Abstract
Background
Colorectal cancer has a strong epigenetic component that is accompanied by frequent DNA methylation (DNAm) alterations in addition to heritable genetic risk. It is of interest to understand the interrelationship of germline genetics, DNAm, and colorectal cancer risk.Methods
We performed a genome-wide methylation quantitative trait locus (meQTL) analysis in 1,355 people, assessing the pairwise associations between genetic variants and lymphocytes methylation data. In addition, we used penalized regression with cis-genetic variants ± 1 Mb of methylation to identify genome-wide heritable DNAm. We evaluated the association of genetically predicted methylation with colorectal cancer risk based on genome-wide association studies (GWAS) of over 125,000 cases and controls using the multivariate sMiST as well as univariately via examination of marginal association with colorectal cancer risk.Results
Of the 142 known colorectal cancer GWAS loci, 47 were identified as meQTLs. We identified four novel colorectal cancer-associated loci (NID2, ATXN10, KLHDC10, and CEP41) that reside over 1 Mb outside of known colorectal cancer loci and 10 secondary signals within 1 Mb of known loci.Conclusions
Leveraging information of DNAm regulation into genetic association of colorectal cancer risk reveals novel pathways in colorectal cancer tumorigenesis. Our summary statistics-based framework sMiST provides a powerful approach by combining information from the effect through methylation and residual direct effects of the meQTLs on disease risk. Further validation and functional follow-up of these novel pathways are needed.Impact
Using genotype, DNAm, and GWAS, we identified four new colorectal cancer risk loci. We studied the landscape of genetic regulation of DNAm via single-SNP and multi-SNP meQTL analyses.Free full text

Genetic regulation of DNA methylation yields novel discoveries in GWAS of colorectal cancer
Abstract
Background:
Colorectal cancer (CRC) has a strong epigenetic component that is accompanied by frequent DNA methylation (DNAm) alterations in addition to heritable genetic risk. It is of interest to understand the interrelationship of germline genetics, DNAm, and CRC risk.
Methods:
We performed a genome-wide methylation quantitative trait locus (meQTL) analysis in 1355 people, assessing the pairwise associations between genetic variants and lymphocytes methylation data. In addition, we used penalized regression with cis-genetic variants +/− 1Mb of methylation to identify genome-wide heritable DNAm. We evaluated the association of genetically predicted methylation with CRC risk based on GWAS of over 125,000 cases and controls using the multivariate sMiST as well as univariately via examination of marginal association with CRC risk.
Results:
Of the 142 known CRC genome-wide association studies (GWAS) loci, 47 were identified as meQTLs. We identified 4 novel CRC associated loci (NID2, ATXN10, KLHDC10 and CEP41) that reside over 1Mb outside of known CRC loci and 10 secondary signals within 1Mb of known loci.
Conclusions:
Leveraging information of DNAm regulation into genetic association of CRC risk reveals novel pathways in CRC tumorigenesis. Our summary statistics-based framework sMiST provides a powerful approach by combining information from the effect through methylation and residual direct effects of the meQTLs on disease risk. Further validation and functional follow-up of these novel pathways are needed.
Impact:
Using genotype, DNA methylation, GWAS, we identified four new CRC risk loci. We studied the landscape of genetic regulation of DNA methylation via single-SNP and multi-SNP meQTL analyses.
Introduction
Large genome-wide association studies (GWAS) have identified 142 genetic variants associated with colorectal cancer (CRC) risk to date (1,2). While large GWAS have been essential for variant discoveries, novel information can be gained by combining GWAS with functional studies of genomic characteristics. Studying association of genetic variants with molecular features such as gene expression and DNA methylation (DNAm) may reveal underlying disease mechanisms and discover additional novel loci. With the availability of genomic studies, results from large-scale GWAS studies, and summary statistics-based methods, it is now feasible to study these relationships. In this paper, we examine the impact of genetic variants on DNAm and utilize this information to study the association of methylation quantitative trait loci (meQTL) with CRC risk.
DNAm refers to the addition of a methyl group to a cytosine phosphate guanine (CpG) site of a DNA strand. It plays a critical role in colorectal carcinogenesis (3–6) and is associated with survival time in CRC (7,8). In addition, a well-studied subtype of CRC is the CpG Island Methylator Phenotype (CIMP), which is defined by the degree of methylation at specific CpG islands in the tumor genome (9–11). Other measures of DNAm and epigenetic modifications have also been used as screening and early detection, such as ColoGuard (NDRG2 and BMP3) and Epi ProColon (SEPT9) (12).
The interrelationships between DNAm, germline genetics and complex phenotypes, have been examined previously. Freytag et al (13) developed a genetic predictive model for DNAm at each CpG site using elastic net penalization and assessed the association of predicted DNAm with complex phenotypes including schizophrenia, Alzheimer’s disease, and irritable bowel disease. Yang et al developed genetic predictive weights of DNAm within the Framingham Heart Study and used these weights to assess associations of predicted DNAm with epithelial ovarian (14) and breast cancers(15). However, to our knowledge, there has been no similar study relating genetically regulated methylation with CRC risk.
Many methods have been developed to evaluate how genetic variants that impact a genomic characteristic are associated with an outcome. Typically these methods assess the associations between SNPs and a genomic feature and then use the resulting effect sizes as weights to impute the genomic feature into a much larger genetic dataset for association with the outcome(16–19). This has been expanded to use only GWAS summary statistics, alleviating both computational and data accessibility burdens. These approaches serve as a vital tool in leveraging genomic information for novel discoveries. They are closely related to two-stage least square Mendelian randomization approaches, when the genetic variants affect the outcome only through the genomic feature. MiST (Mixed effects Score Test) (19,20) and the summary statistics-based sMiST(21) allow for SNP effects through not only the predicted genomics features like in Mendelian randomization but also alternative pathways by modeling the residual genetic effects. By this, MiST or sMiST tests the total effect of SNPs on the outcome.
In this paper, we evaluated the relationship between germline SNPs and DNAm based on the GWAS and methylation data of 1,355 study participants by both single-SNP and multi-SNP meQTLs analyses. We then assessed the association of meQTLs with CRC risk by utilizing summary statistics from over 125,000 CRC cases and controls.
Materials and Methods
Description of study with DNA methylation and GWAS data
The DNAm and GWAS data were from the Ontario Familial Colon Cancer Registry (22). Cases were identified from the Provincial Cancer Registry between 1997 and 2000 and controls were recruited randomly via household telephone interviews (22,23). Informed consent was obtained from participants. All study protocols were approved by the relevant Institutional Review Boards, and informed consent was obtained from study participants in accordance with the Helsinki Accord. After QC and filtering, a total of 1,355 cases and controls had both genome-wide genotyping and methylation data available. All participants were of European descent as confirmed by GWAS data. DNAm was measured in lymphocytes. Detailed information on DNAm pre-processing and QC are provided in Supplementary Methods. After QC, there were 298,248 CpG sites. The majority of cases and controls were plated separately, except for 267 study participants (115 cases and 152 controls).
Study participants were genotyped on the Affymetrix or the Human1M/1Mduo platforms (22,23) and imputed to the Haplotype Reference Consortium panel (1). Variants were excluded if the imputed minor allele frequency (MAF) was < 1%, missing rate > 10%, or imputation R2 < 0.3. In total, there were 7,672,848 imputed genetic variants, which were coded as imputed dosage ranging from 0 to 2.
GWAS summary statistics and linkage disequilibrium (LD) reference panel
The GWAS- summary statistics of over 125,000 colorectal cancer cases and controls were obtained from Huyghe et al(1), including data from GECCO (Genetic and Epidemiology Colorectal Cancer Consortium), CCFR (Colon Cancer Family Registry) and CORECT (Colorectal Transdisciplinary Study). A full list of studies that contribute to the summary statistics is given in Table S1 with more information published previously (1,24–26). We removed variants with MAF < 0.01. The LD reference panel is based on 29,303 individuals of European ancestry from GECCO (1,24).
Single-SNP MeQTL Analysis
We assessed the pairwise association of SNPs and CpGs to identify meQTLs. We undertook a two-stage discovery and replication design by splitting the participants genotyped on Affy Chip in the discovery (n = 856) and those genotyped on Human1M/1Mduo (n = 499) in the replication. We adjusted for sex, case-control status, sample plate, position on plate, age, two principal components (PC’s) for technical effects, and 6 PC’s from a subset of CpG sites to adjust for unobserved cell types. More details on the PC are provided in Supplement Methods. DNAm was logit transformed so that the range would not be restricted (27). The analysis was done using the matrixEQTL R package (28).
We classified SNP-CpG pairs into cis-meQTL when the SNP was within 1 Mb of the CpG site, and trans-meQTL otherwise. A total of 1,710,673,549 cis associations and 2,286,700,896,755 trans associations were tested. We adjusted for multiple testing using the Bonferroni correction with type I error 0.05 for cis- and trans-meQTL associations, separately. For discovery, we set the genome-wide significance threshold 0.05/1,710,673,549 ≈ 2.92e-11 for cis associations, and 0.05/2,286,700,896,755 ≈ 2.19e-14 for trans associations. For replication, the threshold was based on the number of cis (ncis) or trans (ntrans) that passed the discovery stage, i.e., 0.05/(ncis or ntrans). The direction of the association in the replication stage also needed to be the same as in the discovery stage. Top meQTL associations were defined as having the lowest p-value in the replication set.
We performed LD pruning on the meQTLs to account for the high SNP LD. For each CpG site, we selected the lead meQTL (based on the replication p-value) and removed any meQTL with LD R2 > 0.2 with the lead meQTL. We then moved to the next most significant remaining meQTL and repeated until no meQTLs left. For computational ease, we assumed no cross-chromosomal LD.
Association of single-SNP meQTL with CRC
We examined associations of meQTLs identified from the single-SNP meQTL analysis with CRC risk based on GWAS summary statistics (1). For each CpG site, we picked its lead meQTL with the largest test statistic and examined their associations with CRC risk. We adjusted for multiple testing using Bonferroni correction based on the number of SNPs being tested. An association was considered novel if there were no known CRC loci within 1MB. The known loci are those that reached the genome-wide significance level 5e-08 as previously reported (Table S2).
Multi-SNP meQTL analysis
To assess the joint effects of SNPs on DNAm, we fit a penalized regression model on each CpG site. We did not include trans SNPs due to the fact that the majority of meQTLs are cis and substantial computational burden if we include trans SNPs. To improve power, we combined both discovery and replication methylation data and adjusted for the genotyping platform in addition to the covariates in the single-SNP meQTL analysis. These covariates were not penalized. We fit four different elastic net models with α values of 0.25, 0.5, 0.75, and 1.0 (lasso), following previous literature that showed elastic net models tend to provide better prediction when some sparsity was induced (16,18,29,30). We performed ten-fold cross-validation and determined the optimal penalty λ value based on cross-validation MSE. We estimated the weights at the optimal penalty value using the entire dataset. Analyses were done using the glmnet package in R (31). We evaluated performance by the mean cross-validated partial R2 of DNAm variation explained by SNPs across 10 folds
Association of multi-SNP meQTLs with CRC
We tested the joint association of meQTLs identified from the penalized regression with CRC risk for each CpG site using sMiST (21). sMiST combines both the association of the genetically predicted DNAm at that CpG site using the weights obtained from the penalized regression and the association of residual effects of individual meQTLs (random effects) (21) (Supplementary Methods). We used Fisher’s combination procedure to combine the tests for these two components. We restricted our analysis to CpG sites with a mean cross-validated partial R2 above 1%. A p-value < 0.05/# of CpG sites tested was considered genome-wide statistically significant.
We performed conditional analysis of CpG sites that passed the genome-wide significance, adjusting for all known CRC GWAS loci. An association with p-value < 0.05/# of genome-wide significant CpG sites was considered novel if there were no known loci within 1 MB or as a novel secondary signal otherwise. As the participants with methylation data were part of GECCO, which contributed to the GWAS CRC summary statistics, we conducted a sensitivity analysis of significant associations by excluding the GECCO data.
Results
Table 1 provides descriptive statistics of the people in the methylation analysis dataset. There were 1,355 participants, with 856 genotyped using the Affy Chip assay and 499 using the Human1M/1Mduo genotyping array. Roughly 50% were men, 47.5% were CRC cases, and the mean age was 62 years old.
Table 1:
Descriptive statistics of the methylation study.
Affy Chips (n=856) | Human1M/1Mduo (n=499) | Overall (n=1355) | |
---|---|---|---|
Sex | |||
![]() | 454 (53.0%) | 225 (45.1%) | 679 (50.1%) |
![]() | 402 (47.0%) | 274 (54.9%) | 676 (49.9%) |
Case/Control | |||
![]() | 456 (53.3%) | 187 (37.5%) | 643 (47.5%) |
![]() | 400 (46.7%) | 312 (62.5%) | 712 (52.5%) |
Age reference | |||
![]() | 62.3 (7.62) | 60.3 (9.22) | 61.6 (8.30) |
![]() | 63.0 [29.0, 77.0] | 62.0 [27.0, 76.0] | 63.0 [27.0, 77.0] |
Landscape of MeQTL
A total of 5,228,463 cis SNP-DNAm associations passed the genome-wide threshold in the discovery stage and 3,444,113 were replicated. Of these 3,444,113 cis associations, there were 32,686 unique CpG sites and 1,280,770 unique SNPs, with many SNPs associated with multiple CpG sites. For trans-associations, a total of 559,695 SNP-DNAm associations passed the genome-wide threshold in the discovery stage and 292,919 trans-associations were replicated. Of these 292,919 associations, 160,752 were CpG-SNP pairs on the same chromosome but over 1 Mb apart. Across the 2,288,411,570,304 tests performed (every CpG-SNP pair) in the discovery, the median p-value was between 0.499 to 0.501 suggesting no overall inflation.
Of the 142 CRC known variants (Table S2), all but 2 variants (rs1391441 and rs11087784) were either meQTLs (n = 47) or within 1Mb of a meQTL (n=93). For the 47 known loci that are meQTLs, the majority (n=43) were associated with CpGs on the same chromosome (Table S3 and S4). SNP rs3131043 (6:30758466_A/G) was a meQTL for 28 CpG sites including 18 cis, 8 trans on the same chromosome, and 2 trans on the different chromosomes. Amongst the 94 that were not meQTLs but within 1Mb of meQTLs (one variant was not in our dataset), only three were in high LD with a meQTL (R2 above 0.7): rs7121958, rs12594720, and rs6983267.
We examined the number of CpGs associated with each SNP stratified by cis associations and trans associations that on the same and different chromosomes (Figure 1). Not surprisingly, there was a peak of cis-associations on chromosome 6 that map to the MHC region (Figure 2). In total, there were 584,770 unique CpG-SNP associations within 5Mb on either side of HLA-A (the peak), accounting for 15.6% of all CpG-SNP associations and 42.8% of all trans associations (459,341 cis, 97,930 trans on the same chromosome, and 27,499 trans on the different chromosomes). This high amount of trans on the same chromosome is likely due to the extensive LD in the MHC region.
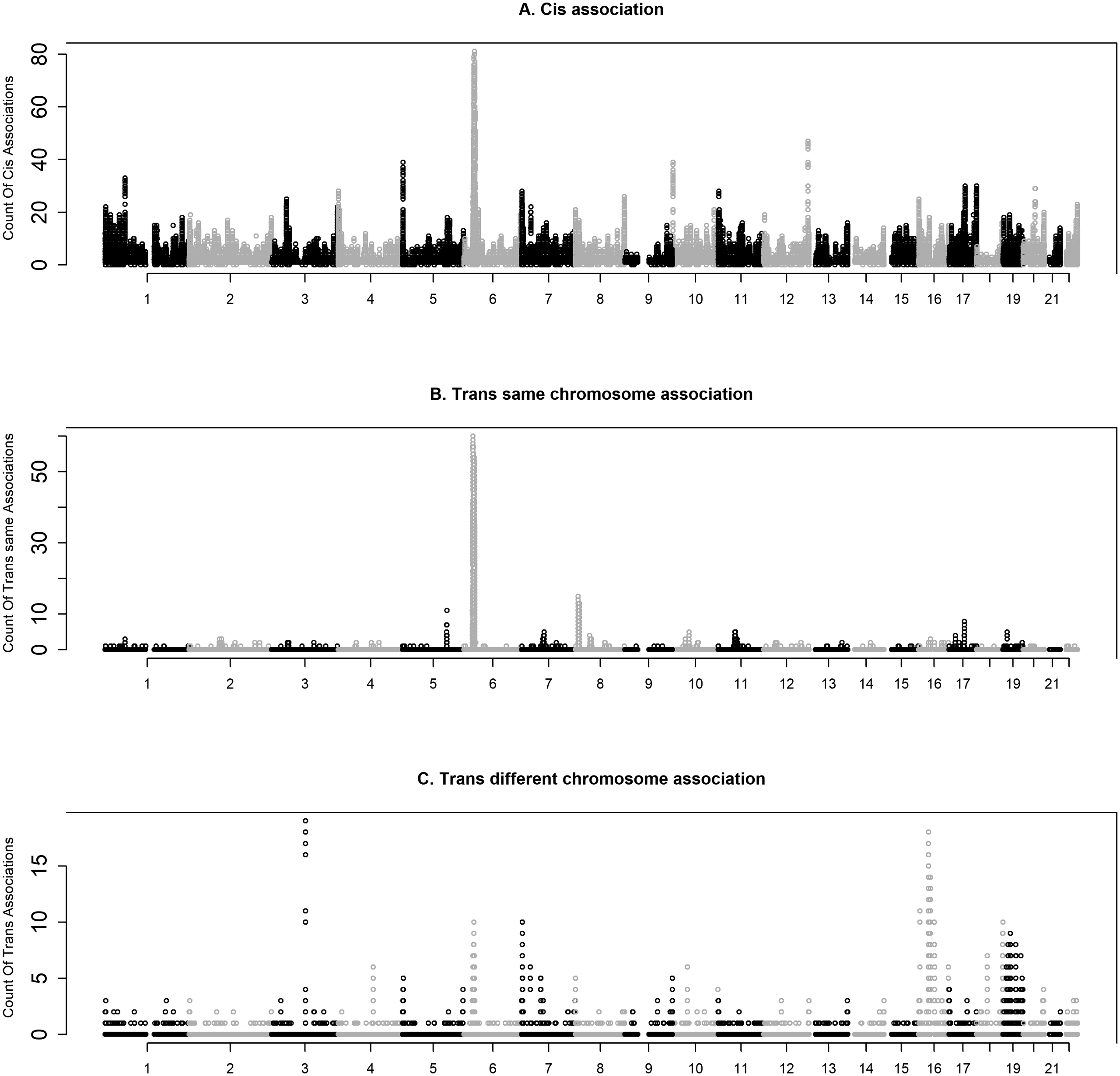
Modified Manhattan plot of frequencies of a SNP being meQTL for cis-association (top), trans on the same chromosome (middle), and trans on different chromosomes (bottom).
As many cis- and trans- associations are in LD, we performed LD pruning for each CpG site. This resulted in 70,106 associations with 67,620 cis associations, 529 trans on the same chromosome, and 1,957 trans on different chromosomes. Due to the uniqueness of the HLA region, the associations where CpGs or SNPs were within 5Mb of HLA-A on either side were excluded in tallying the number of cis and trans associations. The top cis association was between rs7083920 (10:45071890_C/T) and cg02113055 (10: 45072520) (replication p-value < 1e-100) which were 630 bp apart. The top trans on the same chromosome association was rs3764855 (17:66244578_T/C) and cg27123423 (≈ 3.27 Mb, replication p-value < 1e-100). The top trans association on different chromosomes was rs12222783 (11:108531761_T/C) and cg23247274 (MRP63/SKA3, 13:21750649)(replication p-value < 1e-100). These associations were in excellent agreement between the replication and discovery datasets (Figure 3). Figure 3 also displays the next three strongest associations in all three categories (list of associations in Figure 3 are in Table S5).
Novel association of single-SNP meQTLs with CRC
We assessed the association of single-SNP meQTLs with CRC risk. Across all CpG-SNP associations, there were 33,845 unique CpG sites. Taking the top meQTL for each of these CpG sites resulted in 27,287 unique SNPs associated with DNAm, of which 3,917 SNPs were the top meQTL for multiple CpGs. Using the CRC summary statistics, 70 SNPs passed the Bonferroni significance threshold 0.05/27,287 = 1.83e-06 (Table S6). Of these 70 SNPs, 68 were within 1Mb of 142 known GWAS variants. The two SNPs outside 1Mb were in high LD (R2 = 0.99) and they were associated with increased CRC risk (SNP rs2749870, 14:52493068_reference allele/effect allele [G/A], OR = 1.04, 95% CI = 1.03–1.06, p-value = 1.2e-06; SNP rs2516600, 14:52487766_T/C, OR=1.04, 95% CI = 1.03–1.06, p-value = 1.3e-06). Conditioning on known loci did not change the associations greatly (rs2749870 p-value = 1.37e-05, rs2516600 p-value = 1.37e-05).
SNP rs2749870 up-regulated methylation at cg26923084 (14: 52536893, replication effect = 0.39, 95% CI = 0.35,0.41), while SNP rs2516600 down-regulated methylation at cg20550154 (14: 52487779, replication effect = −0.71, 95% CI = −0.76,-0.69) with DNAm at these two CpG sites negatively correlated (Pearson correlation = −0.5) (Figure S1). We performed colocalization for the region from 52.4 to 52.5Mb using coloc (32). There was strong evidence of a common signal for CRC risk and methylation at cg20550154 (posterior probability = 0.96), but not at cg26923084 (posterior probability = 0.24).
Multi-SNP meQTLs and Predictive Weights
We built genetic prediction models for DNAm at each CpG site using cis SNPs. Of the 298,248 CpG sites, 179,087 CpG sites had at least one SNP selected by an elastic net models (178,390, 175,709, 174,647, and 174,015 for α = 0.25, 0.50, 0.75, and 1, respectively). All models had similar partial R2 (Figure S2), but lasso had the smallest model size with mean number (standard deviation [SD], range) of selected SNPs 15.5 (19.2,1–755). For the remaining analyses, we focused on lasso predictive weights, as it provided the most parsimonious models. A total of 170,340 CpG sites had a mean cross-validated partial R2 for predictive DNAm above 1% and 45,965 above 10%. Beyond that the numbers decreased rapidly (Figure 4), with only 4,422 having a partial R2 above 50%. The mean and median partial R2 were 9.8% and 5.4%, respectively. All genetic weights are available upon request from the authors.
Novel Multi-SNP meQTLs with CRC
We ran sMiST on 168,963 CpG sites with partial R2 ≥ 0.01, after removing 1,377 CpG sites where all selected SNPs had MAF < 1% in the GWAS summary statistics. A total of 853 CpG sites passed the significance threshold (0.05/168,963 ≈ 2.96e-07, Figure S3). After adjusting for the known loci, 13 CpG sites were still significant. Of these 13, 3 CpG sites cg10226744 (22: 46068542, ATXN10), cg05743278 (7:129709803, KLHDC10), and cg19037357 (7: 130081024, CEP41) had no CRC known loci within 1 Mb (Table 2). In our sensitivity analysis, removing GECCO from the GWAS summary statistics did not change the result (Table S7).
Table 2:
Multi-SNP meQTL significant associations with CRC risk based on sMiST after adjusting for known GWAS loci
CpG | Gene | NSNP1 | R22 | Unadj P-value3 | Cond P-value4 | Burden P-value5 | Random P-value6 | Nearest Known CRC SNP7 |
---|---|---|---|---|---|---|---|---|
cg18541609 (5:430359) | AHRR | 26 | 0.082 | 1.50E-07 | 2.50E-05 | 3.90E-03 | 4.40E-04 | rs78368589 |
cg23330450 (6:31548285) | 56 | 0.122 | 2.00E-17 | 3.30E-05 | 5.10E-04 | 4.70E-03 | rs2516420 | |
cg14426347 (6:31632827) | BAT4/CSNK2B | 20 | 0.042 | 7.60E-13 | 1.70E-05 | 3.60E-05 | 3.10E-02 | rs2516420 |
cg13653456 (6:31763819) | VARS | 20 | 0.074 | 3.80E-10 | 3.70E-06 | 4.70E-05 | 4.80E-03 | rs2516420 |
cg23945481 (6:31938984) | STK19/DOM3Z | 14 | 0.053 | 4.20E-11 | 5.50E-05 | 1.30E-02 | 3.00E-04 | rs3830041 |
cg22767321 (6:32143681) | AGPAT1 | 12 | 0.043 | 1.20E-08 | 2.40E-06 | 1.90E-06 | 7.60E-02 | rs3830041 |
cg07588430 (6:41561444) | FOXP4 | 4 | 0.014 | 5.80E-10 | 3.80E-08 | 2.20E-07 | 8.30E-03 | rs62396735 |
cg05743278 (7:129709803) | KLHDC10 | 5 | 0.031 | 1.40E-07 | 8.50E-07 | 3.30E-06 | 1.40E-02 | --- |
cg19037357 (7:130081024) | CEP41 | 17 | 0.067 | 1.60E-07 | 2.30E-06 | 6.10E-01 | 2.30E-07 | --- |
cg07005513 (11:61595956) | FADS2 | 10 | 0.076 | 3.70E-11 | 1.00E-06 | 5.50E-02 | 1.10E-06 | rs174533 |
cg18213653 (12:112605734) | C12orf51 | 7 | 0.016 | 2.10E-08 | 2.20E-09 | 2.40E-03 | 3.80E-08 | rs597808 |
cg19193595 (15:67396487) | SMAD3 | 5 | 0.022 | 4.40E-14 | 2.30E-05 | 6.50E-01 | 2.50E-06 | rs56324967 |
cg10226744 (22:46068542) | ATXN10 | 7 | 0.093 | 1.10E-07 | 2.80E-09 | 1.60E-04 | 7.30E-07 | --- |
Upon closer examination, for most CpG sites, both the genetically predicted CpG component and the random effects component contributed to the overall association, highlighting the power of sMiST for testing the total effects of meQTLs and the idea that the SNPs may contribute to CRC risk beyond the CpG site considered. The most significant conditional association was cg18213653 (C12orf51) on chromosome 12 (overall p-value = 2.23e-09) with burden (genetically predicted CpG) p-value = 2.42e-03 and random effects p-value = 3.82e-08. The association signal of the novel CpG site cg05743278 (KLHDC10) was mainly driven by the predicted methylation (p-value 3.3e-06). A total of 5 meQTLs in this CpG explained 3% of the variation. On the other hand, cg19037357, cg07005513, cg19193595 were mainly driven by the random effects, suggesting potential individual variant effects in addition to the effect of the predicted methylation effect. Interestingly, we did not observe much correlation amongst the individual DNAm of these 13 sites (Figure S4).
As a follow-up analysis, we examined the sMiST results of the two CpG sites from the meQTL CRC. The meQTL rs2516600 was selected as one of the 11 SNPs in the lasso regression, which together strongly predicted methylation of cg20550154 (partial R2 = 0.72). The sMiST p-value for this CpG site is 1.04e – 05 with the p-value for predicted methylation 6.9e-07 and the random effects p-value 0.99, suggesting the CRC association was likely driven by methylation. The top meQTL of the other novel CpG site, cg26923084, was also selected as one of cg26923084’s 28 SNPs by lasso. The sMiST for this CpG site had an overall p-value of 4.2e-07 with the p-value for predicted methylation 2.29e-06 and for random effects 0.01, indicating the association was also likely driven by methylation.
Discussion
Herein, we provided a systematic examination of the relationship between genetic variants and DNAm and their subsequent association with CRC risk. We used two complementary approaches: (1) examining pairwise association between SNPs and DNAm and then testing whether the significant meQTLs were associated with CRC risk; and (2) examining joint association of cis-variants with DNAm and using these weights to test the overall association of predicted methylation and residual variant effects with CRC risk via sMiST. The single-SNP meQTL approach revealed a novel CRC risk locus and that about one third of known loci were meQTLs. The multi-SNP approach revealed three novel loci, as well as several novel secondary signals of regions within 1Mb of known loci.
In our single-SNP meQTL approach, the two novel SNPs, rs2516600 and rs2749870, are in high LD and likely represent one signal. However, these two SNPs are not the most associated SNPs in the cis-region of CpG sites and rs1051069 is (Figure S5, CRC p-value = 6.59e-08). The CpG sites for which rs2516600 and rs2749870 are top meQTLs both are mapped to Nidogen 2 (NID2). Interestingly, these two CpG sites are located within two different regions of the NID2 gene, cg20550154 is within the gene body while cg26923084 is within 1500 bp of the transcription start site. NID2 may be involved in maintaining the structure of the basement membrane (33). The encoded cell-adhesion protein NID2 binds to laminins (33). Members of the laminin family, including LAMA5 and LAMC1, have been implicated in CRC risk by GWAS (34). The NID2 promotor is aberrantly methylated in human gastrointestinal cancers, including colon cancer, and methylation has been shown to silence gene expression (35). NID2 gene methylation is also robustly associated with bladder cancer and urine-based methylation biomarker panels containing NID2 have been proposed for the early detection of primary bladder cancer (36,37).
In our multi-SNP approach, we found an association with cg10226744, mapped to the body of the ATXN10 gene. The Ataxin 10 (ATXN10) gene is expressed in most tissues and identified as a downstream effector of the p53 and pRB tumour suppressor pathways involved in cellular senescence (38). Circumventing senescence has been proposed to represent an essential step in tumor progression (39). Four of the seven lasso selected variants for cg10226744 are within ATXN10, two near FAM118A, and one near WNT7B. WNT7B is a member of the WNT pathway, which plays a major role in colorectal cancer signaling (40).
Both CpG sites cg05743278 and cg19037357 from the multi-SNP analysis are mapped to the 7q32.2 loci. This is a known imprinted region, with associations with basal-cell carcinoma and type-2- diabetes (41). cg05743278 is within 1500bp of the transcription start site of KLHDC10 and cg19037357 is within 1500bp of the transcription start site of CEP41. One SNP (rs10277874) was selected for both CpG sites by lasso. KLHDC10 has been found to be a suppressor of PP5 (42). PP5 is an alias for TFPI2 which is a methylation biomarker for CRC (43,44). CEP41, though not yet implicated in CRC, has been found to express in gastrointestinal tissues (45). Besides KLHDC10 and CEP41, gene KLF14, where nearby SNPs were selected for cg05743278 and cg19037357, may also be a candidate, as it has been found to be associated with CRC (46).
The 70,106 unique CpG-SNP associations after LD pruning was made up of 55,209 unique SNPs. Of these 46,254 were associated with only one CpG. Of the remaining 8,955 that were associated with more than one CpG sites, 8,576 were only associated with cis CpG sites, making it difficult to ascertain whether the associations were hotspots or due to correlation amongst the nearby CpG sites. Amongst the remaining SNPs, 12 were meQTLs for at least five CpG sites on different chromosome. The SNP rs11673023 was also reported by Huan et al (2019) as being in hotspot 22 (H22) of their trans-meQTL hotspots (47). In addition, three variants were within the region of the previously reported hotspot 14 (47,48).
While we have found multiple potentially novel loci, there are several limitations to our study. First, to increase the sample size for determining joint associations of SNPs with DNAm, we used the entire methylation dataset to infer meQTLs. The weights from the penalized regression model are likely biased (49). Ideally, the weights would be re-estimated using an independent validation dataset. However, the power loss for identifying novel loci with CRC risk due to biased weights is likely minimal as a scale shift for genetically predicted methylation does not affect testing. Second, our participants are all of the European ancestry. It is well-recognized that many of these SNPs tag causal variants and due to LD and minor allele differences between populations the effects of these SNPs may not be generalizable to other populations. It is important to validate these results in other racial and ethnic populations. Third, the DNAm was collected in whole blood as opposed to the relevant colon and rectal tissues. However, it has been observed that cross-tissue meQTL effects are prevalent (50,51), which, to some extent, are similar to gene expression quantitative trait loci (52). We also observed that gene regulatory elements of immune cell types from blood are enriched for variants with colorectal cancer association (53). Interestingly, when we examined the gene expression of the three novel associations (NID2, KLHDC10, and CEP41) there was higher gene expression in the colon tissue compared to whole blood (Figure S6–S9). Regardless, future works for identifying meQTLs in the relevant colon and rectal tissues and their associations with colorectal cancer risk are needed.
In our meQTL analyses we adjusted for case-control status, which may introduce collider bias. We examined the association of novel CRC with methylation at cg20550154 and cg26923084 ignoring case-control status or restricting the analysis to just controls, the associations did not alter however (Table S8). Further, there may be differential meQTL effects between cases and controls. Towards this end, we assessed the 3,737,032 unique associations from our discovery for case/control-SNP interaction in a subsample of cases-controls where cases and controls were on the same plate. Despite diagnosis/treatment potentially impacting DNAm, only 615 associations had a significant interaction effect (.05/3,737,032), and none overlapped the 70 associations detected in the single-SNP meQTL CRC GWAS. The limited number of interaction effects identified is likely due to low power. We further performed separate cis-meQTL analysis for cases and controls separately. The p-values of SNP-DNAm associations were highly correlated between cases and controls, suggesting meQTLs are generally shared between cases and controls (Figure S10). Thus, taking a combined analysis of cases and controls will have the most power in identifying these shared meQTLs. There were only 979 SNP-DNAm (after LD pruning) that reached our previous discovery cutoff (alpha = 2.9e-11) in cases but showed no association in controls (p-value less than 2.9e-11 in cases but greater than 0.5 in controls), and 1,393 SNP-DNAm that showed associations in controls but not cases, 15 of 979 and 103 of 1,393 of which were associated in our combined analysis. None of the SNPs were known CRC loci. To maximize the utility of the data we studied common effects across cases and controls; studies with larger sample size of cases and controls will be needed to study potentially differential effects.
Our study has many strengths. These include large sample sizes for GWAS CRC and methylation; use of novel powerful statistical methods to account for the total effect of both genetically predicted methylation and individual variant effects via alternative pathways; and the first large-scale examination of methylation association with CRC risk.
In summary, we have used a novel framework for utilizing DNAm data to discover novel loci as well as secondary signals associated with CRC risk. The findings could elucidate novel pathways in CRC tumorigenesis. These findings, in particular, the novel loci NID2, ATXN10, KLHDC10 and CEP41, warrant follow-up in future functional studies. Finally, we applied the summary sMiST analysis, a powerful statistical method to combine both information from the effect through methylation and residual direct effects of the meQTLs. We hope this paper will motivate researchers to utilize functional genomic data such as DNA methylation or gene expression and large-scale GWAS summary statistics with these statistical tools to discover new genetic associations.
Acknowledgments
The authors thank the participants across all studies. In addition, we thank the reviewers for valuable feedback. This project was a part of Richard Barfield’s post-doctoral work at Fred Hutch. L. Hsu was partially funded by the NIH/NCI Grant R01 CA189532. Genetics and Epidemiology of Colorectal Cancer Consortium (GECCO): National Cancer Institute, National Institutes of Health, U.S. Department of Health and Human Services (U01 CA164930, U01 CA137088, R01 CA059045, R01 CA201407) (U. Peters). Analyses were run on the Fred Hutch cluster, which is part of the Scientific Computing Infrastructure funded by ORIP grant S10OD028685. A full list of study-specific acknowledgments is provided in the supplement.
Conflict of Interest Disclosure Statement:
Marios Giannakis receives research funds from Merck, Bristol-Myers Squibb, Servier, and Janssen unrelated to this research. Other authors have declared that no competing interests exist.
References
Full text links
Read article at publisher's site: https://doi.org/10.1158/1055-9965.epi-21-0724
Read article for free, from open access legal sources, via Unpaywall:
https://aacrjournals.org/cebp/article-pdf/31/5/1068/3119503/1068.pdf
Citations & impact
Impact metrics
Alternative metrics

Discover the attention surrounding your research
https://www.altmetric.com/details/124544577
Smart citations by scite.ai
Explore citation contexts and check if this article has been
supported or disputed.
https://scite.ai/reports/10.1158/1055-9965.epi-21-0724
Article citations
Novel DNA methylation-based epigenetic signatures in colorectal cancer from peripheral blood leukocytes.
Am J Cancer Res, 14(5):2253-2271, 15 May 2024
Cited by: 0 articles | PMID: 38859857
Translation of nutrigenomic research for personalised and precision nutrition for cancer prevention and for cancer survivors.
Redox Biol, 62:102710, 22 Apr 2023
Cited by: 5 articles | PMID: 37105011 | PMCID: PMC10165138
Data
Data behind the article
This data has been text mined from the article, or deposited into data resources.
SNPs (Showing 20 of 20)
- (4 citations) dbSNP - rs2516600
- (4 citations) dbSNP - rs2749870
- (3 citations) dbSNP - rs2516420
- (2 citations) dbSNP - rs3830041
- (1 citation) dbSNP - rs56324967
- (1 citation) dbSNP - rs3764855
- (1 citation) dbSNP - rs11673023
- (1 citation) dbSNP - rs1391441
- (1 citation) dbSNP - rs174533
- (1 citation) dbSNP - rs10277874
- (1 citation) dbSNP - rs78368589
- (1 citation) dbSNP - rs12222783
- (1 citation) dbSNP - rs12594720
- (1 citation) dbSNP - rs62396735
- (1 citation) dbSNP - rs6983267
- (1 citation) dbSNP - rs7121958
- (1 citation) dbSNP - rs3131043
- (1 citation) dbSNP - rs11087784
- (1 citation) dbSNP - rs597808
- (1 citation) dbSNP - rs1051069
Show less
Similar Articles
To arrive at the top five similar articles we use a word-weighted algorithm to compare words from the Title and Abstract of each citation.
A general framework for functionally informed set-based analysis: Application to a large-scale colorectal cancer study.
PLoS Genet, 16(8):e1008947, 24 Aug 2020
Cited by: 5 articles | PMID: 32833970 | PMCID: PMC7470748
meQTL and ncRNA functional analyses of 102 GWAS-SNPs associated with depression implicate HACE1 and SHANK2 genes.
Clin Epigenetics, 12(1):99, 02 Jul 2020
Cited by: 13 articles | PMID: 32616021 | PMCID: PMC7333393
The Impact of Inherited Genetic Variation on DNA Methylation in Prostate Cancer and Benign Tissues of African American and European American Men.
Cancer Epidemiol Biomarkers Prev, 33(4):557-566, 01 Apr 2024
Cited by: 0 articles | PMID: 38294689 | PMCID: PMC10990789
Genetic impacts on DNA methylation: research findings and future perspectives.
Genome Biol, 22(1):127, 30 Apr 2021
Cited by: 87 articles | PMID: 33931130 | PMCID: PMC8086086
Review Free full text in Europe PMC
Funding
Funders who supported this work.
NCI NIH HHS (7)
Grant ID: R01 CA195789
Grant ID: U01 CA164930
Grant ID: U01 CA182883
Grant ID: U01 CA137088
Grant ID: R01 CA059045
Grant ID: R01 CA189532
Grant ID: R01 CA201407
NCI NIH U.S. Department of Health and Human Services (4)
Grant ID: U01 CA137088
Grant ID: U01 CA164930
Grant ID: R01 CA201407
Grant ID: R01 CA059045
NIH HHS (1)
Grant ID: S10 OD028685
NIH NCI (1)
Grant ID: R01 CA189532
ORIP (1)
Grant ID: S10OD028685
World Health Organization (1)
WHO generic grant number for open-access policy
World Organization
Grant ID: 001