Abstract
Purpose
Models used to predict the probability of an individual having a pathogenic homozygous or heterozygous variant in a mismatch repair gene, such as MMRpro, are widely used. Recently, MMRpro was updated with new colorectal cancer penetrance estimates. The purpose of this study was to evaluate the predictive performance of MMRpro and other models for individuals with a family history of colorectal cancer.Methods
We performed a validation study of 4 models, Leiden, MMRpredict, PREMM5, and MMRpro, using 784 members of clinic-based families from the United States. Predicted probabilities were compared with germline testing results and evaluated for discrimination, calibration, and predictive accuracy. We analyzed several strategies to combine models and improve predictive performance.Results
MMRpro with additional tumor information (MMRpro+) and PREMM5 outperformed the other models in discrimination and predictive accuracy. MMRpro+ was the best calibrated with an observed to expected ratio of 0.98 (95% CI = 0.89-1.08). The combination models showed improvement over PREMM5 and performed similar to MMRpro+.Conclusion
MMRpro+ and PREMM5 performed well in predicting the probability of having a pathogenic homozygous or heterozygous variant in a mismatch repair gene. They serve as useful clinical decision tools for identifying individuals who would benefit greatly from screening and prevention strategies.Free full text

A validation of models for prediction of pathogenic variants in mismatch repair genes
Abstract
Purpose:
Models used to predict the probability of an individual having a pathogenic homozygous or heterozygous variant in a mismatch repair gene, such as MMRpro, are widely used. Recently, MMRpro was updated with new colorectal cancer penetrance estimates. The purpose of this study was to evaluate the predictive performance of MMRpro and other models for individuals with a family history of colorectal cancer.
Methods:
We performed a validation study of 4 models, Leiden, MMRpredict, PREMM5, and MMRpro, using 784 members of clinic-based families from the United States. Predicted probabilities were compared with germline testing results and evaluated for discrimination, calibration, and predictive accuracy. We analyzed several strategies to combine models and improve predictive performance.
Results:
MMRpro with additional tumor information (MMRpro+) and PREMM5 outperformed the other models in discrimination and predictive accuracy. MMRpro+ was the best calibrated with an observed to expected ratio of 0.98 (95% CI = 0.89–1.08). The combination models showed improvement over PREMM5 and performed similar to MMRpro+.
Conclusion:
MMRpro+ and PREMM5 performed well in predicting the probability of having a pathogenic homozygous or heterozygous variant in a mismatch repair gene. They serve as useful clinical decision tools for identifying individuals who would benefit greatly from screening and prevention strategies.
Introduction
Lynch syndrome (LS), the most common syndrome related to gastrointestinal cancers,1–3 is caused by pathogenic variants in the mismatch repair (MMR) genes (MLH1, MSH2, MSH6, PMS2) or EPCAM.1,2,4–6 These variants confer a 30% to 70% lifetime penetrance (risk of cancer) of colorectal cancer (CRC) and increase the risk of developing other cancers, including endometrial, stomach, and biliary tract cancers.4,7–11
Current clinical guidelines for assessing an individual’s LS risk include the Amsterdam II criteria, Bethesda guidelines, and National Comprehensive Cancer Network guidelines,4,12,13 or more quantitative risk assessment.14 Prediction models such as MMRpro,15 PREMM5,16 MMRpredict,17 and Leiden18 were developed to predict the probability of an individual having a pathogenic homozygous or heterozygous variant in one of the MMR genes or EPCAM. The National Comprehensive Cancer Network guidelines recommend genetic testing for individuals with predicted probability of >5% in MMRpro, MMRpredict, or 2.5% in PREMM5.19
Although the performance of these models was validated previously,16,20–26 the most recent version of MMRpro (version 2.1–6) with updated CRC penetrance estimates based on Wang et al27 has not been validated to date. The main objective of this study was to evaluate the performance of Leiden, PREMM5, MMRpredict, and MMRpro in predicting the probability of having a pathogenic heterozygous or homozygous LS variant in individuals with a family history of CRC. The secondary objective was to combine 2 of the most widely used models, PREMM5 and MMRpro, with the goal of producing a potentially more accurate and applicable consensus model.
Materials and Methods
Data collection
The main analysis for this study included 784 families from 5 sites: Creighton University School of Medicine, Dana-Farber Cancer Institute, Johns Hopkins University, MD Anderson Cancer Center, and Memorial Sloan Kettering Cancer Center. An additional analysis included 2729 population- and clinic-based families from the Colon Cancer Family Registries (CCFRs) across 7 institutions: Ontario Familial Colorectal Cancer Registry, University of Southern California (USC) Consortium, Australasian Colorectal Cancer Family Registry, Hawaii Colorectal Cancer Family Registry, Mayo Colorectal Cancer Family Registry, Seattle Familial Colon Cancer Registry, and UCSF Colon Cancer Family Registry. We excluded these families from the main analysis because the updated penetrance in MMRpro were estimated on the basis of a meta-analysis,27 which included published penetrance estimates from the CCFR data. At each participating site, the investigators obtained permission from participants and compiled data. All subsequent model validation was performed at the BayesMendel Lab at Dana-Farber Cancer Institute. We refer to the individual for whom the probability of having a pathogenic homozygous or heterozygous LS variant is calculated as the counselee.
Models
The characteristics, input, and output of the models are summarized in Table 1. PREMM5, MMRpredict, and Leiden are empirical models that use multivariate logistic regression to characterize the relationship between genetic testing results and family history and/or other clinical characteristics. PREMM5 provides the probability of identifying a pathogenic variant in MLH1, MSH2/EPCAM, MSH6, or PMS2. It was first developed on a cohort of 18,734 individuals tested for all 5 genes and externally validated on 1058 patients with CRC. PREMM5 uses personal history of CRC, endometrial cancer (EC), or other LS-associated cancers of the individual being evaluated and the types of cancer and ages at diagnosis of first-degree and second-degree relatives from the affected side of the family. MMRpredict provides the probability of identifying a pathogenic variant in MLH1 or MSH2. It was developed on 870 patients under the age of 55 years recently diagnosed with CRC. The model incorporates information on the counselee’s age at CRC diagnosis, sex, tumor location (proximal or distal), presence of a synchronous and/or metachronous tumor, and CRC and EC family history. In our analysis, we evaluated MMRpredict on individuals affected by CRC under the age of 55 years. The Leiden model provides the probability of identifying a pathogenic variant in MLH1 or MSH2. It was developed using 184 families and incorporates the mean age at diagnosis of CRC among affected family members, an indicator of EC in any family member, and an indicator of whether the family met the Amsterdam criteria. MMRpro is a Mendelian model29 that incorporates the autosomal dominant inheritance of MMR pathogenic variants with parameters based on meta-analyses of their penetrance and prevalence. It uses family history and tumor information, eg, microsatellite instability (MSI) and location. MMRpro is freely available in the BayesMendel R library.30 In this study, we validated version 2.1–6, which includes updated penetrance estimates based on Wang et al.27
Table 1
Summary of characteristics, input, and output variables of the 4 models studied
Model characteristics | Model | |||
---|---|---|---|---|
Leiden | MMRpredict | MMRpro | PREMM5 | |
Mendelian | • | |||
Empirical | • | • | • | |
Uses full pedigree | • | |||
Available in the CaGene package | • | |||
Trained on high-risk families | • | • | • | |
Updated periodically | • | • | ||
References: development | Wijnen et al18 | Barnetson et al17 | Chen et al15 | Kastrinos et al16 |
References: validation | Monzon et al,20 | Barnetson et al,17 | Chen et al,15 | Kastrinos et al16 |
Green et al,23 | Monzon et al,20 | Monzon et al,20 | ||
Jasperson et al28 | Green et al,23 | Green et al,23 | ||
Pouchet et al,24 | Mercado et al26 | |||
Mercado et al26 | ||||
Model | ||||
Model Input | Leiden | MMRpredict | MMRpro | PREMM5 |
Mendelian Transmission | • | |||
Exact family structure | • | |||
Unaffected counselee age | • | |||
Unaffected relatives age | • | |||
CRC status, counselee | • | • | • | |
CRC status, relatives | • | • | • | |
CRC age of onset, counselee | • | • | • | • |
CRC age of onset, relatives | • | • | • | • |
EC status, counselee | • | • | • | |
EC status, relatives | • | • | • | • |
EC age of onset, counselee | • | • | ||
EC age of onset, relatives | • | • | ||
Both CRC and EC in counselee | • | • | ||
Both CRC and EC in a single relative | • | • | • | |
Current age, counselee | • | • | ||
Extracolonic cancer | • | |||
MSI testing in counselee | • | |||
MSI testing in relatives | • | |||
Tumor location (proximal or distal) in counselee | • | • | ||
Synchronous/metachronous tumor in counselee | • | |||
Model | ||||
Model output | Leiden | MMRpredict | MMRpro | PREMM5 |
Probability of getting a positive test result | • | • | • | |
Probability of having a pathogenic variant | • | |||
Predictions for MLH1 | • | • | • | • |
Predictions for MSH2 | • | • | • | • |
Predictions for MSH6 | • | • | ||
Predictions for PMS2 | • | |||
Future risk of CRC or EC | • |
Bullet point indicates “yes,” and absence of a bullet point indicates “no.”
CRC, colorectal cancer; EC, endometrial cancer; MSI, microsatellite instability.
Statistical analysis
We assessed the models for discrimination, calibration, and predictive accuracy.31–33 In addition to MMRpro, we evaluated the performance of MMRpro with MSI and tumor location information (MMRpro+). MMRpro+ defaults to MMRpro when no MSI and tumor location information is available. We measured discrimination with the c-statistic. A c-statistic of 0 or 1 represents perfect discordance or concordance, respectively, between the predicted and observed outcomes. A value of 0.5 means that the model is the same at discriminating between individuals with a pathogenic homozygous or heterozygous LS variant and those without as random chance. We measured calibration with the ratio of observed to expected (O/E ratio) positive test results. An O/E ratio > 1 indicates underprediction (O/E ratio < 1 indicates overprediction), with 1 corresponding to perfect overall calibration between observed and predicted outcomes. The Brier score measures the extent of disagreement between predicted and observed outcomes, with lower values indicating better model performance. At 5% and 2.5% thresholds in outcome probability, we assessed predictive accuracy with positive predictive value (PPV) and negative predictive value (NPV). We computed 95% CIs for all measures using 1000 bootstrap samples. Because model performance for any pair of models is typically highly correlated across bootstrap replicates, overlap of CIs is not a reliable form of comparison of performance across methods. Therefore, we conducted analysis comparing the bootstrap replicates of model pairs to address how likely one model outperforms the other. Specifically, for each performance metric, we calculated the improvement frequency (IF), defined as the proportion of bootstrap replicates in which one model outperformed the other,34 ie, higher c-statistic, O/E ratio closer to 1, higher PPV and NPV, and lower Brier score. In addition to evaluating the models in the main analysis, we evaluated them on families from CCFR. Analyses were performed using R version 3.6.3 (R Core Team).
Model combination
We analyzed several strategies for combining PREMM5 and MMRpro+: logistic regression, generalized additive models (GAMs) with multivariate smoothing splines, and random forests. These methods are commonly used and differ in their complexity and assumptions. In each, we combined PREMM5 and MMRpro+ predictions by treating the probability of an individual having a pathogenic homozygous or heterozygous LS variant generated by each model as new predictors and using the true status as the outcome. We randomly divided the data into training and test sets of equal sizes and repeated this process using 100 iterations of Monte Carlo cross-validation.35 In addition to c-statistic, O/E ratio, PPV/NPV, and Brier score, we evaluated the combination models on the basis of net reclassification index (NRI)36 and log loss.37 The NRI quantifies how well a combination model correctly reclassifies individuals compared with PREMM5 or MMRpro+ alone, and log loss the agreement between predicted probabilities and observed outcomes. We calculated the performance metrics on each of the 100 testing sets and averaged them to obtain the mean performance. We performed this analysis on the same data set as the main analysis.
Results
Characteristics of the counselees are provided in Table 2. Of the 784 counselees, 413 (52.7%) had CRC, with a mean age of onset of 44.6 years. In total, 230 (29.3%) counselees had one of the MLH1, MSH2, or MSH6 pathogenic variants, and 280 (35.7%) counselees were male. Race information and genetic test results on PMS2 and EPCAM were not available.
Table 2
Clinical information for counselees, by center and overall
Category | CU, n (%) | JHU, n (%) | MDA, n (%) | MSKCC, n (%) | DFCI, n (%) | All Centers, N (%) |
---|---|---|---|---|---|---|
Total counselees | 55 (100) | 59 (100) | 143 (100) | 201 (100) | 326 (100) | 784 (100) |
Male counselees | 24 (43.64) | 24 (40.68) | 69 (48.25) | 74 (36.82) | 89 (27.3) | 280 (35.71) |
Counselees with CRC | 29 (52.73) | 42 (71.19) | 131 (91.61) | 104 (51.74) | 107 (32.82) | 413 (52.68) |
Male counselees with CRC | 15 (27.27) | 15 (25.42) | 65 (45.45) | 49 (24.38) | 48 (14.72) | 192 (24.49) |
Female counselees with CRC | 14 (25.45) | 27 (45.76) | 66 (46.15) | 55 (27.36) | 59 (18.1) | 221 (28.19) |
Female counselees with EC | 7 (12.73) | 2 (3.39) | 5 (3.5) | 25 (12.44) | 68 (20.86) | 107 (13.65) |
Counselees with no cancer | 21 (38.18) | 17 (28.81) | 8 (5.59) | 57 (28.36) | 102 (31.29) | 205 (26.15) |
Counselees with an extracolonic cancera | 9 (16.36) | 0 (0) | 22 (15.38) | 27 (13.43) | 64 (19.63) | 122 (15.56) |
Counselees with multiple primary cancersb | 17 (30.91) | 1 (1.69) | 77 (53.85) | 34 (16.92) | 64 (19.63) | 193 (24.62) |
Counselees with previous adenoma(s)c | 14 (25.45) | 2 (3.39) | 0 (0) | 79 (39.3) | 149 (45.71) | 244 (31.12) |
Number of known proximal tumors | 9 (16.36) | 15 (25.42) | 15 (10.49) | 0 (0) | 0 (0) | 39 (4.97) |
Number of known distal tumors | 8 (14.55) | 14 (23.73) | 24 (16.78) | 0 (0) | 0 (0) | 46 (5.87) |
Number of tumors with unknown location | 12 (21.82) | 13 (22.03) | 92 (64.34) | 104 (51.74) | 107 (32.82) | 328 (41.84) |
MSI+d | 0 (0) | 12 (20.34) | 1 (0.7) | 48 (23.88) | 26 (7.98) | 87 (11.1) |
MSI− (and also germline tested) | 0 (0) | 0 (0) | 0 (0) | 46 (22.89) | 64 (19.63) | 110 (14.03) |
MSI− (and not also germline tested) | 0 (0) | 0 (0) | 0 (0) | 0 (0) | 0 (0) | 0 (0) |
Not MSI tested | 55 (100) | 47 (79.66) | 142 (99.3) | 107 (53.23) | 236 (72.39) | 587 (74.87) |
MLH1+ | 19 (34.55) | 11 (18.64) | 29 (20.28) | 25 (12.44) | 15 (4.6) | 99 (12.63) |
MLH1 tested | 31 (56.36) | 59 (100) | 109 (76.22) | 157 (78.11) | 311 (95.4) | 667 (85.08) |
MSH2+ | 15 (27.27) | 7 (11.86) | 39 (27.27) | 43 (21.39) | 13 (3.99) | 117 (14.92) |
MSH2 tested | 24 (43.64) | 59 (100) | 103 (72.03) | 194 (96.52) | 312 (95.71) | 692 (88.27) |
MSH6+ | 0 (0) | 0 (0) | 0 (0) | 4 (1.99) | 14 (4.29) | 18 (2.30) |
MSH6 tested | 0 (0) | 0 (0) | 0 (0) | 11 (5.47) | 300 (92.02) | 311 (42.22) |
Total positive | 34 (61.82) | 18 (30.51) | 68 (47.55) | 68 (33.83) | 42 (12.88) | 230 (29.34) |
Median family size | 133 | 19 | 19 | 44 | 33 | 34 |
Mean age onset CRC | 43.0 | 43.4 | 41.8 | 46.0 | 47.4 | 44.6 |
Mean age CRC (males) | 42.7 | 41.1 | 40.4 | 46.5 | 47.9 | 44.1 |
Mean age onset CRC (females) | 43.2 | 44.6 | 43.2 | 45.6 | 47.1 | 45.0 |
Mean age onset EC (females) | 46.6 | 54.0 | 44.4 | 49.0 | 49.6 | 49.1 |
n = sample size; % = percent of individuals at a given center.
CRC, colorectal cancer; CU, Creighton University School of Medicine; DFCI, Dana-Farber Cancer Institute; EC, endometrial cancer; JHU, Johns Hopkins University; MDA, MD Anderson Cancer Center; MSI, microsatellite instability; MSKCC, Memorial Sloan Kettering Cancer Center.
Model performance main analysis
We defined the binary outcome as the positive test for a pathogenic homozygous or heterozygous variant in any of the 3 genes, MLH1, MSH2, or MSH6. Table 3 shows c-statistics, O/E ratios, PPVs, NPVs, and Brier scores for the models stratified by CRC status. C-statistics ranged from 77% (95% CI = 0.73–0.81) to 80% (95% CI = 0.76–0.84) overall, 76% (95% CI = 0.72–0.81) to 81% (95% CI = 0.76–0.85) among CRC-affected counselees, and 74% (95% CI = 0.66–0.81) to 79% (95% CI = 0.72–0.84) for CRC-unaffected counselees. For c-statistics, MMRpro+ outperformed Leiden (IF = 96.9%) and performed similar to PREMM5 (IF = 58%). Among CRC-affected counselees, it outperformed MMRpredict, Leiden, and PREMM5 with IFs of 99.5%, 99.4%, and 63.2%, respectively. Among CRC-unaffected counselees, Leiden performed the best in terms of c- statistics with IFs of at least 84% when compared with other models. Figure 1 shows that all models performed best in the 40–49 age interval. According to results from the paired analysis in Supplemental Table 1, MMRpro+ outperformed other models across most age intervals with IFs of at least 63%. In the 50 to 59 years age interval, it performed similarly to Leiden (IF = 45.9%) and PREMM5 (IF = 53.1%).
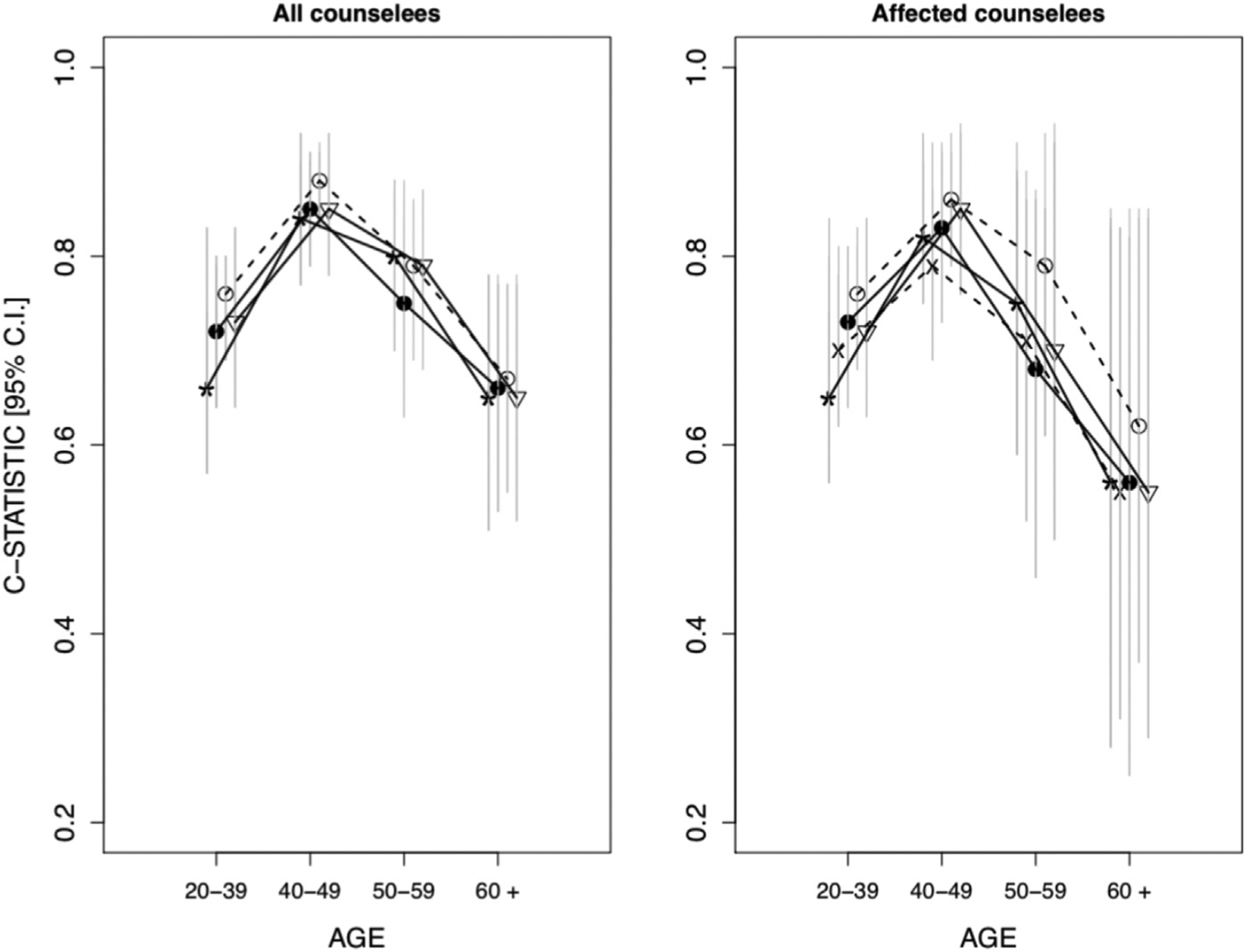
Star indicates Leiden, solid circle indicates MMRpro, hollow circle indicates MMRpro+, upside-down triangle indicates PREMM5, and X indicates MMRpredict. Vertical gray bars represent the 95% bootstrapped CIs.
Table 3
Metrics for the combined outcome status for any of the 3 MMR genes
Models | C-Statistic | Observed to Expected Ratio | PPVa | PPVb | NPVa | NPVb | Brier Score | |||
---|---|---|---|---|---|---|---|---|---|---|
All counselees (N = 784)c | ||||||||||
Leiden | 0.77 (0.73–0.81) | 1.27 (1.14–1.40) | 0.38 (0.34–0.43) | 0.36 (0.33–0.40) | 0.89 (0.86–0.92) | 0.91 (0.88–0.95) | 0.18 (0.16–0.2) | |||
MMRpro | 0.78 (0.74–0.82) | 0.95 (0.86–1.04) | 0.42 (0.38–0.47) | 0.39 (0.34–0.43) | 0.87 (0.84–0.91) | 0.88 (0.84–0.91) | 0.18 (0.16–0.21) | |||
MMRpro+ | 0.80 (0.77–0.84) | 0.98 (0.89–1.08) | 0.46 (0.41–0.51) | 0.42 (0.38–0.46) | 0.88 (0.85–0.91) | 0.88 (0.85–0.92) | 0.17 (0.15–0.19) | |||
PREMM5 | 0.80 (0.76–0.84) | 1.71 (1.54–1.9) | 0.47 (0.42–0.52) | 0.40 (0.35–0.44) | 0.88 (0.85–0.91) | 0.89 (0.85–0.92) | 0.17 (0.15–0.19) | |||
Improvement Frequency Across Bootstrap Replicatesd | ||||||||||
MMRpro > MMRpro+ | 0.001 | 0.124 | 0 | 0 | 0.076 | 0.159 | 0.001 | |||
MMRpro > PREMM5 | 0.058 | 1 | 0 | 0.245 | 0.296 | 0.243 | 0.044 | |||
MMRpro > Leiden | 0.787 | 0.958 | 0.870 | 0.713 | 0.131 | 0.020 | 0.192 | |||
MMRpro+ > PREMM5 | 0.580 | 1 | 0.232 | 0.986 | 0.631 | 0.471 | 0.435 | |||
MMRpro+ > Leiden | 0.969 | 0.985 | 1 | 0.999 | 0.347 | 0.085 | 0.654 | |||
PREMM5 > Leiden | 0.977 | 0 | 1 | 0.917 | 0.242 | 0.052 | 0.806 | |||
CRC-affected counselees (n = 413) | ||||||||||
Model performance | ||||||||||
Leiden | 0.76 (0.72–0.81) | 1.56 (1.39–1.74) | 0.49 (0.43–0.55) | 0.46 (0.41–0.52) | 0.83 (0.76–0.89) | 0.86 (0.78–0.93) | 0.21 (0.18–0.24) | |||
MMRpredict | 0.77 (0.72–0.81) | 0.83 (0.74–0.92) | 0.44 (0.39–0.49) | 0.42 (0.37–0.47) | 0.85 (0.76–0.94) | 0.82 (0.69–0.93) | 0.22 (0.19–0.25) | |||
MMRpro | 0.77 (0.72–0.82) | 0.88 (0.78–0.98) | 0.49 (0.43–0.55) | 0.45 (0.4–0.51) | 0.79 (0.72–0.86) | 0.79 (0.7–0.87) | 0.23 (0.19–0.26) | |||
MMRpro+ | 0.81 (0.77–0.85) | 0.92 (0.82–1.02) | 0.55 (0.48–0.61) | 0.51 (0.45–0.57) | 0.85 (0.79–0.9) | 0.86 (0.79–0.92) | 0.2 (0.17–0.24) | |||
PREMM5 | 0.81 (0.76–0.85) | 1.55 (1.38–1.73) | 0.53 (0.47–0.59) | 0.45 (0.39–0.5) | 0.86 (0.8–0.91) | 0.82 (0.73–0.9) | 0.19 (0.16–0.22) | |||
Improvement Frequency Across Bootstrap Replicatesd | ||||||||||
MMRpro > MMRpro+ | 0 | 0.027 | 0 | 0 | 0.001 | 0.001 | 0 | |||
MMRpro > PREMM5 | 0.005 | 1 | 0.016 | 0.586 | 0.011 | 0.169 | 0.015 | |||
MMRpro > Leiden | 0.625 | 1 | 0.389 | 0.118 | 0.170 | 0.034 | 0.103 | |||
MMRpro > MMRpredict | 0.580 | 0.955 | 0.999 | 0.996 | 0.074 | 0.244 | 0.205 | |||
MMRpro+ > PREMM5 | 0.632 | 1 | 0.849 | 1 | 0.372 | 0.822 | 0.213 | |||
MMRpro+ > Leiden | 0.994 | 1 | 0.997 | 0.995 | 0.698 | 0.443 | 0.610 | |||
MMRpro+ > MMRpredict | 0.995 | 0.994 | 1 | 1 | 0.409 | 0.724 | 0.812 | |||
PREMM5 > Leiden | 0.986 | 0.581 | 0.968 | 0.063 | 0.826 | 0.144 | 0.964 | |||
PREMM5 > MMRpredict | 0.995 | 0.001 | 1 | 0.999 | 0.514 | 0.485 | 0.926 | |||
Leiden > MMRpredict | 0.445 | 0.002 | 1 | 1 | 0.266 | 0.738 | 0.685 | |||
Unaffected counselees (n = 371) | ||||||||||
Model performance | ||||||||||
Leiden | 0.79 (0.72–0.84) | 0.84 (0.67–1.02) | 0.25 (0.20–0.31) | 0.24 (0.19–0.29) | 0.93 (0.90–0.97) | 0.94 (0.90–0.97) | 0.14 (0.12–0.17) | |||
MMRpro | 0.75 (0.68–0.82) | 1.18 (0.95–1.46) | 0.31 (0.24–0.39) | 0.28 (0.21–0.34) | 0.92 (0.88–0.96) | 0.92 (0.88–0.96) | 0.13 (0.11–0.16) | |||
MMRpro+ | 0.74 (0.66–0.81) | 1.20 (0.95–1.47) | 0.31 (0.24–0.39) | 0.27 (0.2–0.34) | 0.91 (0.87–0.94) | 0.90 (0.86–0.94) | 0.13 (0.11–0.16) | |||
premm5 | 0.74 (0.66–0.81) | 2.32 (1.80–2.92) | 0.35 (0.26–0.44) | 0.29 (0.22–0.36) | 0.89 (0.85–0.93) | 0.91 (0.87–0.95) | 0.14 (0.11–0.17) | |||
Improvement Frequency Across Bootstrap Replicatesd | ||||||||||
MMRpro > MMRpro+ | 0.859 | 0.591 | 0.438 | 0.725 | 0.951 | 0.979 | 0.407 | |||
MMRpro > PREMM5 | 0.774 | 1 | 0.121 | 0.249 | 0.980 | 0.734 | 0.738 | |||
MMRpro > Leiden | 0.160 | 0.621 | 0.923 | 0.919 | 0.155 | 0.067 | 0.592 | |||
MMRpro+ > PREMM5 | 0.537 | 1 | 0.152 | 0.184 | 0.900 | 0.311 | 0.772 | |||
MMRpro+ > Leiden | 0.083 | 0.592 | 0.912 | 0.783 | 0.050 | 0.005 | 0.629 | |||
PREMM5 > Leiden | 0.037 | 0 | 0.983 | 0.979 | 0.004 | 0.007 | 0.348 |
Rows correspond to models, columns correspond to metrics: c-statistic, observed to expected ratio, and positive and negative predictive value results across centers, and sections correspond to stratified analyses. Number in parentheses are 95% bootstrap CIs.
LS, Lynch syndrome; MMR, mismatch repair; NPV, negative predictive value; PPV, positive predictive value.
For calibration, Leiden and PREMM5 underpredicted the number of individuals with a pathogenic homozygous or heterozygous LS variant overall with O/E ratios >1. MMRpro and MMRpro+ performed very well with O/E ratios of 0.95 (95% CI = 0.86–1.04) and 0.98 (95% CI = 0.89–1.08), respectively. The CIs for both models contained 1.0 (perfect calibration). For CRC-affected counselees, MMRpredict and MMRpro tended to overpredict, with O/E ratios of 0.83 (95% CI = 0.74–0.92) and 0.88 (95% CI = 0.78–0.98), respectively. For CRC-unaffected counselees, all models except Leiden underpredicted (O/E ratio = 0.84 [95% CI = 0.67–1.02]). Paired analysis for calibration showed MMRpro+ was better calibrated than other models with IFs of 59% or higher on the whole data set. Table 3 also shows the PPVs and NPVs based on 5% and 2.5% thresholds in probability. At both thresholds, all 4 models had higher NPV than PPV with NPVs around 0.90. PREMM5 and MMRpro+ had the highest PPV of 0.47 (95% CI = 0.42–0.52) and 0.42 (95% CI = 0.38–0.46) at 5% and 2.5% thresholds, respectively. At the lower threshold (2.5%), we observed higher NPVs but lower PPVs overall. Paired analysis comparing MMRpro+ to PREMM5 suggested that the 2 models have different PPV performance depending on the threshold, with PREMM5 posting improvements about 77% of the time at the 5% threshold but less than 2% of the time at the 2.5% threshold. Overall, the Brier scores ranged from 0.17 to 0.18 across all models, and paired analysis showed that PREMM5 tended to have lower Brier scores than other models with IFs of >56%.
Additional analysis
Counselee information and model validation results from the additional analysis are shown in Supplemental Tables 2 and 3, respectively. Overall, models performed similarly regardless of whether or not we included the CCFR families as part of the validation data set. MMRpro+ performed the best in terms of discrimination and calibration with a c-statistic of 0.85 (95% CI = 0.83–0.86) and an O/E ratio of 1.02 (95% CI = 0.96–1.06); results from the paired analysis showed that it had IFs of >97% in discrimination and calibration when compared with other models. At the 5% threshold in outcome probability, PREMM5 had the highest PPV of 0.47 (95% CI = 0.44–0.50), and MMRpro+ the highest NPV of 0.93 (95% CI = 0.92–0.94), and these results were supported by the paired analysis. At the 2.5% threshold in outcome probability, MMRpro+ had the highest PPV and NPV. Brier scores ranged from 0.13 (95% CI = 0.12–0.14) to 0.15 (95% CI = 0.14–0.16), and MMRpro+ outperformed the other models, with IFs of more than 99%.
Combination model performance
Table 4 provides the c-statistics, O/E ratios, Brier scores, and paired analysis results for the 3 combination models (logistic regression, GAM with splines, and random forest). Overall, none of these models provided a meaningful improvement over MMRpro+. Logistic regression and GAM with splines had similar c-statistics to MMRpro+ (0.83, 95% CI = 0.80–0.86) and showed improvement over PREMM5 (0.81, 95% CI = 0.77–0.84), whereas random forest had a slightly lower c-statistic (0.78, 95% CI = 0.75–0.82). The O/E ratios for all 3 models logistic regression: 1.00 (95% CI = 0.90–1.09), splines: 0.98 (95% CI = 0.83–1.23), random forest: 1.00 (95% CI = 0.89–1.10) improved over PREMM5 (1.65, 95% CI = 1.53–1.77) but not mean-ingfully over MMRpro+ (0.98, 95% CI = 0.90–1.04). The Brier scores also remained mostly unchanged. Supplemental Table 4 shows the performance measured using the NRI and log loss. For NRI, GAMs and random forests outperformed PREMM5, suggesting that these combination models reclassified more individuals correctly. For log loss, logistic regression and GAMs outperformed MMRpro+ and PREMM5, suggesting that these combination models have a smaller difference between predicted and expected probability distributions. In comparison to the Brier score, for more extreme predictions that correctly predict outcome status, log loss gives higher rewards; for more extreme predictions that incorrectly predict outcome status, it gives higher penalties. Therefore, it was possible for these combination models to have similar Brier scores as MMRpro+ (Table 4) but outperform it in terms of log loss. Supplemental Figure 1 shows the individual predictions based on the combination models. Some individuals with a pathogenic homozygous or heterozygous LS variant had high scores in either MMRpro+ or PREMM5, and the combined model produced a high score. Similarly, some individuals without a pathogenic homozygous or heterozygous LS variant had low scores in either MMRpro+ or PREMM5, and the combined model produced a low score. In general, these individual-level differences could be attributed to differences in model inputs, modeling assumptions, and training populations.
Table 4
Performance metrics for combinations vs existing models
Model Performance | C-Statistic | Observed to Expected Ratio | Brier Score |
---|---|---|---|
MMRpro+ | 0.83 (0.80–0.86) | 0.98 (0.90–1.04) | 0.15 (0.13–0.17) |
PREMM5 | 0.81 (0.77–0.84) | 1.65 (1.53–1.77) | 0.15 (0.14–0.16) |
LR | 0.83 (0.80–0.86) | 1.00 (0.90–1.09) | 0.13 (0.12–0.14) |
Spline | 0.83 (0.79–0.86) | 0.98 (0.83–1.23) | 0.13 (0.12–0.15) |
RF | 0.78 (0.75–0.82) | 1.00 (0.89–1.10) | 0.15 (0.14–0.16) |
Improvement frequency across Monte Carlo CV replicatesa | |||
MMRpro+ > PREMM5 | 0.910 | 1.000 | 0.560 |
MMRpro+ > LR | 0.450 | 0.530 | 0.000 |
MMRpro+ > Spline | 0.510 | 0.700 | 0.030 |
MMRpro+ > RF | 1.000 | 0.640 | 0.490 |
PREMM5 > LR | 0.020 | 0.000 | 0.000 |
PREMM5 > Spline | 0.030 | 0.000 | 0.020 |
PREMM5 > RF | 0.990 | 0.000 | 0.500 |
LR > Spline | 0.720 | 0.670 | 0.930 |
LR > RF | 1.000 | 0.520 | 1.000 |
Spline > RF | 1.000 | 0.370 | 1.000 |
We consider MMRpro+, PREMM5, and 3 model combination techniques combining MMRpro+ and PREMM5. All metrics are obtained through Monte Carlo CV, with 100 iterations splitting the data into half for training and half for testing. Spline refers to GAM with multivariate smoothing splines.
CV, cross-validation; GAM, generalized additive model; LR, logic regression; MMR, mismatch repair; RF, random forest.
Discussion
This study validated the performance of Leiden, MMRpredict, PREMM5, MMRpro (MMRpro+), and their combination in predicting the probability of an individual having a pathogenic homozygous or heterozygous variant in an MMR gene. For all counselees, MMRpro+ outperformed the other models in discrimination and calibration based on results from the paired analysis. Compared with Leiden and MMRpredict, MMRpro+ and PREMM5 had higher PPVs at the 5% and 2.5% thresholds in outcome probability, respectively, with IFs of >91%. At both thresholds, Leiden had higher NPVs than the other models with IFs of >65%. These results were robust to the inclusion of data from CCFR, which featured a mix of population- and clinicbased ascertainment. Although this study focuses on counselees, it would be interesting to validate the models on relatives if their testing results were available.
This study validated the predictive performance of MMRpro version 2.1–6, which incorporates updated penetrance estimates on the basis of the comprehensive meta-analysis of Wang et al.27 Supplemental Table 5 compares the performance between the original MMRpro model (v2.1–5)15 and the new model with updated penetrance (v2.1–6). Overall, both models had similar calibration. MMRpro (v2.1–5) had better discrimination, and MMRpro (v2.1–6) had higher PPV and NPV. This difference may be attributed to the model’s assumptions because MMRpro (v2.1–5) incorporates penetrance estimates that are the same for individuals with a pathogenic MLH1 variant and those with a pathogenic MSH2 variant. Moreover, among individuals with a pathogenic MSH6 variant, MMRpro (v2.1–5) assumes the same penetrance estimates for males and females, whereas MMRpro (v2.1–6) incorporates gene- and sex-specific penetrance.
Leiden, MMRpredict, and PREMM5 were validated in a number of different cohorts, which is briefly reviewed in this article. The c-statistic for the Leiden model in our cohort agreed with that published by Jasperson et al28 (c-statistic = 0.62 [95% CI = 0.46–0.77]) and was lower than that in the study by Monzon et al20 (c-statistic = 0.90 [95% CI = 0.82–0.97]). The c-statistics for MMRpredict generally agreed with those from another study in clinic-based cohorts.24 Both Leiden and MMRpredict showed better discrimina-tive ability (c-statistics = 0.93 [95% CI = 0.91–0.95] and 0.96 [95% CI = 0.94–0.97], respectively) in a study by Green et al,23 in which both models were validated on a population-based cohort. In general, the c-statistic will be higher in population-based studies because discrimination becomes more difficult when the sample is homogeneous in its risk level. In our study, PREMM5 tended to underpredict the probability of having a pathogenic homozygous or heterozygous LS variant, potentially because of the difference in the covariate distributions between the development cohort and our validation cohort, which had a larger proportion of affected individuals. In comparison to the validation results from Kastrinos et al,16 PREMM5 showed slightly worse performance in this study (0.83 compared with 0.80, with overlapping 95% CIs), possibly because of the smaller size of the validation cohort and less widespread diagnostic testing of PMS2 and EPCAM when the data were collected.
A potential limitation in our study was that families from the 5 participating institutions were generally at high risk for having a pathogenic variant. Typically, individuals from clinic-based cohorts have a strong family history of cancer, making it more difficult to distinguish those with a pathogenic homozygous or heterozygous LS variant from those without. Another limitation was the potential overlap in the data used for estimating and validating the new penetrance estimates in MMRpro for the additional analysis with families from CCFR. Finally, although model combination can provide more accurate prediction models, using an external data set to evaluate the combination models would have been ideal because cross-validation is known to provide overly optimistic estimates of prediction performance.
Completeness of family history information is an important consideration for cancer risk assessment. In practice, completeness may be compromised owing to various reasons, such as health literacy-related barriers, the counselees’ lack of knowledge of their family history, among others. In general, families with incomplete information are less informative and may lead to less accurate predictions by Leiden, MMRpredict, MMRpro (MMRpro+), and PREMM5. In comparison to the other models, MMRpro (MMRpro+) requires more extensive family history. In the context of BRCApro, a Mendelian risk prediction model similar to MMRpro but for pathogenic homozygous or heterozygous variants in BRCA1/BRCA2, it was found that requiring less extensive family history information led to only modest loss in overall discrimination compared with BRCApro.38,39 Another important consideration is whether the training and validation data sets used for each model are comparable in terms of completeness of family history information. A model such as PREMM5, eg, was trained using incomplete pedigrees (eg, it does not require the age of diagnosis for individuals affected with LS-associated cancers). Specifically, in this manuscript, although there may be some incomplete family history in the clinic-based cohorts, the median number of relatives for whom some information was available is 33. Furthermore, the proposed combination model was trained and validated using subsets of the same clinic-based cohorts; therefore, completeness of family history is comparable between the training and validation data sets.
Collection of family history information may be challenging among individuals with low health literacy, which disproportionately affects those who are less educated, elderly, minorities, or have limited English proficiency.40,41 Therefore, it is important to validate models on diverse populations to evaluate potential biases. Recently, MMRpro and PREMM5 were validated on a large Hispanic cohort from the Clinical Cancer Genomics Community Research Network, and it was found, reassuringly, that both models perform well in predicting the probability of having a pathogenic homozygous or heterozygous LS variant, with modest underprediction.42 However, this is only 1 validation example, and further validation studies in underrepresented racial and ethnic populations are crucial.
As multigene panel germline testing is becoming widespread, more associations with CRC are being identified with pathogenic variants across genes. PanelPRO was developed to effectively incorporate these associations into models for quantitative assessment; it is a general, computationally efficient framework for Mendelian risk models that incorporates an arbitrary number of genes and cancers or syndromes.43,44 PanelPRO generalizes MMRpro to the multigene and multicancer setting and can provide more accurate risk assessment for pathogenic homozygous or heterozygous LS variants that may be integrated into broader genetic panels.
Quantitative models such as MMRpro and PREMM5 serve as useful tools for identifying individuals who are at high risk of having an LS pathogenic variant. If unidentified, individuals with a pathogenic homozygous or heterozygous LS variant may miss the opportunity to pursue screening or preventive strategies known to reduce the risk of CRC, such as colonoscopies and prophylactic surgeries. Our study confirms the validity of these models and suggests that further improvement in their use can arise from combining them as we propose. We recommend that clinicians and genetic counselors use these models in an informed manner to better implement effective management and targeted surveillance strategies for individuals with LS.
Acknowledgments
We thank late Henry Lynch for his leadership in the creation of the Creighton University familial registry and his willingness to share data for this project.
This work was supported by National Institutes of Health, United States grants 5P50CA06292420 (F.M.G. and G.P.), 5P30CA006516-54 (G.P.), T32CA009001 (T.H.), 5T32CA009337-40 (C.S.), and R01 CA132829 (S.S.). The Colon Cancer Family Registry (Colon CFR, www.coloncfr.org) is supported in part by funding from the National Cancer Institute, United States, National Institutes of Health (award U01 CA167551). Support for case ascertainment was provided in part from the Surveillance, Epidemiology, and End Results (SEER) Program and the following US state cancer registries: Arizona, Colorado, Minnesota, North Carolina, New Hampshire and by the Victoria Cancer Registry (Australia) and Ontario Cancer Registry (Canada).
The funding organizations had no role in the design and conduct of the study; collection, management, analysis, and interpretation of the data; and preparation, review, or approval of the manuscript.
Footnotes
Ethics Declaration
The Dana-Farber Cancer Institute Institutional Review Board determined that this work is not research involving human subjects as defined by Department Of Health And Human Services and US Food and Drug Administration regulations. Institutional Review Board review and approval by this organization is not required. The data used in this study were de-identified.
Conflict of Interest
G.P. is a cofounder and equity holder in Phaeno Inc., a member of the Scientific Advisory Board of Konica Minolta Precision Medicine, Inc (which includes Ambry Genetics and Invicro), and a consultant for Delfi Diagnostics and Foundation Medicine, Inc.
D.B. and G.P. colead the BayesMendel lab, which develops and maintains the BayesMendel software package. This includes a variety of risk assessment tools including BRCAPRO, PancPRO, MelaPRO, MMRpro, and PanelPRO and is licensed for commercial use. All licensing revenues are used for software maintenance and upgrades. Neither BayesMendel lab leaders nor members derive personal income from BayesMendel licenses. D.B. and G.P. are coinventor of the Ask2me tool, which is commercially licensed. D.B.’s conflicts of interest are managed by Harvard T.H. Chan School of Public Health.
S.S. has been a consultant for Myriad Genetics, Inc and has rights to the inventor portion of licensing revenues for the PREMM model.
Z.K.S.’s immediate family member serves as a consultant in Ophthalmology for Alcon, Adverum Biotechnologies, Gyroscope Therapeutics Limited, Neurogene Inc, and REGENXBIO Inc, outside the submitted work. All other authors declare no conflicts of interest.
Additional Information
The online version of this article (https://doi.org/10.1016/j.gim.2022.07.004) contains supplementary material, which is available to authorized users.
Data Availability
Policies on data sharing vary by institution. To access data used in the main analysis, please reach out to the following coauthors: F.M.G. for data from Johns Hopkins University, P.M.L. for data from MD Anderson Cancer Center, K.N., Z.K.S., or K.O. for data from Memorial Sloan Kettering Cancer Center, C.U. or S.S. for data from Dana-Farber Cancer Institute, and email ude.nothgierc@retnecrecnacyratidereh for data from Creighton University. To access data from the Colon Cancer Family Registry, please see the data sharing guidelines at https://www.coloncfr.org/data-sharing
References
Citations & impact
This article has not been cited yet.
Impact metrics
Alternative metrics

Discover the attention surrounding your research
https://www.altmetric.com/details/134896025
Similar Articles
To arrive at the top five similar articles we use a word-weighted algorithm to compare words from the Title and Abstract of each citation.
Performance evaluation of predictive models for detecting MMR gene mutations associated with Lynch syndrome in cancer patients in a Chinese cohort in Taiwan.
Int J Cancer, 155(12):2201-2210, 20 Jul 2024
Cited by: 0 articles | PMID: 39032036
Development and Validation of the PREMM5 Model for Comprehensive Risk Assessment of Lynch Syndrome.
J Clin Oncol, 35(19):2165-2172, 10 May 2017
Cited by: 81 articles | PMID: 28489507 | PMCID: PMC5493047
Prediction of germline mutations and cancer risk in the Lynch syndrome.
JAMA, 296(12):1479-1487, 01 Sep 2006
Cited by: 214 articles | PMID: 17003396 | PMCID: PMC2538673
Criteria and prediction models for mismatch repair gene mutations: a review.
J Med Genet, 50(12):785-793, 16 Aug 2013
Cited by: 16 articles | PMID: 23956446
Review
Funding
Funders who supported this work.
NCI NIH HHS (8)
Grant ID: P50 CA062924
Grant ID: U01 CA167551
Grant ID: P30 CA006516
Grant ID: P30 CA008748
Grant ID: T32 CA009001
Grant ID: P50 CA221745
Grant ID: R01 CA132829
Grant ID: T32 CA009337