Abstract
Background and objectives
To report the genetic etiologies of Emery-Dreifuss muscular dystrophy (EDMD), limb-girdle muscular dystrophy (LGMD), congenital muscular dystrophy (CMD), and distal muscular dystrophy (DD) in 6 geographically defined areas of the United States.Methods
This was a cross-sectional, population-based study in which we studied the genes and variants associated with muscular dystrophy in individuals who were diagnosed with and received care for EDMD, LGMD, CMD, and DD from January 1, 2008, through December 31, 2016, in the 6 areas of the United States covered by the Muscular Dystrophy Surveillance, Tracking, and Research Network (MD STARnet). Variants of unknown significance (VUSs) from the original genetic test reports were reanalyzed for changes in interpretation.Results
Among 243 individuals with definite or probable muscular dystrophy, LGMD was the most common diagnosis (138 cases), followed by CMD (62 cases), DD (22 cases), and EDMD (21 cases). There was a higher proportion of male individuals compared with female individuals, which persisted after excluding X-linked genes (EMD) and autosomal genes reported to have skewed gender ratios (ANO5, CAV3, and LMNA). The most common associated genes were FKRP, CAPN3, ANO5, and DYSF. Reanalysis yielded more definitive variant interpretations for 60 of 144 VUSs, with a mean interval between the original clinical genetic test of 8.11 years for all 144 VUSs and 8.62 years for the 60 reclassified variants. Ten individuals were found to have monoallelic pathogenic variants in genes known to be primarily recessive.Discussion
This study is distinct for being an examination of 4 types of muscular dystrophies in selected geographic areas of the United States. The striking proportion of resolved VUSs demonstrates the value of periodic re-examinations of these variants. Such re-examinations will resolve some genetic diagnostic ambiguities before initiating repeat testing or more invasive diagnostic procedures such as muscle biopsy. The presence of monoallelic pathogenic variants in recessive genes in our cohort indicates that some individuals with muscular dystrophy continue to face incomplete genetic diagnoses; further refinements in genetic knowledge and diagnostic approaches will optimize diagnostic information for these individuals.Free full text

Genetic Patterns of Selected Muscular Dystrophies in the Muscular Dystrophy Surveillance, Tracking, and Research Network
Abstract
Background and Objectives
To report the genetic etiologies of Emery-Dreifuss muscular dystrophy (EDMD), limb-girdle muscular dystrophy (LGMD), congenital muscular dystrophy (CMD), and distal muscular dystrophy (DD) in 6 geographically defined areas of the United States.
Methods
This was a cross-sectional, population-based study in which we studied the genes and variants associated with muscular dystrophy in individuals who were diagnosed with and received care for EDMD, LGMD, CMD, and DD from January 1, 2008, through December 31, 2016, in the 6 areas of the United States covered by the Muscular Dystrophy Surveillance, Tracking, and Research Network (MD STARnet). Variants of unknown significance (VUSs) from the original genetic test reports were reanalyzed for changes in interpretation.
Results
Among 243 individuals with definite or probable muscular dystrophy, LGMD was the most common diagnosis (138 cases), followed by CMD (62 cases), DD (22 cases), and EDMD (21 cases). There was a higher proportion of male individuals compared with female individuals, which persisted after excluding X-linked genes (EMD) and autosomal genes reported to have skewed gender ratios (ANO5, CAV3, and LMNA). The most common associated genes were FKRP, CAPN3, ANO5, and DYSF. Reanalysis yielded more definitive variant interpretations for 60 of 144 VUSs, with a mean interval between the original clinical genetic test of 8.11 years for all 144 VUSs and 8.62 years for the 60 reclassified variants. Ten individuals were found to have monoallelic pathogenic variants in genes known to be primarily recessive.
Discussion
This study is distinct for being an examination of 4 types of muscular dystrophies in selected geographic areas of the United States. The striking proportion of resolved VUSs demonstrates the value of periodic re-examinations of these variants. Such re-examinations will resolve some genetic diagnostic ambiguities before initiating repeat testing or more invasive diagnostic procedures such as muscle biopsy. The presence of monoallelic pathogenic variants in recessive genes in our cohort indicates that some individuals with muscular dystrophy continue to face incomplete genetic diagnoses; further refinements in genetic knowledge and diagnostic approaches will optimize diagnostic information for these individuals.
Introduction
Four classic but less common forms of muscular dystrophy are Emery-Dreifuss muscular dystrophy (EDMD), limb-girdle muscular dystrophy (LGMD), congenital muscular dystrophy (CMD), and distal muscular dystrophy (DD). These disorders share some overlapping associated genes and some phenotypic features. A number of epidemiologic studies that include various combinations of these muscular dystrophies have been published over the years, ranging from broad-based reports1 and a genetic database mining study of LGMD2 to focused population studies on individual genes or even individual variants.3-8 Several geographically defined population-based studies have been conducted for one or more of these muscular dystrophies, primarily outside the United States.9-14 Variants of unknown significance (VUSs) often complicate the interpretation of genetic test reports, either when they are the primary findings or when they are secondary findings in addition to pathogenic or likely pathogenic variants. The presence of such VUSs often leads to ambiguous conclusions from genetic test reports.
The Muscular Dystrophy Surveillance, Tracking, and Research Network (MD STARnet) has reported general sociodemographic and clinical characteristics of individuals with these MDs in specific regions of the United States15,16; however, MD STARnet has not previously analyzed detailed patterns of genes and variants associated with the 4 less common MD types. In this report, we characterize such patterns of genetic test results for the 4 MDs under investigation. Our primary analysis is followed by a review of information in publicly available databases that can be used to inform interpretation and classification of VUSs.
Methods
Standard Protocol Approvals, Registrations, and Patient Consents
Study activities were conducted under protocols that were approved by the institutional review board and/or public health authority for surveillance at each MD STARnet site. These activities qualified for waivers of consent at each site.
Study Population and Data Sources (Standard MD STARnet Methodology)
Individuals with EDMD, LGMD, CMD, and DD were identified through MD STARnet surveillance using previously described methods.15,17 MD STARnet is a multisite, population-based muscular dystrophy surveillance system in the United States that currently identifies individuals who were diagnosed with one of 8 muscular dystrophies (Becker [BMD], CMD, DD, Duchenne [DMD], EDMD, facioscapulohumeral [FSHD], LGMD, and myotonic [DM]). Cohort eligibility for case abstraction included meeting the following criteria from January 1, 2008, to December 31, 2016: clinical diagnosis of an eligible MD and receipt of clinical care and residency in Colorado (CO), Iowa (IA), South Carolina (SC), the Piedmont region of North Carolina (NC), a 21-county area in Western New York State (wNY), or Utah (UT/NV) (Figure).
Case Abstraction (Standard MD STARnet Methodology)
Potential cases were identified by International Classification of Disease codes (ICD-9-CM: 359.0, 359.1, 359.21; ICD-10: G71.0, G71.1)18 from clinic and administrative data and screened for cohort eligibility. Data abstraction of medical records for eligible cases began in 2016 by trained abstractors who reviewed and abstracted medical records for clinical data during the follow-up period. Collected data included demographic characteristics, medical history (including earliest signs and symptoms), diagnostic testing (including genetic testing, muscle biopsy immunostaining, skin biopsy immunostaining, diagnostic Western blot, and/or diagnostic MRI), clinical care, and family history of muscular dystrophy.
Case Review (Standard MD STARnet Methodology)
Abstracted clinical data for each eligible case were reviewed by a panel of MD STARnet neuromuscular physicians who assigned clinical MD type and a case classification of definite, probable, possible, asymptomatic, or not an eligible MD (eAppendix 1, links.lww.com/NXG/A649). MD type was assigned by the panel based on defined patterns of clinical signs and symptoms for each type, as well as diagnostic findings. A case was categorized as definite if there were documented clinical symptoms referable to one of the MD types, a genetic report of DNA analysis with the identification of pathogenic findings in the patient or a family history of genetically confirmed case status in a family member showing a recognizable inheritance pattern, and other confirmatory testing. Probable cases were defined by documented clinical symptoms referable to an MD type and supported by family history and laboratory results referable to one of the selected MDs, but without meeting the criteria for a definite case. Asymptomatic cases were those who had positive genetic test results for an associated gene but showed no signs or symptoms of muscular dystrophy.
Data Pooling
A pooled, analytic data set was created and included clinical data from each MD STARnet site for individuals classified as having definite, probable, and asymptomatic diagnoses of EDMD, LGMD, CMD, and DD. From the pooled data, we excluded asymptomatic individuals, individuals whose genetic tests showed benign genes, individuals whose abstracted genetic test results lacked sufficient details for analysis, and individuals who resided in Nevada and were ascertained under UT authority (cases residing in UT were included in the analysis), the latter due to a small, unrepresentative subgroup (Figure). The asymptomatic cases were excluded because the clinical diagnosis assignment could not be confirmed.
Variables
We studied the ages when the diagnosis was confirmed by genetic testing or the first abnormal neuromuscular diagnostic test (serum creatine kinase [CK], EMG, and/or muscle biopsy). Racial and ethnic classifications were recorded when available. Family history status was defined as yes, no, and unknown.
Statistical Analysis
Frequencies and proportions were calculated for categorical variables; mean and SD or range were used for continuous variables. The distribution of age in years when 50% (25%, 75%) of the study group had each outcome was estimated using the Kaplan-Meier estimator. A minimum of 10 individuals per table cell were required to report numbers and percentages for demographic data to avoid potentially compromising patient privacy, including racial and ethnic identities.
Pathogenicity of Variants and Reanalysis of VUSs
The lists of pathogenic variants and VUSs were obtained from the pooled MD STARnet database. The information in these fields was supplemented by additional variants identified in a free text “description” field of the database. Duplicate entries were deleted, and typographical errors were corrected. The original variant classification categories in the database were pathogenic, VUS, normal, and unknown, with “normal” corresponding to the currently accepted variant classification “benign” and “unknown” corresponding to a variant classification that could not be confirmed based on the abstracted information. In light of the possibility that some of the VUSs and unassigned (“unknown”) variants may be subject to reinterpretation, we included the unassigned variants in the general category of VUS and re-evaluated this combined variant list, with each variant assessed by a pair of authors who determined the current ACMG classification19 and Revel score20 (franklin.genoox.com), along with ClinVar21 interpretation and gnomAD22 allele frequencies. When a pair of authors did not agree on the current ACMG classification of a particular variant, they reviewed their findings with each other to reach consensus, consulting with the lead author (P.B.K.) when needed. Using information from these online databases, we then determined whether a VUS would still be classified as a VUS or be reclassified for the purposes of this analysis as pathogenic, likely pathogenic, likely benign, or benign. For those variants that could be reclassified for this analysis, we determined whether the change would either alter the original overall interpretation of the primary genetic test finding or eliminate a secondary finding. A secondary finding is defined as a VUS that is noted in a genetic test report in the presence of a pathogenic or likely pathogenic variant in a different gene. We also recorded the intervals between the original clinical genetic test date and the date of completion of our reanalysis (December 13, 2022) and calculated mean and median intervals for each diagnosis (EDMD, LGMD, CMD, and DD).
Monoallelic Pathogenic Variants in Genes With Recessive Inheritance (“Single Hits”)
We reviewed all pathogenic variants of muscular dystrophy–associated genes that are known to have recessive or primarily recessive patterns of inheritance and noted when one pathogenic allele was unaccompanied by a second pathogenic allele for the same gene (“single hit”).
Missing Variants
At the time of abstraction, pathogenic variant information from genetic test reports was entered into the MD STARnet database. However, some variants did not have nucleotide or amino acid positions entered, rendering them impossible to characterize or analyze further. In some cases where a VUS test result was missing cDNA or amino acid change information in the structured part of the MD STARnet database, this information was found in the free text “description” field of the database. In those cases, study authors filled in the missing data manually.
Data Availability
Owing to privacy concerns, data from MD STARnet are not publicly available. Researchers interested in MD STARnet data can contact vog.cdc@tenRATSDM.
Results
Demographics
We first examined key demographic data among the 243 individuals in our cohort to characterize basic information (Table 1). 64.6% of our cohort was male and 35.4% was female. The unexpectedly higher proportion of male individuals compared with female individuals was present in all 4 diagnostic categories, despite the presence of only one X-linked gene (EMD) among the commonly associated genes. Regarding other aspects of our cohort, 44.4% had no known family history of the disease in question. The mean age at diagnosis was 27.2 years, and the median age at diagnosis was 22.1 years, and as noted above, the diagnosis had to be made in the 2008–2016 period for inclusion. The mean age at the last abstracted clinic visit was 37 years, and the median age at the last clinic visit was 35 years. These mean and median ages were younger in the CMD group (20.7 and 18 years, respectively) compared with the other groups.
Table 1
Demographic Features of 243 Individuals With EDMD, LGMD, CMD, and DD, With Comparisons Among the 4 Muscular Dystrophy Categories for Each Set of Variables
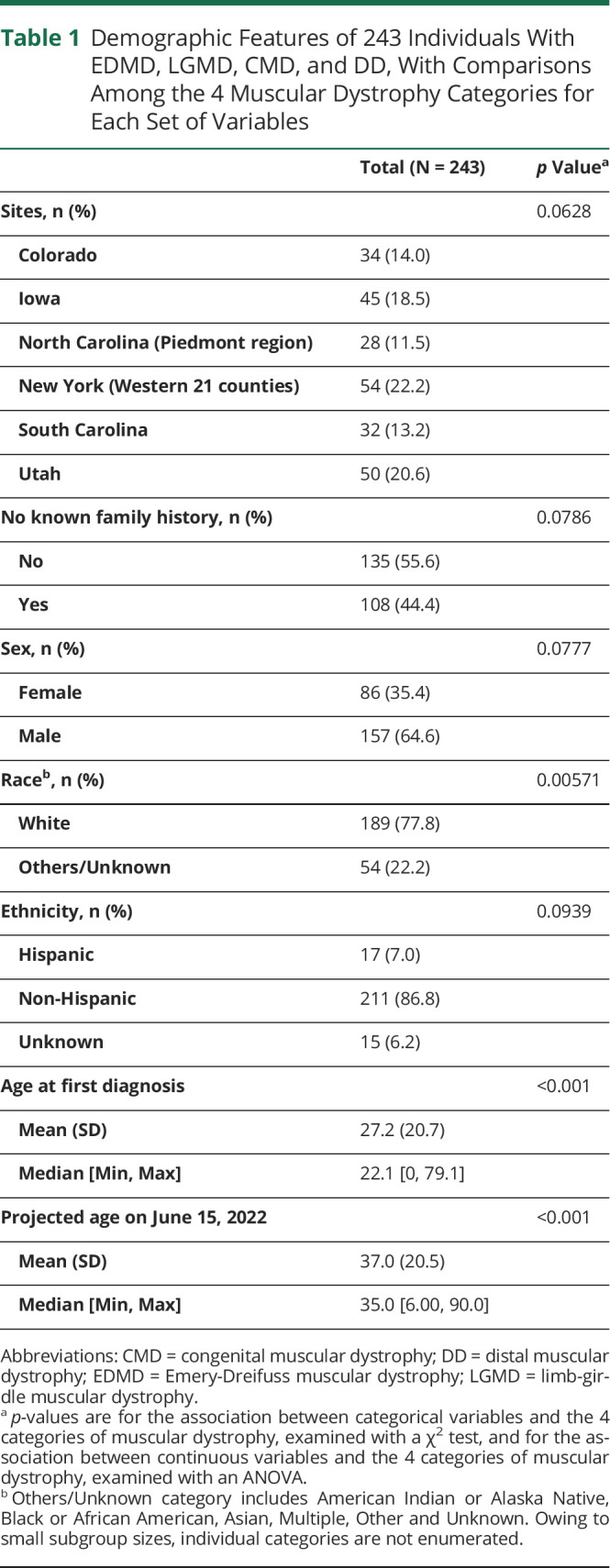
Total (N = 243) | p Valuea | |
Sites, n (%) | 0.0628 | |
![]() | 34 (14.0) | |
![]() | 45 (18.5) | |
![]() | 28 (11.5) | |
![]() | 54 (22.2) | |
![]() | 32 (13.2) | |
![]() | 50 (20.6) | |
No known family history, n (%) | 0.0786 | |
![]() | 135 (55.6) | |
![]() | 108 (44.4) | |
Sex, n (%) | 0.0777 | |
![]() | 86 (35.4) | |
![]() | 157 (64.6) | |
Raceb, n (%) | 0.00571 | |
![]() | 189 (77.8) | |
![]() | 54 (22.2) | |
Ethnicity, n (%) | 0.0939 | |
![]() | 17 (7.0) | |
![]() | 211 (86.8) | |
![]() | 15 (6.2) | |
Age at first diagnosis | <0.001 | |
![]() | 27.2 (20.7) | |
![]() | 22.1 [0, 79.1] | |
Projected age on June 15, 2022 | <0.001 | |
![]() | 37.0 (20.5) | |
![]() | 35.0 [6.00, 90.0] |
Abbreviations: CMD = congenital muscular dystrophy; DD = distal muscular dystrophy; EDMD = Emery-Dreifuss muscular dystrophy; LGMD = limb-girdle muscular dystrophy.
Category Distributions and Genetic Findings
As 4 major categories of muscular dystrophy were represented in our cohort, we examined the distribution of individuals among these categories. LGMD was the most common clinical diagnosis, followed by CMD, with DD and EDMD being the least common and nearly equivalent to each other numerically (Table 2). Overall, 60.1% of the cohort had a definite classification, with LGMD having the lowest proportion (Table 2). As originally characterized by the clinical genetic test reports, the associated genes with the highest overall occurrence of pathogenic variants, excluding VUSs, in the cohort were FKRP, CAPN3, ANO5, and COL6A1 (eTable 1, links.lww.com/NXG/A650), all of which are most commonly found in LGMD, except COL6A1, which is typically found in CMD (eTable 2). In EDMD, the genes with the most frequent pathogenic variants were EMD and LMNA. In CMD, the genes with the most frequent pathogenic variants were COL6A1, LAMA2, COL6A3, COL6A2, and FKRP. For DD, the genes with the most frequent pathogenic variants were DYSF and GNE. Most of the variants were pathogenic for CMD and LGMD, whereas VUSs were proportionately more frequent for EDMD and DD (eTable 3). The proportions of male and female individuals were analyzed in aggregate, both before and after exclusion of the X-linked gene EMD and autosomal genes previously found in a higher proportion of male individuals (ANO5, CAV3, and LMNA)23 (eTable 4).
Table 2
Clinical Classification of the 243 Individuals With Certainty of Diagnoses
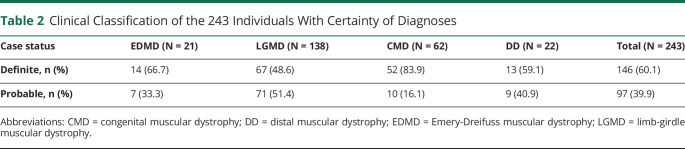
Case status | EDMD (N = 21) | LGMD (N = 138) | CMD (N = 62) | DD (N = 22) | Total (N = 243) |
Definite, n (%) | 14 (66.7) | 67 (48.6) | 52 (83.9) | 13 (59.1) | 146 (60.1) |
Probable, n (%) | 7 (33.3) | 71 (51.4) | 10 (16.1) | 9 (40.9) | 97 (39.9) |
Abbreviations: CMD = congenital muscular dystrophy; DD = distal muscular dystrophy; EDMD = Emery-Dreifuss muscular dystrophy; LGMD = limb-girdle muscular dystrophy.
Pathogenicity of Variants and Reanalysis of VUSs and Unassigned Variants
Given the high number of VUSs that appear in clinical genetic testing, we asked whether genetic findings could be refined by reanalysis using online databases. Before data cleaning, we identified 162 pathogenic variants, 169 VUSs, and 10 unassigned variants. After data cleaning and review of the initial abstraction results, we identified 162 pathogenic variants and 179 VUSs for a total of 341 variants (Figure). Among the 179 VUS results reviewed, 35 were classified as having missing data with the following breakdown: 26 were missing cDNA change or amino acid change information, 4 had unverifiable information, 4 only listed intervening sequence information, and 1 had mtDNA information, yielding 144 VUSs with adequate information for analysis (eTable 5, links.lww.com/NXG/A650). Our review of those 144 VUSs using currently accepted standard classification systems and databases yielded reclassification of 23 variants to pathogenic or likely pathogenic and 37 variants to benign or likely benign. Eighty-four VUSs remained unchanged (Table 3). The reclassifications changed the interpretations of primary genetic test findings for 35 variants and eliminated secondary findings for 23 variants (Table 4 and eTables 6 and 7). Of note, there were individuals with multiple VUSs, thus the number of individuals with reinterpretations of primarily genetic findings was 28 and the number of individuals with elimination of secondary findings was 18 (Table 4). The mean intervals between the original clinical genetic test report and the time of VUS reanalysis was 8.11 years for all 144 VUSs analyzed (Table 5) and 8.62 years for the 60 reclassified VUSs (Table 6).
Table 3
Clinical MD Types and ACMG Classifications for VUSs
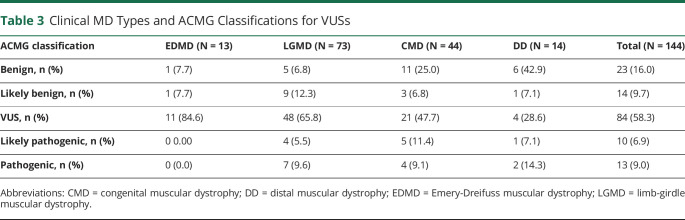
ACMG classification | EDMD (N = 13) | LGMD (N = 73) | CMD (N = 44) | DD (N = 14) | Total (N = 144) |
Benign, n (%) | 1 (7.7) | 5 (6.8) | 11 (25.0) | 6 (42.9) | 23 (16.0) |
Likely benign, n (%) | 1 (7.7) | 9 (12.3) | 3 (6.8) | 1 (7.1) | 14 (9.7) |
VUS, n (%) | 11 (84.6) | 48 (65.8) | 21 (47.7) | 4 (28.6) | 84 (58.3) |
Likely pathogenic, n (%) | 0 0.00 | 4 (5.5) | 5 (11.4) | 1 (7.1) | 10 (6.9) |
Pathogenic, n (%) | 0 (0.0) | 7 (9.6) | 4 (9.1) | 2 (14.3) | 13 (9.0) |
Abbreviations: CMD = congenital muscular dystrophy; DD = distal muscular dystrophy; EDMD = Emery-Dreifuss muscular dystrophy; LGMD = limb-girdle muscular dystrophy.
Table 4
Changes in Interpretation and Elimination of Secondary Findings for VUSs by Clinical MD Type
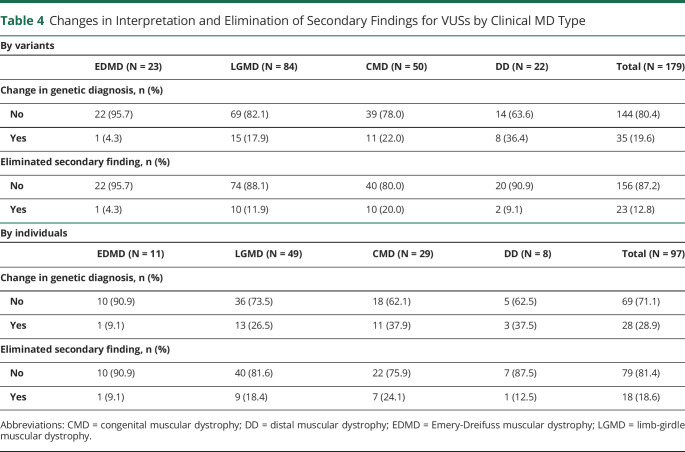
By variants | |||||
EDMD (N = 23) | LGMD (N = 84) | CMD (N = 50) | DD (N = 22) | Total (N = 179) | |
Change in genetic diagnosis, n (%) | |||||
![]() | 22 (95.7) | 69 (82.1) | 39 (78.0) | 14 (63.6) | 144 (80.4) |
![]() | 1 (4.3) | 15 (17.9) | 11 (22.0) | 8 (36.4) | 35 (19.6) |
Eliminated secondary finding, n (%) | |||||
![]() | 22 (95.7) | 74 (88.1) | 40 (80.0) | 20 (90.9) | 156 (87.2) |
![]() | 1 (4.3) | 10 (11.9) | 10 (20.0) | 2 (9.1) | 23 (12.8) |
By individuals | |||||
EDMD (N = 11) | LGMD (N = 49) | CMD (N = 29) | DD (N = 8) | Total (N = 97) | |
Change in genetic diagnosis, n (%) | |||||
![]() | 10 (90.9) | 36 (73.5) | 18 (62.1) | 5 (62.5) | 69 (71.1) |
![]() | 1 (9.1) | 13 (26.5) | 11 (37.9) | 3 (37.5) | 28 (28.9) |
Eliminated secondary finding, n (%) | |||||
![]() | 10 (90.9) | 40 (81.6) | 22 (75.9) | 7 (87.5) | 79 (81.4) |
![]() | 1 (9.1) | 9 (18.4) | 7 (24.1) | 1 (12.5) | 18 (18.6) |
Abbreviations: CMD = congenital muscular dystrophy; DD = distal muscular dystrophy; EDMD = Emery-Dreifuss muscular dystrophy; LGMD = limb-girdle muscular dystrophy.
Table 5
Time Intervals Between Clinical Genetic Tests and Reanalysis of All 144 VUSs That Were Reanalyzed (y)
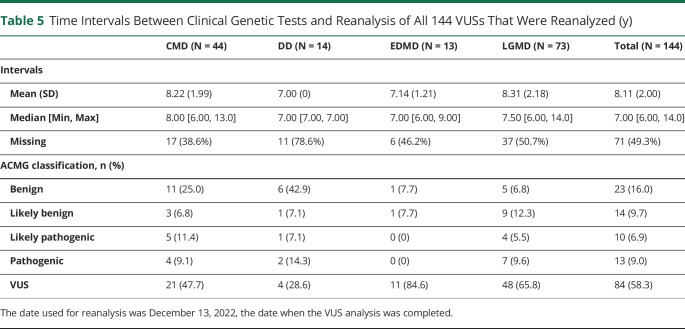
CMD (N = 44) | DD (N = 14) | EDMD (N = 13) | LGMD (N = 73) | Total (N = 144) | |
Intervals | |||||
![]() | 8.22 (1.99) | 7.00 (0) | 7.14 (1.21) | 8.31 (2.18) | 8.11 (2.00) |
![]() | 8.00 [6.00, 13.0] | 7.00 [7.00, 7.00] | 7.00 [6.00, 9.00] | 7.50 [6.00, 14.0] | 7.00 [6.00, 14.0] |
![]() | 17 (38.6%) | 11 (78.6%) | 6 (46.2%) | 37 (50.7%) | 71 (49.3%) |
ACMG classification, n (%) | |||||
![]() | 11 (25.0) | 6 (42.9) | 1 (7.7) | 5 (6.8) | 23 (16.0) |
![]() | 3 (6.8) | 1 (7.1) | 1 (7.7) | 9 (12.3) | 14 (9.7) |
![]() | 5 (11.4) | 1 (7.1) | 0 (0) | 4 (5.5) | 10 (6.9) |
![]() | 4 (9.1) | 2 (14.3) | 0 (0) | 7 (9.6) | 13 (9.0) |
![]() | 21 (47.7) | 4 (28.6) | 11 (84.6) | 48 (65.8) | 84 (58.3) |
The date used for reanalysis was December 13, 2022, the date when the VUS analysis was completed.
Table 6
Time Intervals Between Clinical Genetic Tests and Reanalysis of 60 VUSs That Were Reclassified (y)
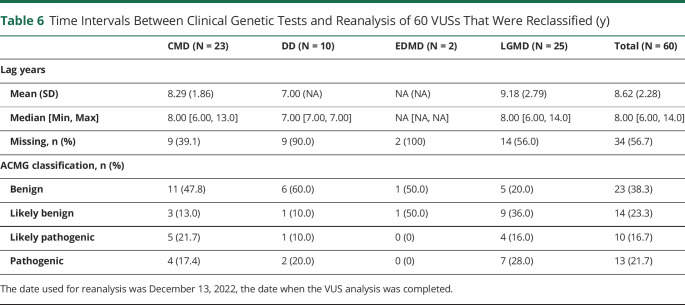
CMD (N = 23) | DD (N = 10) | EDMD (N = 2) | LGMD (N = 25) | Total (N = 60) | |
Lag years | |||||
![]() | 8.29 (1.86) | 7.00 (NA) | NA (NA) | 9.18 (2.79) | 8.62 (2.28) |
![]() | 8.00 [6.00, 13.0] | 7.00 [7.00, 7.00] | NA [NA, NA] | 8.00 [6.00, 14.0] | 8.00 [6.00, 14.0] |
![]() | 9 (39.1) | 9 (90.0) | 2 (100) | 14 (56.0) | 34 (56.7) |
ACMG classification, n (%) | |||||
![]() | 11 (47.8) | 6 (60.0) | 1 (50.0) | 5 (20.0) | 23 (38.3) |
![]() | 3 (13.0) | 1 (10.0) | 1 (50.0) | 9 (36.0) | 14 (23.3) |
![]() | 5 (21.7) | 1 (10.0) | 0 (0) | 4 (16.0) | 10 (16.7) |
![]() | 4 (17.4) | 2 (20.0) | 0 (0) | 7 (28.0) | 13 (21.7) |
The date used for reanalysis was December 13, 2022, the date when the VUS analysis was completed.
Monoallelic Pathogenic Variants in Recessive Genes (“Single Hits”)
The problem of VUSs is often accompanied by the dilemma presented by single pathogenic variants identified in genes that are known to be recessive, leaving diagnostic uncertainty. We identified 10 individuals with such monoallelic pathogenic variants in genes with recessive or primarily recessive patterns of inheritance (“single hits”). These individuals have ambiguous genetic diagnoses despite the presence of pathogenic variants. These single hits were most common for LGMD (eTable 8, links.lww.com/NXG/A650), and after removing individuals with duplicate pathogenic genes, the most common gene in which this phenomenon was observed was FKRP (eTable 9). For all 10 affected symptomatic individuals, there were no other findings in the original genetic test report to indicate the presence of alternative genetic diagnoses.
Cases With Pathogenic Variants in Multiple Genes
The possibility of digenic Mendelian inheritance as an explanation for some genetically unsolved cases of muscular dystrophy has been raised. In our cohort, 3 individuals were found to have pathogenic or likely pathogenic alleles in 2 different genes: (1) DNAJB6 c.271T>G (p.F91V) paired with GAA c.546G>A (p.T183 = ); (2) TTN c.70493dupA paired with FKRP c.826C>A (p.L2761); and (3) ANO5 c.191dupA paired with TTN c.85692_85696delAGCTT.
Discussion
Prior geographically defined studies of EDMD, LGMD, CMD, and DD in the United States consist principally of 2 MD STARnet reports that did not include the genetic analysis presented here.15,16 In other countries, epidemiologic studies focusing on LGMD have been published from Austria,9 Chile,10 Italy,11,23 the Netherlands,12 and Spain13 and CMD from Italy.14 These and other studies from around the world that covered these diagnoses provide valuable information but had differences in scope from our study because they did not include genetic subtype information,24 were broad-based general studies of muscle diseases or neuromuscular disorders,25-35 or focused on genetic subsets of one of these muscular dystrophies.36-41 Our findings are consistent with prior studies for the relative frequency of the 4 MD types and the common genes identified in our cohort.
The skewed sex ratio is striking and cannot be explained by expected genetic distributions, given that only one major gene, EMD, is X-linked.42 There are several autosomal genes that have previously been associated with male-predominant ratios, including ANO5, CAV3, and LMNA.23 A study of EDMD, LGMD, CMD, and DD from the prior MD STARnet cycle only detected a skewed male/female ratio in EDMD.16 Although the available information does not enable us to draw definitive conclusions about the origins of this sex distribution, it is plausible that female individuals affected by these categories of muscular dystrophy were diagnosed at lower rates or sought specialty care less often than affected male individuals during this more recent surveillance period. Milder manifestations in female individuals could account for either explanation. As the most widely known muscular dystrophy, Duchenne muscular dystrophy (DMD) is X-linked and almost exclusively affects male individuals; there may be a misperception that muscular dystrophy of all kinds does not tend to affect female individuals. It is thus important that outreach efforts for the medical community and for the general public emphasize that both female and male individuals can be affected by many of the subtypes of muscular dystrophy.
The excess of individuals in our cohort with probable rather than definite diagnoses indicates that a gap in confirmatory genetic diagnosis persists in these categories of muscular dystrophy. Of note, the case definitions (eAppendix 1, links.lww.com/NXG/A649) classify affected individuals with a family history of genetic confirmation as definite cases. The percentage of probable cases is highest for LGMD, similar to the high unsolved rates for this type of muscular dystrophy found on both clinical genetic testing and research-based genomic analyses.43-45 This may in part be due to uneven access to genetic testing in some populations.
The common occurrence of VUSs in clinical genetic test reports and the unexpectedly high rate of reclassification on reanalysis of these VUSs in this study indicate that further advances are needed in genetic diagnostic technology and interpretation to improve the accuracy and detection rate of genetic testing. At the very least, a basic scan of VUSs identified on clinical genetic testing using online databases is warranted when the original genetic test is more than a few years old. In light of the frequent posting of new online resources for variant interpretation, we recommend consulting with a neuromuscular neurologist, geneticist, or genetic counselor with expertise in these resources to determine which ones to use at a given time.
Cases in which a monoallelic pathogenic variant (“single hit”) is unaccompanied by a pathogenic variant in the same gene on the other allele, for genes known to have recessive or primarily recessive inheritance, are frustrating for the patients and clinicians involved because it leaves the genetic diagnosis without a full resolution. Approaches that promise to improve the genetic diagnosis of muscular dystrophies include transcriptome analysis (RNAseq), computational reanalysis to detect more subtle changes such as splice variants, and long read sequencing. Long read sequencing in particular holds promise to find the “second hits” for those individuals with monoallelic pathogenic variants in genes with recessive inheritance.46
It has been postulated that there may be rare cases in which variants at 2 different loci may together cause disease. For muscular dystrophy, this is best documented for facioscapulohumeral muscular dystrophy type 2, caused by variants in SMCHD1 paired with a D4Z4 allele harboring a polyadenylation signal.47,48 There are sparse reports of compound pathogenic variants in different genes potentially causing muscular dystrophy, including SCGB paired with SCGD49 and COL6A1 paired with COL6A2.50 We found only 3 cases of potential digenic inheritance in our cohort; more extensive studies are required to determine whether both variants are necessary and sufficient to cause disease in these circumstances.
Our study has some limitations. The subgroups for EDMD and DD were small, although the presence of expected common genes in those subgroups indicates that they were to some extent representative of broader populations with these disease categories. The absence of ANO5 in the DD group was likely because of the small cohort size. The numbers for some genetic subtypes did not meet the MD STARnet reporting threshold of at least 10 cases. Thus, we were not able to present details of the distributions of certain variables within these subtypes such as sex ratios. As genetic testing was performed at different times at different diagnostic facilities, variant interpretation practices likely varied throughout the cohort, although all clinical genetic diagnostic test facilities in the United States are required to qualify for and maintain Clinical Laboratory Improvement Amendment certification, providing some standardization in variant interpretation practices over time. Beyond variant reanalysis, reanalysis of raw sequence data and the use of newer technologies such as nanopore whole-genome long read sequencing46 and whole-transcriptome sequencing (RNAseq), as well as review of muscle imaging studies, could yield additional meaningful diagnostic information. However, MD STARnet does not collect raw sequence data, genomic DNA samples, specimens from muscle biopsies, or images from muscle ultrasound and MRI studies; thus those types of investigations are beyond the scope of this study.
EDMD, LGMD, CMD, and DD collectively comprise a significant portion of the muscular dystrophy population. Their genetic heterogeneity, compared with more common muscular dystrophies such as dystrophinopathies (DMD and BMD), facioscapulohumeral muscular dystrophy (FSHD), and myotonic dystrophy (DM1 and DM2), leads to distinct challenges in diagnosis, prognosis, and management. Our findings indicate that periodic reanalysis of VUSs using publicly available databases will at times yield new information. It will be important to continue characterizing these MDs to optimize genetic diagnosis, clinical management, and research studies that will help lead to novel therapies. Encouragingly, investigational therapies are already undergoing human clinical trials for some of these muscular dystrophies, providing a great deal of hope for the future.
Acknowledgment
The authors thank Kristin M. Conway, PhD, at the Department of Epidemiology, College of Public Health, University of Iowa for assistance with this project. Intermountain Healthcare was a source for some of the data from the Utah site for this study.
Glossary
BMD | Becker muscular dystrophy |
CMD | congenital muscular dystrophy |
DD | distal muscular dystrophy |
DMD | Duchenne muscular dystrophy |
EDMD | Emery-Dreifuss muscular dystrophy |
LGMD | limb-girdle muscular dystrophy |
VUS | variants of unknown significance |
Appendix. Authors
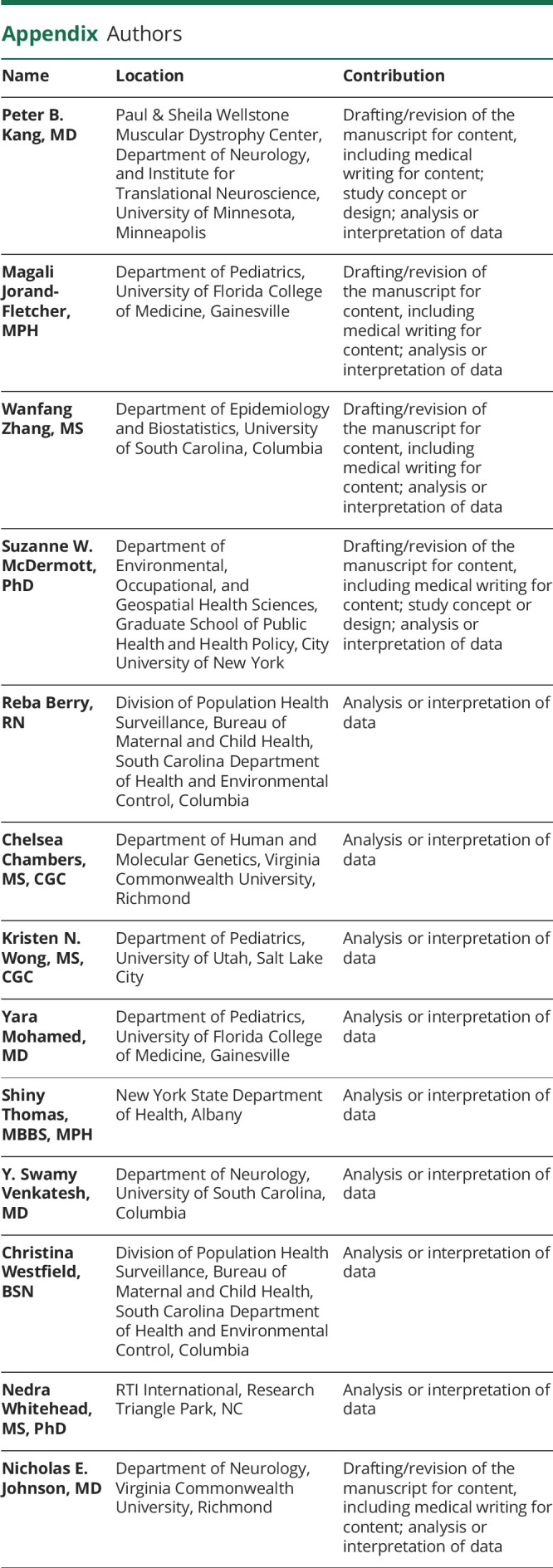
Name | Location | Contribution |
Peter B. Kang, MD | Paul & Sheila Wellstone Muscular Dystrophy Center, Department of Neurology, and Institute for Translational Neuroscience, University of Minnesota, Minneapolis | Drafting/revision of the manuscript for content, including medical writing for content; study concept or design; analysis or interpretation of data |
Magali Jorand-Fletcher, MPH | Department of Pediatrics, University of Florida College of Medicine, Gainesville | Drafting/revision of the manuscript for content, including medical writing for content; analysis or interpretation of data |
Wanfang Zhang, MS | Department of Epidemiology and Biostatistics, University of South Carolina, Columbia | Drafting/revision of the manuscript for content, including medical writing for content; analysis or interpretation of data |
Suzanne W. McDermott, PhD | Department of Environmental, Occupational, and Geospatial Health Sciences, Graduate School of Public Health and Health Policy, City University of New York | Drafting/revision of the manuscript for content, including medical writing for content; study concept or design; analysis or interpretation of data |
Reba Berry, RN | Division of Population Health Surveillance, Bureau of Maternal and Child Health, South Carolina Department of Health and Environmental Control, Columbia | Analysis or interpretation of data |
Chelsea Chambers, MS, CGC | Department of Human and Molecular Genetics, Virginia Commonwealth University, Richmond | Analysis or interpretation of data |
Kristen N. Wong, MS, CGC | Department of Pediatrics, University of Utah, Salt Lake City | Analysis or interpretation of data |
Yara Mohamed, MD | Department of Pediatrics, University of Florida College of Medicine, Gainesville | Analysis or interpretation of data |
Shiny Thomas, MBBS, MPH | New York State Department of Health, Albany | Analysis or interpretation of data |
Y. Swamy Venkatesh, MD | Department of Neurology, University of South Carolina, Columbia | Analysis or interpretation of data |
Christina Westfield, BSN | Division of Population Health Surveillance, Bureau of Maternal and Child Health, South Carolina Department of Health and Environmental Control, Columbia | Analysis or interpretation of data |
Nedra Whitehead, MS, PhD | RTI International, Research Triangle Park, NC | Analysis or interpretation of data |
Nicholas E. Johnson, MD | Department of Neurology, Virginia Commonwealth University, Richmond | Drafting/revision of the manuscript for content, including medical writing for content; analysis or interpretation of data |
Study Funding
This publication was supported by the Cooperative Agreement numbers DD001126, DD001119, DD001123, DD001116, DD001117, DD001108, DD001120, DD001054, DD001242, DD001243, DD001245, DD001248, DD001249, DD001252, and DD001255, funded by the Centers for Disease Control and Prevention (CDC). The findings and conclusions in this report are those of the authors and do not necessarily represent the official position of the Centers for Disease Control and Prevention. The Iowa site was additionally supported by DD001247. Partial support for all data sets with the Utah Population Database (UPDB) was provided by the University of Utah Huntsman Cancer Institute and the Huntsman Cancer Institute Cancer Center Support Grant, P30 CA042014, from the National Cancer Institute.
Disclosure
P.B. Kang has served on advisory boards for Sarepta Therapeutics, NS Pharma, and Teneofour; and has served as a consultant for Novartis and Neurogene. M. Jorand-Fletcher reports no disclosures. W. Zhang reports no disclosures. S.W. McDermott reports no disclosures. R. Berry reports no disclosures. C. Chambers reports no disclosures. K.N. Wong reports no disclosures. Y. Mohamed reports no disclosures. S. Thomas reports no disclosures. Y.S. Venkatesh reports no disclosures. C. Westfield reports no disclosures. N. Whitehead reports no disclosures. N.E. Johnson has received grant funding from the NIH (R01 NS104010 and R21 TR003184), CDC (U01 DD001242), and the FDA (R01 FD006071). He receives research funds from Dyne, AveXis, Vertex Pharmaceuticals, Fulcrum Therapeutics, ML Bio, Sarepta, Triplet Therapeutics, Avidity Biosciences, and AMO Pharma. He has provided consultation for AMO Pharma, AveXis, Fulcrum Therapeutics, Dyne, Avidity, Vertex, and Entrada. He receives licensing fees from the University of Rochester for the CCMDHI and CMTHI. Full disclosure form information provided by the authors is available with the full text of this article at Neurology.org/NG.
References
Articles from Neurology: Genetics are provided here courtesy of American Academy of Neurology
Citations & impact
Impact metrics
Alternative metrics

Discover the attention surrounding your research
https://www.altmetric.com/details/156732443
Article citations
Congenital LMNA-Related Muscular Dystrophy in Paediatrics: Cardiac Management in Monozygotic Twins.
Int J Mol Sci, 25(11):5836, 27 May 2024
Cited by: 0 articles | PMID: 38892025 | PMCID: PMC11171958
Similar Articles
To arrive at the top five similar articles we use a word-weighted algorithm to compare words from the Title and Abstract of each citation.
Limb-Girdle Muscular Dystrophy Overview – RETIRED CHAPTER, FOR HISTORICAL REFERENCE ONLY
University of Washington, Seattle, Seattle (WA), 30 Aug 2012
Cited by: 0 articles | PMID: 20301582
ReviewBooks & documents Free full text in Europe PMC
Muscle Magnetic Resonance Imaging in Patients with Various Clinical Subtypes of LMNA-Related Muscular Dystrophy.
Chin Med J (Engl), 131(12):1472-1479, 01 Jun 2018
Cited by: 8 articles | PMID: 29893365 | PMCID: PMC6006825
LMNA-related muscular dystrophy: Identification of variants in alternative genes and personalized clinical translation.
Front Genet, 14:1135438, 24 Mar 2023
Cited by: 3 articles | PMID: 37035729 | PMCID: PMC10080029
Characterization of individuals with selected muscular dystrophies from the expanded pilot of the Muscular Dystrophy Surveillance, Tracking and Research Network (MD STARnet) in the United States.
Birth Defects Res, 113(7):560-569, 24 Jul 2020
Cited by: 4 articles | PMID: 32710484 | PMCID: PMC8114775