Abstract
Introduction
The role of procurement biopsies in deceased donor kidney evaluation is debated in light of uncertainty about the influence of biopsy findings on recipient outcomes. The literature is filled with conflicting and ambiguous findings typically derived from small studies focused on short-term outcomes or reliant on biopsies prepared by methods impractical in the time-sensitive context of organ procurement.Methods
After manual data entry of DonorNet attachments from 4480 extended criteria donors (ECDs) recovered in the United States from 2008 to 2012, we applied causal inference methods in a Cox regression framework to estimate independent effects of glomerulosclerosis (GS), interstitial fibrosis, and vascular changes on long-term kidney graft survival. Kidney discard rates from 2018 to 2019 were evaluated to characterize contemporary kidney utilization patterns.Results
Effects of interstitial fibrosis and vascular changes were largely attenuated after adjusting for potentially confounding donor and recipient variables, although conclusions are less certain for severe levels due to smaller sample sizes. By contrast, significant effects of GS (>10% vs. 0%-5%) persisted even after adjustment (all-cause, hazard ratio [HR] 1.18; 95% CI 1.06, 1.28; death-censored, HR 1.28; 95% CI 1.08, 1.46) but plateaued beyond 10%. By contrast, kidney discard rates increased precipitously as GS rose >10%.Conclusion
Despite being obtained under less than ideal conditions, estimated GS from a procurement biopsy is independently associated with long-term graft survival, above and beyond standard clinical parameters, in ECD transplants. However, the disproportionately high likelihood of discard for kidneys with GS >10% is unjustified. The outsized effect of GS on kidney utilization should be tempered and commensurate with its effect on outcomes.Free full text

The Independent Effects of Procurement Biopsy Findings on 10-Year Outcomes of Extended Criteria Donor Kidney Transplants
Abstract
Introduction
The role of procurement biopsies in deceased donor kidney evaluation is debated in light of uncertainty about the influence of biopsy findings on recipient outcomes. The literature is filled with conflicting and ambiguous findings typically derived from small studies focused on short-term outcomes or reliant on biopsies prepared by methods impractical in the time-sensitive context of organ procurement.
Methods
After manual data entry of DonorNet attachments from 4480 extended criteria donors (ECDs) recovered in the United States from 2008 to 2012, we applied causal inference methods in a Cox regression framework to estimate independent effects of glomerulosclerosis (GS), interstitial fibrosis, and vascular changes on long-term kidney graft survival. Kidney discard rates from 2018 to 2019 were evaluated to characterize contemporary kidney utilization patterns.
Results
Effects of interstitial fibrosis and vascular changes were largely attenuated after adjusting for potentially confounding donor and recipient variables, although conclusions are less certain for severe levels due to smaller sample sizes. By contrast, significant effects of GS (>10% vs. 0%–5%) persisted even after adjustment (all-cause, hazard ratio [HR] 1.18; 95% CI 1.06, 1.28; death-censored, HR 1.28; 95% CI 1.08, 1.46) but plateaued beyond 10%. By contrast, kidney discard rates increased precipitously as GS rose >10%.
Conclusion
Despite being obtained under less than ideal conditions, estimated GS from a procurement biopsy is independently associated with long-term graft survival, above and beyond standard clinical parameters, in ECD transplants. However, the disproportionately high likelihood of discard for kidneys with GS >10% is unjustified. The outsized effect of GS on kidney utilization should be tempered and commensurate with its effect on outcomes.
The international kidney transplant community continues to debate the value and consequences of obtaining procurement biopsies for evaluating the transplant quality of donated kidneys.1,2 The reliability of biopsy data obtained during the time-pressured environment of deceased donor organ procurement has been challenged on several fronts: use of frozen sections,3,4 unclear optimal sampling technique (e.g., needle vs. wedge),5, 6, 7, 8 varying sample quality,9,10 interpretation by nonexperts,11, 12, 13 poor reproducibility,3 and low interrater agreement.14
Yet, despite being relied on minimally elsewhere,15,16 procurement biopsies continue to be performed routinely in the United States. More than half of kidneys recovered for transplant are biopsied,17 a figure that rose during the 2000s as the donor pool broadened18 but has plateaued.19 Procurement biopsy findings continue to be associated with the decline and discard of kidneys offered for transplant,9,17,19, 20, 21, 22, 23 whereas some patients die waiting for a transplant.24
Given their limitations, should procurement biopsies play any role in determining the transplant suitability of kidneys?
Whether biopsy findings have a clinically meaningful, independent association with transplant recipient outcomes beyond more easily obtained clinical parameters remains elusive, as the literature is filled with conflicting findings.3,25, 26, 27, 28, 29, 30, 31, 32, 33, 34, 35, 36, 37 Conclusions are often drawn from small, single-center studies. Statistical interpretation is often over-reliant on arbitrary P value thresholds, instead of a more nuanced approach mindful of type II errors.38
Research has often focused on short-term, rather than longer-term, post-transplant survival. Some studies are based on biopsy samples prepared using methods that are impractical in the context of deceased donor procurement.4,29 The scope of many studies is limited to GS without consideration of the other compartments. Moreover, GS tends to be evaluated solely in arbitrary, discrete categories (0%–5%, 6%–10%, etc.) instead of along its biological continuum, sacrificing statistical power and precluding precise characterization of potential nonlinear effects.
The BARETO (Biopsy, Anatomy, and Resistance Effects on Transplant Outcomes) study aims to overcome these shortcomings and reliably estimate the independent effects of procurement biopsy findings on long-term graft survival. The conventional approach of using multivariable regression to estimate the independent (i.e., “adjusted”) effects of an exposure relies on strong assumptions: not only that all potential confounders are included but also that their true (possibly nonlinear) functional relationships with the outcome are adequately specified. Commonplace causal inference methods, such as those involving propensity scores,39 avoid this challenge but come with their own assumptions and limitations. A newer approach to causal inference, doubly robust regression (DRR), combines multivariable regression and propensity scores weighting to provide valid inference on an exposure variable if either the multivariable model or the propensity model are properly specified, offering a hedge against producing misleading results.40
By applying doubly robust Cox regression to a large, novel data set of routinely biopsied kidneys, this study seeks to estimate the degree to which the 3 central biopsy compartments (GS, interstitial fibrosis, and vascular changes) are independently associated with long-term outcomes above and beyond standard clinical factors.
Methods
This study used data from the Organ Procurement and Transplantation Network (OPTN). The OPTN data system includes data on all donors, waitlisted candidates, and transplant recipients in the United States, submitted by the members of the OPTN.41,42 The Health Resources and Services Administration, US Department of Health and Human Services, provides oversight to the activities of the OPTN contractor. Data, including DonorNet attachments, were released to United Network for Organ Sharing by the OPTN subsequent to Institutional Review Board approval from Virginia Commonwealth University Ethics Board. DonorNet is the online application that organ procurement organizations use to send electronic organ offers to transplant hospitals.43
This was a cohort study after transplant recipients for up to 10 years. A total of 8126 ECDs44 were recovered during 2008 to 2012, but not all these kidneys were biopsied or transplanted. DonorNet attachments were manually reviewed and biopsy findings entered into a REDCap45 database according to a protocol (Supplementary Figure S1) aligned with Banff definitions6 for 4480 ECD donors recovered during 2008 to 2012 with at least 1 kidney reported as having been biopsied and transplanted. Of these, 3851 (86.0%) had at least 1 kidney transplanted and a corresponding biopsy attachment found. Among these transplanted donors, 2870 (74.5%) had both kidneys transplanted, whereas 981 (25.5%) had just 1 kidney transplanted. ECD donors, which we found to be almost always biopsied (93.2%) during this period, were chosen to avoid selection bias resulting from the inclusion of for-cause biopsies.6 If nonroutinely biopsied kidneys were included, the clinical indications (e.g., visual defects) leading to the decision to biopsy could introduce bias through unmeasured confounding (Figure 1: Consolidated Standards of Reporting Trials diagram46).
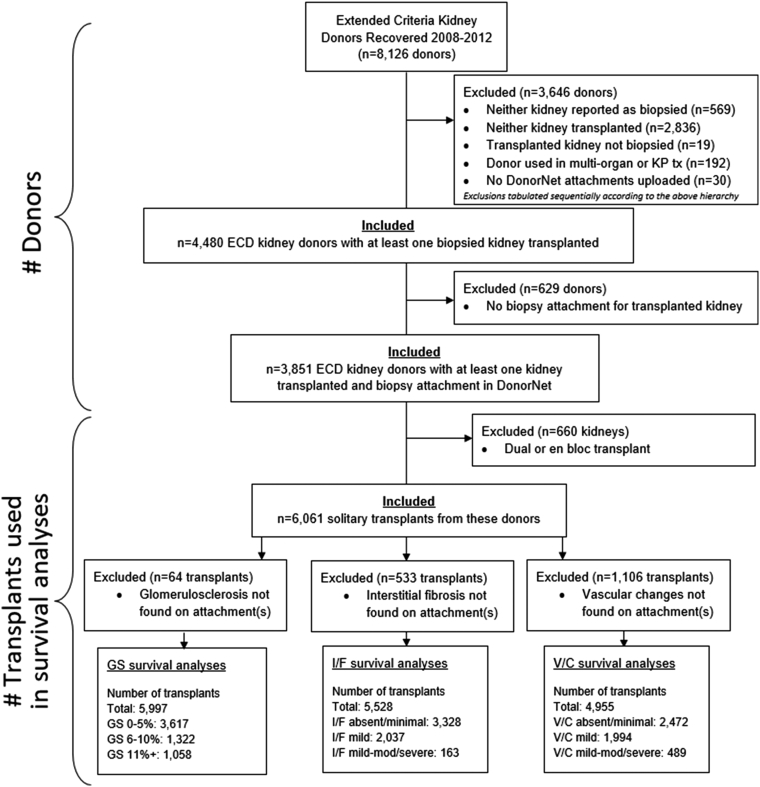
BARETO study survival analysis cohort derivation CONSORT diagram. Cohort selection flow diagram for biopsy, anatomy, and resistance effects on transplant outcomes observational study using the CONSORT format. #, number; CONSORT, Consolidated Standards of Reporting Trials; ECD, extended criteria donor; GS, glomerulosclerosis; I/F, interstitial fibrosis; KP, kidney-pancreas; tx, transplant; V/C, vascular changes.
The 3 biopsy dimensions reported with highest reporting frequency on attachments and studied as exposure variables were GS (99% reported), interstitial fibrosis (91%), and chronic vascular changes (aka, arterial intimal thickening, or arteriosclerosis, or vascular narrowing) (82%). Because it is unknown which of multiple biopsy reports was used for decision-making, for kidneys with multiple biopsy attachments (9.1%), we chose the attachment with the fewest missing or unknown data elements among the exposure variables. Sample preparation method was reported 51% of the time: 94% were frozen sections, 6% permanent/fixed. Sample type was reported 44% of the time: 67% were wedge, 33% core/needle.
The primary outcome was all-cause graft failure up to 10 years post-transplant. Death-censored graft failure was also analyzed. Outcomes were censored as of the earlier of the last reported patient follow-up or at 10 years post-transplant. Among nonfailed grafts, the median follow-up was approximately 8.5 years, whereas the “reverse Kaplan-Meier” median time-to-censoring estimates ranged from 9.0 to 9.8 years, indicating negligible loss to follow-up47,48 (Table 1).
Table 1
Sample sizes, graft outcomes, and length of follow-up
Exposure | N | Graft outcomes | Length of follow-up (yr) | ||||||||||||
---|---|---|---|---|---|---|---|---|---|---|---|---|---|---|---|
All-cause graft failures | Recipient deaths | Graft failures without recipient death | Deaths with functioning graft | Median (failures, all cause) | Median (all cases) | Median (nonfailures) | Median (“reverse KM”) | Max | |||||||
Count | % | Count | % | Count | % | Count | % | Count | % | ||||||
Glomerulosclerosis | |||||||||||||||
0%–5% | 3617 | 60.3 | 2038 | 58.0 | 1597 | 57.9 | 441 | 58.3 | 974 | 56.6 | 4.1 | 6.2 | 8.8 | 9.1 | 10 |
6%–10% | 1322 | 22.0 | 781 | 22.2 | 606 | 22.0 | 175 | 23.1 | 390 | 22.7 | 3.8 | 5.7 | 8.5 | 9 | 10 |
11%–15% | 592 | 9.9 | 398 | 11.3 | 317 | 11.5 | 81 | 10.7 | 200 | 11.6 | 3.8 | 5.3 | 8.4 | 9.8 | 10 |
16%–20% | 247 | 4.1 | 160 | 4.6 | 130 | 4.7 | 30 | 4.0 | 91 | 5.3 | 3.8 | 5.3 | 8.5 | 9.2 | 10 |
21%–30% | 153 | 2.6 | 100 | 2.8 | 78 | 2.8 | 22 | 2.9 | 52 | 3.0 | 3.9 | 5.4 | 8.4 | 9.1 | 10 |
31%+ | 66 | 1.1 | 38 | 1.1 | 30 | 1.1 | 8 | 1.1 | 13 | 0.8 | 3.9 | 6.5 | 8.9 | 9.2 | 10 |
Interstitial fibrosis | |||||||||||||||
Absent/minimal | 3328 | 60.2 | 1902 | 58.7 | 1472 | 58.3 | 430 | 60.1 | 955 | 59.9 | 3.9 | 6 | 8.7 | 9 | 10 |
Mild | 2037 | 36.8 | 1231 | 38.0 | 972 | 38.5 | 259 | 36.2 | 588 | 36.9 | 4 | 6 | 8.9 | 9.4 | 10 |
Mild-moderate | 161 | 2.9 | 105 | 3.2 | 79 | 3.1 | 26 | 3.6 | 52 | 3.3 | 4 | 5.5 | 8.1 | 9.6 | 10 |
Severe | 2 | 0.0 | 0 | 0.0 | 0 | 0.0 | 0 | 0.0 | 0 | 0.0 | NA | 6.9 | 6.9 | NA | 10 |
Vascular changes | |||||||||||||||
Absent/minimal | 2472 | 49.9 | 1430 | 48.8 | 1103 | 48.5 | 327 | 50.0 | 690 | 48.2 | 4.1 | 6.2 | 8.8 | 9 | 10 |
Mild | 1994 | 40.2 | 1188 | 40.6 | 936 | 41.2 | 252 | 38.5 | 577 | 40.3 | 3.7 | 5.6 | 8.7 | 9.1 | 10 |
Mild-moderate | 466 | 9.4 | 293 | 10.0 | 219 | 9.6 | 74 | 11.3 | 157 | 11.0 | 4.3 | 6.1 | 8.8 | 9.8 | 10 |
Severe | 23 | 0.5 | 17 | 0.6 | 16 | 0.7 | 1 | 0.2 | 9 | 0.6 | 3.8 | 4.4 | 6.8 | NA | 10 |
KM, Kaplan-Meier; Max, maximum; NA, not available.
Sample sizes (number of transplants) for each exposure variable level along with recipient follow-up time distribution statistics and graft failure type counts. The median follow-up time, excluding graft failures, was between 8 and 9 years, with maximum follow-up of 10 years due to administrative censoring. The primary study outcome was all-cause graft failures. For death-censored analyses, deaths with a functioning graft were censored.
Reverse KM = reverse Kaplan-Meier estimate measuring the median censoring time. This is the preferred approach to quantifying length of follow-up in survival analyses.48
In addition to Kaplan-Meier analysis, Cox multivariable regression and causal inference methods were used to serve the study’s central aim of characterizing the independent associations between the 3 exposure variables and long-term graft survival. Our primary findings were derived using DRR,40 which combines the strengths of propensity score-based inverse probability weighting49 and multiple regression to adjust for measured confounders. Propensity score methods involve building models that predict the likelihood of a case belonging to a particular exposure group (e.g., GS 0%–5% vs. 6%–10% vs. 11%+). These scores are then used in one of several ways, including 1:1 matching, that is, selecting cases with similar propensity scores in different treatment groups, which generally leads to similar distributions of patient characteristics across groups, allowing for fair/unbiased comparisons. Alternatively, cases can be “weighted” in the analysis by the inverse probability of being in a particular exposure group, with the same aim—covariate balance across exposure groups in the weighted sample.39 DRR weights were based on covariate balancing propensity scores.50 HRs derived from unadjusted, inverse probability weighting, and multiple regression analyses are provided for comparison. Following Stensrud and Hernan,51 we interpret the HR estimates as reflecting the weighted average of the true HRs during the 10 years after transplant. Evidence values (i.e., “E-values”) were computed to quantify the degree to which unmeasured confounding would need to be present to nullify estimated effects.52, 53, 54
Statistical inference was derived by bootstrapping (1000 iterations55) the entire DRR process, including single imputation of missing data using the MICE algorithm56,57 (Supplementary Figure S2), and calculating percentile-based 95% CIs.58 Supplementary Tables S1, S2, and S3 illustrate the degree of missingness for each covariate. GS was modeled categorically (levels were chosen to be consistent with OPTN data collection forms) and continuously, using a restricted cubic spline59 to capture nonlinearity. Pointwise CIs were generated at integer GS values (0%, 1%, 2%, …, 30%).
Potentially confounding covariates were chosen by clinical hypothesis generation, published literature, exposure variable versus covariate correlation analysis, and a philosophy of erring on the side of inclusion while leveraging opportunities for parsimony (e.g., omitting some variables already included in the Kidney Donor Profile Index [KDPI]60 or estimated post-transplant survival score61). Twenty donor, recipient, and kidney-related covariates were included in modeling (Supplementary Tables S1, S2, and S3). Other recipient factors considered but ultimately omitted due to statistically insignificant correlation with biopsy parameters included gender, education, diagnosis, body mass index, race/ethnicity, insurance type, albumin, and HLA matching. Potential collinearities among covariates were not of concern because our aim was to conduct causal inference on exposure variables (GS, interstitial fibrosis, vascular changes), not produce an explanatory, multivariable model.
The number of glomeruli observed varied from 1 to 474. To account for the reduced statistical precision10 in a GS value based on a small number of glomeruli, as a sensitivity analysis, we used empirical Bayes estimation—also known as best linear unbiased prediction or “shrinkage” estimation.62 Shrunken estimates were obtained by modeling GS as a binomial proportion and estimating the random kidney effects. Conceptually, these shrunken estimates reflect a weighted average between the observed GS for a particularly kidney and the overall sample mean GS of 5.95%.
Supplementary Figure S3 illustrates the relationship between the nominal and shrunken GS values. The greater the number of glomeruli observed, the less shrinkage toward the overall mean. For example, the nominal GS of 53% (55 of 104) found in purple only shrunk to 49% due to the large denominator; by comparison, the nominal GS of 60% (3 of 5) found in red shrunk dramatically to 19%. Empirical Bayes estimators have been found to yield statistically better estimates in numerous contexts.63,64
Spline modeling was repeated using shrunken estimates. For 20 (0.3%) kidneys, the observed GS was used because shrinkage estimation was not possible due to unreported number of glomeruli observed.
Left versus right laterality concordance was assessed using correlation analysis for GS and the Kappa statistic for interstitial fibrosis and vascular changes. Histology was examined by laterality for transplanted kidneys in which the mate kidney was discarded.
Contemporary kidney utilization practice was characterized by calculating discard rates—the proportion of kidneys recovered for transplant but not transplanted—for biopsied, ECD kidneys recovered in 2018 to 2019.
We used R Software Version 4.1.0,65 including most notably the following analytical packages: WeightIt,66 cobalt,67 covariate balancing propensity scores,68 mice,69 survival,70 rms,71 prodlim,72 and lme4.73
Results
Unadjusted, Kaplan-Meier graft survival was statistically lower (P < 0.0001) for higher GS in a dose-response relationship (Figure 2: all-cause; Supplementary Figure S4: death-censored). GS 0% to 5% was associated with 10-year graft survival of 34.2% (95% CI: 32.3%, 36.0%), compared with 24.4% (21.3%, 27.6%) for GS 11% or higher. Survival curves were also statistically different (P < 0.0001) when analyzing GS in 5 levels, but the dose-response pattern deteriorated above 10% (Supplementary Figure S5).
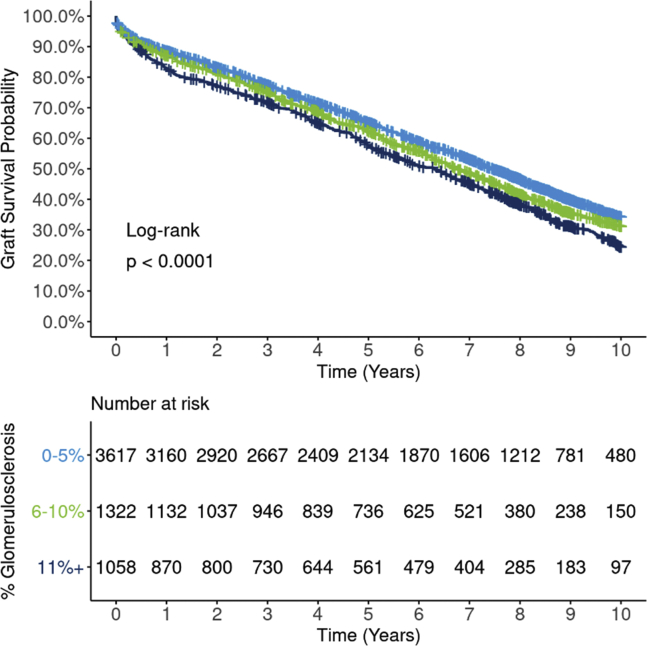
Ten-year, Kaplan-Meier all-cause graft survival by GS (3 levels: 0%–5%, 6%–10%, 11%+). Estimated graft survival rates for 5997 extended criteria donor kidney transplants occurring between 2008 and 2012 are illustrated by 3 levels of GSs. Survival curves are statistically different between the 3 groups (P < 0.0001), with the GS 0% to 5% group having superior unadjusted graft survival compared with 6% to 10% and 11%+ groups. The survival curves suggest a dose-response relationship, where graft survival declines as GS increases. GS, glomerulosclerosis.
Several notable associations were found between GS and potentially confounding factors—KDPI (P < 0.0001), donor hypertension (P = 0.0086), donor diabetes (P < 0.0001), interstitial fibrosis (P < 0.0001), vascular changes (P < 0.0001), arterial plaque (P = 0.0007), and recipient estimated post-transplant survival (P < 0.0001) (Supplementary Table S1). Though the association between GS and KDPI is statistically significant, the correlation is weak (rho = 0.12, Supplementary Figure S6).
The 10-year, unadjusted graft failure HR for GS 11%+ versus 0% to 5% of 1.29 (95% CI: 1.18, 1.40) was only partially attenuated after adjusting for 20 potential confounders: DRR-adjusted HR 1.18 (1.06, 1.28). Adjusted results were remarkably similar (identical out to 2 decimal places) using propensity weighting (HR 1.18; 1.07, 1.28) and multivariable regression (HR 1.18; 1.07, 1.28), suggesting robustness of these findings (Figure 3). The adjusted hazard of death-censored graft failure was also higher for GS 11%+ versus 0% to 5% (DRR HR 1.28; 1.08, 1.46). E-values of 1.49 and 1.66 were obtained for the 1.18 all-cause and 1.28 death-censored HR estimates, respectively.
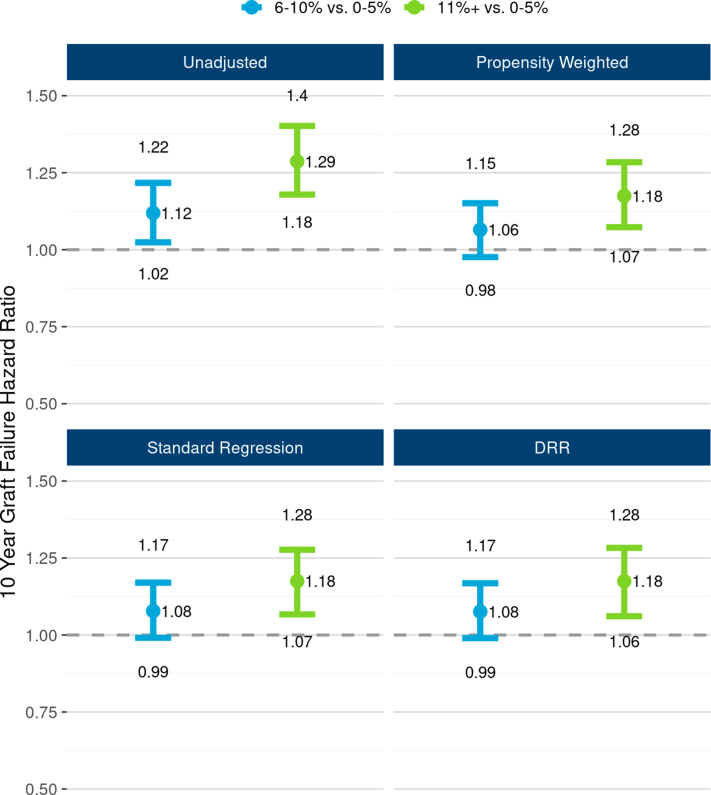
The unadjusted and adjusted associations between 3-level GS and 10-year, all-cause graft failure risk. The figure illustrates all-cause graft failure hazard ratios and 95% CIs comparing 3 levels of GS using the following 4 different analyses: unadjusted Cox regression (top left panel), propensity weighted Cox regression (top right), standard multivariable regression (bottom left), and doubly robust regression (DRR; bottom right). In unadjusted analysis, the risk of graft failure was 29% higher with GS 11%+ compared with the reference group, GS 0% to 5%. All 3 risk adjusted methods reveal that though GS effect was tempered after adjusting for correlations with donor and recipient factors, a statistically and clinical significant effect of GS persisted. In DRR analysis, the graft failure hazard ratio for GS 11%+ (vs. 0%–5%) was 1.18 (1.07, 1.28). DRR, doubly robust regression; GS, glomerulosclerosis.
When modeling continuous GS, a sharp, statistically significant increasing hazard was observed when GS rose from 0% to approximately 10%, but the effect plateaued for GS values beyond 10%. This pattern manifested in both unadjusted and adjusted results (Figure 4) and in 5-level categorical analysis (Supplementary Figure S7). Though propensity-weighted and DRR-based results suggest, prima facie, a counterintuitive decline in graft failure risk as GS increases beyond 10%, statistical inference reveals that this surprising improvement in outcomes does not reach statistical significance: the CI for the graft failure hazard beyond GS of 20% is wide and overlaps substantially with the estimated hazard at GS of 10%. Given the a priori clinical assumption that more GS, all else equal, does not portend better outcomes, these results should be not be interpreted to suggest that graft failure risk improves with rising GS but rather that the strength of the relationship substantially tapers beyond approximately 10% compared with the steep slope observed <10%.
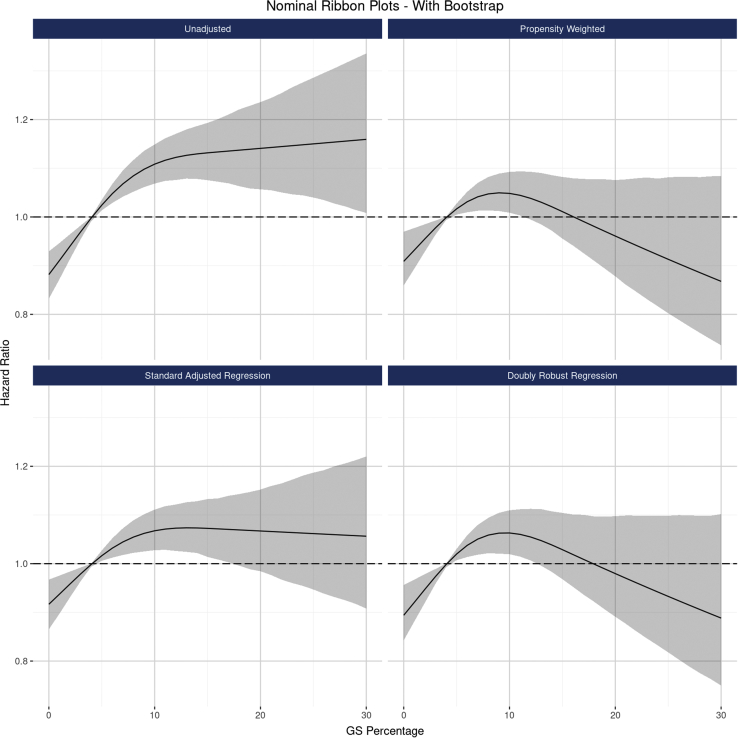
The associations between continuous GS and 10-year, all-cause graft failure risk, modeled as a nonlinear function. The figure illustrates the estimated relationship between GS along a continuum from 0% to 30%, as modeled by nonlinear splines using the following 4 analytical approaches: unadjusted Cox regression (top left panel), propensity weighted Cox regression (top right), standard multivariable regression (bottom left), and doubly robust regression (DRR; bottom right). Though all 3 risk adjusted methods revealed that the GS effect was somewhat attenuated after adjusting for correlations with donor and recipient factors, a statistically and clinical significant effect of GS persisted. The relationship between GS and graft failure risk is clearly nonlinear, with a steep effect between 0% and approximately 10%, followed by a plateauing effect beyond 10%. CIs (found in gray) indicate that the apparent declining risk for higher GS is not statistically significant. DRR, doubly robust regression; GS, glomerulosclerosis.
Figure 5 reveals a sharp discordance on the relationship between GS and graft failure risk, which tapered after approximately 10%, and the kidney discard rate, which rose precipitously beyond 10%. If, instead of being discarded 54.1%, 64.7%, and 85.8% of the time, the discard rate for kidneys with GS 11% to 15%, 16% to 20%, and 20%+ had matched the 45.4% rate observed in the 6% to 10% group, an additional 412 ECD kidneys would have been transplanted per year during 2018 to 2019.
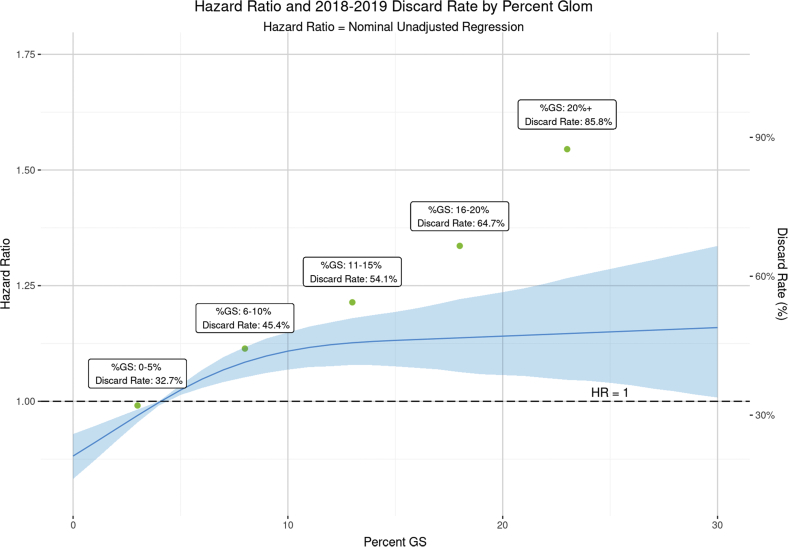
The discordant relationship between GS, all-cause graft survival, and kidney discard. The figure illustrates the estimated, nonlinear relationship between GS and 10-year graft failure risk, based on extended criteria donor transplants from 2008 to 2012. Kidney discard rates among ECD donors by GS category are superimposed using a second vertical axis. To provide a meaningful comparison, both analyses are unadjusted. The steep relationship between kidney discard rates for GS beyond 10% stands in sharp relief juxtaposed against the tapered relationship between GS and graft failure risk. CIs are found in blue. ECD, extended criteria donor; GS, glomerulosclerosis.
A sensitivity analysis using Empirical Bayes “shrunken” GS values adjusting for number of glomeruli observed revealed essentially the same findings as the nominal GS analysis: a steep increase in graft failure hazard that tapers beyond approximately 10% (Supplementary Figure S8).
Unadjusted graft survival differences by 3 levels of interstitial fibrosis were of borderline statistical significance (P = 0.052; Figure 6) but were fully attenuated after adjusting for potential confounders (Figure 7). In DRR analysis, the graft failure hazard for mild interstitial fibrosis was statistically no different from absent/minimal (HR 0.99; 95% CI: 0.91, 1.08). The hazard for mild-moderate/severe was also statistically similar from absent/minimal (HR 1.13; 95% CI 0.83, 1.39), although this estimate has greater statistical uncertainty. Death-censored graft survival did not differ statistically by interstitial fibrosis (P = 0.49; Supplementary Figure S9).
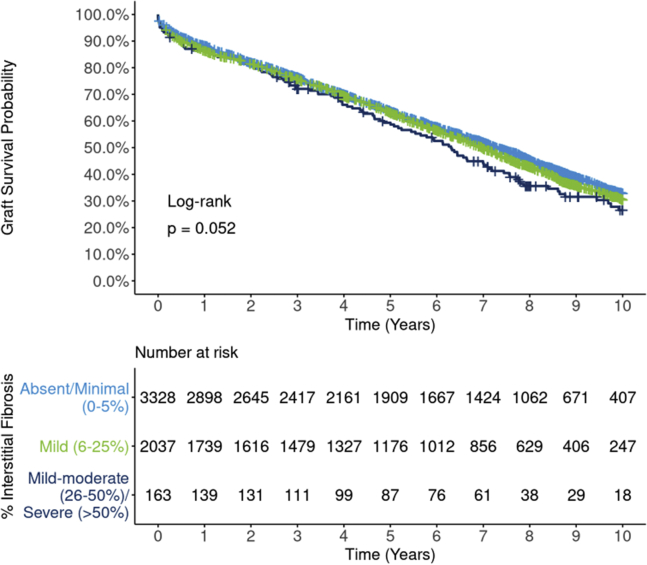
Ten-year, all-cause Kaplan-Meier graft survival by interstitial fibrosis. Estimated graft survival rates for 5528 extended criteria donor kidney transplants occurring between 2008 and 2012 are found by 3 levels of interstitial fibrosis. Differences between survival curves are of borderline statistical significance (P = 0.052), with the “absent/minimal” group having slightly superior unadjusted graft survival compared with the other groups. The survival curve for “mild-moderate/severe” transplants stands out to some degree as lower than the other groups but is based on a comparatively modest sample size.
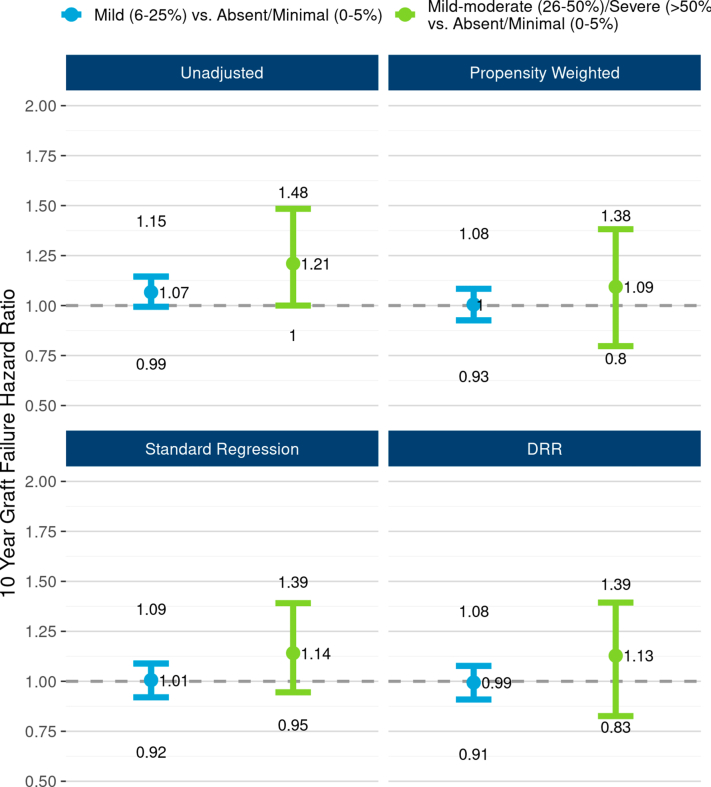
The unadjusted and adjusted associations between interstitial fibrosis and 10-year, all-cause graft failure risk. The figure illustrates all-cause graft failure hazard ratios and 95% CIs comparing 3 levels of interstitial fibrosis using the following 4 different analyses: unadjusted Cox regression (top left panel), propensity weighted Cox regression (top right), standard multivariable regression (bottom left), and doubly robust regression (DRR; bottom right). In unadjusted analysis, the risk of graft failure was 21% higher with “mild-moderate/severe” interstitial fibrosis compared with the reference group, “absent/minimal.” However, apparent effect of interstitial fibrosis was greatly tempered after adjusting for correlations with donor and recipient factors. In DRR analysis, the graft failure hazard ratio for “mild-moderate/severe” (vs. “absent/minimal”) was 1.13 (0.83–1.39). DRR, doubly robust regression.
Similarly, unadjusted graft survival differences by 3 levels of vascular changes were of borderline statistical significance (P = 0.052; Figure 8) but effects were attenuated after adjustment (Figure 9). In DRR analysis, the graft failure hazard for mild vascular changes was statistically no different from absent/minimal (HR 1.03; 95% CI: 0.93, 1.15). The hazard for mild-moderate/severe was also statistically similar to absent/minimal (HR 1.06; 95% CI 0.90, 1.26), but again with greater statistical uncertainty. However, both unadjusted (P = 0.031; Supplementary Figure S10) and DRR-adjusted analyses suggest a possible death-censored graft survival decrement for mild-moderate/severe vascular changes versus absent/minimal (DRR HR 1.30; 95% CI 1.02, 1.62). This effect seems to be driven largely by graft failures occurring beyond the 8 post-transplant year.
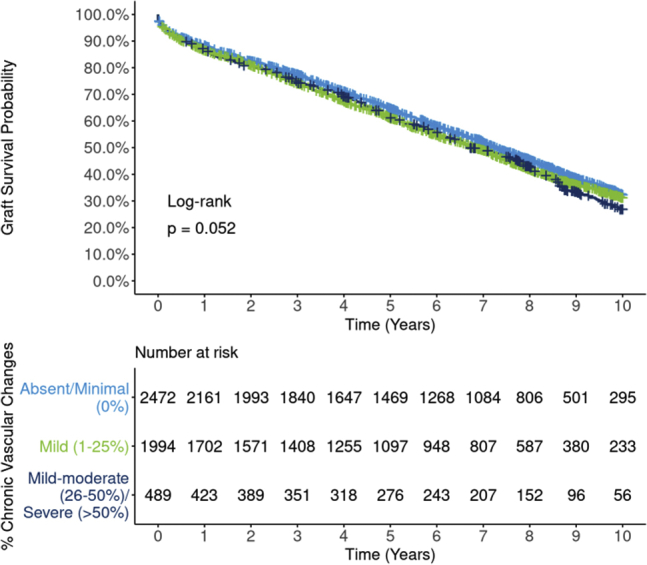
Ten-year Kaplan-Meier, all-cause graft survival by vascular changes. Estimated graft survival rates for 4995 extended criteria donor kidney transplants occurring between 2008 and 2012 are illustrated by 3 levels of vascular changes. Differences between survival curves are of borderline statistical significance (P = 0.052), with the “absent/minimal” group having slightly superior unadjusted graft survival compared with the other groups.
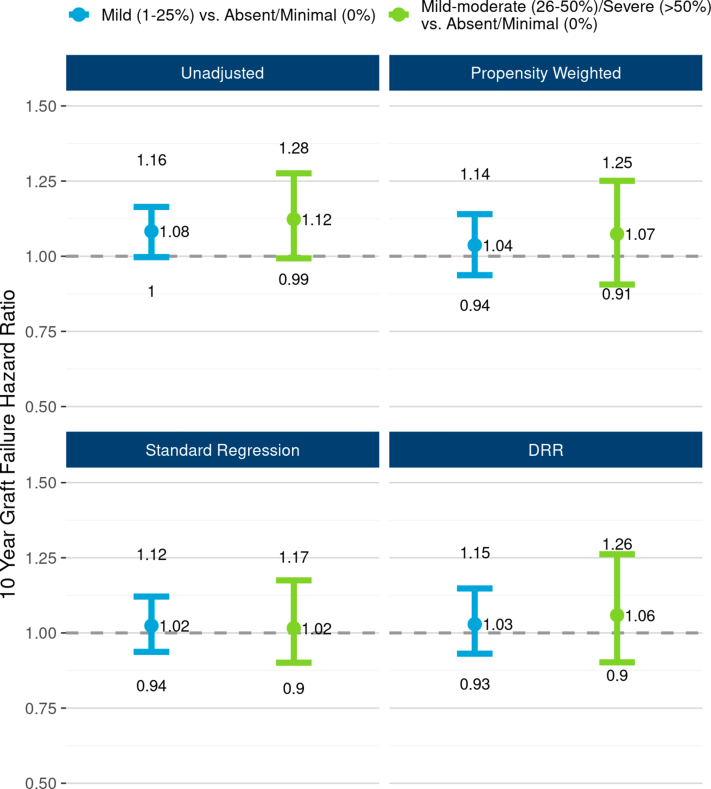
The unadjusted and adjusted associations between vascular changes and 10-year, all-cause graft failure risk. The figure illustrates all-cause graft failure hazard ratios and 95% CIs comparing 3 levels of vascular changes using the following 4 different analyses: unadjusted Cox regression (top left panel), propensity weighted Cox regression (top right), standard multivariable regression (bottom left), doubly robust regression (DRR; bottom right). In unadjusted analysis, the risk of graft failure was 12% higher with “mild-moderate/severe” vascular changes compared with the reference group, “absent/minimal.” However, apparent effect of vascular changes was greatly attenuated after adjusting for correlations with donor and recipient factors. In DRR analysis, the graft failure hazard ratio for “mild-moderate/severe” (vs. “absent/minimal”) was 1.06 (0.90–1.26). DRR, doubly robust regression.
Red data points found in Love plots74 (Supplementary Figures S11, S12, and S13) reveal particularly high correlations (large standardized differences among exposure groups) among biopsy compartments and between GS and KDPI. Teal data points indicate highly successful covariate balancing among exposure groups after propensity weighting, with all standardized differences falling near or below 0.1.75
Biopsy findings’ concordance was high among biopsied kidneys from the same donor: GS (rho = 0.55; Supplementary Figure S14), interstitial fibrosis (kappa = 0.78; Supplementary Table S4), and vascular changes (k = 0.75; Supplementary Table S5). Discarded mate kidneys tended to have higher GS and “worse” interstitial fibrosis and vascular changes (Supplementary Table S6). Though the number of glomeruli observed tended to be higher for wedge versus needle biopsies, the GS distributions were quite similar (Supplementary Table S7).
Discussion
After rigorous adjustment for possible confounders, the BARETO study found a clinically and statistically significant effect of GS on 10-year graft survival among ECD kidney transplants. Kidneys having GS > 10% were found to have 18% higher risk of graft failure compared with kidneys with GS of 0% to 5%. According to the familiar kidney donor risk index, an approximately 18% increased graft failure hazard is akin to the increased risk associated with a history of diabetes in the donor; a 0.7 higher creatinine (e.g., 1.4 vs. 0.7); or 7 additional years in donor age.76,77 Crucially, though a dose-response relationship between GS and graft failure risk was evident from 0% to 10%, the effect waned beyond 10%, suggesting little or no incremental risk associated with a GS of 20% compared with a GS of 10%. These findings echo death-censored, 5-year survival results published by Cheungpasitporn et al.77
Though we found no independent effect of mild (1%–25%) arteriosclerosis, this study suggests a possible, meaningfully large effect of mild-moderate (>25%) or worse vascular changes on long-term graft survival. This result echoes Kayler, who found reduced 1-year graft survival in ECD kidneys with moderate arteriosclerosis.78 However, because our finding manifested only in death-censored (not all-cause) analyses was of only borderline statistical significance and seems to be driven largely by a cluster of graft failures occurring after the 8 post-transplant year, interpretative caution is warranted and further study is needed.
By contrast, the apparent effects of interstitial fibrosis on graft survival were greatly attenuated after covariate-adjustment, suggesting this compartment provides minimal, if any, prognostic value above and beyond the usual donor quality parameters. This finding is consistent with the systematic review of Wang et al.,29 which concluded “the balance of the evidence does not currently support an association between tubular interstitial damage and GF, DGF, or long-term graft function.” This lack of association may reflect the more subjective nature of grading interstitial fibrosis in contrast to the more concretely defined (though still subject to error) GS. Despite evidence that frozen section preparation can exaggerate interstitial fibrosis,11 we were unable to identify a statistically significant, independent effect of this parameter on graft outcomes.
This study reveals that despite limitations such as varying sampling technique, quality, and interpretation, GS from procurement biopsies provides meaningful prognostic information beyond basic clinical and demographic parameters, such as donor age and KDPI. Yet, current practice suggests that data from biopsies may be doing more harm than good, perhaps because the degree to which these results affect graft outcomes has remained elusive.
A controlled experiment on transplant decision-making using hypothetical kidney offers found that “good” biopsy findings (compared with no biopsy) led to a sharp rise in acceptance of acute kidney injury kidneys, suggesting use of biopsies to rule-in kidneys in this clinical context.21,79 However, that same study found that “good” biopsy findings (compared with no biopsy) had virtually no effect on kidney transplant surgeons’ and nephrologists’ likelihood of ruling-in moderate-to-high KDPI kidneys. Lentine et al.17 found that performing a biopsy was not associated with a reduction in the discard rate among KDPI > 85% kidneys.
Currently, clinicians may currently be relying on questionable rules of thumb, such as GS > 20%9 or resistance > 0.4,80 to decline viable kidneys for transplantation. Though higher GS was found to be independently associated with graft failure risk, this study casts doubt on the justification for unilaterally relying on a GS > 20% threshold for declining a kidney, given the diminished decrement to graft survival beyond GS of 10%. Moreover, generally speaking, neither GS nor any other clinical parameter should be used in isolation to reject a transplant-quality kidney. Rather, a better approach is to leverage carefully developed multivariable risk predictions that empirically combine information to reduce decision-maker subjectivity and avoid double-counting correlated variables, compared with the “all or nothing” approach based on single-variable thresholds.
All else equal, offer acceptance rates were found to be 37% lower when interstitial fibrosis was reported as mild-moderate compared with absent in a controlled experiment.21 Our study found that the apparent increased risk associated with interstitial fibrosis is largely, if not entirely, accounted for by other factors. Clinical prediction models statistically adjust for such correlations to avoid the double counting trap.
The next phase of the BARETO study aims to incorporate biopsy, anatomy, and pumping parameters into augmented clinical prediction models, such as KDPI. If the practice of routinely obtaining a procurement biopsy in these kidneys is going to continue, incorporation of GS into an improved KDPI and/or other transplant prediction models81, 82, 83 may help allow “good” biopsy findings to help rule-in kidneys that might otherwise be discarded. Research has revealed that the KDPI is highly associated with organ discard rates and that changes in the KDPI “numeric label” itself can make a difference in kidney utilization decisions.84,85
The incorporation of GS into clinical prediction scores may help reduce discards by tempering the outsized effect this parameter has on transplant decision-making, particularly beyond 10%.86,87 If clinicians began to rely on new-and-improved, biopsy-informed prediction models for decision-making, knowing that the biopsy findings were already included in an evidence-based way, the unjustifiably high discard rates associated with high GS values (Figure 4) might also begin to taper. In fact, our analysis of contemporary kidney utilization practices suggests that upward of 400 more ECD kidneys could be transplanted annually in the United States if the runaway GS effect was tamed through more evidence-driven decision-making.
The BARETO study overcomes some of the limitations found in previously published biopsy analyses. Leveraging national registry data provided large sample sizes for increased statistical power. Using biopsy data uploaded into DonorNet reflects the real-world context in which biopsies are obtained and used. Our focus on ECD kidneys—which are universally almost always biopsied—reduces concerns about selection bias potentially introduced by for-cause biopsy data. Analysis of 10-year graft survival aligns more closely with outcomes that are meaningful to patients compared with the 1-year horizon typically reported. The ability to study GS along its continuum, instead of solely in arbitrary categories, has provided novel insights into an apparent tempering of the dose-response relationship between this parameter and graft survival.
Still, though rigorous causal inference methods were used, the usual limitations of observational studies still apply. It is conceivable that unmeasured variables and selection bias resulting from decisions to transplant versus discard kidneys may affect the results. However, the onus falls on the skeptic to postulate the existence of clinically plausible, unaccounted for factors that are sufficiently and independently correlated with both GS and graft survival, to cast serious doubt on the existence of a meaningfully large effect of GS >10% versus <5%. Effect sizes as large as our calculated E-values (e.g., HRs of 1.5–1.7) on long-term kidney graft survival are unusual,76,81 suggesting unmeasured confounding that is sufficiently and independently associated with both GS and graft failure to negate our estimated GS effects is highly unlikely. The combination of selection bias and small sample sizes likely explains the counterintuitive (though not quite statistically significant) apparent decline in hazard for GS beyond 20%; our findings should not be interpreted as suggesting outcomes actually improve with higher GS, but merely that the strong effect observed among lower GS values seems to attenuate quite sharply above approximately 10%.
Other study limitations include smaller sample sizes for the most extreme values of the 3 biopsy dimensions, particularly interstitial fibrosis (n = 163, mild-moderate/severe). The absence of statistical significance, which at times may merely reflect small sample sizes, should not nullify the potential importance of extreme findings—including GS values beyond approximately 30%—in organ utilization decisions. The central findings of this study should not be construed as a call to reduce the information provided in a biopsy report (e.g., by only reporting GS). In fact, the OPTN is currently proposing both the augmentation and standardization of data reported from procurement biopsies to aid in decision-making.88
Due to varying reporting standards on biopsy reports, we were also unable to stratify results by wedge versus needle, frozen versus permanent, or expert versus general pathologist. Inference from our study only applies to older, marginal donor kidneys, which are routinely biopsied; further research that carefully avoids selection bias driven by for-cause biopsies could help verify findings in non-ECD kidneys.
The US transplant community is divided on whether routine biopsies do more harm than good in the context of evaluating the suitability of marginal (e.g., ECD or high KDPI) kidneys for transplantation.1,89 The UK’s National Health Service is conducting a trial to determine whether routine use of biopsies through a centralized histopathology service will boost or hamper kidney utilization.90 Some have rightly questioned whether the additional information gained from procurement biopsies is worth the added cost and time in the context of an already pressing organ donation and transplant process.2
Should the European model of limited reliance on biopsies15 be universally adopted, or should transplant systems aim to standardize and improve on both the criteria for performing a biopsy23 and techniques used to obtain, interpret, and share biopsy information?91, 92, 93, 94, 95, 96 The goal of both camps is the same: to improve outcomes for patients with end-stage renal failure through timely and successful transplantation.
Given the widely recognized challenge of accurately predicting transplant outcomes,77,97,98 if biopsies do indeed contain statistically and clinically significant information beyond standard parameters, then they can improve our limited ability to risk stratify donor kidneys. However, though in theory more information should lead to better decisions, in the case of biopsy findings, more information may currently be causing more harm than good.1
If procurement biopsies are routinely used, a more evidence-driven approach to characterizing biopsy findings’ association with recipient outcomes to inform (and at times, temper) their use in clinical decision-making has the potential to reduce discards and increase the number of successful transplants. In this way, more data can yield what we would hope and expect—better, not worse, decisions on behalf of patients with renal failure.
Acknowledgments
The data reported here have been supplied by United Network for Organ Sharing as the contractor for the Organ Procurement and Transplantation Network. The interpretation and reporting of these data are the responsibility of the authors and in no way should be seen as an official policy of or interpretation by the Organ Procurement and Transplantation Network or the US Government. This research was supported by a grant from the Mendez National Institute of Transplantation Foundation. The authrs are grateful for the following contributions: Lindsey Jennings of United Network for Organ Sharing for identifying the funding opportunity. Virginia Commonwealth University students Perray Saravanene, Rym Yusfi, Shirley Yu, Charmy Patel, Ohm Tripathi, and Farhan Rasheed entered biopsy and anatomy data into REDCap; Duke University Sociology Professor Steve Vaisey provided valuable guidance along the way on doubly robust regression; Noah Greifer of Johns Hopkins provided troubleshooting assistance with the R WeightIt package.
Data Availability Statement
OPTN data are available on request from the OPTN. These requests may be submitted online at https://optn.transplant.hrsa.gov/data/request-data/.
Author Contributions
DES: BARETO study co-principal investigator; designed the study; led and guided all aspects of study execution; drafted the manuscript; incorporated edits and finalized the manuscript.
JF: contributed to the design of the study; conducted most of the statistical analyses; generated figures; reviewed and provided critical feedback on draft manuscript.
LK: contributed to the design of the study; provided clinical input; oversaw data entry to ensure quality; reviewed and provided critical feedback on draft manuscript.
SW: contributed to the design of the study; conducted statistical analyses; generated figures; reviewed and approved draft manuscript.
HSM: contributed to the design of the study; developed and maintained REDCap database; conducted data quality assessments; reviewed and provided critical feedback on draft manuscript.
MC: reviewed and provided critical feedback on clarity and interpretation of draft manuscript.
GG: BARETO study co-principal investigator; contributed to the design of the study; provided clinical input; reviewed and provided critical feedback on draft manuscript.
Footnotes
Figure S1. REDCap biopsy and anatomy data collection instrument.
Figure S2. Bootstrapped doubly robust regression process flow.
Figure S3. Nominal versus empirical Bayes (“Shrinkage”) estimates of glomerulosclerosis, by number of glomeruli observed.
Figure S4. Ten-year Kaplan-Meier death-censored graft survival by 3-level glomerulosclerosis.
Figure S5. Ten-year Kaplan-Meier all-cause graft survival by 5-level glomerulosclerosis.
Figure S6. Correlation between kidney donor profile index and kidney glomerulosclerosis.
Figure S7. Unadjusted and adjusted associations between 5-level glomerulosclerosis and 10-year all-cause graft failure risk.
Figure S8. Unadjusted and adjusted association between 10-year all-cause graft failure risk and empirical Bayes (“Shrunken”) estimate of glomerulosclerosis, modeled as a nonlinear function.
Figure S9. Ten-year Kaplan-Meier death-censored graft survival by interstitial fibrosis.
Figure S10. Ten-year Kaplan-Meier death-censored graft survival by vascular changes.
Figure S11. Love plot illustrating covariate balance across 3-level glomerulosclerosis after propensity score inverse probability weighting.
Figure S12. Love plot illustrating covariate balance across 3-level interstitial fibrosis after propensity score inverse probability weighting.
Figure S13. Love plot illustrating covariate balance across 3-level vascular changes after propensity score inverse probability weighting.
Figure S14. Correlation between left versus right kidney glomerulosclerosis in the same donor.
Table S1. Associations between glomerulosclerosis and modeled covariates, 2008 to 2012 biopsied ECD kidney transplants.
Table S2. Associations between interstitial fibrosis and modeled covariates, 2008 to 2012 biopsied ECD kidney transplants.
Table S3. Associations between vascular changes and modeled covariates, 2008 to 2012 biopsied ECD kidney transplants.
Table S4. Association between left versus right kidney interstitial fibrosis in the same donor.
Table S5. Association between left versus right kidney vascular changes in the same donor.
Table S6. Mate kidney analysis: biopsy finding comparison for transplanted versus discarded kidneys from the same donor.
Table S7. Number of glomeruli observed and glomerulosclerosis by biopsy sample type.
STROBE Checklist.
Supplementary Material
Figure S1. REDCap biopsy and anatomy data collection instrument.
Figure S2. Bootstrapped doubly robust regression process flow.
Figure S3. Nominal versus empirical Bayes (“Shrinkage”) estimates of glomerulosclerosis, by number of glomeruli observed.
Figure S4. Ten-year Kaplan-Meier death-censored graft survival by 3-level glomerulosclerosis.
Figure S5. Ten-year Kaplan-Meier all-cause graft survival by 5-level glomerulosclerosis.
Figure S6. Correlation between kidney donor profile index and kidney glomerulosclerosis.
Figure S7. Unadjusted and adjusted associations between 5-level glomerulosclerosis and 10-year all-cause graft failure risk.
Figure S8. Unadjusted and adjusted association between 10-year all-cause graft failure risk and empirical Bayes (“Shrunken”) estimate of glomerulosclerosis, modeled as a nonlinear function.
Figure S9. Ten-year Kaplan-Meier death-censored graft survival by interstitial fibrosis.
Figure S10. Ten-year Kaplan-Meier death-censored graft survival by vascular changes.
Figure S11. Love plot illustrating covariate balance across 3-level glomerulosclerosis after propensity score inverse probability weighting.
Figure S12. Love plot illustrating covariate balance across 3-level interstitial fibrosis after propensity score inverse probability weighting.
Figure S13. Love plot illustrating covariate balance across 3-level vascular changes after propensity score inverse probability weighting.
Figure S14. Correlation between left versus right kidney glomerulosclerosis in the same donor.
Table S1. Associations between glomerulosclerosis and modeled covariates, 2008 to 2012 biopsied ECD kidney transplants.
Table S2. Associations between interstitial fibrosis and modeled covariates, 2008 to 2012 biopsied ECD kidney transplants.
Table S3. Associations between vascular changes and modeled covariates, 2008 to 2012 biopsied ECD kidney transplants.
Table S4. Association between left versus right kidney interstitial fibrosis in the same donor.
Table S5. Association between left versus right kidney vascular changes in the same donor.
Table S6. Mate kidney analysis: biopsy finding comparison for transplanted versus discarded kidneys from the same donor.
Table S7. Number of glomeruli observed and glomerulosclerosis by biopsy sample type.
STROBE Checklist (PDF).
References
Articles from Kidney International Reports are provided here courtesy of Elsevier
Full text links
Read article at publisher's site: https://doi.org/10.1016/j.ekir.2022.05.027
Read article for free, from open access legal sources, via Unpaywall:
http://www.kireports.org/article/S2468024922014292/pdf
Citations & impact
Impact metrics
Citations of article over time
Alternative metrics

Discover the attention surrounding your research
https://www.altmetric.com/details/129445465
Article citations
Galileo-an Artificial Intelligence tool for evaluating pre-implantation kidney biopsies.
J Nephrol, 02 Oct 2024
Cited by: 0 articles | PMID: 39356416
Decoding the hallmarks of allograft dysfunction with a comprehensive pan-organ transcriptomic atlas.
Nat Med, 18 Jun 2024
Cited by: 0 articles | PMID: 38890530
Kidney transplants from elderly donors: what we have learned 20 years after the Crystal City consensus criteria meeting.
J Nephrol, 37(6):1449-1461, 06 Mar 2024
Cited by: 3 articles | PMID: 38446386 | PMCID: PMC11473582
Review Free full text in Europe PMC
Value of original and modified pathological scoring systems for prognostic prediction in paraffin-embedded donor kidney core biopsy.
Ren Fail, 46(1):2314630, 12 Feb 2024
Cited by: 0 articles | PMID: 38345067 | PMCID: PMC10863519
The Independent Effects of Kidney Length and Vascular Plaque on Ten-Year Outcomes of Extended Criteria Donor Kidney Transplants.
Transpl Int, 36:11373, 14 Jul 2023
Cited by: 0 articles | PMID: 37519905 | PMCID: PMC10379651
Go to all (8) article citations
Similar Articles
To arrive at the top five similar articles we use a word-weighted algorithm to compare words from the Title and Abstract of each citation.
Degree of Glomerulosclerosis in Procurement Kidney Biopsies from Marginal Donor Kidneys and Their Implications in Predicting Graft Outcomes.
J Clin Med, 9(5):E1469, 14 May 2020
Cited by: 7 articles | PMID: 32422905 | PMCID: PMC7291279
Association of Deceased Donor Acute Kidney Injury With Recipient Graft Survival.
JAMA Netw Open, 3(1):e1918634, 03 Jan 2020
Cited by: 21 articles | PMID: 31913491 | PMCID: PMC6991314
The Independent Effects of Kidney Length and Vascular Plaque on Ten-Year Outcomes of Extended Criteria Donor Kidney Transplants.
Transpl Int, 36:11373, 14 Jul 2023
Cited by: 0 articles | PMID: 37519905 | PMCID: PMC10379651
A systematic review of kidney transplantation from expanded criteria donors.
Am J Kidney Dis, 52(3):553-586, 01 Sep 2008
Cited by: 163 articles | PMID: 18725015
Review
Funding
Funders who supported this work.