Abstract
Free full text

Auditory stimulation and deep learning predict awakening from coma after cardiac arrest
Abstract
Assessing the integrity of neural functions in coma after cardiac arrest remains an open challenge. Prognostication of coma outcome relies mainly on visual expert scoring of physiological signals, which is prone to subjectivity and leaves a considerable number of patients in a ‘grey zone’, with uncertain prognosis. Quantitative analysis of EEG responses to auditory stimuli can provide a window into neural functions in coma and information about patients’ chances of awakening. However, responses to standardized auditory stimulation are far from being used in a clinical routine due to heterogeneous and cumbersome protocols. Here, we hypothesize that convolutional neural networks can assist in extracting interpretable patterns of EEG responses to auditory stimuli during the first day of coma that are predictive of patients’ chances of awakening and survival at 3 months. We used convolutional neural networks (CNNs) to model single-trial EEG responses to auditory stimuli in the first day of coma, under standardized sedation and targeted temperature management, in a multicentre and multiprotocol patient cohort and predict outcome at 3 months. The use of CNNs resulted in a positive predictive power for predicting awakening of 0.83 ± 0.04 and 0.81 ± 0.06 and an area under the curve in predicting outcome of 0.69 ± 0.05 and 0.70 ± 0.05, for patients undergoing therapeutic hypothermia and normothermia, respectively. These results also persisted in a subset of patients that were in a clinical ‘grey zone’. The network’s confidence in predicting outcome was based on interpretable features: it strongly correlated to the neural synchrony and complexity of EEG responses and was modulated by independent clinical evaluations, such as the EEG reactivity, background burst-suppression or motor responses. Our results highlight the strong potential of interpretable deep learning algorithms in combination with auditory stimulation to improve prognostication of coma outcome.
Introduction
Most survivors of cardiac arrest are initially in a coma. Outcome prognostication has become an integral part of post-resuscitation care.1,2 Currently used outcome prediction techniques mainly rely on expert multimodal assessments of clinical variables and physiological signals1 like EEG, which is routinely used to evaluate the integrity of neural functions at the patients’ bedside. EEG evaluations consist of visual assessments, which can be time-consuming and prone to subjectivity.3 In addition, current clinical markers for outcome prognostication are unable to provide a clear prognosis for a considerable proportion of patients, classifying them as indeterminate, or part of a ‘grey zone’,2 and highlighting a clear need for developing novel markers of outcome.
A putative marker for assessing the integrity of neural functions in coma patients are EEG responses to auditory stimulation.4–7 Auditory event-related potentials (ERPs) have been previously linked to chances of awakening from coma,4–7 but standardized ERPs, assessed in a quantitative way, are not routinely used for outcome prognosis.8 Typically, auditory ERPs are evaluated by averaging hundreds of EEG responses to the same standardized auditory stimuli and by extracting aggregate characteristics, such as the presence or absence of characteristic ERP deflections, like the N100,9,10 their amplitude or latency9 or differential responses to sequences of standard and deviant sounds.9,11–13 These approaches have the disadvantage that the features used to predict coma outcome are selected a priori at an average ERP level, overlooking the richness of EEG responses and neglecting potentially important characteristics, thus likely leading to unreliable prognosis.
More recent attempts to explore the prognostic value of auditory stimulation consist of modelling single-trial EEG responses to sounds, with the use of machine learning techniques to extract patient-specific EEG patterns that quantify auditory discrimination.14,15 These studies have shown that the progression of auditory discrimination from first to second day of coma is informative of patients’ chances of awakening.15–17 Moreover, the neural synchrony across voltage measurements of auditory EEG responses in the first day of coma is also predictive of awakening, further corroborating the early prognostic value of auditory ERPs.18 However, despite clear links between auditory processing in coma and patients’ outcome, shown over multiple studies and approaches, standardized auditory stimulation is not currently used in the clinical routine as a prognostic marker. A major limitation for this discrepancy is that existing studies report findings either in small patient cohorts with highly curated features (i.e. average EEG responses over predefined time windows and specific electrodes) and limited predictive power6,7,9,12,13 or require two EEG recordings over two consecutive days.14–17 In order to fully exploit the multidimensional ERP features and their relevance to coma outcome, there is a critical need to assess EEG responses to auditory stimulation in a more robust and straightforward way.
In recent years, advances in the field of machine learning have given rise to powerful tools for modelling brain signals.19,20 Convolutional neural networks (CNNs) are particularly promising in extracting in a data-driven way rich features of EEG data, and have been shown to outperform traditional techniques.19–22 Despite their huge potential, the use of CNNs in acute neuro-critical prognostication remains limited. One challenge in using CNNs in medical applications is that it is difficult to trace which features of the EEG data are relevant for the decisions that CNNs are making.22 The very few studies that have used CNNs to predict outcome from coma rely on the same continuous EEG recordings of resting state activity that are used in the clinics via visual evaluations,23–26 and have shown a remarkable precision in discriminating patients who later survive from those who do not. It remains unknown whether CNNs can be applied on EEG responses to standardized auditory stimuli to assist in outcome prognosis and to provide additional insights for those patients for whom existing clinical assessments do not result in a conclusive prognostication.
Here, we made the hypothesis that CNNs would be able to extract patterns of EEG responses to standardized auditory stimuli that relate to patients’ chances of awakening from coma and survival at 3 months. We additionally hypothesized that the outcome prediction of CNNs would be complementary to currently used clinical variables for prognostication and would have the potential to improve prognosis for patients with indeterminate prognosis. Last, we performed exploratory analyses to identify which features of the EEG data are relevant for the outcome prediction provided by the CNNs. We extracted measures of confidence of the network’s decisions and linked those to (i) clinical variables currently used for outcome prognosis27; and (ii) features of EEG responses to sounds that quantify neural synchrony, which have been recently shown to be informative of patients’ outcome.1,18,27 To this aim, we analysed EEG responses to auditory stimulation during the first day of coma, recorded in a multicentre and multiprotocol cohort of coma patients following cardiac arrest at four different hospitals.28
Materials and methods
Patients and procedure
We recorded data from a cohort of 145 comatose patients following cardiac arrest (33 females, 63.3 ± 1.2 years old, mean ± SE), admitted to the intensive care units of the University Hospitals Lausanne (121 patients), Bern (18 patients), Sion (four patients) and Fribourg (two patients) between December 2009 and April 2017. Patients have been previously described.16–18 Informed written consent was obtained prior to EEG recordings from a family member, legal representative or treating clinician not involved in this study. The ethical committees of the Cantons of Bern, Fribourg, Valais and Vaud, Switzerland approved the experimental protocol.
Upon admission to the hospital, patients were in an acute coma, i.e. a score of <6 on the Glasgow Coma Scale (GCS) and treated with targeted temperature management for 24 h; 79 patients were treated with targeted temperature management at 33°C (therapeutic hypothermia; HT) and 55 patients at 36°C (normothermia; NT). Controlled temperature treatment was based on ice packs or intravenous ice-cold fluids together with a feedback-controlled cooling device (Arctic Sun System, Medivance or Thermogard XP; ZOLL Medical), for 24 h after cardiac arrest and was subsequently removed. Propofol (2–3 mg/kg/h), midazolam (0.1 mg/kg/h) and fentanyl (1.5 lg/kg/h) were administered for analgesia and sedation, with vecuronium, rocuronium or atracurium for controlling shivering.
Decisions to withdraw clinical care were based on a multidisciplinary approach.1 Namely, care was withdrawn if two or more of the following criteria were present at 72 h or more, after sedation weaning29,30: unreactive background EEG; epileptiform EEG and/or myoclonous that was resistant to treatment; incomplete return of brainstem reflexes; bilateral absence of N20 in somatosensory evoked potentials; the concomitant presence of major hypoxic/ischaemic lesions in structural MRI and neuron-specific enolase levels more than twice above 75 μg/l were additionally considered.31 Importantly, all clinical decisions were blinded to the output of the neural networks.
Patients’ outcome was defined at 3 months after cardiac arrest via a semistructured phone interview via the Cerebral Performance Category32 (CPC). A CPC of 1 indicates full recovery; of 2 return of consciousness with moderate disability; a CPC of 3 return of consciousness with severe disability; while a CPC of 4 coma or persistent vegetative state; and a CPC of 5 death. For our analyses we considered patients with CPC 1–3 at 3 months after coma onset as 'survivors' (n = 79). Patients with a CPC of 5 were considered as patients with poor outcome ('non-survivors', n = 55). In our cohort, no patient was classified with a CPC of 4, possibly due to the clinical practices in the participating hospitals, where the decision to withdraw life-sustaining treatment for patients who fail to regain consciousness is regularly reassessed even after the acute phase.
In accordance with previous investigations focusing on prediction of outcome in coma patients following cardiac arrest,17 we did not analyse patients who regained consciousness during their stay at the hospital but later died, for example because of other comorbidities. This resulted in the exclusion of 11 patients, resulting in a cohort of 134 patients in total.
Auditory stimulation protocol
EEG recordings were conducted at the patients’ bedside, within 24 h after cardiac arrest while all patients were in a comatose state. Patients were presented with a series of pure tones as previously described.15–17 Tones consisted of 16-bit stereo sounds, sampled at 44.1 kHz, with a 10 ms linear amplitude envelope applied at stimulus onset and offset to avoid clicks. Between each sound, there was a 700 ms interstimulus interval. Standard sounds were presented in 70% of the trials and had a pitch of 1000 Hz and duration of 100 ms. Deviant sounds differed from the standards in duration (150 ms), interaural time difference, with left ear leading with 700 μs, or pitch at 1200 Hz. Stimuli were presented in a pseudo-randomized order, in a way that at least one standard sound was presented between two deviant ones. The auditory stimulation protocol consisted in total of 1500 tones, split into three blocks, each lasting ~7 min. Similar to a previous study investigating neural properties of auditory processing in coma,18 in the present study we focused on responses to standard and duration deviant sounds, as they have been previously shown to be highly informative of coma outcome.15,18 A detailed evaluation of the predictive value of EEG responses to all sound types is provided in the Supplementary material.
Recording setup and preprocessing
As the data of this study were multicentre and multiprotocol, EEG was recorded at the patient's bedside with 19 or 62 electrodes, depending on the original study design,15–17 positioned according to the international 10–20 system. EEG data were collected with a sampling frequency of either 1000 or 1200 Hz. For consistency, data recorded with 1200 Hz were downsampled to 1000 Hz and data recorded with 62 electrodes were downsampled to the overlapping set of 19 electrodes. Across all channels the impedance was kept below 10 kΩ. The online reference for the electrodes was set as Fpz. In the course of preprocessing electrodes were re-referenced to a common average reference. Epochs were extracted from 50 ms before stimulus onset to 500 ms after. Artefacts were rejected with a criterion of ±100 μV on all electrodes. Noisy electrodes were interpolated using three-dimensional splines.33 After re-referencing, the data were filtered from 0.1 to 40 Hz, and in a control analysis, to ensure that our findings are not driven by muscle activity, from 0.1 to 20 Hz. Additionally, the EEG epochs were visually inspected and noisy epochs were manually removed. After preprocessing, we obtained 347.22 ± 9.23 trials (mean ± SE) per patient, 204.76 ± 8.66 in response to standard sounds and 142.46 ± 1.42 in response to duration deviants.
Training of convolutional neural network
For training the neural network we adopted a 10-fold cross-validation procedure, i.e. we split the patients 10 times into 3 different sets of train, validation and test patients, in a way that data from each patient were included in only one of the three sets. The train set had 60% of patients (80 patients), the validation set 20% (27 patients) and the test set 20% (27 patients). The single trials of all patients from the train set were used to train the neural network and the trials from the validation set were used to evaluate any hyperparameters (e.g. learning rate, early stopping, etc.). The test set was used only after training and optimizing the network, for an objective evaluation of the model’s performance on unseen patients.
We trained a CNN called EEGNet21 to predict patients’ outcomes, which has been designed specifically for EEG data. The overall network size is moderate, giving smaller training times while still achieving robust results. The original network architecture was changed slightly for the purpose of our study. First, according to the recommendations as in the original study21 the filter length of the first convolutional layer was changed to 512, instead of the original 64. Consequently, the number of temporal, spatial and pointwise filters were increased to 16, 4 and 64, respectively, while the activation function was set to ReLu. These changes were made as preliminary analysis on a small subset of patients showed a more stable training.
For optimizing the model we used the binary cross-entropy loss function and the Adaptive Moment Estimation (Adam),34 with a learning rate of 5 × 10−6. All other parameters were unchanged from the default suggestions. We trained the network for a maximum of 100 epochs, but employed early stopping after 30 epochs if the validation loss of two consecutive epochs was smaller than a threshold, as is standard practice in the field.35 To evaluate the network performance we additionally used the area under the curve (AUC).36
Outcome prediction based on network’s output
As the neural network was trained on single-trial EEG responses to sounds (Fig. 1), it classified single-trial EEG responses as belonging to a survivor versus a non-survivor. To link the network’s output to coma outcome at the single patient level, for each patient we computed the mean classification performance across all trials, which we term ‘confidence of predicting survival’. The confidence score ranged between zero and one and indicated the confidence of the model’s prediction of coma outcome, as the mean label that the model assigned across single-trial EEG responses to sounds of a given patient. If that score was above 0.5, then a patient was classified as a survivor, while below 0.5 as a non-survivor, as per convention based on a sigmoid function (Fig. 1).
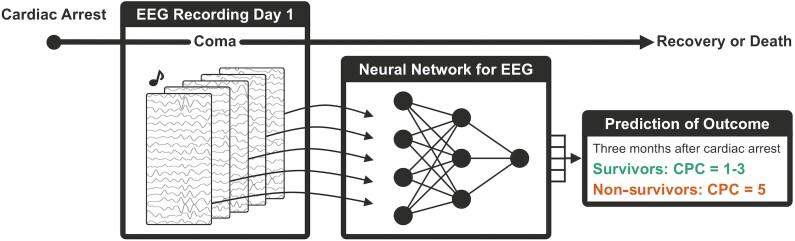
Schematic description of the deep learning algorithm for predicting outcome from coma. Auditory stimulation and EEG recordings are performed on the first day of coma, shortly after a cardiac arrest. Single-trial EEG responses are then given as input to a CNN which classifies them as belonging to a survivor versus a non-survivor. The average of all single-trial predictions provides the network’s confidence of predicting survival. Survival is defined at 3 months via the CPC score.
For evaluating the network’s performance, for all metrics we report mean ± SE values obtained across the 10 folds of cross-validation. We additionally plot the network’s output in relation to patients’ outcome for the best model, defined as the one with the highest AUC score of the validation set. The reported final results remain objective, as they concern the test set of patients which was not used to train, optimize or select the best model. We additionally evaluated the positive and negative predictive values (PPV and NPV, respectively), as the ratio of correctly predicted survivors among all predicted survivors (PPV) or correctly predicted non-survivors among all predicted non-survivors (NPV).
Outcome prediction in patients with indeterminate prognosis
To assess the additional utility of our method for the current clinical practice, we evaluated the network’s predictions for a subset of coma patients whose outcome prognosis was inconclusive based on existing clinical tests. Based on previous literature and recommendations, we considered the motor response, EEG reactivity, EEG continuity and brainstem reflexes.1,2 If the above-mentioned variables showed a discrepancy (e.g. present motor response and brainstem reflexes, but a discontinuous and irritative EEG), a patient was defined as being in a ‘grey zone’. For this definition, we did not include patients where only brainstem reflex was present and all other variables predicted a negative outcome, due to the low positive predictive power of brainstem reflex for good outcome.1 This resulted in 48 patients fulfilling the criteria for a clinical ‘grey zone’ (32 survivors, 16 non-survivors). Because of their relatively low number, for this analysis we merged patients from train, validation and test sets, and examined the overall distribution of the outcome prediction resulting from the CNN. Moreover, we performed two control analyses to further evaluate the generalizability of the network on ‘grey zone’ patients. First, we curated this split of patients to train, validation and test sets, to ensure that a fixed but high number of ‘grey zone’ patients are part of the test set (Supplementary material). Second, we trained one network using exclusively patients with determinate outcomes for training and validation, and kept all ‘grey zone’ patients as a test set, to evaluate generalization of outcome prediction results (Supplementary material).
Exploring links between network’s output and electrophysiological features of EEG responses
To explore the features of EEG responses related to the network’s decisions, we explored (i) measures of neural synchrony and complexity, previously shown to relate to patients’ outcome and presence of consciousness, respectively18; and (ii) well established clinical variables currently used for outcome prognosis.1,2
We first computed the phase-locking value (PLV), which quantifies the synchrony of EEG responses to auditory stimuli.37 The PLV was computed for electrode pairs in the alpha range, which has been recently shown to be predictive of patients’ outcome in two different cohorts of patients undergoing HT.18 Here, we calculated the mean PLV per patient across electrode pairs, applying the same procedure as previously reported, based on a subset of patients included in the present study.18 The neural complexity was quantified via the Lempel–Ziv (LZ) complexity, which measures the number of unique patterns present in a signal.38 Both PLV and LZ were calculated on single-trial EEG responses, similar to the neural network, to capture trial-by-trial characteristics of EEG responses to the auditory stimuli. Here, we expanded the analysis that was recently reported18 for both measures on a larger patient cohort. Our goal on the one hand was to link these measures to the network’s output (via Pearson correlation coefficient, Pcorr < 0.01, Bonferoni corrected) and on the other hand, to validate these measures in patients treated with targeted temperature management at 36°C, as they were previously only reported for patients treated at 33°C. We excluded two patients that were part of a previous study,18 because they awoke in the hospital but subsequently died before the CPC assessment at 3 months (see the 'Patients and procedure' section).
Second, we compared predictions of the network in subgroups of patients defined according to the following binary markers: presence of brainstem reflexes (pupil and corneal reflexes present), presence of motor response (motor GCS ≥ 4), reactive EEG background (change in amplitude and/or frequency after stimulus, judged visually), discontinuous or suppressed EEG background39 and irritative EEG (presence of electrographic seizures or status epilepticus, sporadic epileptiform discharges, spiky or sharp periodic discharges or rhythmic spike waves), using Mann–Whitney tests (Pcorr < 0.01), and Bonferroni correction for multiple comparisons. We additionally explored correlations between the network’s confidence and time to return of spontaneous circulation (ROSC), with a Pearson correlation coefficient, as well as links between confidence and hospital site or CPC at 3 months with Kruskal–Wallis H-tests.
Data availability
Because of the sensitive nature of the clinical data, data and materials can be made available from the corresponding authors upon reasonable request.
Results
Outcome of coma patients
Of the 134 patients analysed, 79 (59%) survived, where survival was defined as CPC 1 (46 patients), 2 (22 patients) and 3 (11 patients) at 3 months. Of 134 patients, 55 (41%) had a poor outcome, corresponding to a CPC of 5, and no patient was in a vegetative state (CPC of 4).
Prediction of outcome based on the neural network
The neural networks trained to discriminate auditory EEG responses of patients that later survived from those who did not reached a mean AUC score of 0.81 ± 0.00 on the train, 0.75 ± 0.03 on the validation and 0.70 ± 0.04 on the test sets (Fig. 2 and Table 1). On the test set we obtained a PPV of 0.83 ± 0.03 and an NPV of 0.57 ± 0.04 (Table 1). For patients treated with targeted temperature management at 33°C, the average PPV was 0.83 ± 0.04 and for those treated at 36°C 0.81 ± 0.06. The difference in PPV over the two treatments over the 10 cross-validation folds was not significant (P = 0.67, Wilcoxon signed rank test), implying that the networks performed at similar levels for patients treated with targeted temperature management at 33°C and 36°C. The AUC scores were also replicated with a control analysis where the neural networks were trained with EEG data filtered between 0.1 and 20 Hz, resulting in a mean AUC score of 0.74 ± 0.03, PPV of 0.86 ± 0.02 and NPV of 0.62 ± 0.03 on the test set.
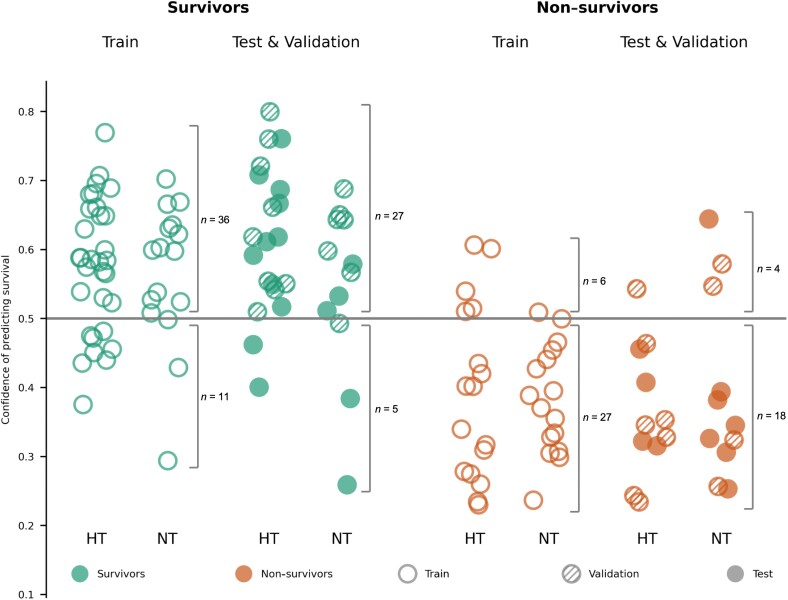
Prediction of outcome based on the CNN. Confidence scores were computed for each patient by averaging the network’s outcome prediction for all single-trial EEG responses to sounds of a given patient. A patient was predicted to be a survivor if the confidence score was above 0.5, otherwise a non-survivor. Data from patients of the train set (empty circles) were used to train the network, while data from patients in the validation set (shaded circles) were used to evaluate hyperparameters. The predictive value of the network was evaluated on a separate test set of patients (full circles), whose data were never used to train or optimize the network. For each of the sets, we split patients into HT (hypothermia, targeted temperature management at 33°C) and NT (patients with targeted temperature management at 36°C). For the numerical performance scores see Table 1.
Table 1
Prediction of outcome, PPV and NPV for awakening based on the neural network
All | Hypothermia | Normothermia | |
---|---|---|---|
Mean over 10 folds | |||
AUC train | 0.81 ± 0.00 | 0.81 ± 0.01 | 0.80 ± 0.01 |
AUC validation | 0.75 ± 0.03 | 0.72 ± 0.04 | 0.72 ± 0.05 |
AUC test | 0.70 ± 0.04 | 0.69 ± 0.05 | 0.70 ± 0.05 |
PPV train | 0.90 ± 0.01 | 0.92 ± 0.02 | 0.87 ± 0.01 |
PPV validation | 0.85 ± 0.02 | 0.86 ± 0.02 | 0.71 ± 0.10 |
PPV test | 0.83 ± 0.03 | 0.83 ± 0.04 | 0.81 ± 0.06 |
NPV train | 0.70 ± 0.01 | 0.65 ± 0.02 | 0.70 ± 0.02 |
NPV validation | 0.66 ± 0.04 | 0.56 ± 0.08 | 0.66 ± 0.06 |
NPV test | 0.57 ± 0.04 | 0.53 ± 0.05 | 0.57 ± 0.05 |
Best fold | |||
AUC train | 0.79 | 0.72 | 0.88 |
AUC validation | 0.83 | 0.93 | 0.68 |
AUC test | 0.83 | 0.91 | 0.73 |
PPV train | 0.86 | 0.82 | 0.93 |
PPV validation | 0.83 | 0.90 | 0.75 |
PPV test | 0.92 | 1.00 | 0.75 |
NPV train | 0.71 | 0.60 | 0.83 |
NPV validation | 0.89 | 1.00 | 0.67 |
NPV test | 0.71 | 0.67 | 0.75 |
Prediction of outcome of the trained neural network. We report the mean ± SE over all 10 trained folds, as well as the performance of the best fold. We report the AUC, PPV and NPV, with respect to survival, of the train, validation and test sets, for all patients and separately the subcohorts of patients treated with hypothermia and NT. The performance of the best fold is also visualized in Fig. 2.
We next focused on one single fold of the cross-validation, and evaluated the confidence scores assigned by the network to individual patients (Fig. 2), as our goal was to investigate the neural properties of EEG signals that may mediate the network’s outcome prediction. In the validation and test sets, we obtained a sensitivity of 84% for survivors and a specificity of 82% for non-survivors. Out of all the patients classified as survivors in the train set (n = 42 patients), 36 awoke from coma, resulting in a PPV of 86%, while in the validation/test sets 27 of 31 patients that were predicted as survivors awoke, resulting in a PPV of 87%. These results were largely similar for patients treated with different temperature treatments (Fig. 2 HT, NT and Table 1).
Outcome prediction for patients in a ‘grey zone’
We next focused on patients who, from a clinical viewpoint, were part of a ‘grey zone’, i.e. cases where currently used outcome predictors indicated indeterminate outcome. Forty-eight patients fulfilled these criteria (n = 32 survivors and n = 16 non-survivors, Fig. 3). The distribution of confidence values assigned by the network in this subset of patients followed the distribution of the full cohort (Figs 2 and and3).3). For this subset of patients we obtained a PPV of 0.86, an NPV of 0.60 and an AUC score of 0.75, based on the CNN. These scores were at similar levels as those obtained with the full cohort, suggesting that although this group of patients had indeterminate prognosis based on existing clinical tests, they were not ‘peculiar’ cases for the neural network (see the Supplementary material for additional analyses on 'grey zone' patients).
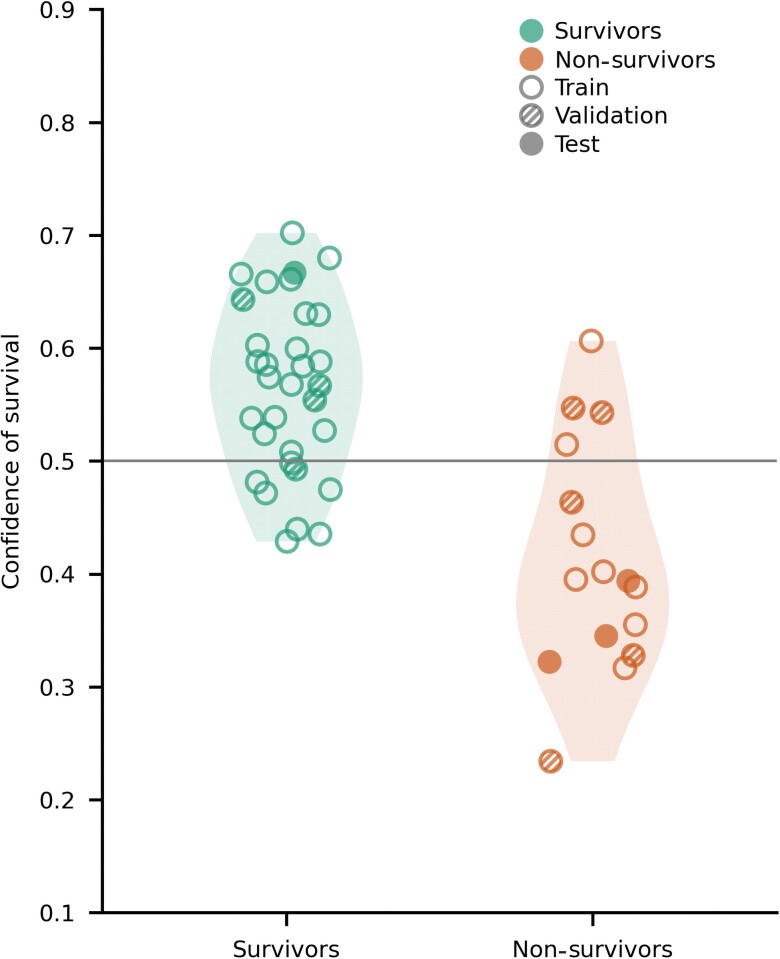
Confidence of survival assigned by the network for patients with uncertain outcome prognosis based on existing outcome predictors. Confidence scores for patients in this subset followed the distribution of confidence scores in the entire patient cohort (Fig. 2). The empty circles show patients in the train set, shaded circles patients in the validation and full circles patients in the test set. The left side of the figure represents survivors and the right side non-survivors.
Investigating the interpretability of the neural network’s outcome prediction
We next evaluated the electrophysiological properties of EEG signals that the network’s output may be reflecting while providing a prediction about patients’ outcome. We first evaluated the PLV of EEG responses to sounds, previously shown to be predictive of patients’ outcome when treated with hypothermia.18 Here, we first replicated these results for patients in NT. The mean PLV for survivors treated with hypothermia was 0.75 ± 0.01 and for survivors treated with normothermia 0.72 ± 0.02. For non-survivors, we found a mean PLV of 0.55 ± 0.02 and 0.57 ± 0.03 for patients treated with hypothermia and NT, respectively. When statistically tested, we found a significant main effect of outcome on PLV (F = 101.68; Pcorr < 0.01), while neither the main effect of temperature treatment (F = 4.07; Pcorr = 0.046) nor the outcome by temperature interaction were significant (F = 2.17; P = 0.14). Next, we explored the predictive power of the PLV in patients treated with NT, as done previously for the subset of patients treated with hypothermia (partially overlapping with those included in our previous study18). For patients in hypothermia, the PLV provided a PPV of 0.85 and an NPV of 0.83, as previously reported.18 For patients in NT, the PLV resulted in a PPV of 0.77 and an NPV of 0.80 (Fig. 4A).
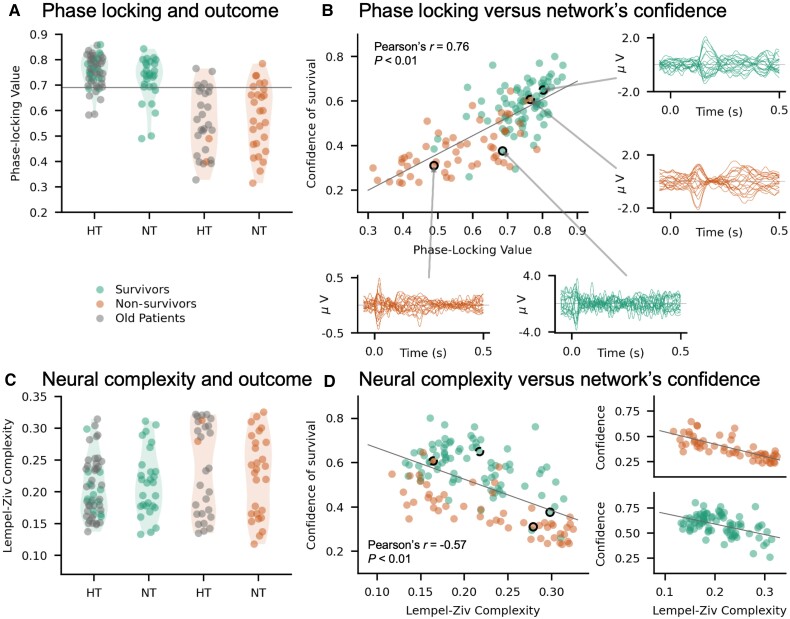
Investigating the interpretability of the neural network’s output. (A) PLV for survivors (left) and non-survivors (right). The horizontal line corresponds to a threshold PLV of 0.69, identified and already evaluated in a subset of patients, previously reported in Alnes et al.18 The previously reported distribution of PLV values was replicated in a new cohort, predominantly treated with NT. (B) Correlation of network confidence to PLV. The network’s confidence of survival was strongly correlated to the average PLV (Pearson’s r = 0.76; Pcorr < 0.01). Exemplar EEG responses to sounds have been plotted for two survivors and two non-survivors, one correctly and one incorrectly classified based on the neural network. (C) LZ complexity of auditory ERPs for each patient. As previously reported in a subset of patients,18 LZ complexity was not informative of patients’ outcome. (D) Correlation between LZ complexity and the network’s confidence of survival for the entire patient cohort (r = −0.53; Pcorr < 0.01). The correlation values are also plotted separately for survivors (bottom plot) and non-survivors (top plot). The highlighted circles with borders mark the patients whose exemplar EEG responses are shown in B.
Importantly, the confidence of survival assigned by the network to each patient strongly correlated with the mean PLV across electrodes (r = 0.76; Pcorr < 0.01; Fig. 4B). This correlation was not trivially driven by the fact that both measures predict outcome, as it remained significant when tested for survivors (r = 0.49; Pcorr < 0.01) and non-survivors (r = 0.65; Pcorr < 0.01) separately.
Next, we computed the LZ complexity of EEG responses to sounds, which by itself has been shown not to be predictive of outcome when only including patients treated at 33°C.18 We confirmed this previous finding for patients of the present study who were treated at 36°C, whose distribution of LZ values was similar to the distribution of LZ values for patients treated at 33°C (Fig. 4C).
Interestingly, although LZ complexity by itself was not predictive of outcome, it showed a significant negative correlation with the network’s confidence (r = −0.57; Pcorr < 0.01; Fig. 4D), so that the higher the network’s confidence in predicting survival, the lower the complexity of EEG responses to sounds. A strong correlation between network’s confidence and EEG complexity was also observed for survivors (r = −0.48; Pcorr < 0.01) and non-survivors (r = −0.76; Pcorr < 0.01) separately. PLV and LZ complexity were also negatively correlated, albeit with a weaker correlation than each of these measures did with the confidence of the neural network (Pearson’s r = −0.39; Pcorr < 0.01).
Overall, these results suggest that the network assigned higher confidence scores for awakening for patients with high PLV and low complexity, or in other words, with stronger neural synchrony and temporal structure in their EEG responses. Exemplar EEG traces of these responses for a correctly classified survivor and a misclassified non-survivor show rather smooth EEG responses to the sounds, compared to exemplar traces of a correctly classified non-survivor and misclassified survivor, where the EEG responses are more ‘stochastic’ (Fig. 4B).
Comparison with clinical variables
Lastly, we compared the confidence of the network’s prediction with clinical variables currently used for outcome prognosis. We found a significant difference (Pcorr < 0.01; Mann–Whitney U-test) in the network’s confidence values between patients with and without presence of brainstem reflex, motor response, reactive EEG background, discontinuous or suppressed EEG background and irritative EEG (Fig. 5A–E). Patients with brainstem reflex, motor response or reactive EEG, all of which are considered indicators of good outcome, had significantly higher confidence scores compared to patients without (Fig. 5A–C). The opposite was observed for patients with discontinuous or irritative EEG, which are considered indicators of poor outcome (Fig. 5D and andE).E). Interestingly, EEG reactivity, which has a prognostic value for good outcome,27 provided similar levels of predicting awakening as the neural network (PPV = 0.88). It is worth noting, however, that the prognostic performance of EEG reactivity is likely biased, as this score is used in the clinical interventions to influence outcome.
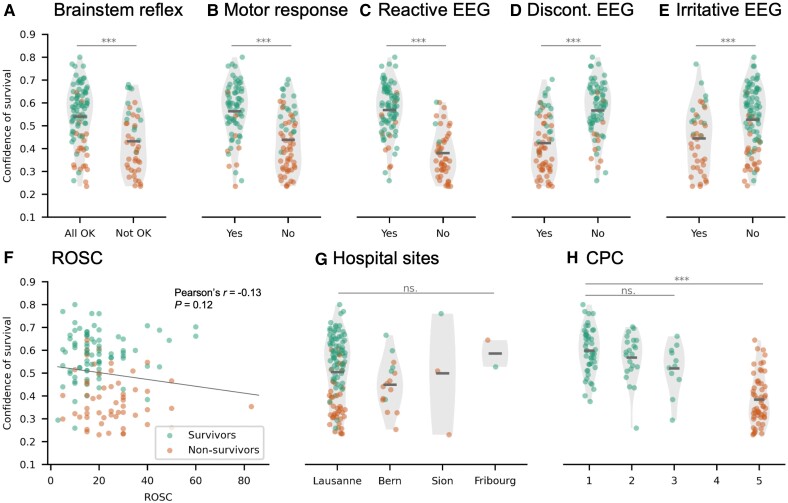
Links between network’s confidence in predicting survival and clinical variables currently used for outcome prognosis. The network’s confidence scores were statistically compared for patients with and without: (A) brainstem reflex (Pcorr < 0.01); (B) motor response (Pcorr < 0.01); (C) reactive EEG (Pcorr < 0.01); (D) discontinuous EEG (Pcorr < 0.01); and (E) irritative EEG (Pcorr < 0.01). (F) Correlation of the network’s confidence of survival and time to return of spontaneous circulation (ROSC, r = −0.13; P = 0.12). (G) Absence of link between hospital sites and the network’s confidence scores (H = 3.48; P = 0.32). (H) Differences in the network’s confidence scores across CPC outcomes. The main effect of CPC was significant when considering all outcomes (CPC 1, 2, 3, 5) (H = 61.90; Pcorr < 0.01), but not within the group of survivors (CPC 1–3, H = 4.36; P = 0.11).
Last, the network’s confidence did not correlate with ROSC (r = −0.13; P = 0.12, Fig. 5F), while no significant difference was found in confidence scores across the four hospital sites (Kruskal–Wallis, H = 3.48; P = 0.32, Fig. 5G). As expected based on the outcome prediction results, there was a main effect of CPC on network’s confidence when testing for CPC 1–5 (Kruskal–Wallis, H = 61.91; Pcorr < 0.01; Fig. 5H). However, there was no significant difference of confidence within the survivors group, for CPC 1, 2 and 3 (Kruskal–Wallis, H = 4.35; P = 0.11).
Discussion
We studied the prognostic value of EEG responses to auditory stimulation, combined with deep learning in predicting coma outcome after cardiac arrest. We showed that CNNs are powerful in extracting single-trial information from auditory ERPs on the first day of coma, and at predicting survival 3 months later, with a positive predictive power of 0.83 ± 0.03, negative predictive power of 0.57 ± 0.04 and an overall AUC of 0.70 ± 0.04. These results were not available to clinicians treating the patients and did not influence patients’ outcome. Predicting patients’ chances of awakening was at similar levels for patients receiving targeted temperature management at 33 and 36°C. The performance of the neural network was separately evaluated on patients in a ‘grey zone’, where clinical variables gave inconclusive results, reaching a PPV of 0.86, suggesting that neural networks might have the potential to assist in prognostication in these currently indeterminate cases. Lastly, we showed that the confidence scores of the neural network in predicting survival were strongly correlated to the phase locking and complexity of auditory EEG responses to the auditory stimuli, so that patients that were confidently characterized as survivors had high synchrony and low complexity in their neural responses.
Auditory stimulation for predicting outcome from coma
The main novelty and advantages of our approach of combining auditory stimulation with deep learning to predict coma outcome are 3-fold: (i) it is semi-automatic, based on a single EEG recording performed within 24 h after cardiac arrest and, if confirmed in other data sets, can be objectively used to assist in predicting patients’ chances of awakening from coma; (ii) it relies on the auditory pathway, which is currently not actively used in the clinical routine for outcome prediction, and can therefore provide additional clinical insights for patients in a ‘grey zone’, whose outcome is indeterminate based on existing techniques; and (iii) the output of the neural network is not a simple binary prediction of outcome, but exploits a continuum of confidence values, which are then directly linked and strongly correlated to interpretable features of EEG responses.
Our work follows a large body of literature showing links between neural responses to auditory stimulation in comatose or unresponsive patients and patients’ outcome.4–7 Standardized auditory stimulation in particular has been proposed to be informative of patients’ chances of awakening.9–13 However, to date it is not regularly used in the clinical routine, as the majority of existing techniques are not robust enough, rendering a clinical implementation challenging.15–17 Here, we overcame this challenge by focusing on one single EEG recording of ~20 min, and analysed EEG responses to auditory stimulation with CNNs. These networks have the strong advantage that they can detect discriminative patterns even in heterogeneous data sets, with minimal a priori assumptions and preprocessing.19,20,22–24,26 Importantly, all steps of the presented method are automatic, apart from visual inspection of the data and manual rejection of artefacts, which were done to ensure high-quality data. Future studies can investigate whether this step can also be automated and replaced with existing algorithms for rejection of EEG artefacts.40
Neural networks assisting prognostication of coma outcome
Neural networks have been used in several fields showing an astonishing potential to automate and improve prognostication of various neurological disorders,19,20 but their use in neuro-critical care and coma outcome prognosis remains limited. The few existing studies using neural networks to predict coma outcome are based on EEG recordings in the absence of external stimulation. These have shown a remarkable performance (AUC = 0.8924; AUC at 24 h = 0.8823; best AUC at 24 h = 0.8925), in predicting outcome. In our study, we obtained a mean AUC score of 0.70 ± 0.04 and 0.83 for the best fold (Table 1), and a mean PPV of 0.83 ± 0.03 and 0.92 for the best fold (Table 1). The AUC values we obtained are slightly lower than previous studies. However, it is worth noting that our study relies on a complementary piece of information to what is currently used in the clinical routine, that is, standardized auditory stimulation. Our approach is predictive of awakening and not of overall outcome, and therefore the most informative measure of performance is the PPV and not the AUC.
As such, we could show that neural networks have the potential to provide concrete diagnostic information for patients in a clinical ‘grey zone’, for whom currently available clinical tests are inconclusive. The PPV for these patients was at similar levels as for the full cohort. Moreover, control analyses confirmed that the network’s prediction generalizes to ‘grey zone’ patients, even when it has been trained with few or no ‘grey zone’ patients (Supplementary material). Future studies with larger patient cohorts can evaluate the clinical applicability of neural networks and confirm these results.
One main limitation of our approach, and also of most previous studies (with few exceptions, such as Juan et al.41), is that we only predict a binary outcome of survival versus non-survival. Survivors are defined as patients with a CPC 1–3, which corresponds to varying levels of autonomy and quality of life.32,42 Although a CPC of 1–2 generally represents a satisfactory quality of life, a CPC of 3 can be a heterogeneous class. Because we trained the network to specifically discriminate between patients with CPC 1–3 versus patients with a CPC of 5 (death), we could not find a more fine-grained link between the network’s output and the state of survival at 3 months. Future studies, expanding on larger patient cohorts, could use the CPC score already during network training to evaluate whether auditory responses in the acute coma phase can be informative not only of survival, but also of its quality.
Electrophysiological features contributing to the network’s confidence in outcome prediction
One major concern for the use of neural networks in a clinical environment is that of interpretability, i.e. tracing features in the data that were important for decisions made by a network. Here, we addressed this concern by showing that the output of the neural network was strongly correlated to features of EEG activity like the phase locking, previously shown to reflect coma severity,18,43,44 and also to clinical evaluations.27,45 The phase locking of EEG responses to sounds, recently shown to be predictive of patients’ chances of awakening from coma,18 was strongly correlated to the networks’ confidence in predicting survival, such that the higher the phase locking, the stronger the network’s confidence. Crucially, this link was observed not only across survivors and non-survivors, which might be considered trivial as both measures predict outcome, but also within the groups of survivors and non-survivors separately. This suggests that the decisions made by the neural network for assessing the confidence of survival are strongly linked to the strength of neural synchrony across EEG electrodes. Importantly, not only did we observe a strong correlation between PLV and network’s output, but we also replicated previous findings about the predictive value of PLV18 in a new patient cohort, treated with targeted temperature management at 36°C.
The advantage of using CNNs over hand-crafted and preselected features, such as the PLV, is that the network automatically extracts multivariate features of the ERP response, which are most discriminant between patient outcomes. This approach is fully data-driven, and the input signal is minimally preprocessed, as opposed to computing the PLV, where one needs to have strong a priori assumptions about the electrode pairs, frequency bands and specific measure to be used (see the supplementary material in Alnes et al.18).
More surprisingly, we also observed a strong negative correlation between the network’s confidence of predicting survival and the complexity of EEG responses to sounds. Neural complexity per se, at the single-patient level, was not predictive of patients’ outcome. This finding has been previously reported for patients treated with targeted temperature management at 33°C,18 and here we replicated it for patients treated at 36°C. Neural complexity can be considered a proxy to neural 'noise', of how structured EEG responses are across time.46 Interestingly, here we found that the higher the network’s confidence for a patient to survive the coma, the lower the complexity of the patient's EEG responses to auditory stimulation. This effect was particularly strong for non-survivors. This finding suggests that although complexity per se is not informative of coma outcome, the network is extracting some features in EEG responses that relate to the levels of neural 'noise' or structure in the EEG signal, which are in turn informative of coma outcome.
Finally, we also found significantly higher confidence in the network’s predictions of survival assigned to patients that have intact brainstem reflexes, motor responses and reactive EEG, compared to those who do not (Fig. 5A–C). All these features are considered to be markers of preserved neural functioning and to indicate good outcome.1 Interestingly, when focusing on reactive EEG, which has a prognostic value for good outcome,27 we found that it provided similar levels of predicting awakening as the neural network (PPV = 0.88). Notably, unlike the EEG reactivity, the neural network was not used to inform clinical decisions about these patients. By contrast, the network’s confidence of survival was significantly lower for patients with discontinuous EEG and irritative EEG than patients without (Fig. 5D and andE),E), which are considered signs of poor outcome .27
In summary, the strong links between the network’s output and clinical or electrophysiological features across patients strengthen the view that the neural network’s decisions (i) are based on clinically relevant features; and (ii) can provide similar levels of performance as currently used techniques,27 but with higher level of automation and objectivity47 and minimal preprocessing in the EEG data.
Conclusions
In summary, we show, for the first time, the strong potential of standardized auditory stimulation in combination with deep learning to predict awakening from coma. Our approach provides an objective and semi-automatic way to quantify a patient’s chances of awakening and surviving at 3 months, already from the first few hours after coma onset. For two different patient cohorts, treated with controlled temperature management at 33°C and 36°C and recorded across four different hospitals, the neural network provides a high PPV of awakening, of 0.83 ± 0.04 and 0.81 ± 0.06, respectively. This finding was also confirmed for a subgroup of patients whose outcome prognosis was indeterminate with currently used diagnostic criteria. Importantly, we could show strong links between the output of the neural network and electrophysiological characteristics of the EEG responses to sounds reflecting neural synchrony and complexity, which have been previously associated with the presence of consciousness from a theoretical point of view. Our work calls for a more systematic use of standardized auditory stimulation in the clinical routine, in combination with state-of-the-art deep learning algorithms, to assist and improve early prognostication of coma outcome.
Contributor Information
Florence M Aellen, Institute of Computer Science, University of Bern, Bern, Switzerland. Zentrum für Experimentelle Neurologie, Department of Neurology, Inselspital, Bern University Hospital, University of Bern, Bern, Switzerland.
Sigurd L Alnes, Institute of Computer Science, University of Bern, Bern, Switzerland. Zentrum für Experimentelle Neurologie, Department of Neurology, Inselspital, Bern University Hospital, University of Bern, Bern, Switzerland.
Fabian Loosli, Institute of Computer Science, University of Bern, Bern, Switzerland.
Andrea O Rossetti, Neurology Service, Department of Clinical Neurosciences, Lausanne University Hospital and University of Lausanne, Lausanne, Switzerland.
Frédéric Zubler, Sleep-Wake-Epilepsy-Center, Department of Neurology, Inselspital, Bern University Hospital, University of Bern, Bern, Switzerland.
Marzia De Lucia, Laboratory for Research in Neuroimaging (LREN), Department of Clinical Neurosciences, Centre Hospitalier Universitaire Vaudois (CHUV), Lausanne, Switzerland.
Athina Tzovara, Institute of Computer Science, University of Bern, Bern, Switzerland. Zentrum für Experimentelle Neurologie, Department of Neurology, Inselspital, Bern University Hospital, University of Bern, Bern, Switzerland. Sleep Wake Epilepsy Center—NeuroTec, Department of Neurology, Inselspital, Bern University Hospital, University of Bern, Bern, Switzerland. Helen Wills Neuroscience Institute, University of California Berkeley, Berkeley, CA, USA.
Funding
This work is supported by the Interfaculty Research Cooperation ‘Decoding Sleep: From Neurons to Health & Mind’ of the University of Bern (F.M.A, S.L.A. and A.T.), the Swiss National Science Foundation (#320030_188737 to A.T.) and the Fondation Pierre Mercier pour la science (A.T.). M.D.L. was funded by the University of Lausanne grant ‘Pro-Femmes’.
References
Articles from Brain are provided here courtesy of Oxford University Press
Full text links
Read article at publisher's site: https://doi.org/10.1093/brain/awac340
Read article for free, from open access legal sources, via Unpaywall:
https://academic.oup.com/brain/article-pdf/146/2/778/49171789/awac340.pdf
Citations & impact
Impact metrics
Citations of article over time
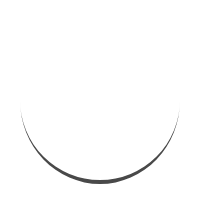
Smart citations by scite.ai
Explore citation contexts and check if this article has been
supported or disputed.
https://scite.ai/reports/10.1093/brain/awac340
Article citations
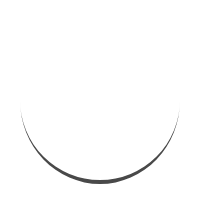
Data
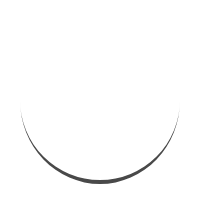
Similar Articles
To arrive at the top five similar articles we use a word-weighted algorithm to compare words from the Title and Abstract of each citation.
Auditory discrimination improvement predicts awakening of postanoxic comatose patients treated with targeted temperature management at 36°C.
Resuscitation, 118:89-95, 14 Jul 2017
Cited by: 7 articles | PMID: 28713043
Automated auditory mismatch negativity paradigm improves coma prognostic accuracy after cardiac arrest and therapeutic hypothermia.
J Clin Neurophysiol, 31(4):356-361, 01 Aug 2014
Cited by: 11 articles | PMID: 25083848
Complementary roles of neural synchrony and complexity for indexing consciousness and chances of surviving in acute coma.
Neuroimage, 245:118638, 06 Oct 2021
Cited by: 13 articles | PMID: 34624502
Deep learning for EEG-based prognostication after cardiac arrest: from current research to future clinical applications.
Front Neurol, 14:1183810, 24 Jul 2023
Cited by: 0 articles | PMID: 37560450 | PMCID: PMC10408678
Review Free full text in Europe PMC
Funding
Funders who supported this work.
Fondation Pierre Mercier pour la science
Interfaculty Research Cooperation
Swiss National Science Foundation (2)
Grant ID: 188737
Grant ID: 320030