Abstract
Background
Few studies have investigated the collaborative potential between artificial intelligence (AI) and pulmonologists for diagnosing pulmonary disease. We hypothesised that the collaboration between a pulmonologist and AI with explanations (explainable AI (XAI)) is superior in diagnostic interpretation of pulmonary function tests (PFTs) than the pulmonologist without support.Methods
The study was conducted in two phases, a monocentre study (phase 1) and a multicentre intervention study (phase 2). Each phase utilised two different sets of 24 PFT reports of patients with a clinically validated gold standard diagnosis. Each PFT was interpreted without (control) and with XAI's suggestions (intervention). Pulmonologists provided a differential diagnosis consisting of a preferential diagnosis and optionally up to three additional diagnoses. The primary end-point compared accuracy of preferential and additional diagnoses between control and intervention. Secondary end-points were the number of diagnoses in differential diagnosis, diagnostic confidence and inter-rater agreement. We also analysed how XAI influenced pulmonologists' decisions.Results
In phase 1 (n=16 pulmonologists), mean preferential and differential diagnostic accuracy significantly increased by 10.4% and 9.4%, respectively, between control and intervention (p<0.001). Improvements were somewhat lower but highly significant (p<0.0001) in phase 2 (5.4% and 8.7%, respectively; n=62 pulmonologists). In both phases, the number of diagnoses in the differential diagnosis did not reduce, but diagnostic confidence and inter-rater agreement significantly increased during intervention. Pulmonologists updated their decisions with XAI's feedback and consistently improved their baseline performance if AI provided correct predictions.Conclusion
A collaboration between a pulmonologist and XAI is better at interpreting PFTs than individual pulmonologists reading without XAI support or XAI alone.Free full text

Collaboration between explainable artificial intelligence and pulmonologists improves the accuracy of pulmonary function test interpretation
Abstract
Background
Few studies have investigated the collaborative potential between artificial intelligence (AI) and pulmonologists for diagnosing pulmonary disease. We hypothesised that the collaboration between a pulmonologist and AI with explanations (explainable AI (XAI)) is superior in diagnostic interpretation of pulmonary function tests (PFTs) than the pulmonologist without support.
Methods
The study was conducted in two phases, a monocentre study (phase 1) and a multicentre intervention study (phase 2). Each phase utilised two different sets of 24 PFT reports of patients with a clinically validated gold standard diagnosis. Each PFT was interpreted without (control) and with XAI's suggestions (intervention). Pulmonologists provided a differential diagnosis consisting of a preferential diagnosis and optionally up to three additional diagnoses. The primary end-point compared accuracy of preferential and additional diagnoses between control and intervention. Secondary end-points were the number of diagnoses in differential diagnosis, diagnostic confidence and inter-rater agreement. We also analysed how XAI influenced pulmonologists’ decisions.
Results
In phase 1 (n=16 pulmonologists), mean preferential and differential diagnostic accuracy significantly increased by 10.4% and 9.4%, respectively, between control and intervention (p<0.001). Improvements were somewhat lower but highly significant (p<0.0001) in phase 2 (5.4% and 8.7%, respectively; n=62 pulmonologists). In both phases, the number of diagnoses in the differential diagnosis did not reduce, but diagnostic confidence and inter-rater agreement significantly increased during intervention. Pulmonologists updated their decisions with XAI's feedback and consistently improved their baseline performance if AI provided correct predictions.
Conclusion
A collaboration between a pulmonologist and XAI is better at interpreting PFTs than individual pulmonologists reading without XAI support or XAI alone.
Short abstract
This study demonstrates that pulmonologists improve their individual diagnostic interpretation of pulmonary function tests when supported by AI-based computer protocols with automated explanations. Such teamwork may become commonplace in the future. https://bit.ly/3ZKK4Eu
Introduction
When correctly interpreted, pulmonary function tests (PFTs) are a useful tool to address the differential diagnosis of respiratory diseases [1]. However, interpretation of PFTs requires expertise in combining the understanding of normal values, lung function patterns (obstructive, restrictive, mixed and normal) and appearance of flow–volume curves within the patient's medical history, clinical presentation and results of other diagnostic assessments [2, 3]. Although various algorithms exist to aid the interpretation of PFTs [4, 5], it has been shown that neither pulmonologists nor the American Thoracic Society (ATS)/European Respiratory Society (ERS)’s guideline-derived algorithms are sufficiently accurate for a correct reading [6, 7].
It could be argued that artificial intelligence (AI) may help in automating the complex reasoning that drives the process of interpreting PFTs. Indeed, when all the PFT indices are taken together, the data-based AI approach captures subtle characteristics of respiratory disorders that are not always identified by the clinician, resulting in a powerful algorithm for differential diagnosis [8]. In the past, such AI-driven algorithms have been shown to perform as well if not better than pulmonologists alone and might help support pulmonologists to interpret lung function [6]. However, most clinical studies often report AI outperforming clinicians’ diagnostic performance in head-to-head comparisons [9, 10], giving way to an irrational claim that clinicians will soon be replaced by AI-equipped devices. Unlike the narrow task-based scope of AI, clinicians carry out a multitude of duties involving diagnostics, treatment and management of patients while also bringing a vital element of empathy to healthcare [11]. While clinicians are irreplaceable, there remains a vast potential for AI and clinicians to work together in improving routine clinical outcomes [11]. Presently, no data exist on the benefits of a collaboration between AI and a pulmonologist at interpreting PFTs. Furthermore, AI algorithms are often regarded as black boxes, i.e. they cannot provide explanations on their output [12]. Understanding the rationale behind a prediction is critical to gaining trust, especially if a clinician plans an action based on the algorithm's output. On the other hand, it has also been suggested that explanations may help in mitigating automation bias and other errors that arise from over-reliance on AI systems [13]. Today, several methods exist that allow us to produce explanations, rendering AI more transparent and hence easier to decipher. This new paradigm of AI is called explainable AI (XAI) [14].
In this study, we hypothesised that a pulmonologist with the help of XAI's suggestions would be superior at interpreting PFTs to the pulmonologist working alone. Our primary goal was to compare the preferential and differential diagnostic accuracy between the pulmonologist's view (control) and the pulmonologist's view assisted with suggestions provided by a machine-learning model (intervention) [6]. We also compared whether the intervention was better than the AI's standalone diagnostic performance. Additionally, we investigated how pulmonologists updated their diagnostic choices following the assistance of XAI.
Methods
Study design
In this study with a repeated measures design, pulmonologists were requested to interpret 24 anonymised PFT reports including pre- and/or post-bronchodilator spirometry, lung volumes, airway resistance and diffusing capacity (with access to z-scores and data colour coding indicating deviation from normal). Limited clinical information (smoking history and symptom presentation) was also provided. Each PFT report was interpreted in two steps: 1) a control step in which pulmonologists provided their responses after reading the PFT report only, then 2) an intervention step in which pulmonologists provided their responses for the same report with suggestions of XAI available to them. Thus, each pulmonologist performed 48 interpretations in one exercise.
We carried out the study in two phases. Phase 1 (P1) was a monocentric study in which 16 out of 25 invited pulmonologists from University Hospitals Leuven (Leuven, Belgium) completed the study. In phase 2 (P2), 62 out of 88 invited pulmonologists from across European institutions completed the study (supplementary table S1). P2 was initiated only after we observed that primary end-points in P1 were met. The set of 24 PFT reports differed completely between the two phases.
We used the Gorilla Experiment Builder online platform to carry out the study [15]. Participants could complete the study at their own pace with no time limits. They began by indicating their informed consent, years of clinical experience (<5 or ≥5 years), any prior experience with AI-based clinical decision support system (Yes/No) and their enthusiasm on AI applications in general on a 5-point Likert scale (supplementary material S2).
Afterwards, participants were guided to complete a tutorial to familiarise themselves with the online platform and XAI's suggestions (supplementary material S3). During the main tasks, pulmonologists provided a differential diagnosis including a mandatory preferential diagnosis and up to three additional diagnoses ranked in the order of preference. The diagnostic choices were: 1) healthy or normal, 2) asthma (including obstructive or non-obstructive), 3) COPD (including emphysema or chronic bronchitis), 4) interstitial lung disease (ILD) (including idiopathic pulmonary fibrosis and non-idiopathic pulmonary fibrosis), 5) neuromuscular disease (NMD) (including diaphragm paralysis), 6) other obstructive disease (OBD) (including cystic fibrosis, bronchiectasis and bronchiolitis), 7) thoracic deformity (TD) (including pleural disease and pneumonectomy) and 8) pulmonary vascular disease (PVD) (including pulmonary hypertension, vasculitis and chronic thromboembolic pulmonary hypertension).
The pulmonologists also provided an overall confidence of their diagnosis on a 5-point Likert scale (1=least confidence, 5=highest confidence). In addition, they indicated their level of agreement with XAI's suggestion on a 5-point Likert scale (1=strongly disagree, 2=disagree, 3=neutral, 4=agree, 5=strongly agree) in the intervention phases. Supplementary material S3 shows an example of a control and intervention phase for one particular PFT report.
An ethics committee approval was obtained for P1 (S60243), while a separate ethics committee approval was obtained for the international multicentre P2 phase (S65162).
PFT cases
Between November 2017 and August 2018 at University Hospitals Leuven, 1003 subjects performed complete lung function testing. All PFTs were performed with standardised equipment by respiratory operators (Masterlab; Jaeger, Würzburg, Germany), according to ATS/ERS criteria [16]. Global Lung Function Initiative equations were used to calculated reference values for spirometric forced expiratory volume in 1 s (FEV1), forced vital capacity (FVC) and FEV1/FVC [17], while the 1993 European Community for Steel and Coal standards were used for diffusion capacity, lung volumes and airway resistance measurements [18]. A single clinician assigned a preliminary diagnosis across each of the eight disease categories in 794 subjects by referring to electronic health records of clinical history, symptoms, PFT reports and additional tests. A high prevalence of COPD (23%), ILD (25%), asthma (9%) and normal (30%) subjects characterised the sample. All subjects were Caucasians older than 18 years. From this group, we shortlisted 92 subjects, by randomly selecting 15 subjects from each of the most prevalent groups (COPD, asthma, ILD and normal lung function) and eight subjects from each of the least prevalent diseases (NMD, TD, PVD and OBD). Two pulmonologists jointly adjudicated the gold standard diagnosis in each of these cases using all available clinical data including PFTs. If there was disagreement or doubt about the diagnosis another case was selected to end up with a set of 24 PFT cases with a gold standard diagnosis, for P1 and P2 separately. In each set, we randomly included four subjects from the most prevalent diseases and two subjects from the least prevalent diseases. We then slightly inflated the sample of incorrectly predicted cases by the AI to study how clinicians would respond to incorrect AI suggestions. Following an additional review by the pulmonologists, three cases in each set that were correctly predicted by the AI were deliberately replaced by cases in which the AI did not correctly predict the adjudicated gold standard diagnosis. Thus in both sets, the preferential diagnostic accuracy of the AI was set at 62.5% (15 out of 24 cases), which was lower than its reported validation accuracy of 74% [6].
Explainable artificial intelligence
We used our previously reported machine-learning model that predicts eight respiratory disorders (COPD, asthma, ILD, healthy, NMD, TD, PVD and OBD) [6]. Its preferential diagnostic accuracy (disease with the highest calculated probability) was reported at 74% during inter-validation, while similar accuracies (76–82%) were also observed during testing on external cohorts [6]. In this study we also reported explanations on AI's second diagnostic suggestion when its probability was >15%, in addition to explanations for AI's preferential diagnosis. To render the AI model explainable, we used a game-theoretic concept called Shapley values (SVs) to estimate the evidence of different PFT indices towards AI's diagnostic suggestions [19]. A positive SV is interpreted as evidence supporting the model's prediction, while a negative SV is counter-evidence. The magnitude of the SV denotes the strength of the contribution. For each diagnostic suggestion, we included a SV plot of the top five PFT indices in descending order of magnitude of evidence. We also normalised the SVs by dividing them by the highest magnitude. We show an example of a PFT case with XAI's suggestions in figure 1.
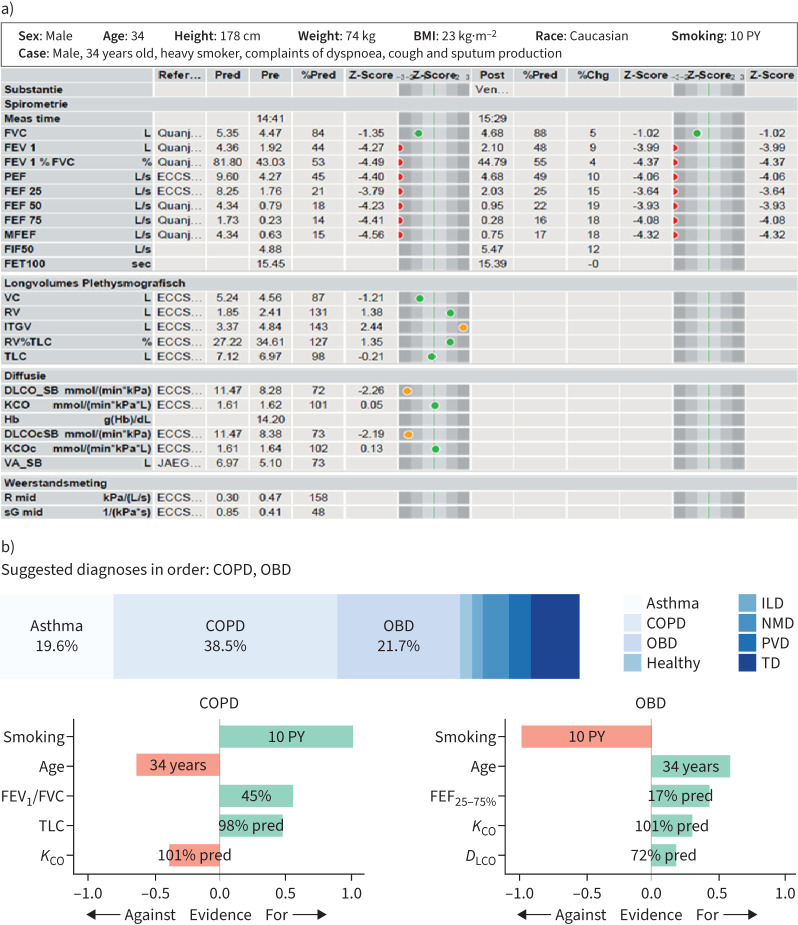
a) A sample pulmonary function test (PFT) report (see [16–18] for details) and b) explainable artificial intelligence (XAI)'s diagnostic suggestions with Shapley value (SV) evidence. The gold standard diagnosis was COPD based on emphysema on computed tomography scan and passive smoke exposure during childhood (normal α1-antitrypsin levels). In this case, XAI makes two diagnostic suggestions (COPD and other obstructive disease (OBD)) since the probability of the second disease (OBD) is >15%. Additionally, we show a normalised SV plot of the top five PFT indices that contributed towards the prediction of COPD and OBD, respectively. A positive SV (in green) is supporting evidence, while a negative SV (in red) is counter-evidence. BMI: body mass index; PY: pack-years; ILD: interstitial lung disease; NMD: neuromuscular disease; PVD: pulmonary vascular disease; TD: thoracic deformity; FEV1: forced expiratory volume in 1 s; FVC: forced vital capacity; KCO: transfer coefficient of the lung for carbon monoxide; FEF25–75%: forced expiratory flow at 25–75% of FVC; DLCO: diffusing capacity of the lung for carbon monoxide.
Study end-points
Our primary end-point was to compare pulmonologists’ mean preferential and differential diagnostic accuracy between the control and the intervention setting. The mean preferential accuracy is calculated as the number of cases in which a pulmonologists’ preferential diagnosis matched the gold standard, averaged over the entire cohort. Mean differential accuracy is calculated as the number of times in which a pulmonologists’ differential diagnosis (preferential diagnosis+additional diagnoses) included the gold standard, averaged over the entire cohort. As secondary end-points, we explored the number of additional diagnoses, clinicians’ diagnostic confidence on the overall diagnostic performance as well as their inter-rater agreement on the preferential diagnosis. We also analysed how pulmonologists updated their diagnostic decisions between control and intervention, and further studied if pulmonologists followed XAI's incorrect suggestions, indicating automation bias.
Sample size calculation
The minimum sample size for pulmonologists was calculated at 11, using the two-sided paired t-test with the assumption that the accuracy of both preferential and differential diagnosis improves between control and intervention with a mean of three cases out of 24 (12.5%), a standard deviation of three cases, a significance of 0.05 and power of 0.8. The premise of our assumption is that the intervention setting will show a mean improvement in preferential and differential diagnostic accuracy of at least 10% [6].
Statistical analysis
We evaluated our quantitative end-points using the paired t-test. Inter-observer agreement in preferential diagnostic choice was assessed using Fleiss’ κ. Continuous variables were assumed to be normally distributed with homogenous variance and the Shapiro–Wilk test was used to test assumptions of normality. We performed all our analysis with R statistical software (www.r-project.org) using a significance level of 0.05.
Results
Participant demographics
P1 and P2 saw the participation of 16 and 62 pulmonologists, respectively (supplementary table S4). More than three-quarters of the participants in both phases had at least 5 years of clinical experience. Over half of P1 participants had prior experience with AI-based decision support systems, but that percentage was much lower in P2 (11%). Mean baseline enthusiasm about AI on a 5-point Likert scale was high in both groups (3.56 and 3.92, respectively), suggesting an overall bias towards accepting AI's decisions.
PFT sample characteristics and baseline XAI's performance
PFT sample characteristics were similar for P1 and P2 (n=24 each) (table 1). Both samples included four groups each of high prevalence (COPD, asthma, ILD and normal lung function) and two diseases each of low prevalence (NMD, TD, PVD and OBD).
TABLE 1
Overview of pulmonary function test (PFT) characteristics in the monocentric phase 1 (P1) and multicentric phase 2 (P2) studies, with 24 PFT reports each
Healthy | COPD | Asthma | ILD | NMD | OBD | TD | PVD | |
P1 study | ||||||||
![]() | 4 | 4 | 4 | 4 | 2 | 2 | 2 | 2 |
![]() | 3/1 | 3/1 | 2/2 | 3/1 | 0/2 | 2/0 | 0/2 | 1/1 |
![]() | 36–62 | 58–72 | 26–48 | 51–84 | 59–59 | 20–49 | 65–67 | 70–82 |
![]() | 0–0 | 30–56 | 0–5 | 0–12 | 10–35 | 0–0 | 0–25 | 0–30 |
![]() | −0.78–1.05 | −3.08–![]() | −4.13–![]() | −3.84–0.64 | −4.41–![]() | −4.87–![]() | −4.09–![]() | −0.33–1.19 |
![]() | −0.93–0.93 | −1.16–0.22 | −1.61–![]() | −4.31–![]() | −5.02–![]() | −3.59–![]() | −4.8–![]() | −1.01–1.44 |
![]() | 77–86 | 54–64 | 49–82 | 83–90 | 77–81 | 43–60 | 79–80 | 72–89 |
![]() | −1.44–0.19 | 1.21–2.28 | −0.63–2.89 | −3.43–![]() | −1.64–![]() | 3.68–3.73 | −3.39–![]() | 0.44–0.88 |
![]() | −1.49–1.3 | −0.34–1.13 | −0.37–1.06 | −4.12–![]() | −3.37–![]() | −0.07–1.84 | −4.78–![]() | −0.09–0.54 |
![]() | −0.81–0.45 | −2.66–0.4 | −1.12–![]() | −3.86–![]() | −1.96–![]() | −2.45–![]() | −3.24–![]() | −3.29–![]() |
![]() | −0.97–2.24 | −1.88–0.39 | −0.26–0.71 | −2.24–0.95 | 1.52–4.72 | −0.22–1.31 | 0.02–3.36 | −2.3–![]() |
P2 study | ||||||||
![]() | 4 | 4 | 4 | 4 | 2 | 2 | 2 | 2 |
![]() | 3/1 | 1/3 | 0/4 | 2/2 | 2/0 | 0/2 | 2/0 | 1/1 |
![]() | 27–67 | 48–84 | 21–59 | 35–85 | 31–56 | 30–68 | 54–90 | 50–64 |
![]() | 0–25 | 18–50 | 0–20 | 0–25 | 0–3 | 0–0 | 0–0 | 10–20 |
![]() | −0.69–0.79 | −4.96–![]() | −1.02–1.38 | −4.35–![]() | −4.6–![]() | −5.35–![]() | −3.05–![]() | −1.16–![]() |
![]() | −1.21–0.7 | −4.1–0.19 | −0.16–1.85 | −4.39–![]() | −4.83–![]() | −3.56–![]() | −3.03–![]() | −1.35–![]() |
![]() | 77–92 | 49–60 | 69–73 | 77–87 | 81–81 | 42–61 | 74–80 | 67–82 |
![]() | −1.08–0.72 | −1.14–4.56 | −0.53–3.43 | −1.84–2.53 | −1.11–![]() | 1.77–4.64 | −2.07–![]() | −0.55–0.48 |
![]() | −0.06–0.01 | −2.7–2.05 | −0.32–2.81 | −3.63–![]() | −2.74–![]() | −0.77–![]() | −3.42–![]() | −1.04–0.16 |
![]() | −1.28–![]() | −3.73–![]() | −0.62–0.76 | −5.2–![]() | −4.95–![]() | −1.69–1.15 | −2.28–![]() | −2.19–![]() |
![]() | −0.78–0.23 | −0.44–0.39 | −0.44–0.58 | −1.79–![]() | −1.17–2.27 | 0.41–1.4 | 0.89–1.15 | −1.47–![]() |
Data are presented as n or minimum–maximum. ILD: interstitial lung disease; NMD: neuromuscular disease; OBD: other obstructive disease; TD: thoracic deformity; PVD: pulmonary vascular disease; FEV1: forced expiratory volume in 1 s; FVC: forced vital capacity; RV: residual volume; TLC: total lung capacity; DLCO: diffusing capacity of the lung for carbon monoxide; KCO: transfer coefficient of the lung for carbon monoxide.
AI's preferential diagnosis was set to match the gold standard in 15 out of 24 cases (62.5%) in both P1 and P2 samples, while its differential diagnosis (preferential diagnosis+second diagnostic suggestion) included the gold standard in 22 (91.7%) of the P1 cases and 21 (87.5%) of the P2 cases. A breakdown of AI's diagnostic performance across different disease groups is given in supplementary table S5.
Primary end-points
In P1, the use of XAI improved the mean preferential and differential diagnostic accuracy by 10.4% and 9.4%, respectively (p<0.001), which was somewhat higher than in P2 (5.4% and 8.7%, respectively; p<0.0001). Thus, primary end-points were met as mean diagnostic accuracies significantly increased between control (pulmonologist) and intervention (pulmonologist+XAI) (table 2 and figure 2). However, the improvements were smaller than anticipated (12.5%) from our sample size estimation.
TABLE 2
Primary end-points in the monocentric phase 1 (P1) and multicentric phase 2 (P2) studies
XAI alone,
% |
Control (pulmonologist),
% |
Intervention (pulmonologist+XAI),
% | Mean improvement, % | ||
Intervention
on control |
Intervention
on XAI alone | ||||
P1 study (16 pulmonologists) | |||||
![]() | 62.5 | 65.1±8.2 | 75.5±9.3 | 10.4*** | 13**** |
![]() | 91.7 | 85.4±10.5 | 94.8±5.8 | 9.4**** | 3.1* |
P2 study (62 pulmonologists) | |||||
![]() | 62.5 | 69.3±9.1 | 74.6±7.6 | 5.4**** | 12.1**** |
![]() | 87.6 | 81.7±11.2 | 90.4±8.8 | 8.7**** | 2.9* |
Data are presented as mean±sd, unless otherwise stated. XAI: explainable artificial intelligence. #: differential diagnosis includes preferential diagnosis and up to three additional diagnoses. *: p<0.05; ***: p<0.001; *****: p<0.0001.
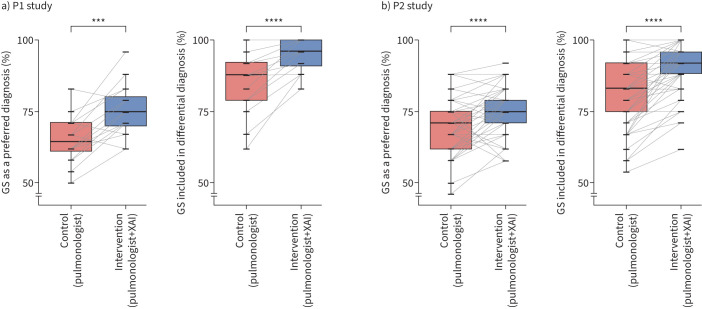
Percentage change of preferential and differential diagnostic performance between control (individual pulmonologists) and intervention (pulmonologists and explainable artificial intelligence (XAI)) in a) the phase 1 (P1) study with 16 pulmonologists and b) the phase 2 (P2) study with 62 pulmonologists. Boxes indicate median and interquartile range. ***: p<0.001; ****: p<0.0001. GS: gold standard.
When we compared the diagnostic performance between XAI and the intervention setting (pulmonologist+XAI) as an exploratory analysis, we also observed a mean improvement of 13% (p<0.0001) and 3.1% (p=0.01) for preferential and differential diagnostic accuracy in P1 (n=16), which was similar to P2 (n=62) with a mean improvement of 12.25% and 2.9%, respectively. Thus, we noted that pulmonologists with the help of XAI's suggestion not only improved their individual performance, but they also significantly outperformed AI's predictive performance in both P1 and P2 (supplementary figure S6).
Secondary end-points
We included a number of secondary end-points in our study (table 3). In both studies, mean Likert scale confidence in diagnosis significantly increased (p<0.01), while the number of differential diagnostic choices remained unchanged between control and intervention. Fleiss' κ quantifying inter-clinician agreement in preferential diagnosis also increased. Pulmonologists indicated a moderately high level of agreement with the suggestions of XAI.
TABLE 3
Secondary end-points in the monocentric phase 1 (P1) and multicentric phase 2 (P2) studies
Control
(pulmonologist) |
Intervention
(pulmonologist+XAI) | p-value | |
P1 study (16 pulmonologists) | |||
![]() | 1.86±0.32 | 1.8±0.33 | 0.197 |
![]() ![]() | 3.71±0.5 | 3.98±0.42 | <0.01 |
![]() ![]() | 3.76±0.3 | ||
![]() | 0.52 | 0.64 | |
P2 study (62 pulmonologists) | |||
![]() | 1.67±0.35 | 1.64±0.32 | 0.22 |
![]() ![]() | 3.93±0.34 | 4.03±0.34 | <0.0001 |
![]() ![]() | 3.49±0.36 | ||
![]() | 0.53 | 0.63 |
Data are presented as mean±sd, unless otherwise stated. XAI: explainable artificial intelligence.
Demographics-based performance
In P2 (n=62), we further analysed the diagnostic performance of the enhanced setting (pulmonologist+XAI) by stratifying on experience. We observed no significant differences in interventional diagnostic accuracies between participants with <5 years (n=12) and ≥5 years (n=50) of experience. Similarly, no significant differences were observed when the subjects were stratified on their baseline enthusiasm about AI applications (supplementary table S7).
Change in responses
In both phases, pulmonologists’ diagnostic responses changed between control and intervention in almost half of the 24 cases (table 4). Diagnostic confidence at baseline was significantly lower in cases where responses changed compared with cases in which responses remained unchanged. Whenever responses changed, we observed a significant improvement (p<0.001) in differential diagnostic accuracy: in the 55% changed cases of P1, the differential diagnosis contained the gold standard in 78% within the control arm versus 95% after the intervention; in the 48% changed cases in P2, the differential diagnosis included the gold standard in 73% of the control arm versus 91% after the intervention. The changed responses always contained at least one diagnostic suggestion of XAI.
TABLE 4
Change (percentage of cases) in diagnostic responses between control and intervention in the phase 1 (P1) study with 16 pulmonologists and the phase 2 (P2) study with 62 pulmonologists
Change (cases, %) | Baseline confidence# | |
P1 study (16 pulmonologists) | ||
![]() | 45±16.3 | 3.87±0.54 |
![]() | 55±16.3 | 3.56±0.48 |
![]() ![]() | 27.1±10 | p<0.01 |
![]() ![]() | 27.9±11.5 | |
P2 study (62 pulmonologists) | ||
![]() | 51.7±15.8 | 4.09±0.36 |
![]() | 48.5±15.8 | 3.76±0.39 |
![]() ![]() | 18±14.2 | p<0.01 |
![]() ![]() | 30.4±13.9 |
Data are presented as mean±sd, unless otherwise stated. #: baseline confidence is the overall diagnostic confidence on a 5-point Likert scale indicated by pulmonologists during control. t-test comparison between baseline Likert scales is given with p-value.
Automation bias
We studied if pulmonologists’ performance reduced between control and intervention whenever AI suggested a correct or incorrect preferential diagnosis (nine cases in P1 and P2, respectively) (supplementary table S8). While it was found that preferential diagnostic accuracy reduced slightly but significantly in cases where an incorrect XAI diagnosis was given, we observed much larger increases in accuracy when the XAI diagnosis was correct. We also observed that pulmonologists placed a significantly higher (p<0.001) level of agreement with XAI's suggestions in cases with correct preferential predictions as opposed to with incorrect preferential predictions, indicating little risk for automation bias.
Discussion
In this study conducted in two separate phases, we observed that pulmonologists when aided by XAI significantly improved on their individual preferential and differential diagnostic accuracy in interpreting PFTs. Among secondary end-points, we noted a significant increase in diagnostic confidence but no reduction in the number of differential diagnostic choices. Our results support the hypothesis that a pulmonologist aided by XAI improves on the interpretation of PFTs for the differential diagnosis of respiratory diseases when compared with individual pulmonologists with no support. Interestingly, we also observed that pulmonologists when aided by XAI significantly outperformed XAI itself in preferential and differential diagnostic accuracy.
Most clinical studies involving AI have emphasised the diagnostic superiority of AI using head-to-head comparisons [10], while few have studied the benefits of a collaborative approach. In fact, our post-hoc head-to-head comparison revealed no clear differences in diagnostic accuracy between AI and individual pulmonologists in both P1 and P2. This was expected because unlike most studies that typically compare AI with non-experts diluting average human performance, our participants were respiratory medicine specialists. It is likely that the use of XAI will be even more beneficial when used by medical practitioners less experienced in interpreting PFTs. Although this was not the aim of our study, the use of XAI could be expanded to these populations if proven advantageous. Secondly, a lower than expected improvement can also be explained by the fact that we purposefully included PFT cases in which AI made mistakes to study the effect of incorrect predictions on clinicians’ decision making. A random selection of cases based on actual disease prevalence in the real word would have seen a higher AI accuracy and pushed up pulmonologist's performance by a larger margin.
The superiority of the collaborative approach is in line with several clinical decision support systems (CDSSs) that have been reported to improve practitioners’ performance in the past [20]. Our study adopted a repeated measures design instead of a placebo-controlled trial, not only due to the limited availability of participants. We also wanted to recreate a setting in which the pulmonologist arrives at a diagnostic work-up and updates, if needed, based on an automated protocol. Although there might be an element of learning effect present through the repeated measure design, our results showed that XAI's suggestions effected a change in pulmonologists’ responses in almost of half of the cases. Whenever responses changed, pulmonologists were more likely to improve over their baseline performance. An analysis of changed responses revealed that the updated diagnosis always contained at least one diagnostic suggestion of XAI.
Our study also allowed a preliminary investigation into automation bias, a known error that arises due to clinicians over-relying on CDSSs’ output even when it is incorrect [13]. The present results showed that pulmonologists preferential diagnostic performance decreased slightly whenever AI made incorrect predictions, but increased considerably when a correct diagnostic suggestion was made. Moreover, agreement with XAI's suggestions was significantly higher (p<0.0001) with correct suggestions compared with those in which AI made incorrect predictions, indicating only limited risk for automation bias. Researchers have suggested that explanations, as we provided with the SVs, allow the clinician to develop an internal picture on how the system operates. It has the potential to mitigate misplaced trust and over-reliance on CDSSs [13, 21]. Nonetheless, a controlled study with and without explanations must be conducted to conclusively establish the impact of explanations on automation bias.
The current study is in line with the novel ATS/ERS standards for lung function interpretation stating that PFTs are to detect and quantify disturbances of the respiratory system [22]. Based on certain patterns, clinicians will use PFTs in their diagnostic work-up towards a preferential diagnosis and a reduced list of differential diagnoses. As the AI and XAI algorithms provide probability estimates for diagnostic disease clusters but no final disease diagnoses, they completely support this clinical diagnostic process. A major limitation of our study is that our definition of diagnostic superiority as a positive outcome may be construed as narrow in scope. In real life, a diagnostic work-up is achieved through an extensive anamnesis, clinical exam and a multitude of tests such as exhaled nitric oxide fraction and histamine challenge, blood samples, and even computed tomography scan, which were not available to the pulmonologists in the current study. Vice versa, future AI models may also benefit from multimodal layers of information to improve on their granularity and accuracy. Our study could also have benefitted from a larger sample of PFT reports as the current sample over-represents disease groups like NMD, TD, OBD and PVD. It distorts the actual prevalence of diseases that pulmonologists routinely encounter in clinical practice. Due to the limited sample size and good individual baseline performance of clinicians, the improvements in diagnostic accuracy from introducing AI were small and may be clinically not very relevant. The lack of ethnic diversity was also a major limitation that hinders extrapolation of current results to the general population. In the future, prospective studies using randomised clinical trial settings including less experienced practitioners and using PFTs of a more diverse population, with specific end-points such as time to final diagnosis, number of diagnostic or redundant tests, total costs for the healthcare system, etc., are required to establish the real effectiveness of XAI.
To conclude, our study demonstrates that pulmonologists can improve their individual diagnostic interpretation of PFTs with the help of AI. Such teamwork between AI and clinicians may become commonplace in the future, with the potential to drive healthcare improvements particularly in areas where clinical expertise is less available.
Supplementary material
Please note: supplementary material is not edited by the Editorial Office, and is uploaded as it has been supplied by the author.
Supplementary material ERJ-01720-2022.Supplement
Shareable PDF
Footnotes
Conflict of interest: N. Das holds a patent on automated quality control of spirometry. E. Derom reports consultancy fees from Chiesi, GlaxoSmithKline, AstraZeneca and Boehringer Ingelheim. G. Brusselle reports payment or honoraria for lectures from AstraZeneca, Boehringer Ingelheim, Chiesi, GlaxoSmithKline, Novartis and Sanofi. F. Burgos reports consultancy fees from Medical Graphics Corporation Diagnostics. M. Contoli reports grants from the University of Ferrara, Chiesi and GlaxoSmithKline, consultancy fees and honoraria from AstraZeneca, Boehringer Ingelheim, Chiesi, GlaxoSmithKline and Novartis, as well as support for attending meetings from Chiesi, AstraZeneca, GlaxoSmithKline and ALK-Abelló. W.D-C. Man is part funded by a NIHR Artificial Intelligence Award, and reports grants from the NIHR and British Lung Foundation, as well as honoraria from Mundipharma, Novartis, European Conference and Incentive Services DMC; and is the Honorary President of the Association for Respiratory Technology and Physiology (ARTP, UK). J.K. Quint reports grants from the MRC, HDR UK, GlaxoSmithKline, AstraZeneca and Chiesi, and consultancy fees from Insmed and Evidera. E. Vanderhelst reports grants from Chiesi, and consultancy fees and honoraria from Boehringer Ingelheim, Vertex and GlaxoSmithKline. M. Topalovic is part funded by a NIHR Artificial Intelligence Award, and is co-founder and shareholder of ArtiQ. W. Janssens reports grants from Chiesi and AstraZeneca, consultancy and lecture fees from AstraZeneca, Chiesi and GlaxoSmithKline, and he is co-founder and shareholder of ArtiQ. The remaining authors report no potential conflicts of interest.
This article has an editorial commentary: https://doi.org/10.1183/13993003.00625-2023
Support statement: The study was supported by a VLAIO research grant of ArtiQ and KU Leuven (HB.2020.2406). N. Das, I. Gyselinck and W. Janssens are supported by the Flemish Research Foundation (FWO Vlaanderen). Funding information for this article has been deposited with the Crossref Funder Registry.
References
Full text links
Read article at publisher's site: https://doi.org/10.1183/13993003.01720-2022
Read article for free, from open access legal sources, via Unpaywall:
https://erj.ersjournals.com/content/erj/early/2023/03/15/13993003.01720-2022.full.pdf
Citations & impact
Impact metrics
Citations of article over time
Alternative metrics

Discover the attention surrounding your research
https://www.altmetric.com/details/147595878
Article citations
Machine learning for accurate detection of small airway dysfunction-related respiratory changes: an observational study.
Respir Res, 25(1):286, 24 Jul 2024
Cited by: 0 articles | PMID: 39048993 | PMCID: PMC11270925
Integrating Artificial Intelligence in the Diagnosis of COPD Globally: A Way Forward.
Chronic Obstr Pulm Dis, 11(1):114-120, 01 Jan 2024
Cited by: 0 articles | PMID: 37828644 | PMCID: PMC10913925
How to interpret parameters of routine lung function tests in 2023?
Tunis Med, 101(3):323-333, 05 Mar 2023
Cited by: 2 articles | PMID: 38263920
Similar Articles
To arrive at the top five similar articles we use a word-weighted algorithm to compare words from the Title and Abstract of each citation.
Artificial intelligence outperforms pulmonologists in the interpretation of pulmonary function tests.
Eur Respir J, 53(4):1801660, 11 Apr 2019
Cited by: 64 articles | PMID: 30765505
Dermatologist-like explainable AI enhances trust and confidence in diagnosing melanoma.
Nat Commun, 15(1):524, 15 Jan 2024
Cited by: 11 articles | PMID: 38225244 | PMCID: PMC10789736
Effects of explainable artificial intelligence in neurology decision support.
Ann Clin Transl Neurol, 11(5):1224-1235, 05 Apr 2024
Cited by: 1 article | PMID: 38581138 | PMCID: PMC11093252
Applications of Explainable Artificial Intelligence in Diagnosis and Surgery.
Diagnostics (Basel), 12(2):237, 19 Jan 2022
Cited by: 51 articles | PMID: 35204328 | PMCID: PMC8870992
Review Free full text in Europe PMC
Funding
Funders who supported this work.