Abstract
Background
Bacterial colonization (BC) of the lower airways is common in lung transplant recipients (LTRs) and increases the risk of chronic lung allograft dysfunction. Diagnosis often requires bronchoscopy. Exhaled breath analysis using electronic nose (eNose) technology may noninvasively detect BC in LTRs. Therefore, we aimed to assess the diagnostic accuracy of an eNose to detect BC in LTRs.Methods
We performed a cross-sectional analysis within a prospective, single-center cohort study assessing the diagnostic accuracy of detecting BC using eNose technology in LTRs. In the outpatient clinic, consecutive LTR eNose measurements were collected. We assessed and classified the eNose measurements for the presence of BC. Using supervised machine learning, the diagnostic accuracy of eNose for BC was assessed in a random training and validation set. Model performance was evaluated using receiver operating characteristic analysis.Results
In total, 161 LTRs were included with 80 exclusions because of various reasons. Of the remaining 81 patients, 16 (20%) were classified as BC and 65 (80%) as non-BC. eNose-based classification of patients with and without BC provided an area under the curve of 0.82 in the training set and 0.97 in the validation set.Conclusions
Exhaled breath analysis using eNose technology has the potential to noninvasively detect BC.Free full text

Detection of Bacterial Colonization in Lung Transplant Recipients Using an Electronic Nose
Abstract
Background.
Bacterial colonization (BC) of the lower airways is common in lung transplant recipients (LTRs) and increases the risk of chronic lung allograft dysfunction. Diagnosis often requires bronchoscopy. Exhaled breath analysis using electronic nose (eNose) technology may noninvasively detect BC in LTRs. Therefore, we aimed to assess the diagnostic accuracy of an eNose to detect BC in LTRs.
Methods.
We performed a cross-sectional analysis within a prospective, single-center cohort study assessing the diagnostic accuracy of detecting BC using eNose technology in LTRs. In the outpatient clinic, consecutive LTR eNose measurements were collected. We assessed and classified the eNose measurements for the presence of BC. Using supervised machine learning, the diagnostic accuracy of eNose for BC was assessed in a random training and validation set. Model performance was evaluated using receiver operating characteristic analysis.
Results.
In total, 161 LTRs were included with 80 exclusions because of various reasons. Of the remaining 81 patients, 16 (20%) were classified as BC and 65 (80%) as non-BC. eNose-based classification of patients with and without BC provided an area under the curve of 0.82 in the training set and 0.97 in the validation set.
Conclusions.
Exhaled breath analysis using eNose technology has the potential to noninvasively detect BC.
Lung transplantation (LTx) is a life-prolonging treatment for end-stage lung disease with a median survival of 7.8 y.1 Chronic lung allograft dysfunction (CLAD) and infections are among the most frequent complications of LTx and limit the long-term survival of LTx recipients (LTRs).2
Within the multifactorial cause of CLAD, bacterial colonization (BC) of the lower respiratory airways plays an important role. BC is a common problem after LTx, especially in LTRs colonized before transplantation, with up to one-third of LTRs being affected within the first mo after transplantation.3-5 Currently, BC is diagnosed when cultures—for example, obtained by bronchoalveolar lavage (BAL)—demonstrate the same pathogen without signs of acute infection.6 Early diagnosis and eradication attempts are beneficial. Data on outcomes secondary to BC are contradictory, and trials assessing the effects of eradication treatment are lacking. Nonetheless, LTx programs generally recommend therapy with aerosolized antibiotics posttransplantation to treat colonization because of the potential benefit of CLAD-free graft survival. However, given the invasive aspect of BAL—although being the most accurate diagnostic method—diagnosis and treatment are often delayed.7,8
Electronic nose (eNose) technology might provide an alternative approach for the detection of BC after LTx. An eNose performs real-time analyses on exhaled breath for patterns in volatile organic compounds (VOCs) derived from bodily processes and the environment. These “breathprint” patterns represent the current health status and can be analyzed using machine learning based on pattern recognition. The potential of eNose technology has been demonstrated in the diagnosis of chronic obstructive pulmonary disease, interstitial lung disease, and asthma,9,10 but its application has only rarely been explored after LTx.11 Small earlier studies in other pulmonary diseases indicate bacterial infection or colonization may be detected using eNose technology.12-14 Given the implications and diagnostic challenges of BC after LTx, we aimed to assess the diagnostic accuracy pattern recognition-based eNose technology to detect BC in LTRs.
MATERIALS AND METHODS
Study Design and Population
We performed a cross-sectional analysis within a prospective, single-center, cohort study assessing diagnostic accuracy of BC after LTx performed at the Erasmus University Medical Centre, Rotterdam, the Netherlands (Netherlands Trials Register Identifier NL9251). Between July 2020 and September 2021, all consecutive LTRs visiting the outpatient clinic were asked to participate. All patients provided written informed consent. The study was approved by the medical ethics committee (MEC-2019-0497).
Patient demographics and cultures were collected from electronic health records. We excluded all patients who received a unilateral LTx, had no available cultures within 6 mo from an eNose measurement, and those who received maintenance inhalation antibiotics at the time of eNose measurement for this analysis because they could not be adequately classified. Of every other patient, 1 measurement was included and classified as BC or non-BC.
BC was deemed present if either
Two or more positive sputum or BAL cultures with the same pathogen, taken at least 1 mo apart, and no antibiotic treatment was started.
One positive sputum or BAL culture, no antibiotic treatment initiated, and interval of eNose measurement and sample no >2 mo.
Non-BC was defined as no presence of BC criteria and at least 1 negative sputum or BAL culture within 6 mo of the eNose measurement. Measurements at the time of alternative diagnoses, such as pneumonia, acute cellular rejection, or unexplained decrease of pulmonary function at the time of the eNose measurement, were excluded.
BALs were performed according to the latest International Society for Heart and LTx consensus on BAL after LTx with three to four 50-mL aliquots.15 Aspiration was performed shortly after instilling each aliquot. Subsequently, semiquantitative cultures were used. Molecular diagnostics were not performed.
Exhaled breath was analyzed in real time using a cloud-connected eNose, SpiroNose (Breathomix, Leiden, the Netherlands). The SpiroNose contains 8 sensor arrays, both inside and outside the SpiroNose, with 7 different types of cross-reactive metal-oxide semiconductor sensors. Each sensor is present in a duplex configuration, allowing for measuring the VOCs in both exhaled breath and ambient air. An elaborate description of the methods and setup have been described previously.16 In short, a SpiroNose measurement contains 5 tidal breaths, followed by an inspiratory capacity maneuver to total lung capacity, a 5-s breath hold, and finally a slow expiration to residual volume. Measurements were performed in duplicate. Sensor responses were transmitted directly to an online analysis platform, the BreathBase. This platform contains the secured online database of Breathomix and is developed to conform to the requirements of standards International Organization for Standardization 27001 (information security) and Nederlandse Norm 7510 (information security in health care). eNose sensor responses were processed and corrected for ambient air as described previously.16,17
Statistical Analysis
Dimensionality reduction of eNose sensor data was achieved by applying partial least squares discriminant analysis. Included samples were randomly split into a trainingand a test set using a 2:1 ratio. Leave-one-out cross-validation was used for training, and model fit was assessed in a test set. Model performance was evaluated by the area under the receiver operating characteristics curve (AUC) and the model sensitivity, specificity, positive predictive value (PPV), negative predictive value (NPV), and accuracy. The overall accuracy of the model on the test set was compared with the no-information rate (NIR), the proportion of the largest class in the set. NIR needs to be exceeded to prove that the obtained model is significant and not a random classifier. A Pvalue of <0.05 was considered significant. The 95% confidence interval was calculated by bootstrapping. Analyses were performed using RStudio (version 2021.09.1) with R software (version 4.1.2) supported with the following packages: mixOmics, caret, and pROC.
RESULTS
In total, 161 patients were assessed (Figure (Figure1),1), of whom 80 were excluded because of lack of available cultures (n=
41), antibiotic treatment (n
=
9), unclear BC status (n
=
20), or because of having received a unilateral LTx (n
=
10). Of the remaining patients, 16 (20%) were classified as BC and 65 (80%) as non-BC. Patients with BC were younger than non-BC (P
=
0.002). In patients with BC, underlying diagnoses were cystic fibrosis (75%) and obstructive diseases (25%), and in non-BC patients, underlying diagnoses were pulmonary fibrosis (43%) and obstructive diseases (43%). Gender, type of transplantation, and time since transplantation were similar. BC patients were mostly colonized with Pseudomonas species (81%). Baseline characteristics are shown in Table Table11.
TABLE 1.
Baseline characteristics
Non-BC (n![]() ![]() | BC (n![]() ![]() | P | |||
---|---|---|---|---|---|
Age, y, median (range) | 59 | (27–71) | 47 | (30–66) | 0.002 |
Gender, n (%) | |||||
![]() | 33 | (51%) | 8 | (50%) | 0.96 |
Time after LTx, y, median (range) | 3.2 | (0.1–14.8) | 4.0 | (0.2–11.7) | 0.41 |
Underlying disease, n (%) | <0.001 | ||||
![]() | 28 | (43%) | 4 | (25%) | |
![]() | 2 | (3%) | 12 | (75%) | |
![]() | 2 | (3%) | – | ||
![]() | 11 | (17%) | – | ||
![]() | 17 | (26%) | – | ||
![]() | 5 | (8%) | – | ||
CLAD development, n (%) | 8 | (12%) | 3 | (19%) | 0.50 |
Chronic sinusitis, n (%) | 1 | (2%) | 5 | (31%) | <0.001 |
A1AT, alpha-1 antitrypsin; CF, cystic fibrosis; CLAD, chronic lung allograft dysfunction; COPD, chronic obstructive pulmonary disease; LTx, lung transplantation; OB, Obliterative bronchiolitis; PAH, Pulmonary arterial hypertension; PF, Pulmonary fibrosis.
In the training set the AUC for the detection of BC was 0.82 (95% confidence interval, 0.63-1.00) with a sensitivity of 60%, a specificity of 100%, an accuracy of 92%, PPV of 100%, and an NPV of 91% (Figure (Figure2).2). In the test set, the AUC was 0.97 (0.92-1.00) with a sensitivity of 67%, a specificity of 100%, a PPV of 100%, and an NPV of 92%. The overall accuracy of the test set was 93%, which was significantly better than the NIR (80%, P=
0.04).
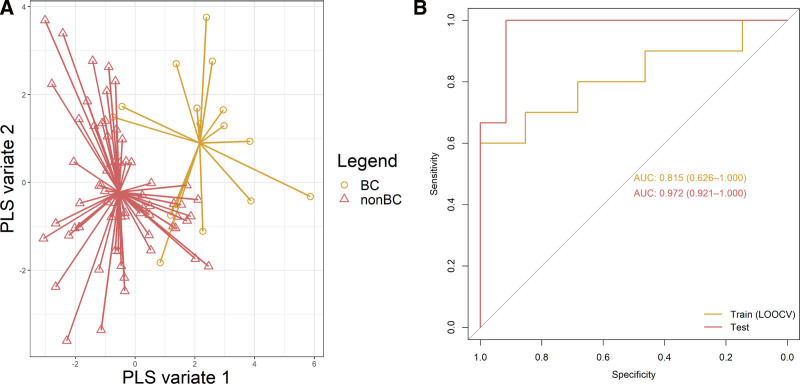
Discrimination between LTR with and without BC. A, Scatterplot of BC and non-BC samples plotted against the first 2 PLS components. Samples of the same class are connected to the class center identifier for improved visual assessment. B, ROC curves of train and test set. AUC, area under the curve; BC, bacterial colonization; LOOCV, leave-one-out cross-validation; non-BC, no bacterial colonization; PLS, partial least square; ROC, receiver operating characteristic.
DISCUSSION
In this study, we demonstrated for the first time that eNose technology can aid in the identification of BC after LTx in a noninvasive matter with high specificity (100%), moderately high sensitivity (67%), and high accuracy (93%).
Untreated BC may result in inferior patient and graft outcomes after transplantation.18,19 Early, successful eradication has been shown to lead to longer CLAD-free survival, longer graft survival, and higher pulmonary function. Nonetheless, adequate monitoring for BC after LTx can be challenging and often requires invasive procedures.
Our findings indicate that BC results in a change of exhaled VOCs and, hence, a distinct breathprint. This builds on prior findings regarding the detection of BC with eNose in small studies in chronic obstructive pulmonary disease, cystic fibrosis, and bronchiectasis, despite differences in definitions of BC between studies and different eNose devices used.12-14
This finding further stresses the promise of eNose for clinical applications, although actual implementation is currently lagging behind, among others due to different eNose devices and lack of external validation and implementation studies.9 Bringing the eNose from promising innovation to an implemented real-time point-of-care test will be the focus of current research projects at our institution.
The lower sensitivity could be related because of the class imbalance in our data set. BC is less frequent than non-BC, the majority class. This could lead to a bias toward the majority class, resulting in a lower sensitivity. Analysis of the breathprint in a larger data set with more LTRs with BC should reveal the sensitivity of detecting BC.
A limitation of this study is that true class labels were determined on the basis of cultures of different, not always BAL, specimens. Additionally, occasionally, there was a period of several mo between specimen culture and eNose measurement. Ideally, true class labels would be determined on the basis of (repeated) BAL samples in a close time frame from the eNose measurement. Likewise, defining colonization based on only 1 positive culture without initiation of antibiotic treatment may lead to overdiagnosis of colonization. Nonetheless, in clinical practice, these are patients without symptoms of acute infection, and in the majority of these patients, colonizing pathogens have been found earlier—beyond the study window. Additionally, the influence of inhaled antibiotics remains unclear because this group was too small for sensitivity analysis in this cohort. Also, 82.4% of the patients with BC were colonized with Pseudomonas species. Although this is the most frequently colonizing species after LTx,20,21 this limits the extrapolation of our results to other species. Furthermore, the influence of chronic sinusitis remains unclear. There are a few studies conducted on detecting chronic sinusitis using eNose technology, most of them using sample headspace analysis instead of exhaled breath analysis. These studies found potential in using eNose technology for detecting sinusitis.22,23 Because only a very small proportion of patients had chronic sinusitis, this is very unlikely to have affected current outcomes. Nonetheless, the results in this consecutive series of LTx patients demonstrate proof of concept and the clear potential for its application.
Overall, we demonstrated that eNose technology may be used to noninvasively detect BC after LTx. Although this is a proof of concept, these results encourage further exploration of eNose technology to detect BC after LTx. Ultimately, after prospective and external validation, eNose technology might be applicable in clinical practice for noninvasive monitoring of LTRs for BC and guide early eradication attempts.
ACKNOWLEDGMENTS
The authors would like to thank the Erasmus MC Thorax Foundation for supporting their research.
Footnotes
J.G.J.V.A. reports personal fees and nonfinancial support from Merck Sharp & Dohme and personal fees from Bristol Myers Squibb's, Boehringer Ingelheim, Amphera, Eli Lilly, Takeda, Bayer, Roche, and Astra Zeneca outside the submitted work. In addition, J.G.J.V.A. has a patent on allogenic tumor cell lysate licensed to Amphera, a patent combination immunotherapy in cancer pending, and a patent biomarker for immunotherapy pending. O.M. reports personal fees from Astra Zeneca, Boehringer Ingelheim, and Novartis outside the submitted work. In addition, O.M. is Chair of the Working Group Heart Failure of the Dutch Society of Cardiology. The other authors declare no conflicts of interest.
M.E.H., O.C.M., D.B., N.W., and N.L.A.d.J. participated in research idea and design. N.W. and N.L.A.d.J. participated in patient inclusion. N.W., N.L.A.d.J., M.E.H., O.C.M., and D.B. participated in data analysis and interpretation. N.W., N.L.A.d.J., M.E.H., O.C.M., R.A.S.H., B.J.M., L.S., D.B., and J.G.J.V.A. participated in drafting and/or critically reviewing the article. R.A.S.H., B.J.M., L.S., and J.G.J.V.A. advised on study design. All authors have read and agreed to the published version of the article.
REFERENCES
Articles from Transplantation Direct are provided here courtesy of Wolters Kluwer Health
Citations & impact
This article has not been cited yet.
Impact metrics
Alternative metrics

Discover the attention surrounding your research
https://www.altmetric.com/details/155283166
Similar Articles
To arrive at the top five similar articles we use a word-weighted algorithm to compare words from the Title and Abstract of each citation.
Diagnostic performance of electronic nose technology in chronic lung allograft dysfunction.
J Heart Lung Transplant, 42(2):236-245, 20 Sep 2022
Cited by: 1 article | PMID: 36283951
Diagnostic accuracy of eNose 'breathprints' for therapeutic drug monitoring of Tacrolimus trough levels in lung transplantation.
J Breath Res, 17(4), 05 Sep 2023
Cited by: 0 articles | PMID: 37582348
Prospective Detection of Early Lung Cancer in Patients With COPD in Regular Care by Electronic Nose Analysis of Exhaled Breath.
Chest, 164(5):1315-1324, 19 May 2023
Cited by: 11 articles | PMID: 37209772 | PMCID: PMC10635840
The smell of lung disease: a review of the current status of electronic nose technology.
Respir Res, 22(1):246, 17 Sep 2021
Cited by: 20 articles | PMID: 34535144 | PMCID: PMC8448171
Review Free full text in Europe PMC