defined: (1) older than 18 years; (2) NMOSD diagnosed in accordance with the diagnosis criteria14; and (3) experiencing acute attacks without receiving high-dose methylprednisolone pulse, IV immunoglobulin, or plasma exchange treatment. Exclusion criteria included presence of other autoimmune diseases, acute myocardial infarction, heart failure, liver diseases, tumor or hematologic system diseases, and concomitant use of B cell–depleting therapies. CSF and peripheral blood were pooled from patients with NMOSD (n = 4 CSF samples and n = 5 blood samples). Blood from 3 healthy controls was collected as controls (n = 3 samples) (eTable 1, links.lww.com/NXI/A565). Bone marrow samples were acquired from a subset of 2 patients with NMOSD (n = 2 samples). Sampling was approved by the Institutional Review Board of Beijing Tiantan Hospital. All participants signed the written informed consents.
Single-Cell RNA Sequencing (scRNA-seq)
Fresh samples of CSF, blood, and bone marrow aspirant were processed immediately after collection.8 Single B cells were obtained via flow cytometry sorting (cell viability >90%), and sequencing was performed on the 10× Genomics platform.
Single-cell RNA-seq libraries were constructed with 5′ Library and Gel Bead Kit and V(D)J Enrichment Kit, as previously reported.15 RNA sequence reads and BCR sequences were aligned and quantified using the Cell Ranger Single-Cell Software Suite (version 3.0.2, 10× Genomics) against the GRCh38 human reference genome. Cells were filtered to retain those with >600 detected genes and those with a proportion of mitochondrial gene counts lower than 10%. In all, 30,349 cells were kept for further analysis using Scanpy 1.4.3.16 Gene expression data were normalized and log transformed using the “sc.pp.normalized_per_cell” and “sc.pp.log1p” functions with default parameters.
To detect possible batch effects, we build batch-balanced K nearest neighbor graph with Python module bbknn. Bbknn is an effective and intuitive tool for minimizing batch effects, and its functions create a corresponding neighbor graph for subsequent use in clustering, pseudotime, and uniform manifold approximation and projection for dimension reduction (UMAP) visualization. Following implementation of batch correction through bbknn across patients, the resulting graph showed no obvious batch-biased clustering pattern.
Unsupervised Clustering and Dimensionality Reduction
The genes used for dimensionality reduction and clustering were chosen on the “sc.pp.highly_variable_genes” function with the patient ID specified as batch keys. Nine hundred eighteen highly variable genes were detected and used for scaling with “sc.pp.scale.” Next, principal component analysis matrix was calculated with “sc.tl.pca” function, and the top 40 primary components were used to build batch-balanced K nearest neighbor graph with Python module bbknn, which was then used to identify clusters.17,18 Cluster-specific genes were identified using the Wilcoxon rank-sum test. Clusters were annotated based on their expression of well-characterized marker genes. Gene Ontology (GO) term enrichment analysis was performed using the R package cluster Profiler.19
Initial clustering identified 5 major cell types including T and NK cells, hematopoietic stem cells and erythroid cells, plasmacytoid dendritic cells, B cells, and antibody-secreting cells (ASCs). Excluding B cells and ASCs, other major cell types were excluded from the downstream analysis, leaving 30,587 cells for the following steps. After clustering of B cells and ASCs, detailed status of memory B cells were further extracted. Each round of clustering contained the following steps: finding highly variable genes, calculating principal component analysis matrix, and building batch-balanced K nearest neighbor graphs.
To achieve dimensionality reduction, we calculated UMAP and diffusion-map on the batch-balanced nearest neighbor graphs using default parameters. For visualization, partition-based graph abstraction was also calculated using Scanpy 1.4.3. The gene expression changes shown in figures are all significant (adjusted p value <0.05, Wilcoxon test).
B-Cell Receptor (BCR) Analysis
The 5′ protocol generated cells with both expression data and BCR information were retained for analysis. BCR sequences with high confidence, detectable V genes, J genes, CDR3 nucleotide sequences, and those with more than 2 unique molecular identifier (UMI) counts were retained for downstream analysis. For cells with more than 1 heavy (IGH) or light (IGK or IGL) assembled, heavy-light chain pairs with the highest UMI counts were defined as the dominant heavy-light chain pair in the corresponding cell. If 2 cells had identical dominant heavy-light chain pairs (that is, identical V gene, J gene, and CDR3 nucleotide sequence), these cells were identified as clonal B cells with identical BCRs. In application, 21,063 B cells with BCR information were used in the following integrated clonal analysis; 744 of them were B cells with clonal BCRs, these clonal cells were then analyzed using the previously reported STARTRAC method.20
Gene Set Variation Analysis (GSVA)
To illustrate possible differential activity among cell groups, we calculated GSVA matrices for B cells and ASCs.21 Hallmark gene sets were downloaded from MSigDB (v7.0) and filtered out shared genes within each gene set. The “gsva” function implemented in R package GSVA was applied to calculate the matrix with parameter “min.sz = 10”; this limited the minimum size of the resulting gene sets. The resulting matrix was then divided into unique groups of cells and tested for significantly changed pathway using the Wilcoxon sum test. p Values were adjusted (Benjamini-Hochberg method) and then log transformed for visualization in bar plots. We also assessed the statistical significance of interferon-stimulated genes, inflammation-related genes, and exhaustion genes in all B-cell subpopulations (eTables 2–4, links.lww.com/NXI/A565). Scores based on these specific gene sets were calculated.
Trajectory Analysis and Visualization of the Temporarily Expressed Genes
When calculating pseudotime of naive B cells, the cell with the smallest value on the UMAP1 axis was assigned as the root cell; diffusion pseudotime was then calculated.22 This pseudotime scale was further separated into 3 groups, according to the expression pattern of the displaying genes. Differentially expressed genes were identified using the function “FindAllMarkers,” with parameters “only.pos = T, min.pct = 0.1, logfc.threshold = 0.25.” Annotations for these groups of cells were made based on differentially expressed genes.
B-Cell Subset Analysis by Flow Cytometry and Specific Expression of Type I Interferon–Related Genes
To analyze B-cell subset distribution and expression of type I interferon–related genes in NMOSD, patients with MS, and healthy controls, we enrolled to another 3 cohorts made up of 27 patients with NMOSD with attacks, 15 patients with relapsing-remitting MS, and 16 healthy controls whose age and sex were matched to patients with NMOSD or MS (eTable 5, links.lww.com/NXI/A565).
Peripheral and CSF B cells were acquired and stained with the following antibodies: anti-CD19 (APC-Cy7), anti-CD38 (Alexa Fluor 488), anti-CD27 (BV421), anti-CD24 (PE-Cy7), and anti-IgD (PE) (all from BioLegend). The gating strategy for B-cell subpopulations used in flow cytometry is illustrated in eFigure 1, links.lww.com/NXI/A565.
For PCR experiments, B cells were isolated from PBMCs by negative selection using magnetic beads (Human B cell Isolation kit II, Miltenyi Biotec). The final B-cell purity was >90% (determined by fluorescence activated cell sorting [FACS]). All isolation steps were performed on ice and with ice-cold solutions. We selected genes that best reflected the global Type I interferon (IFN) signature. We performed real-time PCR with on CFX connect (Bio-Rad). These genes and primers used are listed in eTable 6, links.lww.com/NXI/A565. Relative expression levels were normalized against GAPDH, a house housekeeping gene, calculated by the 2_ΔΔCt method. Data are presented as fold change relative to control samples.
B-Cell Culture, Interferon Stimulation, and AQP4-IgG Measurement
B cells were obtained by magnetic cell sorting for cell culture experiments. Purified B cells were cultured in 96-well plates (2 × 105 cells per well) in complete Roswell Park Memorial Institute-1640 medium. To test the effects of interferon on cell viability and apoptosis, varying concentrations of IFN-α2b (PBL Assay Science) were applied and assessed by an Apoptosis Detection Kit (BioLegend). In B cell differentiation experiments, cells were stimulated with anti-IgM (20 ug/mL, Invitrogen), and IFN-α2b (5,000 U/mL), preincubated with or without IFN α-interferon-α/β receptor-inhibitor (IFNAR-IN)-1 hydrochloride (5 μM, MedChemExpress), an inhibitor of IFN-α2b and IFNAR interaction before IFN-α2b treatment.23,24 Following 3-day culture, B-cell subtypes were assessed by FACS. In the last set of experiments, cells were plated in duplicates in the B cell culture medium, at 2 × 105 cells/200 ul in 96-well plates in the presence of Soluble CD40-ligand (sCD40L; 100 ng/ml, R&D Systems), interleukin-2 (IL-2; 50 ng/ml R&D Systems), interleukin- 21 (IL-21; 50 ng/ml R&D Systems), interleukin-6 (IL-6; 5 ng/ml R&D Systems), tumor necrosis factor-α (TNF-α; 5 ng/ml PeproTech), with or without IFN-α2b (5000 U/ml). After 10 days in vitro, culture supernatant was collected to quantify AQP4-IgG, as previously reported.4,25
Assessment of Peripheral Blood ASCs Before and After Treatment
Ten patients with NMOSD receiving rituximab and another 10 patients receiving tocilizumab were enrolled (eTable 7, links.lww.com/NXI/A565). ASCs were measured using flow cytometry. Peripheral blood was collected 6 months before and after rituximab or tocilizumab treatment.
Statistical Analysis
In comparing the parameters of B-cell subsets between groups, the Kruskal-Wallis test with Dunn procedure was used. The 2-tailed Mann-Whitney U test compared the gene set-based scores of patients with NMOSD with healthy controls. The comparison of blood ASC counts before and after the therapeutic intervention paired t test was used. All statistical analyses were performed in SAS software (version 9.4).
Data Availability
Anonymized data are available on request from any qualified investigator.
Results
Distinctive Distribution Pattern of B-Cell Subpopulations Across Bone Marrow, Blood, and CSF in NMOSD
We sought to determine B-cell populations across different anatomic compartments in patients with NMOSD. To this end, a total of 30,349 B cells were pooled from CSF, blood, and bone marrow. We performed scRNA-seq using 10× Genomics on CD3−CD14−CD16−CD56− B cells, including ASCs.26 Integrated analysis reveals distinct clusters of B cells: IGHDhighCD27low naive B cells, IGHDlowCD27high memory B cells, CD38highCD27high ASCs, and ITGAXhighTBXA21high age-associated B cells across tissues (Figure 1A).
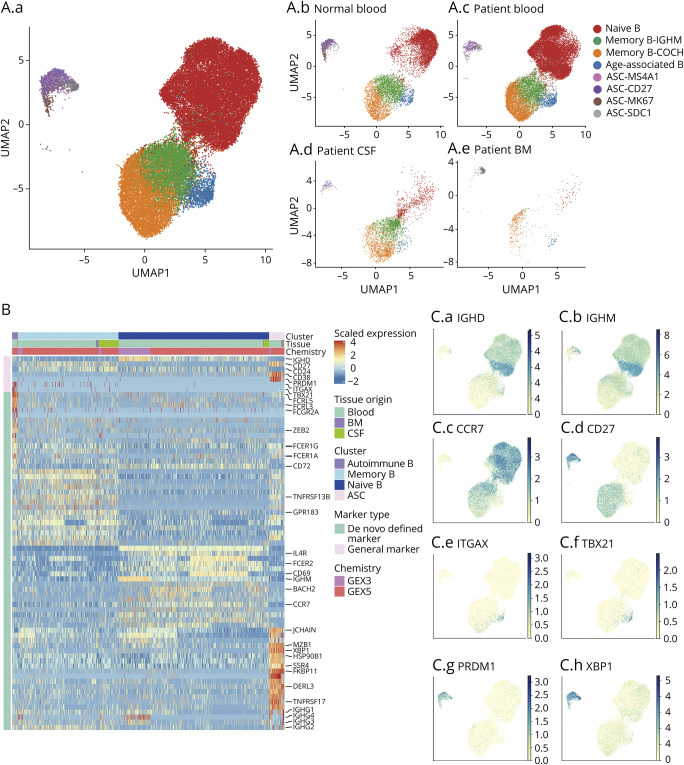
(A. A.a) Integrated analysis reveals distinct subpopulations of B cells in NMOSD including naive B cells, memory B cells, age-associated B cells, and antibody-secreting cells (ASCs). (A.b-e) Individual plots showing B-cell subpopulations in control blood (A.b), NMOSD blood (A.c), NMOSD CSF (A.d), and NMOSD bone marrow (BM) (A.e). Two clusters of memory B cells were identified: IGHMhighCOCHlowITGB1low and IGHMlowCOCHhigh ITGB1high memory B cells. Four clusters of ASCs were identified: MS4A1high, CD27high, MK67high, and SDC1high ASCs. (B) Heatmap showing unsupervised hierarchical clustering of gene expression pattern in B cells from NMOSD blood, bone marrow, and CSF. Scaled expression of discriminative gene sets for each B cell subpopulation is shown. (C) Plots showing the characteristic expression of indicated molecules in B-cell subpopulations. NMOSD = neuromyelitis optica spectrum disorder.
Naive B cells express maker genes IGHD, IGHM, and IL4R, but with very low levels of CD27. In contrast, memory B cells upregulated markers CD27, concurrent with low expression of IGHD. High levels of CD86 and IGHG1 on memory cells suggest enhanced B-cell activation and differentiation. ASCs comprising plasmablasts and plasma cells highly expressed XBP1 and MZB1 and TNFRSF17 (BCMA). Intriguingly, age-associated B cells expressed upregulated ITGAX (CD11C) and TBX21 (T-BET) with minimal expression of IGHD, CD27, and CD24.27 High expression of FCGR2A, FCER1G, and FCER1A in age-associated B cells suggests increased antigen-presenting activity (Figure 1, B and C).
Unbiased analysis revealed 2 subclusters of memory B cells, IGHMhighCOCHlowITGB1low and IGHMlowCOCHhighITGB1high B cells (Figure 1A). Cochlin, encoded by the COCH gene, is a critical modulator of maturation for innate immune cells.28,29 It is reported to be differentially expressed in B cells, predominately switched memory B cells.28 We speculate that the upregulation of cochlin may promote maturation of B cells as well as AQP4-IgG production in NMOSD.
In addition, we identified 4 clusters of ASCs: MS4A1high, CD27high, MK67high, and SDC1high ASCs. We performed an integrated analysis to assess the expression of inflammatory markers among all identified B-cell clusters. IL-6 was mainly expressed in naive B cells as well as IGHMhigh memory B cells. Memory B cells highly expressed IL-1α. IL-1β was exclusively expressed in SDC1high plasma cells. Age-associated B cells upregulated genes related to the TNF-α signaling pathway, including TNF-α, TRAF3, and TRAF6 (eFigure 2, links.lww.com/NXI/A565).
Compartmental Features of B Cells and Chemokine Receptor–Expressing CSF B Cells in NMOSD
Having determined the distribution patterns, we next compared the features of B cells across CSF, blood, and bone marrow via comprehensive analysis of B-cell signatures through Seurat package. In CSF, we identified 9 subsets of B cells that are heterogeneous among CSF samples (eFigure 3A–B, links.lww.com/NXI/A565). Naive B cells, switched memory B cells, ASCs, and age-associated B cells were also clustered in CSF. Furthermore, we found 3 different B cell status expressing intermediate levels of IGHD and CD27, and they were identified as IGHD+CD27+ unswitched memory B cells. Two B-cell subsets expressing minimal levels of IGHD and CD27 were identified as IGHD−CD27− double-negative memory B cells; low expressions of CD86 and CD24 were also featured in these cells (eFigure 3C–D, links.lww.com/NXI/A565). GO pathway enrichment revealed enriched MHC II protein complex and type I interferon pathways in CSF memory B cells and age-associated B cells, suggesting augmented inflammatory activity of B cells in CSF in NMOSD (eFigure 3E, links.lww.com/NXI/A565).
Using canonical protein markers on B cells, we next compared the proportions of B-cell subsets in NMOSD, MS, and healthy controls. CSF double-negative B cells and ASCs were significantly elevated in patients with NMOSD in comparison with patients with MS or healthy controls. In peripheral blood, double-negative B cells were increased in patients with NMOSD compared with those with MS. Both patients with NMOSD and MS presented increased percentages of ASCs compared with healthy controls (eFigure 4, links.lww.com/NXI/A565).
Compared with healthy controls, NMOSD blood B cells upregulated genes related to antigen presentation, including CD40, CD83, and HLA-DQB1. There were also increased expression of inflammatory markers FOS, CD69, JUN, JUNB, and DUSP1 in B cells from NMOSD. However, regulatory TGF-β signaling (TGFB1 and TGFBR2) was reduced in blood NMOSD B cells (Figure 2, A and B, eTable 8, links.lww.com/NXI/A566). These results suggest that peripheral B cells acquired activated and inflammatory status in NMOSD. In contrast, bone marrow B cells in NMOSD displayed a less active inflammatory profile, with reduced expression of inflammatory markers when compared with those in blood (Figure 2A, eTable 9, links.lww.com/NXI/A567). Notably, CSF B cells in NMOSD expressed higher levels of CXCR3 and CXCR4, implying a potential involvement of chemotactic effect for B cells to enter CNS from blood. Proinflammatory molecules JAK1, SOCS1, and STK17A were significantly increased in NMOSD CSF B cells compared with those from NMOSD blood (Figure 2A, eTable 10, links.lww.com/NXI/A568).
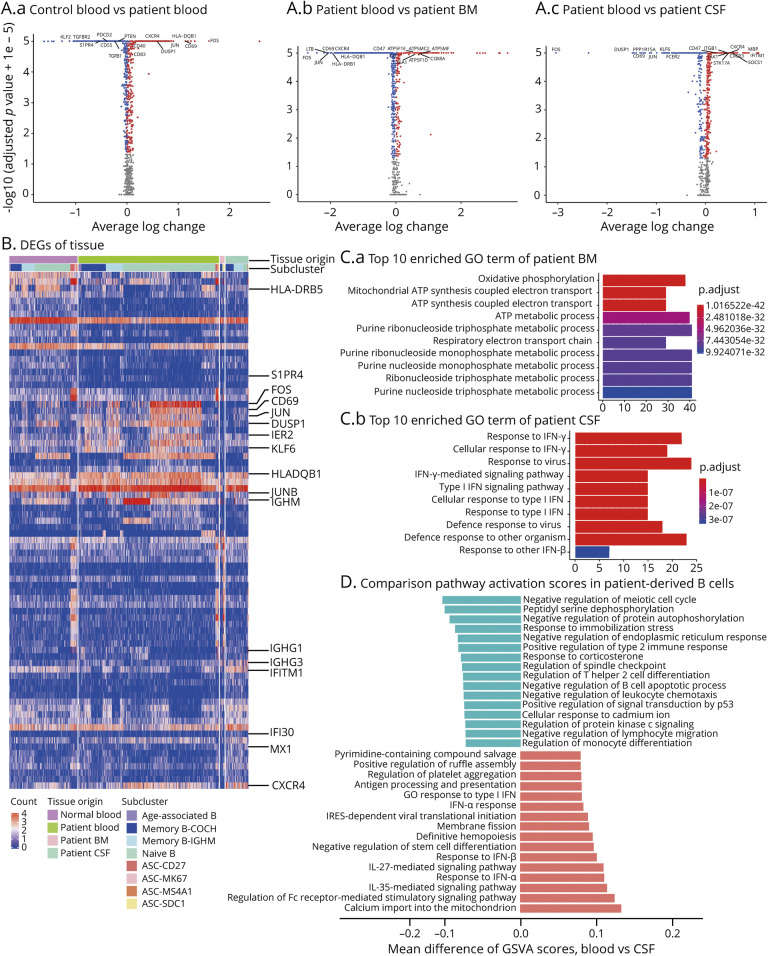
(A) Scatter dot plots showing expression of inflammation-related genes in B cells from NMOSD blood (A.a), bone marrow (A.b), and CSF (A.c). Blood from healthy individuals was used as control. Red dots: upregulated genes. Blue dots: downregulated genes. (B) Heatmap showing differential expression pattern of selected inflammation-related genes in B-cell subsets across blood, CSF, and bone marrow. NMOSD blood vs control blood: increased genes include FOS, CD69, JUN, DUSP1, PPP1R15A, IER2, ZFP36, and HLA-DQB1; reduced genes include S1PR4, YBX3, and SNHG7. (C) Bar graphs showing top 10 enriched gene ontology (GO) terms of indicated pathways in B cells from NMOSD bone marrow (C.a) or blood (C.b). Color scheme is based on adjusted p values from high (blue) to low (red). GO pathway analysis shows significant increase of type I interferon pathway in CSF B cells but not in bone marrow B cells. (D) Bar graph showing the differences in indicated pathways between blood B cells and CSF B cells in NMOSD. Shown in t-statistics from low (green) to high (red) resulting from the GSVA score. NMOSD = neuromyelitis optica spectrum disorder.
GO pathway analyses reveal that increased oxidative phosphorylation and ATP synthesis in bone marrow B cells, pointing to augmented metabolic activity, is reflected by COX genes (COX 6A, COX7C, COX5A, and COX8A) and ATP synthase genes (ATP5F1E, ATP5F1D, ATP5MG, and ATP5ME). CSF B cells possessed amplified type I interferon pathway activation (Figure 2C). Furthermore, GSVA scores of major inflammatory-related genes revealed the upregulation of Fc receptor–mediated stimulatory pathway and IL-27/35–mediated pathway in CSF B cells (Figure 2D).
Together, these results imply that peripheral B cells have increased activity related to antibody production in NMOSD. In contrast, B cells in the CNS have higher activity related to antigen presentation and proinflammatory activity.
B Cells Become Hyperreactive to Type I Interferon in NMOSD
To understand the mechanisms underlying the compartmental differences of B cells in the CNS vs the periphery, we screened major B-cell pathways including STAT1/3, proteasome activation, and interferon signaling. Among the upregulated genes in CSF B cells, we found a prominent increase of interferon-related signatures in CSF B cells (Figure 3A). Thereafter, we assessed IFN response score of each cell subset. We used unsupervised hierarchical clustering of 145 IFN-related genes that distinguished IFNhigh cells (upregulated) and IFNlow (downregulated) cells. In patients with NMOSD, IFNhigh B cells were exclusively dominant in all subclusters of CSF B cells. However, all clusters of B cells in bone marrow expressed IFNlow signatures (Figure 3B). Correspondingly, patients with NMOSD had significantly increased IFN scores in all B-cell clusters compared with healthy controls (Figure 3C, eFigure 5A, links.lww.com/NXI/A565).
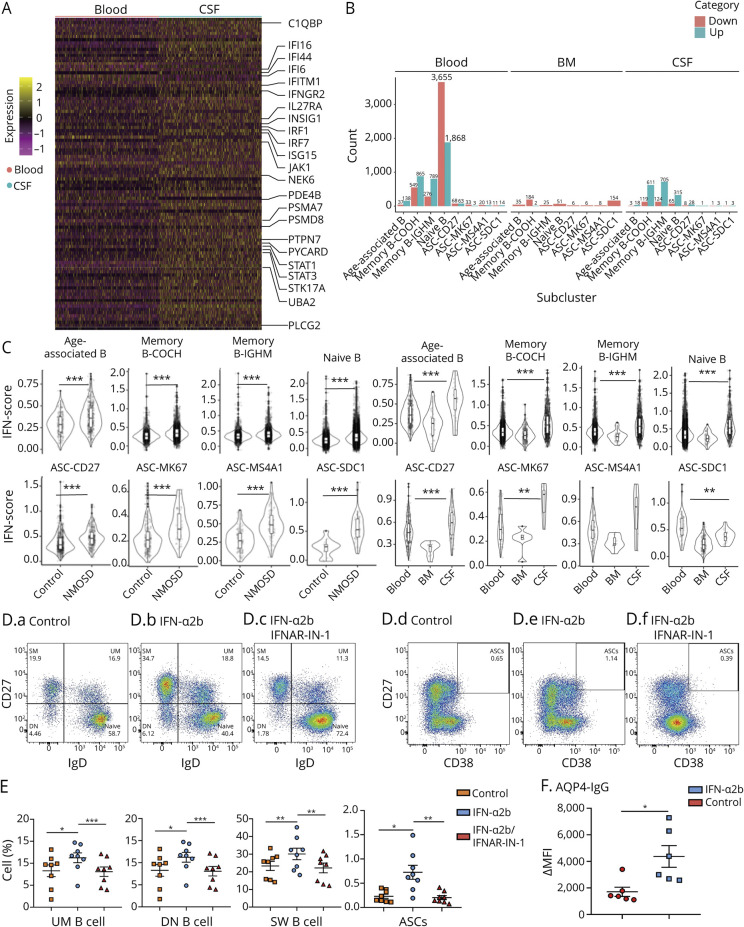
(A) Unsupervised hierarchical clustering of 145 IFN-related genes expressed in blood B cells vs CSF B cells obtained from patients with NMOSD. (B) Counts of B-cell subpopulations with upregulation or downregulation of interferon-related genes in blood, bone marrow, and CSF. Upregulation: green; downregulation: red. (C) Left panel: Z-scores of IFN-related genes in patients with NMOSD vs controls. Right panel: Z-scores of IFN-related genes in main clusters of B cells from blood, CSF, and bone marrow in patients with NMOSD. (D and E) Flow cytometry plots and bar graphs show B-cell subpopulations from NMOSD blood in response to IFN-α2b stimulation with or without an IFN-α2b inhibitor. UM B cells: memory IgM cells (IgM+IgD+CD27+); DN B cells: double-negative B cells (CD27−IgD−); SW B cells: switched memory B cells (IgD−CD27+); ASCs: antibody-secreting cells (CD27highCD38high). n = 8 per group. (F) Effects of IFN-α2b stimulation on production of AQP4-IgG in the supernatant of cultured B cells. B cells were obtained from NMOSD blood and cultured in the presence of sCD40L (100 ng/mL), IL-2 (50 ng/mL), IL-21 (50 ng/mL), IL-6 (5 ng/mL), and TNF-α (5 ng/mL) for 10 days, with or without IFN-α2b (5,000 U/mL). Data for AQP4-IgGs are expressed as median fluorescence intensity (ΔMFI) values. n = 6 per group. ASC = antibody-secreting cell; NMOSD = neuromyelitis optica spectrum disorder. *p < 0.05, **p < 0.01, ***p < 0.001.
In addition, we found that naive and memory B cells had higher scores for inflammation-related genes (efigure 5B–C, links.lww.com/NXI/A565). MS4A1high and CD27high ASCs additionally displayed higher scores for exhaustion genes in patients with NMOSD (eFigure 5D–E, links.lww.com/NXI/A565). Compartmental comparison analysis showed that naive and memory B cells had higher Z-scores of inflammation-related and exhaustion genes in the CSF. Memory B cells and CD27high ASCs had higher Z-scores for exhaustion-related genes in CSF (eFigure 6, links.lww.com/NXI/A565).
Type I Interferon Signaling Promotes B-Cell Maturation and Anti-AQP4 Antibody Production
We next investigated whether type I interferon signaling could promote AQP4-IgG production in NMOSD. Using an established culture system,4 we cultured sorted blood B cells and stimulated them with a stepwise concentration of IFN-α2b and various cytokines. After 3 days of culture, we found that IFN-α2b stimulation led to a reduced number of naive B cells together with an increased activation of CD27+IgD− class-switched memory B cells, CD27highCD38high ASCs, and CD27−IgD− double-negative B cells. Treatment with IFN-α receptor inhibitor IFNAR-IN-1 reduced the differentiation of naive B cells (Figure 3, D and E). Furthermore, increased AQP4-IgG was detected in the supernatant following IFN-α2b stimulation (Figure 3F). To compare the activation status of type I interferon-related genes between NMOSD, MS, and healthy controls, we examined the expression levels of IRF4, IFI44, IFI44L, MX1, and IFI6 genes in blood B cells using RT-PCR. We found that these genes were consistently upregulated in NMOSD compared with MS or healthy controls (eFigure 7, links.lww.com/NXI/A565).
B-Cell Development and Its Association With Type I Interferon Signaling in NMOSD
Pathogenic AQP4-IgG may originate from autoreactive naive B cells13; therefore, we performed transcriptome analysis of naive B cells from NMOSD blood and CSF to investigate whether type I interferon pathway affects the development of pregerminal center inexperienced B cells that were heterogeneous for IGHDhighCD27low B cells. We identified the altered expression of IGHD, CCR7, SELL, CD24, NR4A1, and SOX4 in naive B cells from NMOSD blood (Figure 4A). Trajectory analysis indicates a stepwise reduction in the expression of CD24 otherwise an upregulation of CCR7. New emigrant marker CD24 and MME (membrane metalloendopeptidase) were highly expressed in early naive B cells, which were defined as transitional B cells (Figure 4, A and B). Unbiased analysis identifies CCR7 as a critical marker upregulated in naive B cells from NMOSD CSF and blood (Figure 4C). CCR7 was hardly detectable in transitional B cells but enriched in other naive B cells. In light of differential expression of CCR7, we identified 3 different subsets of naive B cells in NMOSD CSF and blood: transitional B cells (CD38highCCR7low), early naive B cells (CD38low CCR7int), and late naive B cells (CD38lowCCR7high) (Figure 4C). Compared with early naive B cells, late mature naive B cells had higher expression of CD83 and JUN but lower KLF2 (Kruppel-like factor 2) and PFN1 (profilin 1). These results suggest that inhibition of CCR7-dependent B-cell chemotaxis may serve as a potential approach to increase the retention of naive B cells in the periphery and inhibit their homing into the CNS.

(A) Expression of indicated markers in naive B cells from NMOSD blood. (B) Trajectory analysis shows early development signature membrane metalloendopeptidase (MME) expressed mainly in transitional B cells. (C) Heatmap of naive B cells in NMOSD blood, bone marrow (BM), and CSF showing 3 clusters: CCR7− transitional B cells; CCR7int-naive B cells; and CCR7high-naive B cells. (D) Interferon response among 3 clusters of naive B cells. Intermediate and late naive B cells displayed strong type I interferon response. (E and F) Indicated groups of cultured blood B cells were treated with vehicle, IFN-α2b, or IFN-α2b + IFN-α receptor inhibitor for 3 days. Flow cytometry analysis revealed increased proportion of transitional B cells (CD24highCD38high) after IFN-α treatment. Inhibition of IFN-α receptor abolished the effects of IFN-α2b treatment, n = 8 per group. NMOSD = neuromyelitis optica spectrum disorder. *p < 0.05, **p < 0.01, ***p < 0.001.
Next, we examined type I IFN signaling activation in 3 B-cell subsets during development. Mature naive B cells presented highest levels of interferon-related pathway activation (Figure 4D). We also find that IFN-α2b treatment can promote naive B cell differentiation toward CD24highCD38high transitional B cells in vitro (Figure 4, E and F). Moreover, IFN-α2b maintained the survival of transitional B cells in a dose-dependent manner (eFigure 8, links.lww.com/NXI/A565). These data show that developmental status of B cells is associated with type I interferon hyperreactivity in NMOSD.
Characteristics of ASCs in NMOSD and Their Responsiveness to Rituximab and Tocilizumab
We report an increased inflammatory activity in CSF ASCs vs blood ASCs in NMOSD. A total of 1,779 genes (1,437 upregulated in the CSF) were differentially expressed between CSF and peripheral blood ASCs. Compared with their peripheral counterparts, CSF ASCs upregulated CD138, XBP1, CD74, and BCR, which are related to antigen exposure. We also find that CSF ASCs upregulated genes related to cytokine/chemokine receptors including IL21R, CXCR4, CX3CR1, CXCR3, and CCR5 (eTable 11, links.lww.com/NXI/A569). RIPOR2, an inhibitor of the small GTPase Ras homolog family member A that controls T lymphocyte proliferation, neutrophil chemotaxis, and adhesion,30 was observed among the downregulated genes in CSF ASCs. In CSF ASCs, we found reduced expression of TNFRSF13B, which are receptors for TNFSF13/APRIL, TALL1, BAFF, and BLYS (Figure 5A). Their related transcription factors NFAT, AP1, and NF-kappa-B are known to control humoral immunity.31 However, bone marrow ASCs upregulated KRTCAP2 and TMEM258, which are related to N-linked glycosylation and protein modification,32 I-kappa-B-α phosphorylation, and proteasome activation (eTable e-12, links.lww.com/NXI/A570). Bone marrow ASCs also possessed reduced expression of cell adhesion signatures such as ITGB7 and ANXA2 compared with blood counterparts (eFigure 9A, links.lww.com/NXI/A565). Bone marrow ASCs also had lower antigen processing and presentation signatures reflected by reduced HLA-A, HLA-B, and HLA-DRB1 compared with CSF ASCs (eFigure 9B, links.lww.com/NXI/A565). GSVA score analysis also shows that blood ASCs in NMOSD had increased responsiveness to type I interferon compared with those of healthy controls. Similar observations were found in other B-cell subsets (Figure 5B).
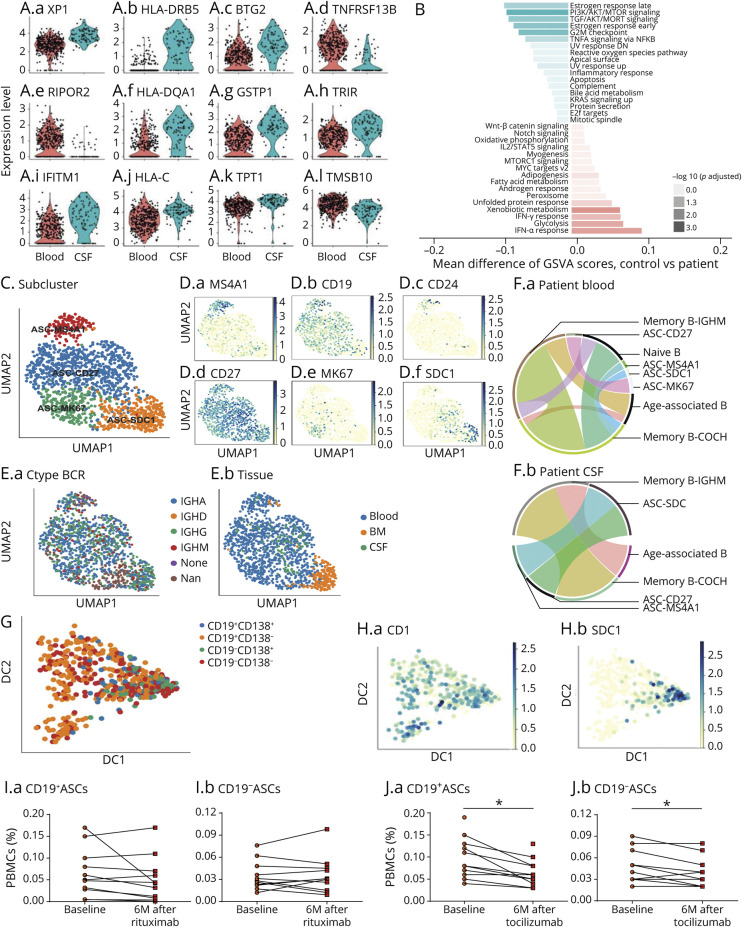
(A) Plots showing different expression of inflammatory signatures in CSF ASCs vs blood ASCs in NMOSD. (B) GSVA scores of indicated pathways expressed in NMOSD ASCs vs healthy controls. Shown in t-statistics from low (green) to high (red) that results from the GSVA score comparison tests comparing ASCs from NMOSD blood vs control blood. (C) UMAP analysis showing 4 subpopulations of ASCs that highly expressed MS4A1, SDC1, MK67, or CD27, respectively. (D) Differential expression of MS4A1 (D.a), CD19 (D.b), CD24 (D.c), CD27 (D.e), MKI67 (D.e), and SDC1 (D.f) in 4 subpopulations of ASCs. (E) Expression of immunoglobulin constant region genes, IGHA, IGHD, IGHG, and IGHM, in subpopulations of ASCs. (F) Plots showing transitioning B-cell clones in patients with NMOSD vs controls (F.a, controls; F.b, NMOSD). (G and H) Trajectory analysis of ASCs reveals 4 subpopulations of ASCs based on the expression level of CD19 and CD138. CD19−CD138+ plasma cells were obviously terminally differentiated. (I and J) Changes of peripheral blood CD19+ and CD19− ASCs before and after rituximab (I) or tocilizumab (J) treatment. The counting of 2 subsets was expressed as the percentage of peripheral blood mononuclear cells (PBMCs). n = 10 patients with NMOSD per group. *p < 0.05. NMOSD = neuromyelitis optica spectrum disorder.
We identified 4 subsets of ASCs: early plasmablasts (MS4A1high), intermediate plasmablasts (MK67high), further differentiated late plasmablasts (CD27high), and plasma cells (CD138high) (Figure 5, C and D). Although all 4 subsets can produce IGHG, clonal BCR was mostly found in intermediate and late plasmablasts (Figure 5, E and F). We also found that a portion of CSF B cells maintained their clonal status in nearly all donors; however, 1 of the 3 healthy donors had no clonal expansion of peripheral B cells (eFigure 10A, links.lww.com/NXI/A565). SDC1high ASCs have the highest STARTRAC-expa index across all clusters (eFigure 10B, links.lww.com/NXI/A565), revealing a strong degree of expansion. Clone analyses showed that plasma cells in NMOSD could be differentiated from mature memory B cells in blood, whereas differentiation in the CNS derives from late plasmablasts and early plasmablasts (Figure 5F).
Next, we analyzed the pseudo-time trajectory transcriptomic graph labeled by CD19 and CD138 in the ASCs. CD19+ CD138− and CD19−CD138− plasmablasts tended to differentiate into terminal CD138+ plasma cells (Figure 5, G and H). Using in silico FACS based on original expression, long-lived CD19−CD138+ plasma cells are counted among CSF ASCs (eFigure 11A–B, links.lww.com/NXI/A565). These cells expressed XBP1, CD38, and MZB1, which are typically observed in short-lived CD19+CD138+ ASCs (eFigure 11C, links.lww.com/NXI/A565).
CD19+ and CD19− ASCs in blood and CSF samples were quantified in patients with NMOSD using flow cytometry (eFigure 12A, links.lww.com/NXI/A565). Both CD19+ and CD19− ASCs were significantly increased in NMOSD compared with MS or healthy controls (eFigure 12B–C, links.lww.com/NXI/A565). Notably, CD19+ and CD19− ASCs cannot be depleted by rituximab treatment (Figure 5I, eFigure 12D, links.lww.com/NXI/A565). Divergently, reduced counts of CD19+ and CD19− ASCs were quantified in patients with NMOSD receiving tocilizumab (Figure 5J, eFigure 12E, links.lww.com/NXI/A565).
Discussion
By producing autoantibodies, B cells directly induce immunopathology in an array of systemic and organ-specific autoimmune disorders. In organ-specific disorders, chronic autoimmunity persists in affected organs such as the joints, pancreas, thyroid, and brain. B-cell features within these organs, either migrated or generated in situ in the case of brain, relative to periphery, are not known. Because B cells are capable of initiating peripheral T-cell proliferation in MS and can mature in cervical lymph node or de novo genesis,33-35 knowledge of organ-specific features is of paramount importance in determining the driving force of autoimmune reaction progression and developing new targeted organ resident B-cell therapies. However, obtaining sufficient and viable B cells for in-depth characterization in target organs of autoimmune diseases, especially in the CNS, has proven challenging. Technical limitations adopted by many prior investigations have restricted the derived insights of organ-specific B cells.
In this work, we have overcome these obstacles by obtaining B cells from CSF, bone marrow, and blood. Eventually, we successfully adopt high-dimensional scRNA-seq to comprehensively characterize and depict B-cell transcriptomic profiles in NMOSD. Classical B cells, such as naive, memory, and ASCs, were clearly clustered, and subclusters of each type were closely functionally related. Intriguingly, we identified CD11c+ age-associated B cells that are dependent on T-bet for generation. These cells show low expressions of CD27 and IGHD, but display strong signatures related to antigen presentation. This cluster of B cells may be associated with double-negative B cells that scarcely express CD27 and IgD but are clonally expanded both in blood and in the CSF, indicating a potential role in activation of NMOSD autoimmunity.36
Overall, blood B cells display proinflammatory profiles in NMOSD. Naive B cells mostly harbored the blood-bearing activation signatures including FOS, CD69, and JUN. Expansion of memory B cells and ASCs is particularly distinct in the brain. CSF B cells including ASCs also expressed high levels of chemotactic CXCR3 and CXCR4, implying a potential central role in cell trafficking in NMOSD. Previous studies showed that B cells that express CXCR3 are abundant in the CNS in MS, which was in line with elevated CXCL10, a CXCR3 ligand, in the CSF. High expression of CXCR3 in NMOSD B cells indicates a common pathway of CXCR3-related B-cell recruitment in MS. As the ligand of CXCR4, CXCL12 is important for lymphocytes to traffic through the blood vessel wall. CXCL12 may also maintain the survival and differentiation of plasma cell.37 In experimental autoimmune encephalomyelitis, synthetic blockade of CXCR3 and CXCR4 contributed to amelioration of CNS inflammation through inhibiting lymphocytes to enter the CNS.38 We speculate that CXCR3 and CXCR4 may also be therapeutic targets in NMOSD.
In contrast, despite bone marrow serves as niches to harbor ASCs, increased expression of genes related to oxidative and metabolic activity such as COX and ATP synthase genes is found. The expression pattern and distribution of B cells among CSF, blood, and bone marrow suggest that B cells exhibit aberrant tolerance and become activated in the periphery. Specifically in CSF, activation of B cells spanning from naive B cells to ASCs indicates that autoimmunity to neuronal injury may be driven in situ.
Intriguingly, patients with NMOSD acquire an interferon response signature in peripheral blood B cells, and the same signature is enhanced in the brain. This is different from MS, in which the basal interferon response is relatively low.39 Indeed, type I interferon, often leading to restriction of CNS autoimmunity and indicated as the first-line therapy for MS, is otherwise noted to exacerbate NMOSD activity.40-42 It is conceivable that type I interferon–induced neurologic deterioration in NMOSD could result from the high serum interferon level and the direct effects of type I interferon to promote B cell maturation.43 Here, we further demonstrate that type I interferon treatment significantly increased AQP4-IgG production by NMOSD blood B cells, and this effect was abolished by interferon pathway inhibition. Thus, identification of hyperresponsiveness of B cells to type I interferon provides pivotal clues for worsening of NMOSD. The distinct effects of type I interferon on NMOSD vs MS suggest different mechanisms of B cells in the overall landscape of 2 diseases, which requires future clarification. For patients with interferon signatures, as in systemic lupus erythematosus, anifrolumab, a type I interferon receptor subunit 1 mAbs, may be a promising treatment in NMOSD.44 This study depicts a molecular picture that explains the differential responsiveness to major disease-modifying drugs in patients with NMOSD. Trajectory analysis reveals CD19− or CD19+ ASCs in patients with NMOSD. These CD19− or CD19+ ASCs in NMOSD display profiles of long-lived plasma cells, which may also reside in CSF. Considering that a portion of patients with NMOSD do not respond well to rituximab or inebilizumab, and CD19− or CD19+ ASCs are spared after rituximab therapy, it is reasonable to infer that these cells may contribute to the nonresponsiveness to rituximab treatment in some patients with NMOSD. Notably, both CD19− and CD19+ ASCs highly express IL-6R. Blood CD19− or CD19+ ASCs were reduced in patients with NMOSD after tocilizumab treatment that block IL-6R signaling pathway, suggesting that targeting these cells using tocilizumab might be beneficial to patients who are refractory to B-cell depletion therapy.
Defining key B-cell clusters and their activation states in NMOSD is an indispensable step to explore potential therapeutic targets. In addition to hyperresponsiveness of type I interferon signaling, our results reveal potential new targets to facilitate future development of immune therapies in NMOSD. Comparison of B-cell subpopulations across different compartments in NMOSD shows that these cells exhibit distinct expression profiles of chemokine receptors in different compartments. The upregulation of CXCR3/CXCR4 in CSF B cells suggests that these chemokine receptors may be a major contributor to B-cell chemotaxis and homing into the CNS. Therefore, targeting chemokine receptors such as CXCR3/CXCR4 may restrict B-cell transmigration to the CNS, cognate therapeutic effects that warrant further investigations in NMOSD.
There were several limitations in this study. First, each CSF sample could not be matched to blood sample for each patient. Because of relatively fewer number of CSF B cells, we pooled CSF B cells from 2 or 3 patients with NMOSD for each CSF sample. Second, in contrast to NMOSD, healthy control CSF contained comparatively very few B cells, which limited us to compare the profiles of CSF B cells with NMOSD. Third, although scRNA-seq obtains expression of large amounts of genes, this approach also requires decent number of cells for analysis.
Collectively, accessibility of B cells in CSF, blood, and bone marrow coupled with the adoption of high-dimensional scRNA-seq allows us to depict B-cell clusters with unique distributions and molecular landscapes. Such a picture offers new insights into disease pathogenesis, the molecular basis for current, and potential new therapies in patients with NMOSD. Results here further stimulate new investigations to address the cost and labor intensiveness associated with this type of study and therefore enable experiments with longitudinal and larger samples to yield more fruitful results.
Acknowledgment
The authors thank members of Jing-Jin Center for Neuroinflammation for support and Samuel Shi for editing.
Glossary
AQP4 | aquaporin-4 |
ASC | antibody-secreting cell |
BCR | B-cell receptor |
FACS | fluorescence activated cell sorting |
GO | Gene Ontology |
GSVA | Gene Set Variation Analysis |
IFN | interferon |
IFNAR-IN | interferon-α/β receptor-inhibitor |
mAb | monoclonal antibody |
MS | multiple sclerosis |
NMOSD | neuromyelitis optica spectrum disorder |
scRNA-seq | single-cell RNA sequencing |
UMAP | uniform manifold approximation and projection for dimension reduction |
UMI | unique molecular identifier |
Study Funding
This study was supported in part by the National Science Foundation of China (91642205, 81830038, 81601019, and 81701176); Advanced Innovation Center for Human Brain Protection, Capital Medical University, Beijing, China; and National Key Research and Development Program of China (2018YFC1312200).
Disclosure
The authors declare no disclosures relevant to the manuscript. Go to Neurology.org/NN for full disclosures.
References
Articles from Neurology® Neuroimmunology & Neuroinflammation are provided here courtesy of American Academy of Neurology
Full text links
Read article at publisher's site: https://doi.org/10.1212/nxi.0000000000001070
Read article for free, from open access legal sources, via Unpaywall:
https://nn.neurology.org/content/nnn/8/6/e1070.full.pdf
Citations & impact
Impact metrics
Citations of article over time
Alternative metrics

Discover the attention surrounding your research
https://www.altmetric.com/details/112726507
Article citations
Interleukin-6 Signaling Blockade Induces Regulatory Plasmablasts in Neuromyelitis Optica Spectrum Disorder.
Neurol Neuroimmunol Neuroinflamm, 11(4):e200266, 18 Jun 2024
Cited by: 2 articles | PMID: 38889374 | PMCID: PMC11188987
CD11chigh B Cell Expansion Is Associated With Severity and Brain Atrophy in Neuromyelitis Optica.
Neurol Neuroimmunol Neuroinflamm, 11(2):e200206, 13 Feb 2024
Cited by: 1 article | PMID: 38350043 | PMCID: PMC11073873
Single-cell RNA sequencing reveals cell type-specific immune regulation associated with human neuromyelitis optica spectrum disorder.
Front Immunol, 15:1322125, 19 Feb 2024
Cited by: 0 articles | PMID: 38440735 | PMCID: PMC10909925
The landscape of PBMCs in AQP4-IgG seropositive NMOSD and MOGAD, assessed by high dimensional mass cytometry.
CNS Neurosci Ther, 30(2):e14608, 01 Feb 2024
Cited by: 1 article | PMID: 38334017 | PMCID: PMC10853888
Bruton's tyrosine kinase-bearing B cells and microglia in neuromyelitis optica spectrum disorder.
J Neuroinflammation, 20(1):309, 21 Dec 2023
Cited by: 4 articles | PMID: 38129902 | PMCID: PMC10740299
Go to all (13) article citations
Similar Articles
To arrive at the top five similar articles we use a word-weighted algorithm to compare words from the Title and Abstract of each citation.
Condition-dependent generation of aquaporin-4 antibodies from circulating B cells in neuromyelitis optica.
Brain, 141(4):1063-1074, 01 Apr 2018
Cited by: 58 articles | PMID: 29447335 | PMCID: PMC5889028
Early B cell tolerance defects in neuromyelitis optica favour anti-AQP4 autoantibody production.
Brain, 142(6):1598-1615, 01 Jun 2019
Cited by: 47 articles | PMID: 31056665 | PMCID: PMC6536857
Effects of Tocilizumab Therapy on Circulating B Cells and T Helper Cells in Patients With Neuromyelitis Optica Spectrum Disorder.
Front Immunol, 12:703931, 29 Jul 2021
Cited by: 6 articles | PMID: 34394101 | PMCID: PMC8360623
Individualized B cell-targeting therapy for neuromyelitis optica spectrum disorder.
Neurochem Int, 130:104347, 01 Dec 2018
Cited by: 14 articles | PMID: 30513364
Review