Abstract
Background
Individuals with bacteriologically confirmed pulmonary tuberculosis (TB) disease who do not report symptoms (subclinical TB) represent around half of all prevalent cases of TB, yet their contribution to Mycobacterium tuberculosis (Mtb) transmission is unknown, especially compared to individuals who report symptoms at the time of diagnosis (clinical TB). Relative infectiousness can be approximated by cumulative infections in household contacts, but such data are rare.Methods
We reviewed the literature to identify studies where surveys of Mtb infection were linked to population surveys of TB disease. We collated individual-level data on representative populations for analysis and used literature on the relative durations of subclinical and clinical TB to estimate relative infectiousness through a cumulative hazard model, accounting for sputum-smear status. Relative prevalence of subclinical and clinical disease in high-burden settings was used to estimate the contribution of subclinical TB to global Mtb transmission.Results
We collated data on 414 index cases and 789 household contacts from three prevalence surveys (Bangladesh, the Philippines, and Viet Nam) and one case-finding trial in Viet Nam. The odds ratio for infection in a household with a clinical versus subclinical index case (irrespective of sputum smear status) was 1.2 (0.6-2.3, 95% confidence interval). Adjusting for duration of disease, we found a per-unit-time infectiousness of subclinical TB relative to clinical TB of 1.93 (0.62-6.18, 95% prediction interval [PrI]). Fourteen countries across Asia and Africa provided data on relative prevalence of subclinical and clinical TB, suggesting an estimated 68% (27-92%, 95% PrI) of global transmission is from subclinical TB.Conclusions
Our results suggest that subclinical TB contributes substantially to transmission and needs to be diagnosed and treated for effective progress towards TB elimination.Funding
JCE, KCH, ASR, NS, and RH have received funding from the European Research Council (ERC) under the Horizon 2020 research and innovation programme (ERC Starting Grant No. 757699) KCH is also supported by UK FCDO (Leaving no-one behind: transforming gendered pathways to health for TB). This research has been partially funded by UK aid from the UK government (to KCH); however, the views expressed do not necessarily reflect the UK government's official policies. PJD was supported by a fellowship from the UK Medical Research Council (MR/P022081/1); this UK-funded award is part of the EDCTP2 programme supported by the European Union. RGW is funded by the Wellcome Trust (218261/Z/19/Z), NIH (1R01AI147321-01), EDTCP (RIA208D-2505B), UK MRC (CCF17-7779 via SET Bloomsbury), ESRC (ES/P008011/1), BMGF (OPP1084276, OPP1135288 and INV-001754), and the WHO (2020/985800-0).Free full text

Estimating the contribution of subclinical tuberculosis disease to transmission: An individual patient data analysis from prevalence surveys
Abstract
Background:
Individuals with bacteriologically confirmed pulmonary tuberculosis (TB) disease who do not report symptoms (subclinical TB) represent around half of all prevalent cases of TB, yet their contribution to Mycobacterium tuberculosis (Mtb) transmission is unknown, especially compared to individuals who report symptoms at the time of diagnosis (clinical TB). Relative infectiousness can be approximated by cumulative infections in household contacts, but such data are rare.
Methods:
We reviewed the literature to identify studies where surveys of Mtb infection were linked to population surveys of TB disease. We collated individual-level data on representative populations for analysis and used literature on the relative durations of subclinical and clinical TB to estimate relative infectiousness through a cumulative hazard model, accounting for sputum-smear status. Relative prevalence of subclinical and clinical disease in high-burden settings was used to estimate the contribution of subclinical TB to global Mtb transmission.
Results:
We collated data on 414 index cases and 789 household contacts from three prevalence surveys (Bangladesh, the Philippines, and Viet Nam) and one case-finding trial in Viet Nam. The odds ratio for infection in a household with a clinical versus subclinical index case (irrespective of sputum smear status) was 1.2 (0.6–2.3, 95% confidence interval). Adjusting for duration of disease, we found a per-unit-time infectiousness of subclinical TB relative to clinical TB of 1.93 (0.62–6.18, 95% prediction interval [PrI]). Fourteen countries across Asia and Africa provided data on relative prevalence of subclinical and clinical TB, suggesting an estimated 68% (27–92%, 95% PrI) of global transmission is from subclinical TB.
Conclusions:
Our results suggest that subclinical TB contributes substantially to transmission and needs to be diagnosed and treated for effective progress towards TB elimination.
Funding:
JCE, KCH, ASR, NS, and RH have received funding from the European Research Council (ERC) under the Horizon 2020 research and innovation programme (ERC Starting Grant No. 757699) KCH is also supported by UK FCDO (Leaving no-one behind: transforming gendered pathways to health for TB). This research has been partially funded by UK aid from the UK government (to KCH); however, the views expressed do not necessarily reflect the UK government’s official policies. PJD was supported by a fellowship from the UK Medical Research Council (MR/P022081/1); this UK-funded award is part of the EDCTP2 programme supported by the European Union. RGW is funded by the Wellcome Trust (218261/Z/19/Z), NIH (1R01AI147321-01), EDTCP (RIA208D-2505B), UK MRC (CCF17-7779 via SET Bloomsbury), ESRC (ES/P008011/1), BMGF (OPP1084276, OPP1135288 and INV-001754), and the WHO (2020/985800-0).
Introduction
An estimated 1.5 million people died from tuberculosis (TB) disease in 2020, and TB is on course to retake its position as the largest cause of death by a single infectious agent (World Health Organisation, 2021). Fuelled by ongoing transmission through exhaled or expectorated Mycobacterium tuberculosis (Mtb) bacteria, TB incidence is declining at a rate of 1–2% per annum, which is too slow given the risk and scale of mortality (World Health Organisation, 2021; Ragonnet et al., 2021), lifelong impairment (Dodd et al., 2021; Alene et al., 2021), poverty (Pedrazzoli et al., 2021), and macroeconomic consequences (Silva et al., 2021). Problematically, most Mtb transmission in high-incidence settings remains unaccounted for (Dodd et al., 2021), with less than 1-in-10 occurrences of TB explained by transmission from a known contact (Glynn et al., 2015).
In recent decades the prevailing paradigm in TB policy held that symptoms and infectiousness commence simultaneously as part of ‘active disease’ (World Health Organization, 2020; World Health Organisation, 1974; Houben et al., 2019). As a consequence, a policy of passive case-finding (Golub et al., 2005), in which individuals are expected to attend a health facility with TB-related symptoms before receiving diagnosis and treatment, was relied upon to prevent deaths from TB, which it has (World Health Organisation, 2021; Mandal et al., 2017), and reduce incidence by interrupting transmission, which it has not (World Health Organisation, 2021).
Over the last decade, this classic paradigm of TB has been increasingly challenged (Barry et al., 2009; Drain et al., 2018; Behr et al., 2018). One important advance has been the finding in population surveys that not all individuals identified with bacteriologically confirmed TB report having symptoms such as cough at the time of screening for TB (Onozaki et al., 2015; Law et al., 2020). As such we can make a distinction between clinical and subclinical TB, where subclinical TB (sometimes referred to as ‘asymptomatic’ [Frascella et al., 2021] or ‘early’ TB [Kendall et al., 2021]) refers to individuals who have detectable Mtb bacteria in their sputum but do not experience, are not aware of, or do not report symptoms (Houben et al., 2019). In contrast, individuals with clinical TB disease report symptoms. We distinguish both disease states from Mtb infection, whereby individuals may test positive on a tuberculin skin test (TST) or interferon-gamma release assay (IGRA) but do not have bacteriologically confirmed disease.
Empirical data have shown that bacteriological state (i.e. whether Mtb is detectable in pulmonary secretions) is a strong predictor of the potential for transmission. For example, molecular epidemiological studies show that sputum smear-positive individuals (i.e. Mtb detected via microscopy) are 3–6 times more likely to be sources for TB disease in contacts compared to smear-negative individuals (Behr et al., 1999; Hernández-Garduño et al., 2004; Tostmann et al., 2008). Surveys of Mtb infection prevalence in household contacts provide similar values (Grzybowski et al., 1975). These studies focussed on passively diagnosed individuals with clinical disease. It is, however, increasingly clear that the presence of respiratory symptoms, such as a persistent cough, is not required for the exhalation of potentially Mtb-containing aerosols (Patterson and Wood, 2019; Asadi et al., 2019; Leung et al., 2020; Dinkele et al., 2022). Indeed, whilst recent empirical studies have suggested that tidal breathing may contribute significantly to Mtb transmission (Dinkele et al., 2022), exhalation of infectious aerosols appears unrelated to the presence of symptoms (Theron et al., 2020) or cough frequency (Williams et al., 2020) in TB patients. This supports the hypothesis that subclinical disease can contribute, potentially substantially, to transmission (Houben et al., 2019; Kendall et al., 2021; Dowdy et al., 2013).
A recent review found that about half of prevalent bacteriologically confirmed pulmonary TB disease is subclinical (Frascella et al., 2021) and it is becoming increasingly apparent that subclinical TB can persist for a long period without progressing to clinical disease (Richards et al., 2021; Ku et al., 2021). As individuals with subclinical TB will not be identified by current passive case-finding strategies, they will continue to contact susceptible individuals and, if infectious, transmit throughout their subclinical phase. It is therefore possible that those with subclinical TB may be a major contributor to ongoing, and unaccounted for, Mtb transmission. If this is the case, and if the ambitious goal to end TB as a global health problem by 2035 is to be met (World Health Organisation, 2022), TB policy needs to shift away from solely focussing on symptom-dependent case-finding (e.g. patient-initiated passive case-finding) towards strategies that are symptom-independent.
To motivate and inform such a shift in research and policy priorities, two key questions that to date remain unanswered must be addressed. Firstly, how infectious are individuals with subclinical TB compared to those with clinical TB per unit time, and, secondly, what is their contribution to overall transmission in the current TB epidemic?
In TB, data sources on the transmission potential from sputum smear-negative individuals relative to smear-positive (e.g. molecular epidemiological studies; Behr et al., 1999; Hernández-Garduño et al., 2004; Tostmann et al., 2008) have often been directly interpreted as relative infectiousness, which is incorrect (Kendall, 2021). Instead of representing the metric of interest, which is the potential for transmission per unit time for a particular group relative to a reference group (i.e. relative infectiousness), these data actually provided a relative estimate of cumulative exposure (as acknowledged by these studies’ authors; Behr et al., 1999; Hernández-Garduño et al., 2004; Tostmann et al., 2008). Cumulative exposure is a composite of relative infectiousness per unit time and disease duration (technically duration of infectiousness), which until now have been unavailable and can be hard to disentangle from each other (Kendall, 2021).
In this work we look to overcome these challenges by harnessing increased understanding of the natural history and prevalence of subclinical TB and re-analysing data from existing population studies.
Methods
Data
To estimate the infectiousness of subclinical TB relative to clinical TB, we considered studies in which Mtb infection surveys were performed amongst household contacts of culture and/or nucleic acid amplification test (NAAT) confirmed cases where data on their symptom and sputum smear status at the time of diagnosis was available. We considered only studies in which households with no index case were also surveyed for Mtb infection as a measure of the background rate of infection.
Such studies identified index cases using symptom-independent screening either via a TB prevalence survey (in which all individuals are screened with a chest X-ray; World Health Organisation, 2011) or community-wide active case-finding amongst a representative sample of a target population. Subclinical and clinical index cases were defined as being culture and/or NAAT-positive and responding negatively or positively to an initial symptom screening, respectively. Households with a single subclinical or clinical index case were defined as subclinical and clinical households, respectively. Such households were then stratified by the sputum smear status of the index case at the time of diagnosis. Background households were defined as having no index case. Finally, Mtb infection surveys were performed amongst all households, providing the prevalence of infection amongst each household type.
We reviewed the literature for household contact studies that measure Mtb infection via TST or IGRA as an outcome and provide sufficient information to stratify households by symptom and sputum smear status, including households with no index case (see Appendix 1 for the detailed search strategy). Individual, patient-level data from each of these studies were analysed to provide the prevalence of infection amongst each household type (see Appendix 1 for detailed data analysis). These data are presented in Appendix 1—table 1. Odds ratios (ORs) for infection in members of a household with a sputum smear-positive versus a smear-negative index case (irrespective of symptoms) and in members of a household with a clinical versus subclinical index case (irrespective of sputum smear status) were also calculated for purposes of illustration.
Cumulative hazard model
To estimate the infectiousness of subclinical TB per unit time relative to clinical TB, we fitted a cumulative hazard model of infection to the prevalence of infection amongst each household type for each study separately using the data described above.
For each study, household contacts were pooled into five cohorts: background; subclinical and sputum smear-negative; subclinical and sputum smear-positive; clinical and sputum smear-negative; clinical and sputum smear-positive. It was assumed that each cohort is exposed to the same background hazard, reflecting the force of infection from outside the household. It was then assumed that all cohorts except the background were exposed to an additional hazard, reflecting the force of infection from the cohort’s respective index cases.
The final prevalence of infection in each cohort will then depend on the background cumulative hazard ΛB and an additional cumulative hazard ΛI specific to each household type I (see Appendix 1 for model equations). We use the cumulative hazard from clinical (C), smear-positive (+) index cases as a benchmark with which to define the cumulative hazards from the remaining index case types. We assume that being subclinical (S) or smear-negative (-) have separate, multiplicative effects, such that
where rs and r- are the subclinical and sputum smear-negative relative cumulative hazards, respectively.
Model fitting
The model described above was fitted to the prevalence of infection in each of the five household types for each study separately. Fitting was performed in a Bayesian framework using Markov-Chain Monte Carlo methods (see Appendix 1 for further details of model fitting). We report median and 95% equal-tailed posterior intervals (PoIs).
Relative infectiousness of subclinical TB
To infer the infectiousness of subclinical TB per unit time relative to clinical TB from our posterior estimate for the subclinical relative cumulative hazard rs, we note that, assuming constant hazards, the relative cumulative hazards from index cases will depend on the product of the relative per unit time infectiousness and relative durations of infectiousness. We assume that per unit time infectiousness depends on symptom status and sputum smear status, whilst durations of infectiousness depend on symptom status only. It follows then that:
where αs and α- are the per unit time infectiousness of subclinical relative to clinical index cases and sputum smear-negative relative to smear-positive index cases, respectively, and γs is the duration of infectiousness for subclinical relative to clinical index cases.
To provide a value for the duration of infectiousness of subclinical relative to clinical index cases, we used the results from a recent study that estimated the durations of subclinical and clinical TB using a Bayesian analysis of prevalence and notification data (Ku et al., 2021). With the result that the subclinical phase represented between 27% and 63% of the time as a prevalent case, we used a duration of subclinical TB relative to clinical TB of 0.8 (0.4–1.7, 95% PoI). We assumed that there was no difference in duration for sputum smear-negative versus smear-positive TB.
Finally, we sampled from the posterior estimate for the subclinical relative cumulative hazard and an assumed duration of disease for subclinical index cases relative to clinical index cases, providing a median and 95% equal-tailed posterior estimate for the relative infectiousness of subclinical index cases relative to clinical index cases for each study separately. Thereafter we provide a summary estimate by mixed-effects meta-analysing the individual estimates across the separate studies. Analogous results are presented for the relative infectiousness per unit time of sputum smear-negative TB relative to smear-positive TB.
Subclinical versus clinical TB: Prevalence and bacteriological indicators
To estimate the proportion of overall transmission from subclinical TB, we first estimated the proportion of prevalent TB that is subclinical as well as the proportion of prevalent subclinical and clinical TB that is smear-positive.
We began with a recent review of TB prevalence surveys in Asia and Africa (Frascella et al., 2021) (see Appendix 1 for details of the search strategy). Such surveys generally performed an initial screening using both a questionnaire, which includes questions about recent symptoms typical of TB, as well a chest radiograph. Those screening positive from either method were then tested via culture and/or NAAT. A sputum smear test was often additionally performed.
We reviewed the surveys in Frascella et al., 2021 and, for each survey where sufficient information was available, extracted the number of culture and/or NAAT confirmed cases of TB, stratified by both symptom status at initial screening and sputum smear status (see Appendix 1 for detailed data analysis). Extracted data can be found in Appendix 1—table 2. We defined subclinical and clinical TB as being culture and/or NAAT-positive and responding negatively or positively to an initial symptom screen, respectively, consistent with the definitions for subclinical and clinical index cases in the previous section. The most common screening question was a productive cough of greater than 2-week duration, although other diagnostic algorithms were included.
For each survey, we calculated the proportion of prevalent TB that is subclinical (PSTB) as well as the proportion of prevalent subclinical and clinical TB that is smear-positive (P+S and P+C, respectively).
We performed univariate, random-effects meta-analyses on PSTB, P+S and P+C. We meta-analysed the inverse logit transformed variables, before transforming the results back to proportions and presenting a central estimate and 95% prediction interval for each variable.
The contribution of subclinical TB to transmission
To estimate the contribution of subclinical TB to transmission, we applied our estimates of relative infectiousness to the prevalence surveys that reported the required data by symptom and smear status (see Appendix 1 for further details).
All analyses were conducted using R version 4.0.3 (R Development Core Team, 2014). Bayesian fitting was performed in Stan version 2.21.0 (Stan Development Team, 2021) using RStan (Stan Development Team, 2020) as an interface.
No new human subject data was collected for this work, which re-analysed individual patient data collected during four observational studies in three countries. Procedures, including consent where available, are described in the original publications. Local and institutional Ethics Approval was in place for each survey, through the Department of Health (the Philippines survey; Tupasi et al., 1999), Institutional Review Board of the Viet Nam National Lung Hospital (Viet Nam survey; Hoa et al., 2010), the Ministry of Health and Family Welfare of Bangladesh as well as the Research Review Committee and Ethics Review Committee of the iccdr,b (Bangladesh survey; Zaman et al., 2012), and Institutional Review Board of the Viet Nam National Lung Hospital as well as Human Research Ethics Committee of the University of Sydney (ACT3 survey; Marks et al., 2019). The ethics committee of the London School of Hygiene and Tropical Medicine gave ethical approval for this project (#16396).
Sensitivity analyses
Sensitivity analysis 1
Given the different designs of the Bangladesh (2007) prevalence survey (Zaman et al., 2012) (which provided only sputum smear-positive index cases) and the active case-finding trial in Viet Nam (2017) (Marks et al., 2019) (which provided index cases via repeated case-finding-related screening and prevalence surveys), the above analysis was repeated omitting these studies.
Sensitivity analysis 2
As a sensitivity the above analysis was repeated with an alternative estimate for the relative duration of subclinical TB versus clinical TB using instead data from a recent systematic review and data synthesis study (Richards et al., 2021) and a simple competing risk model (see Appendix 1 for further details).
Sensitivity analysis 3
To explore the impact of a differential background risk of infection amongst households with and without index cases, the analysis was repeated assuming a 50% increase in the background risk of infection for those households with an index case.
Sensitivity analysis 4
Instead of assuming equal durations for sputum smear-positive and smear-negative TB, the above analysis was repeated assuming that sputum smear-positive TB has twice the duration of smear-negative TB.
Sensitivity analysis 5
In the main analysis, each study was modelled separately, with the results combined using meta-analyses. As a sensitivity we model all studies simultaneously, assuming local background risks of infection for each study and global values across all studies for the remaining cumulative hazards.
Results
Data
Four studies were included for analysis: three prevalence surveys of TB disease with associated Mtb infection surveys in Viet Nam (2007) (Hoa et al., 2010), Bangladesh (2007) (Zaman et al., 2012), and the Philippines (1997) (Tupasi et al., 1999) and a community-wide active case-finding trial in Viet Nam (2017) (Marks et al., 2019).
ORs for infection in members of a household with a clinical versus subclinical index case (irrespective of sputum smear status), based on the result of their symptom screen at the time of diagnosis, are shown in Figure 1A. A mixed-effects meta-analysis across studies provides OR = 1.2 (0.6–2.3, 95% confidence interval [CI]). Figure 1B shows the OR for infection in members of a household with a sputum smear-positive versus a smear-negative index case (irrespective of symptoms), where the Bangladesh prevalence survey is omitted as this study only included smear-positive individuals. In contrast to the analysis by symptom status, evidence for a difference in cumulative infection was found by smear status, with OR = 2.3 (1.3–3.9, 95% CI), which is in line with previous estimates (Grzybowski et al., 1975).
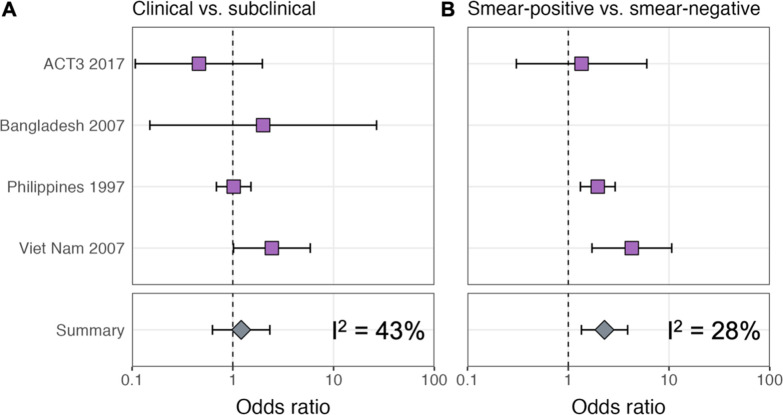
Illustrated are central estimates and 95% confidence intervals for each study separately and the results of a mixed-effects meta-analysis. Results for sputum smear status are omitted for Bangladesh as the survey considered only sputum smear-positive individuals.
Estimating the relative infectiousness of subclinical TB
The estimated infectiousness of subclinical TB per unit time relative to clinical TB is shown in Figure 2A, both for each study separately as well as the mixed-effects meta-analysed result across studies of 1.93 (0.62–6.18, 95% prediction interval [PrI]). Figure 2B shows the analogous results for the infectiousness per unit time of sputum smear-negative versus smear-positive TB, with a summary value of 0.26 (0.03–2.47, 95% PrI). Detailed model results are shown in Appendix 1—table 4 and Appendix 1—figures 2–5.
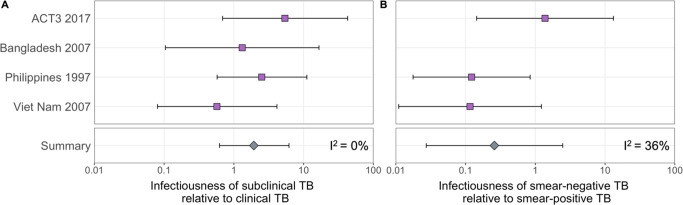
Illustrated are the median and 95% confidence intervals for each study separately and the median and 95% prediction interval results from mixed-effects meta-analyses across studies with an associated measure of heterogeneity (I2).
Prevalence and bacteriological indicators for subclinical and clinical TB
Data from 15 prevalence surveys where the proportion of subclinical and clinical TB was reported by sputum smear status were included, detailed in Appendix 1—table 2. These represented a range of high TB burden countries in Africa (n = 5) and Asia (n = 9, with two surveys in Viet Nam). In this subset, the overall proportion of prevalent TB that is subclinical was 58% (29–82%, 95% PrI), whilst the proportion smear-positive was 33% (18–52%, 95% PrI) for subclinical TB and 53% (25–80%, 95% PrI) for clinical TB. Detailed results for each variable are shown in Figure 3A–C.
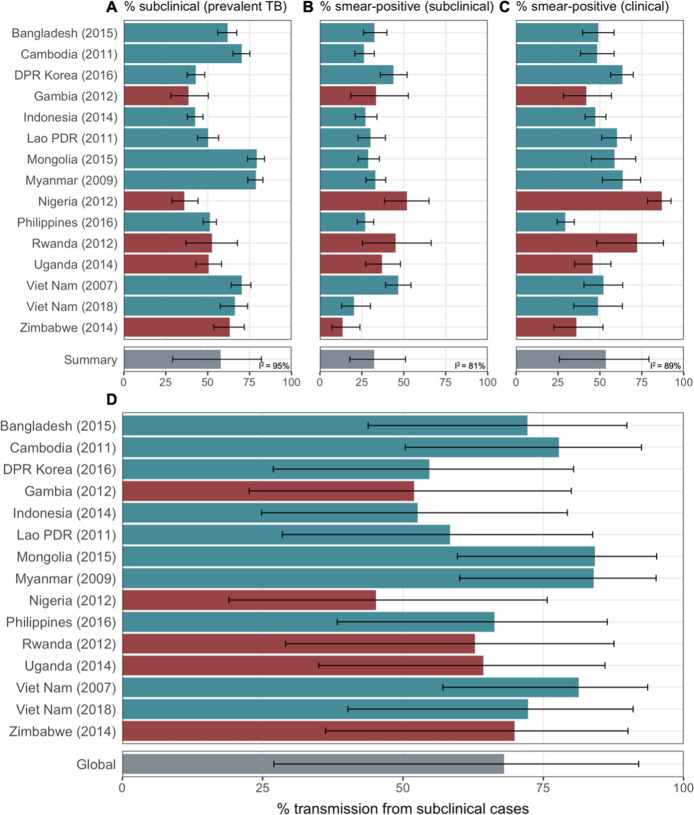
Illustrated are median and 95% confidence intervals for each study separately and the median and 95% prediction intervals from mixed-effects meta-analyses across studies with an associated measure of heterogeneity (I2). Also shown is the estimated proportion of transmission from subclinical TB at the time of and in the location of each of the prevalence surveys in Africa and Asia (D). Illustrated is the median and 95% prediction intervals for each study separately as well as the global value. DPR = Democratic People’s Republic; PDR = People’s Democratic Republic.
The contribution of subclinical TB to transmission: Global and country levels
We quantified the contribution of subclinical TB to ongoing Mtb transmission by combining the estimates for the infectiousness of subclinical TB per unit time relative to clinical TB (Figure 2A), the infectiousness of sputum smear-negative TB relative to smear-positive TB (Figure 2B), and the proportion of prevalent TB that is subclinical and the proportion of subclinical and clinical TB that is sputum smear-positive (Figure 3A–C). The 14 included countries are a reasonable reflection of the geography and epidemiological characteristics of high TB burden countries in the WHO African, South-East Asia, and Western Pacific regions, which together represent around 85% of current global TB incidence (World Health Organisation, 2021). As such we used a summary value for the included surveys as a global estimate.
Figure 3D shows the results by country and globally, where 68% (27–92%, 95% PrI) of global Mtb transmission is estimated to come from prevalent subclinical TB, ranging from 45% (19–76%, 95% PrI) in Nigeria to 84% (60–95%, 95% PrI) in Mongolia.
Sensitivity analyses
Sensitivity analysis 1
The above analysis was repeated excluding two studies with methodologies that differed from the remaining two: the Bangladesh (2007) prevalence survey (Zaman et al., 2012) (which provided sputum smear-positive index cases only) and the active case-finding trial in Viet Nam (ACT3 [2017]) (Marks et al., 2019) (which provided index cases via repeated screening related to case-finding as well as prevalence surveys). Affected results are shown in Appendix 1—figure 6. The infectiousness of subclinical TB per unit time relative to clinical TB decreased to 1.39 (0.17–11.2, 95% PrI), and the infectiousness of sputum smear-negative TB relative to smear-positive TB decreased to 0.12 (0.03–0.53, 95% PrI), with corresponding values of 57% (10–94%, 95% PrI) of global transmission from subclinical TB, ranging from 34% (6–81%, 95% PrI) in Nigeria to 76% (28–97%, 95% PrI) in Mongolia.
Sensitivity analysis 2
The above analysis was repeated using an alternative estimate for the relative duration of subclinical TB versus clinical TB of 0.72 (0.60–0.89, 95% PoI), from Richards et al., 2021. Affected results are shown in Appendix 1—figure 7. The infectiousness of subclinical TB per unit time relative to clinical TB increased to 2.19 (0.91–5.26, 95% PrI), with corresponding values of 71% (32–92%, 95% PrI) of global transmission from subclinical TB, ranging from 48% (25–74%, 95% PrI) in Nigeria to 86% (68–95%, 95% PrI) in Mongolia.
Sensitivity analysis 3
The above analysis was repeated assuming that households with an index case have a 50% greater background risk of infection than households with no index case. Affected results are shown in Appendix 1—figure 8. The infectiousness of subclinical TB per unit time relative to clinical TB increased to 2.44 (0.60–10.06, 95% PrI) whilst the infectiousness of sputum smear-negative TB relative to smear-positive TB increased to 0.36 (0.03–4.51, 95% PrI), with corresponding values of 74% (29–95%, 95% PrI) of global transmission from subclinical TB, ranging from 53% (20–85%, 95% PrI) in Nigeria to 88% (61–97%, 95% PrI) in Mongolia.
Sensitivity analysis 4
The above analysis was repeated assuming sputum smear-positive TB has twice the duration of smear-negative TB. Affected results are shown in Appendix 1—figure 9. The infectiousness of subclinical TB per unit time relative to clinical TB was largely unchanged at 1.94 (0.63–6.16, 95% PrI) whilst the infectiousness of sputum smear-negative TB relative to smear-positive TB increased to 0.51 (0.06–4.39, 95% PrI), with corresponding values of 70% (29–93%, 95% PrI) of global transmission from subclinical TB, ranging from 49% (21–79%, 95% PrI) in Nigeria to 86% (63–96%, 95% PrI) in Mongolia.
Sensitivity analysis 5
The above analysis was repeated with all studies modelled simultaneously, assuming local background risks of infection for each study and global values across all studies for the remaining cumulative hazards. Affected results are shown in Appendix 1—figure 10. The infectiousness of subclinical TB per unit time relative to clinical TB decreased to 1.39 (0.50–4.02, 95% PrI) whilst the infectiousness of sputum smear-negative TB relative to smear-positive TB decreased to 0.11 (0.02–0.68, 95% PrI), with corresponding values of 58% (20–88%, 95% PrI) of global transmission from subclinical TB, ranging from 34% (15–62%, 95% PrI) in Nigeria to 76% (52–91%, 95% PrI) in Mongolia.
Discussion
By fitting a cumulative hazard model of infection to prevalence data amongst household contacts of subclinical and clinical index cases, we were able to provide quantitative estimates for the relative infectiousness per unit time of subclinical TB and its contribution to ongoing Mtb transmission. Despite wide uncertainty intervals, the raw data, as well as the results of our analysis, do not suggest subclinical TB is substantially less infectious than clinical TB. Given the high prevalence of subclinical TB found in surveys (Frascella et al., 2021), it is therefore likely that subclinical TB contributes substantially to ongoing Mtb transmission in high-burden settings.
Our results were relatively robust to the sensitivities that were performed. In two cases, that is, the removal of two studies (sensitivity analysis 1) and the use of a single model to account for all studies (sensitivity analysis 5), our estimates for the relative infectiousness of subclinical TB relative to clinical TB and the proportion of transmission from subclinical TB were lower than in the primary analysis. Our qualitative results and conclusions remain unchanged however. All other sensitivities resulted in higher estimates.
There are no other estimates for the infectiousness of subclinical TB relative to clinical TB in the literature with which to compare our results. Using data from the 2007 Viet Nam prevalence survey, however, Nguyen et al., 2023 find that among children aged 6–10 years, those living with clinical, smear-positive TB, and those living with subclinical, smear-positive TB had similarly increased risks of TST positivity compared with those living without TB. Moreover, a recent small study from Uganda found no evidence of a difference in cumulative infection rates in household contacts of patients who did or did not report symptoms (Baik et al., 2021). Our results are also in keeping with recent results from whole-genome sequencing (Xu et al., 2019) in which 36% of individuals likely transmitted Mtb before symptom onset, assuming a linear SNP mutation rate. We also note that previous hypothetical modelling of subclinical TB used assumed values for relative infectiousness that are in keeping with our estimated range (Dowdy et al., 2013; Arinaminpathy and Dowdy, 2015). More broadly, recent work on SARS-CoV-2 and malaria has similarly shown how ‘asymptomatic’ or ‘subpatent’ infections can be important drivers of transmission (Slater et al., 2019; Emery et al., 2020; Johansson et al., 2021), meaning a role for asymptomatic transmission would not be unique to TB.
Whilst we have presented a novel approach to investigating transmission from individuals with subclinical TB using pre-existing data, limitations in our methodology remain. Identifying relative infectiousness is challenging. Our estimates rely on studies which screened a minimum of 252,000 individuals for bacteriologically confirmed TB disease and 63,000 individuals for Mtb infection. Even at this scale, the small number of studies and diagnosed cases of TB still leads to substantial uncertainty, highlighting the challenge faced by single studies to estimate such values (Marks et al., 2019; Baik et al., 2021). Indeed, the paucity of the data provides an estimate that is consistent with subclinical TB being more infectious than clinical TB. Whilst we consider this to be implausible, we have avoided introducing priors that rule out this possibility. Instead we would emphasise that our results reflect the uncertainty of the data. The lower bound of our estimate precludes subclinical TB being significantly less infectious than clinical TB, while there is no evidence against subclinical TB being as infectious as clinical TB. Despite such uncertainty, this study brings together the best currently available epidemiological data which, combined with appropriate analysis techniques, provides a data-driven estimate for this important question.
Although infection studies in household contacts have provided a novel window into transmission from subclinical individuals, it is not possible to establish a transmission link between presumed index cases and infections amongst household contacts using molecular methods (Kendall, 2021). Such household contact studies are therefore liable to biases and our study necessarily inherits such limitations. For example, whilst our model does use a background rate of infection as a baseline from which to estimate the additional force of infection from presumed index cases within the household, there remains the residual risk that certain household types may systematically contain more or less infections from transmission outside the household than on average.
An important limitation of our cumulative hazard model is the assumption that index cases only ever had the disease type they were diagnosed with during screening (e.g. sputum smear-positive, subclinical). Instead, it is more likely that individuals will fluctuate between being, for example, subclinical and clinical (Richards et al., 2021). The impact such additional dynamics would have on our results remains uncertain since they would depend on the detailed model of tuberculosis natural history assumed. Such a model would require additional data to prevent the need for additional assumptions.
We estimated the contribution of subclinical TB to transmission at the population level, including transmission outside the household, using information on relative infectiousness inferred from household contact studies. A more refined estimate may need to take additional factors into account. For example, it is likely that, whilst inside the household the contact rates for subclinical and clinical individuals are likely to be similar, contact with individuals outside the household may differ (Glynn et al., 2020).
We defined subclinical and clinical TB as being culture and/or NAAT-positive and responding negatively or positively to an initial symptom screen, respectively. In practice subclinical and clinical TB are part of a continuous spectrum and alternative definitions could be defined according to different criteria. Here we have used the definition most closely aligned with the methodology of the majority of prevalence surveys, which is consistent with other studies of subclinical TB (Frascella et al., 2021) and pragmatic for inclusion of future surveys.
Meta-analyses were used to provide ranges for several quantities of interest. Whilst the heterogeneity for the relative infectiousness of subclinical and smear-negative TB were low (I2 = 0% and I2 = 36%, respectively), the heterogeneity for the proportion of prevalent TB that is subclinical and the proportions of subclinical and clinical TB that are smear-positive were high (I2 = 95%, I2 = 81% and I2 = 89%, respectively). As such, we have used the more conservative prediction interval (as opposed to credible interval) to reflect this heterogeneity in the final results (Riley et al., 2011; Deeks et al., 2021).
Finally, our data are from populations with a low prevalence of HIV co-infection and the HIV status of individuals with TB was mostly unavailable, making a sub-analysis by HIV and antiretroviral (ART) status impossible. Whilst the subclinical TB presentation is likely affected by HIV in terms of duration and prevalence, it is unknown whether or by how much the relative differences in duration and prevalence between subclinical and clinical TB also change (Frascella et al., 2021; National Department of Health - South Africa, 2021). If they exist, any such differences by HIV-coinfection status are likely to be reduced by effective viral suppression, which an estimated two-thirds of people living with HIV have achieved (UNAIDS, 2021). So while it remains highly valuable to accumulate additional relevant data (Baik et al., 2021), we feel our main findings are broadly robust to this limitation.
Our observation that reported symptoms are a poor proxy for infectiousness fits with historical and contemporary observations that symptom-independent TB screening and treatment policies can reduce TB burden at higher rates than usually seen under DOTS (Marks et al., 2019; Krivinka et al., 1974). This is in keeping with increasing data showing that symptoms, in particular the classic TB symptom of cough, are not closely correlated to the amount of Mtb exhaled (Williams et al., 2020) and observations of other pathogens, including SARS-CoV-2 infection (Emery et al., 2020; Johansson et al., 2021; Liu et al., 2020).
Whilst earlier diagnosis (i.e. before symptom onset) will likely bring individual-level benefits in terms of mortality and extent of post-TB sequelae (Allwood et al., 2019), the question of potential population benefits has hampered decisions from policymakers and funders on whether to invest resources in technologies and strategies that can identify subclinical TB. Our results suggest that a non-trivial proportion of all transmission would likely be unaffected by strategies that are insensitive to subclinical TB.
As our results show that subclinical TB likely contributes substantially to transmission, an increased emphasis on symptom agnostic screening in, for example, the TB screening guidelines (World Health Organization, 2021) should be considered, as should the inclusion of subclinical TB in the planned update of WHO case definitions. Target Product Profiles for diagnostic tools should consider all infectious TB, regardless of whether individuals are experiencing or aware of symptoms, and interventions using such tools should be critically evaluated for their impact on Mtb transmission and cost-effectiveness. While symptom-independent tools exist for screening (Williams et al., 2020; Marks et al., 2019; Yoon et al., 2017; Madhani et al., 2020; Scriba et al., 2021; Williams et al., 2014), specificity, costs, and logistics remain an obstacle. In addition, individuals are usually required to produce sputum as part of a confirmatory test, which around half of eligible adults in the general population are unable to do (Marks et al., 2019). Screening or diagnostic technologies that are symptom- as well as sputum-independent, while remaining low-tech and low-cost, remain the goal. Indeed, the advent of bio-aerosol measurements in TB may uncover additional infectious individuals whose sputum-based bacteriological test is negative, although these tools require validation in larger populations (Williams et al., 2020; Williams et al., 2014; Nathavitharana et al., 2022). Any bio-aerosol-positive, sputum-negative individuals are more likely to be subclinical and as such would mean we underestimated the contribution of subclinical TB to global Mtb transmission, even if their relative infectiousness may be lower than sputum-positive TB. As new diagnostic approaches are developed to capture the spectrum of TB disease, policymakers will need to decide on how to treat subclinical TB. In treatment, as in diagnosis, it is key that more tailored approaches are developed and tested so as to prevent over- or undertreatment of individuals with subclinical TB (Esmail et al., 2022).
Conclusion
Subclinical TB likely contributes substantially to transmission in high-burden settings. If we are to meet EndTB targets for TB elimination, the TB community needs to develop technologies and strategies beyond passive case finding to address subclinical TB.
Acknowledgements
The authors acknowledge the work of Dr Thelma Tupasi who led the Philippines (2007) prevalence survey and founded the Tropical Disease Foundation (Philippines), who sadly passed away in 2019.
Appendix 1
Supplementary methods
Search strategy
We sought studies in which Mtb infection surveys were performed amongst household contacts of bacteriologically confirmed index cases, where data on their symptom and sputum smear status at the time of diagnosis was available.
We began with a recent systematic review of population-based TB prevalence surveys completed since 1990, with reports or articles publicly available through August 2019 (Frascella et al., 2021). Surveys were included if both a symptom screening interview and X-ray were performed on all eligible participants and if surveys reported the proportion of bacteriologically confirmed cases by screening modality as well as the proportion of bacteriologically confirmed cases that were negative on symptom screening (see Frascella et al., 2021 for full details of the review process). We then reviewed the reports of the 28 national and subnational TB prevalence surveys included for quantitative analysis in Frascella et al., 2021 and identified three such studies that were conducted alongside Mtb infection surveys amongst household contacts: Viet Nam (2007) (Hoa et al., 2010), Bangladesh (2007) (Zaman et al., 2012), and the Philippines (1997) (Tupasi et al., 1999). Authors of these studies and affiliated institutions were then invited to collaborate using original, individual-level data and all accepted.
In addition to prevalence surveys, we also considered active case-finding studies with associated household infection surveys with which to measure any resultant impact on transmission. A non-systematic review of the literature identified one such study in Viet Nam: ACT3 (2017) (Marks et al., 2019). Again the authors of this study and affiliated institutions were invited to collaborate with original, individual-level data and accepted.
Data analysis
Index cases were identified in the three prevalence surveys (Viet Nam [2007] [Hoa et al., 2010], Bangladesh [2007] [Zaman et al., 2012], and the Philippines [1997] [Tupasi et al., 1999]) via culture and/or NAAT and defined as subclinical or clinical depending on whether they responded negatively or positively to an initial symptom screening, respectively. Index cases were further stratified by their sputum smear status at the time of diagnosis.
Households with multiple co-prevalent index cases were excluded from the analysis to retain the premise of the analysis which links infections above the community level to the characteristics of the single index case. Co-prevalent cases were absent in one study (ACT3; Marks et al., 2019) and below 10% in other studies, limiting the impact on power or introduction of bias.
Linked records were then used to stratify participants of the associated Mtb infection survey into different household types depending on the status of the index case: background (no index case); subclinical and sputum smear-negative; subclinical and sputum smear-positive; clinical and sputum smear-negative; clinical and sputum smear-positive. For each household type the total number of contacts and number of TST or IGRA-positive contacts was extracted, shown in Appendix 1—table 1.
Index cases were identified in ACT3 (Marks et al., 2019) either through routine passive case-finding, in any of the TB screening rounds as part of active case-finding, or in the TB prevalence surveys used to measure the impact of such screening. Those identified through passive case-finding were designated clinical, whilst those identified either through screening or the prevalence surveys were stratified as subclinical or clinical depending on whether they responded negatively or positively to an initial symptom screening, respectively. Index cases were further stratified by their sputum smear status at the time of diagnosis.
The same approach to that described above was then used to find the total number of household contacts and the number of TST or IGRA-positive contacts for each household type, also shown in Appendix 1—table 1.
Appendix 1—table 1.
A negative/positive response to ‘symptoms’ defines subclinical/clinical tuberculosis (TB) in the corresponding study. Infected = number of tuberculin skin test (TST) or interferon-gamma release assay (IGRA)-positive household contacts; Contacts = number of household contacts with a TST or IGRA result; NA = not applicable
Study | Background | Subclinical | Clinical | Symptoms | |||||||
---|---|---|---|---|---|---|---|---|---|---|---|
Smear-negative | Smear-positive | Smear-negative | Smear-positive | ||||||||
Infected | Contacts | Infected | Contacts | Infected | Contacts | Infected | Contacts | Infected | Contacts | ||
ACT3 2017 (Marks et al., 2019) | 128 | 2893 | 2 | 8 | 2 | 10 | 1 | 16 | 4 | 27 | Cough >2 wk |
Bangladesh 2007 (Zaman et al., 2012) | 702 | 17,566 | NA | NA | 1 | 5 | NA | NA | 3 | 9 | Any cough |
Philippines 1997 (Tupasi et al., 1999) | 3823 | 20,259 | 48 | 227 | 32 | 82 | 23 | 108 | 34 | 109 | Cough >2 wk |
Vietnam 2007 (Hoa et al., 2010) | 1556 | 21,298 | 3 | 59 | 5 | 28 | 4 | 42 | 16 | 59 | Cough >2 wk |
Cumulative hazard model
Model equations
The prevalence of infection in background households (i.e. with no index case) is given by
where ΛB is the cumulative hazard from the background, representing transmission outside the household. The prevalence of infection in households with an index case is given by
where ΛI is the cumulative hazard from index case type I = subclinical and smear-negative (S-); subclinical and smear-positive (S+); clinical and smear-negative (C-); clinical and smear-positive (C).
The cumulative hazard from clinical and smear-positive index cases is used as a benchmark to define the cumulative hazards from the remaining index case types. We assume that being subclinical or smear-negative have separate, multiplicative effects, such that
where rs and r- are the subclinical and sputum smear-negative relative cumulative hazards, respectively.
Model fitting
The model was fitted to the prevalence of infection in each of the five household types for each study separately. Fitting was performed in a Bayesian framework using Markov-Chain Monte Carlo methods. We use binomial distributions for the prevalence in the likelihood and estimate the following parameters: the background cumulative hazard (ΛB); the cumulative hazard from clinical, smear-positive index cases (ΛC+), the subclinical relative cumulative hazard (rs), and the sputum smear-negative relative cumulative hazard (r-). We use truncated gamma and normal distributions as weak priors:
A total of 50,000 iterations were performed for each study, the first 25,000 of which were discarded as burn-in. Model fit, trace, correlation, and autocorrelation plots were used to ensure model suitability and convergence. We report median and 95% equal-tailed posterior intervals (PoIs).
Subclinical versus clinical TB: Prevalence and bacteriological indicators
Search strategy
We sought TB prevalence surveys where data on the symptom and sputum smear status at the time of diagnosis was available for those identified in the survey. We began again with the recent systematic review of population-based TB prevalence surveys (Frascella et al., 2021), all of which included information on the symptom status at the time of diagnosis of those identified in the survey. We again reviewed the reports of the 28 national and subnational TB prevalence surveys included for quantitative analysis in Frascella et al., 2021 and identified 14 such studies that also included information on the sputum smear status at the time of diagnosis of those identified in the survey. Data from the second TB prevalence survey in Viet Nam in 2018 (Nguyen et al., 2020), which was not included in Frascella et al., 2021, were additionally included.
Data analysis
From the respective survey reports, we extracted the symptom threshold used for initial symptom screening, the total number of individuals screened and the number of identified cases that were subclinical and sputum smear-negative; subclinical and sputum smear-positive; clinical and sputum smear-negative; clinical and sputum smear-positive. Results of the data extraction are shown in Appendix 1—table 2.
Appendix 1—table 2.
The ‘symptom threshold’ used for initial symptom screening is the metric used here to define subclinical (negative) and clinical (positive). Neg = negative, Pos = positive.
Survey setting (ref) | Year | Subclinicalsmear neg. | Subclinicalsmear pos. | Clinical smear neg. | Clinicalsmear pos. | Number screened | Symptom threshold |
---|---|---|---|---|---|---|---|
Viet Nam (Nguyen et al., 2020) | 2018 | 67 | 17 | 22 | 21 | 61,763 | Cough >2 wk |
Viet Nam (Ministry of Health - Vietnam, 2008) | 2007 | 87 | 76 | 33 | 36 | 94,179 | Productive cough >2 wk |
Myanmar (Ministry of Health - Myanmar, 2010) | 2009 | 164 | 81 | 24 | 42 | 51,367 | Any symptom |
Lao PDR (Law et al., 2015) | 2011 | 83 | 36 | 47 | 71 | 39,212 | Cough >2 wk and/or other |
Cambodia (Ministry of Health - Cambodia, 2011) | 2011 | 163 | 58 | 48 | 45 | 37,417 | Cough >2 wk and/or other |
Gambia (Ministry of Health and Social Welfare - The Gambia, 2011) | 2012 | 18 | 9 | 25 | 18 | 43,100 | Cough >2 wk and/or other |
Rwanda (Ministry of Health - Rwanda, 2014) | 2012 | 11 | 9 | 5 | 13 | 43,128 | Any symptom |
Nigeria (Federal Republic of Nigeria, 2012) | 2012 | 25 | 27 | 12 | 80 | 44,186 | Cough >2 wk |
Indonesia (Ministry of Health, Republic of Indonesia, 2015) | 2014 | 132 | 49 | 129 | 116 | 67,944 | Cough >2 wk and/or other |
Uganda (The Republic of Uganda, 2015) | 2014 | 51 | 30 | 43 | 36 | 41,154 | Cough >2 wk |
Zimbabwe (Ministry of Health and Child Care – Zimbabwe, 2014) | 2014 | 58 | 9 | 25 | 14 | 33,736 | Any symptom |
Bangladesh (DGHS Ministry of Health and Family Welfare - Bangladesh, 2015) | 2015 | 116 | 56 | 54 | 52 | 98,710 | Cough >2 wk and/or other |
Mongolia (Ministry of Health - Mongolia, 2016) | 2015 | 139 | 56 | 21 | 30 | 50,309 | Cough >2 wk |
DPR Korea (Democratic People’s Republic of Korea, 2016) | 2016 | 82 | 64 | 71 | 123 | 60,683 | Cough >2 wk and/or other |
Philippines (Department of Health - Philippines, 2016) | 2016 | 231 | 85 | 212 | 88 | 46,689 | Cough >2 wk and/or other |
The contribution of subclinical TB to transmission
We combined our estimates for the relative infectiousness of subclinical TB per unit time relative to clinical TB (αS), the relative infectiousness of sputum smear-negative TB relative to smear-positive TB (α-), the meta-analysed proportion of prevalent TB that is subclinical (PSTB), and the proportion of prevalent subclinical and clinical TB that is smear-positive (P+S and P+C, respectively) to estimate the per unit time contribution of subclinical TB to overall transmission (
To this end, we used the posterior distributions for αS and α- from the earlier model fitting. We also modelled P+S, P+C and PSTB as normal distributions with means and variances taken from the univariate meta-analysis described above. The expression for the contribution of subclinical TB to overall transmission was then evaluated using 107 samples where we report the median and equal-tailed 95% prediction intervals.
The above was then re-performed on a survey-by-survey basis. Here, P+S, P+C and PSTB were modelled separately for each survey and assumed to be distributed binomially. The distributions used for αS and α- remained unchanged.
Sensitivity analyses
Sensitivity analysis 2
We calculate the duration of infectiousness for subclinical relative to clinical index cases using the model and transition values from Richards et al., 2021. The model is shown in Appendix 1—figure 1A with associated transition values, which are also detailed in Appendix 1—table 3. Disease durations are given by the inverse sum of all transitions out of subclinical (regression or progression) or clinical disease (regression, diagnosis, and treatment or death). We find durations of 5.4 mo (4.6–6.7 mo, 95% PoI) and 7.5 mo (7.0–8.2 mo, 95% PoI) for subclinical and clinical TB, respectively (Appendix 1—figure 1B), giving a relative duration of subclinical versus clinical TB of 0.72 (0.60–0.89, 95% PoI).
Appendix 1—figure 1.

Appendix 1—table 3.
See Richards et al., 2021 for data sources and methods for estimating the above parameters.
Parameter | Value (95% posterior interval) | Units |
---|---|---|
Regression from subclinical | 1.54 (1.23–1.90) | Per year |
Progression from subclinical | 0.67 (0.54–0.86) | Per year |
Regression from clinical | 0.57 (0.47–0.69) | Per year |
Treatment from clinical | 0.70 | Per year |
Death from clinical | 0.32 (0.27–0.37) | Per year |
Supplementary results
Estimating the relative infectiousness of subclinical TB
Detailed model results
Appendix 1—table 4.
Shown are the effective sample size (n_eff); the ‘R hat’ statistic (Rhat); sample mean (mean); Monte Carlo standard error (mcse); sample standard deviation (sd); and sample quantiles (2.5%, 50%, 97.5%).
n_eff | Rhat | Mean | mcse | sd | 2.5% | 50% | 97.5% | |
---|---|---|---|---|---|---|---|---|
Viet Nam | ||||||||
lambda_B | 16,683 | 1 | 0.076 | 0.000 | 0.002 | 0.072 | 0.076 | 0.080 |
lambda_Cp | 14,000 | 1 | 0.222 | 0.001 | 0.078 | 0.089 | 0.216 | 0.393 |
r_s | 11,465 | 1 | 0.653 | 0.006 | 0.610 | 0.050 | 0.524 | 1.988 |
r_n | 15,677 | 1 | 0.195 | 0.002 | 0.197 | 0.006 | 0.140 | 0.682 |
Philippines | ||||||||
lambda_B | 12,836 | 1 | 0.209 | 0.000 | 0.003 | 0.203 | 0.209 | 0.216 |
lambda_Cp | 8107 | 1 | 0.145 | 0.001 | 0.065 | 0.028 | 0.142 | 0.281 |
r_s | 4532 | 1 | 2.644 | 0.044 | 2.940 | 0.683 | 1.910 | 9.700 |
r_n | 15,022 | 1 | 0.172 | 0.001 | 0.131 | 0.010 | 0.145 | 0.484 |
ACT3 | ||||||||
lambda_B | 13,924 | 1 | 0.046 | 0.000 | 0.004 | 0.038 | 0.046 | 0.054 |
lambda_Cp | 10,476 | 1 | 0.058 | 0.001 | 0.055 | 0.002 | 0.042 | 0.203 |
r_s | 9133 | 1 | 6.843 | 0.074 | 7.052 | 0.612 | 4.406 | 27.192 |
r_n | 7628 | 1 | 2.696 | 0.049 | 4.273 | 0.143 | 1.337 | 15.314 |
Bangladesh | ||||||||
lambda_B | 10,804 | 1 | 0.041 | 0.000 | 0.002 | 0.038 | 0.041 | 0.044 |
lambda_Cp | 11,415 | 1 | 0.349 | 0.002 | 0.240 | 0.037 | 0.297 | 0.944 |
r_s | 7640 | 1 | 2.101 | 0.036 | 3.174 | 0.076 | 1.113 | 10.723 |
Appendix 1—figure 2.
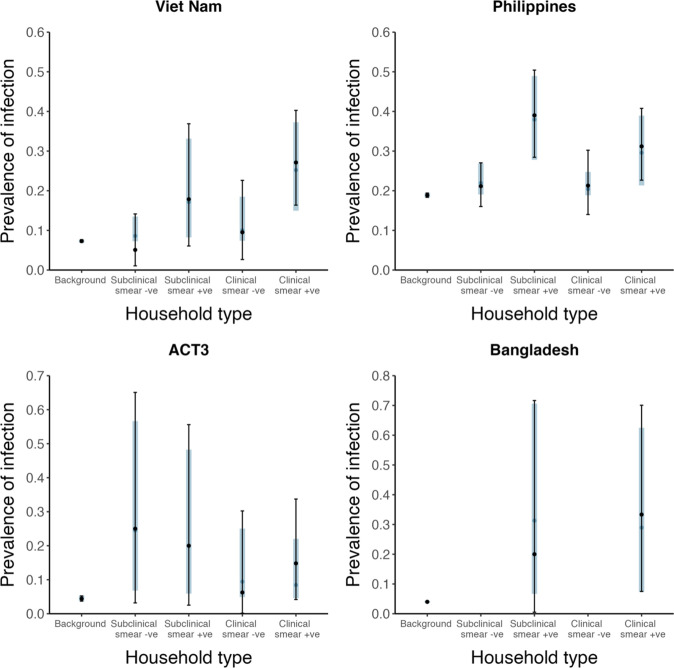
Shown are prevalence of infection in members of households with different index case types (background, subclinical and smear-negative, subclinical and smear-positive, clinical and smear-negative, clinical and smear-positive). Error bars show median and 95% credible intervals. Shaded regions show posterior median and 95% posterior intervals. +ve = positive, -ve = negative.
Appendix 1—figure 3.
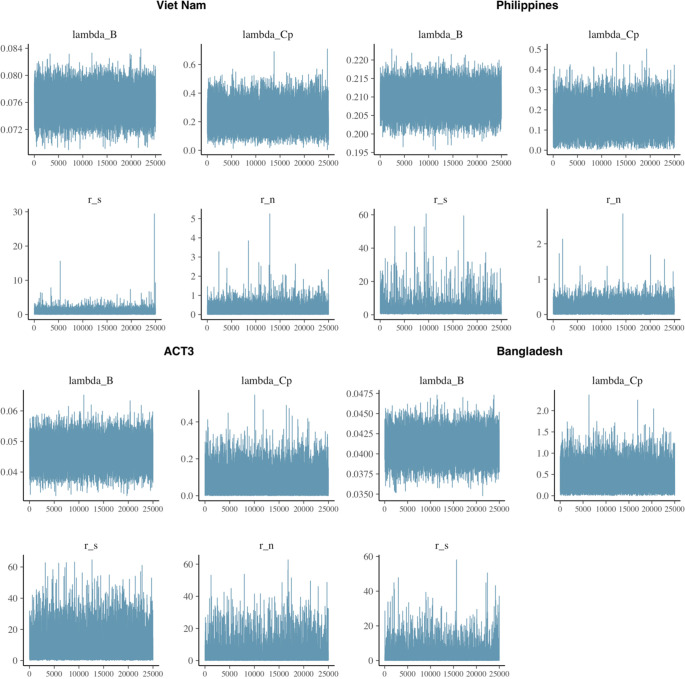
Appendix 1—figure 4.
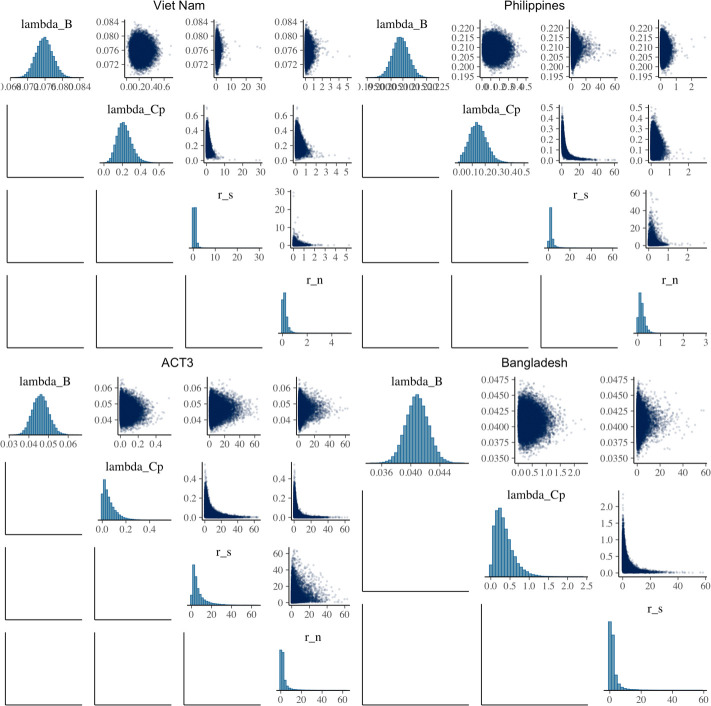
Appendix 1—figure 5.
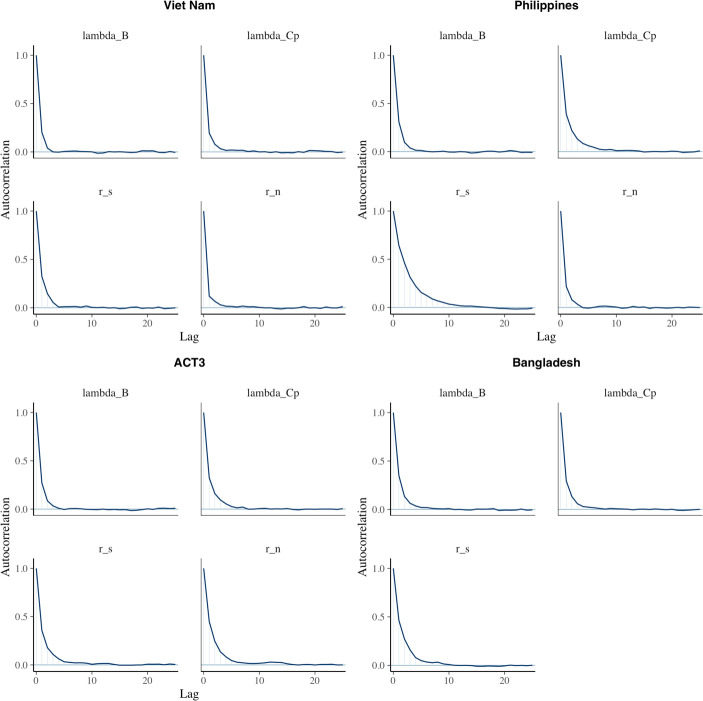
Sensitivity analyses
Sensitivity analysis 1
Omitting Bangladesh (2007) (Zaman et al., 2012) and ACT3 (2017) (Marks et al., 2019) from the analysis.
Appendix 1—figure 6.
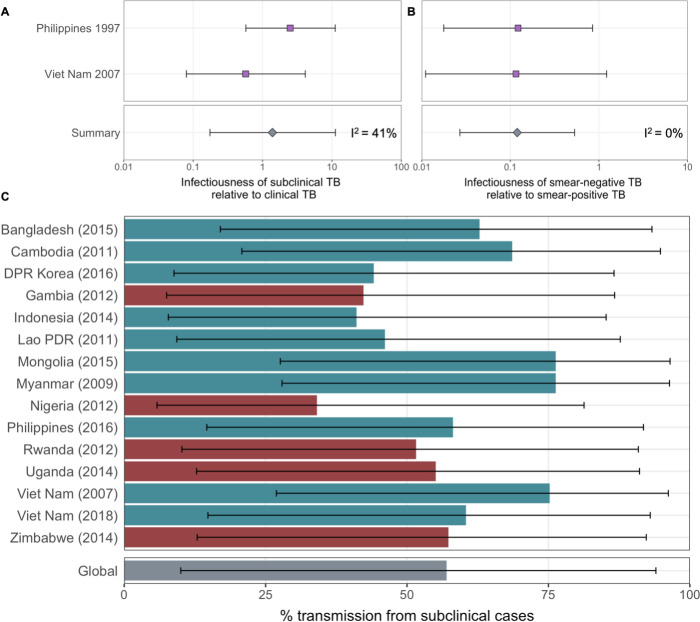
Figure details for A, B and C are as per Figures 2A, B, ,3D3D in the main text, respectively.
Sensitivity analysis 2
Using an alternative duration of subclinical TB relative to clinical TB from Richards et al., 2021.
Appendix 1—figure 7.
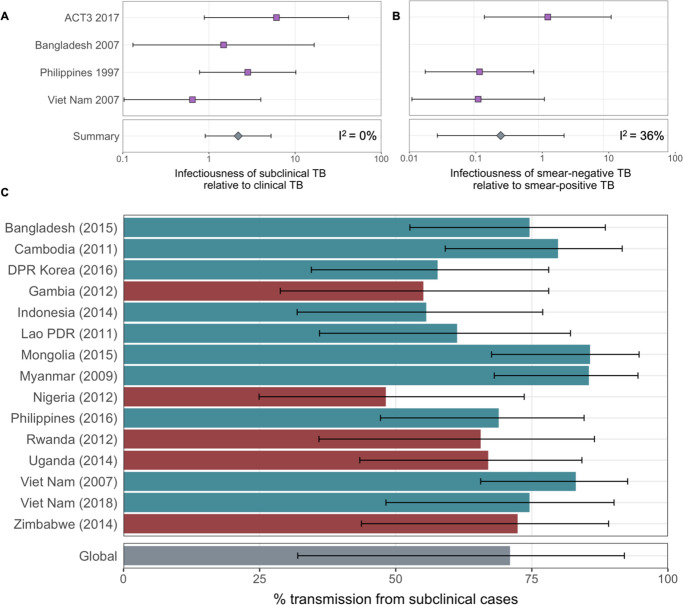
Figure details for A, B and C are as per Figures 2A, B, ,3D3D in the main text, respectively.
Sensitivity analysis 3
Assuming infected households have a 50% greater background risk of infection than non-infected households.
Appendix 1—figure 8.
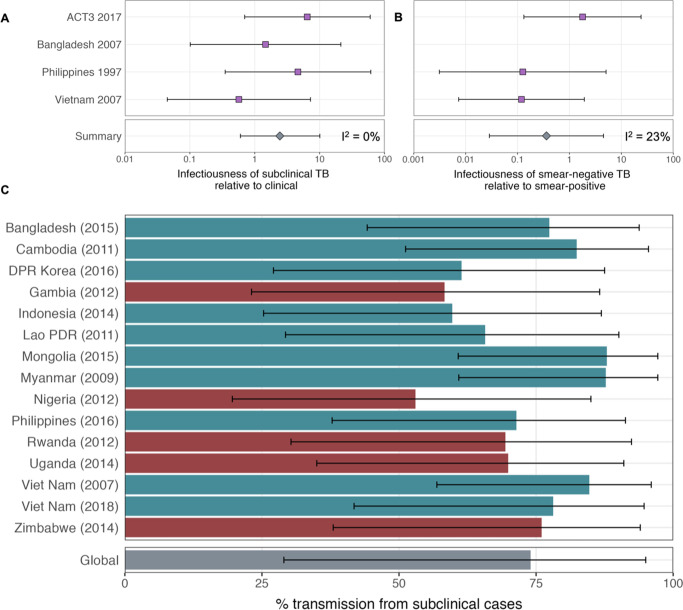
Figure details for A, B and C are as per Figures 2A, B, ,3D3D in the main text, respectively.
Sensitivity analysis 4
Assuming sputum smear-positive TB has twice the duration of smear-negative TB.
Appendix 1—figure 9.
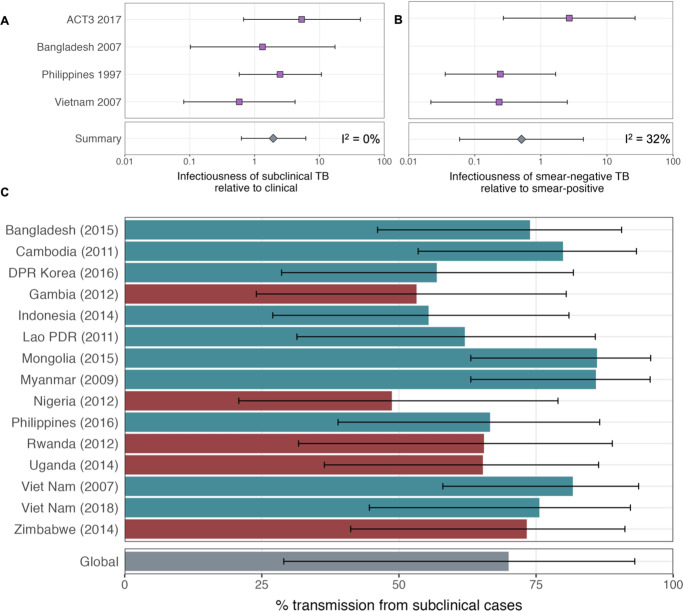
Figure details for A, B and C are as per Figures 2A, B, ,3D3D in the main text, respectively.
Sensitivity analysis 5
Modelling all studies simultaneously, assuming local background risks of infection for each study and global values across all studies for the remaining cumulative hazards.
Appendix 1—figure 10.
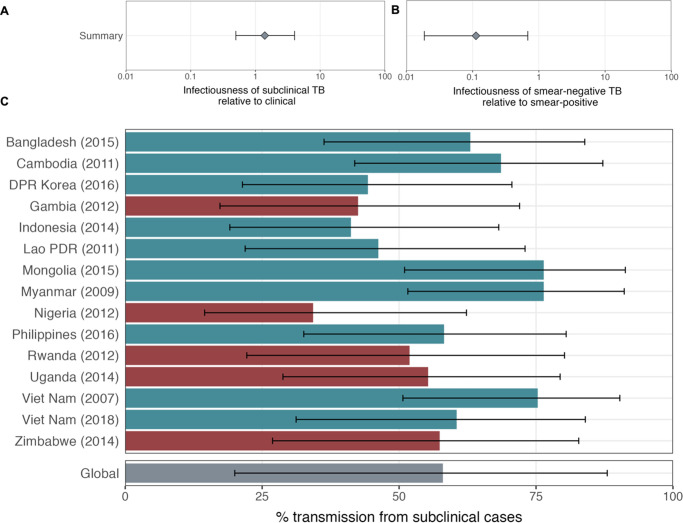
Figure details for A, B and C are as per Figures 2A, B, ,3D3D in the main text, respectively.
Funding Statement
The funders had no role in study design, data collection and interpretation, or the decision to submit the work for publication. For the purpose of Open Access, the authors have applied a CC BY public copyright license to any Author Accepted Manuscript version arising from this submission.
Contributor Information
Bavesh D Kana, University of the Witwatersrand South Africa,
Bavesh D Kana, University of the Witwatersrand South Africa,
Funding Information
This paper was supported by the following grants:
European Research Council ERC Starting Grant No. 757699 to Jon C Emery, Katherine C Horton, Alexandra S Richards, Nabila Shaikh, Richard G White.
Foreign, Commonwealth and Development Office to Katherine C Horton.
UK Government to Katherine C Horton.
UK Medical Research Council MR/P022081/1 to Peter J Dodd.
Wellcome Trust 218261/Z/19/Z to Richard G White.
National Institutes of Health 1R01AI147321-01 to Richard G White.
European and Developing Countries Clinical Trials Partnership RIA208D-2505B to Richard G White.
UK Medical Research Council CCF17-7779 to Richard G White.
Economic and Social Research Council ES/P008011/1 to Richard G White.
Bill & Melinda Gates Foundation OPP1084276 to Richard G White.
Bill & Melinda Gates Foundation OPP1135288 to Richard G White.
Bill & Melinda Gates Foundation INV-001754 to Richard G White.
World Health Organization 2020/985800-0 to Richard G White.
Additional information
Competing interests
No competing interests declared.
has received consultancy fees from WHO (TB burden estimation) and participates as chair of SAB for NIHR grant on TBI screening. The author has no other competing interests to declare.
acts as President of the International Union Against TB & Lung Disease. The author has no other competing interests to declare.
has received grants from National TB Program Cambodia, WHO and DFAT (Australia) and has received consulting fees from WHO Myanmar Office. The author is on the Board of the Directors, UNION IUATLD and WHO’s Global Task Force on TB Impact Measurement. The author has no other competing interests to declare.
received a grant from the Bill and Melinda Gates Foundation and owns stock/stock options in Sanofi Aventis Pharma LTD. The author has no other competing interests to declare.
Author contributions
Software, Formal analysis, Investigation, Visualization, Methodology, Writing – original draft, Writing – review and editing.
Software, Formal analysis, Methodology, Writing – review and editing.
Writing – review and editing.
Formal analysis, Writing – review and editing.
Formal analysis, Writing – review and editing.
Writing – review and editing.
Writing – review and editing.
Writing – review and editing.
Writing – review and editing.
Writing – review and editing.
Writing – review and editing.
Formal analysis, Writing – review and editing.
Writing – review and editing.
Writing – review and editing.
Writing – review and editing.
Visualization, Writing – review and editing.
Writing – review and editing.
Writing – review and editing.
Writing – review and editing.
Methodology, Writing – review and editing.
Conceptualization, Formal analysis, Methodology, Writing – review and editing.
Ethics
No new human subject data was collected for this work, which re-analysed individual patient data collected during four observational studies in three countries. Procedures, including consent where available, are described in the original publications. Local and institutional Ethics Approval was in place for each survey, through the Department of Health (Philippines survey [Tupasi et al., 1999]), Institutional Review Board of the Viet Nam National Lung Hospital (Viet Nam survey [Hoa et al., 2010]), the Ministry of Health and Family Welfare of Bangladesh as well as the Research Review Committee and Ethics Review Committee of the iccdr,b (Bangladesh survey [Zaman et al., 2012]) and Institutional Review Board of the Viet Nam National Lung Hospital as well as Human Research Ethics Committee of the University of Sydney (ACT3 study [Marks et al., 2019]). The Ethics committee of the London School of Hygiene and Tropical Medicine gave ethical approval for this project (#16396).
Data availability
Replication data and analysis scripts are available at GitHub (copy archived at Emery JC, 2022).
References
- Alene KA, Wangdi K, Colquhoun S, Chani K, Islam T, Rahevar K, Morishita F, Byrne A, Clark J, Viney K. Tuberculosis related disability: a systematic review and meta-analysis. BMC Medicine. 2021;19:203. 10.1186/s12916-021-02063-9. [Europe PMC free article] [Abstract] [CrossRef] [Google Scholar]
- Allwood B, van der Zalm M, Makanda G, Mortimer K, Steering Committee of the First International Post-Tuberculosis Symposium The long shadow post-tuberculosis. The Lancet. Infectious Diseases. 2019;19:1170–1171. 10.1016/S1473-3099(19)30564-X. [Abstract] [CrossRef] [Google Scholar]
- Arinaminpathy N, Dowdy D. Understanding the incremental value of novel diagnostic tests for tuberculosis. Nature. 2015;528:S60–S67. 10.1038/nature16045. [Abstract] [CrossRef] [Google Scholar]
- Asadi S, Wexler AS, Cappa CD, Barreda S, Bouvier NM, Ristenpart WD. Aerosol emission and superemission during human speech increase with voice loudness. Scientific Reports. 2019;9:2348. 10.1038/s41598-019-38808-z. [Europe PMC free article] [Abstract] [CrossRef] [Google Scholar]
- Baik Y, Nalutaaya A, Kitonsa PJ, Dowdy DW, Katamba A, Kendall EA. Infection status of contacts is not associated with severity of TB in the index case. The International Journal of Tuberculosis and Lung Disease. 2021;25:237–240. 10.5588/ijtld.20.0700. [Europe PMC free article] [Abstract] [CrossRef] [Google Scholar]
- Barry CE, Boshoff HI, Dartois V, Dick T, Ehrt S, Flynn J, Schnappinger D, Wilkinson RJ, Young D. The spectrum of latent tuberculosis: rethinking the biology and intervention strategies. Nature Reviews. Microbiology. 2009;7:845–855. 10.1038/nrmicro2236. [Europe PMC free article] [Abstract] [CrossRef] [Google Scholar]
- Behr MA, Warren SA, Salamon H, Hopewell PC, Ponce de Leon A, Daley CL, Small PM. Transmission of Mycobacterium tuberculosis from patients smear-negative for acid-fast bacilli. Lancet. 1999;353:444–449. 10.1016/s0140-6736(98)03406-0. [Abstract] [CrossRef] [Google Scholar]
- Behr MA, Edelstein PH, Ramakrishnan L. Revisiting the timetable of tuberculosis. BMJ. 2018;362:k2738. 10.1136/bmj.k2738. [Europe PMC free article] [Abstract] [CrossRef] [Google Scholar]
- Deeks J, Higgins J, Altman D. In: Cochrane Handbook for Systematic Reviews of Interventions Version. Deeks J, editor. Cochrane; 2021. pp. 6–8. [Google Scholar]
- Democratic People’s Republic of Korea . National TB Prevalence Survey. WHO; 2016. [Google Scholar]
- Department of Health - Philippines . National Tuberculosis Prevalence Survey. DOH; 2016. [Google Scholar]
- DGHS Ministry of Health and Family Welfare - Bangladesh . National Tuberculosis Prevalence Survey Bangladesh. WHO; 2015. [Google Scholar]
- Dinkele R, Gessner S, McKerry A, Leonard B, Leukes J, Seldon R, Warner DF, Wood R. Aerosolization of Mycobacterium tuberculosis by tidal breathing. American Journal of Respiratory and Critical Care Medicine. 2022;206:206–216. 10.1164/rccm.202110-2378OC. [Europe PMC free article] [Abstract] [CrossRef] [Google Scholar]
- Dodd PJ, Yuen CM, Jayasooriya SM, van der Zalm MM, Seddon JA. Quantifying the global number of tuberculosis survivors: a modelling study. The Lancet. Infectious Diseases. 2021;21:984–992. 10.1016/S1473-3099(20)30919-1. [Abstract] [CrossRef] [Google Scholar]
- Dowdy DW, Basu S, Andrews JR. Is passive diagnosis enough? The impact of subclinical disease on diagnostic strategies for tuberculosis. American Journal of Respiratory and Critical Care Medicine. 2013;187:543–551. 10.1164/rccm.201207-1217OC. [Europe PMC free article] [Abstract] [CrossRef] [Google Scholar]
- Drain PK, Bajema KL, Dowdy D, Dheda K, Naidoo K, Schumacher SG, Ma S, Meermeier E, Lewinsohn DM, Sherman DR. Incipient and subclinical tuberculosis: a clinical review of early stages and progression of infection. Clinical Microbiology Reviews. 2018;31:e00021-18. 10.1128/CMR.00021-18. [Europe PMC free article] [Abstract] [CrossRef] [Google Scholar]
- Emery JC, Russell TW, Liu Y, Hellewell J, Pearson CA, CMMID COVID-19 Working Group. Knight GM, Eggo RM, Kucharski AJ, Funk S, Flasche S, Houben RM. The contribution of asymptomatic SARS-CoV-2 infections to transmission on the Diamond Princess cruise ship. eLife. 2020;9:e58699. 10.7554/eLife.58699. [Europe PMC free article] [Abstract] [CrossRef] [Google Scholar]
- Emery JC Subclinical_Transmission. swh:1:rev:0e2457d1c7660ed07e3327a045858d44b25fc07eSoftware Heritage. 2022 https://archive.softwareheritage.org/swh:1:dir:38f4bcd1ec49905466dda378142d50962f2268d6;origin=https://github.com/epidemery/subclinical_transmission;visit=swh:1:snp:f788d6abafa11d062f531c61d7bbc91f244563c3;anchor=swh:1:rev:0e2457d1c7660ed07e3327a045858d44b25fc07e
- Esmail H, Macpherson L, Coussens AK, Houben RMGJ. Mind the gap - Managing tuberculosis across the disease spectrum. EBioMedicine. 2022;78:103928. 10.1016/j.ebiom.2022.103928. [Europe PMC free article] [Abstract] [CrossRef] [Google Scholar]
- Federal Republic of Nigeria . Report First National TB Prevalence Survey. Report; 2012. [Google Scholar]
- Frascella B, Richards AS, Sossen B, Emery JC, Odone A, Law I, Onozaki I, Esmail H, Houben RMGJ. Subclinical Tuberculosis Disease—A Review and Analysis of Prevalence Surveys to Inform Definitions, Burden, Associations, and Screening Methodology. Clinical Infectious Diseases. 2021;73:e830–e841. 10.1093/cid/ciaa1402. [Europe PMC free article] [Abstract] [CrossRef] [Google Scholar]
- Glynn JR, Guerra-Assunção JA, Houben RMGJ, Sichali L, Mzembe T, Mwaungulu LK, Mwaungulu JN, McNerney R, Khan P, Parkhill J, Crampin AC, Clark TG, Cardona P-J. Whole Genome Sequencing Shows a Low Proportion of Tuberculosis Disease Is Attributable to Known Close Contacts in Rural Malawi. PLOS ONE. 2015;10:e0132840. 10.1371/journal.pone.0132840. [Europe PMC free article] [Abstract] [CrossRef] [Google Scholar]
- Glynn JR, McLean E, Malava J, Dube A, Katundu C, Crampin AC, Geis S. Effect of acute illness on contact patterns, malawi, 2017. Emerging Infectious Diseases. 2020;26:44–50. 10.3201/eid2601.181539. [Europe PMC free article] [Abstract] [CrossRef] [Google Scholar]
- Golub JE, Mohan CI, Comstock GW, Chaisson RE. Active case finding of tuberculosis: historical perspective and future prospects. The International Journal of Tuberculosis and Lung Disease. 2005;9:1183–1203. [Europe PMC free article] [Abstract] [Google Scholar]
- Grzybowski S, Barnett GD, Styblo K. Contacts of cases of active pulmonary tuberculosis. Bulletin of the International Union against Tuberculosis. 1975;50:90–106. [Abstract] [Google Scholar]
- Hernández-Garduño E, Cook V, Kunimoto D, Elwood RK, Black WA, FitzGerald JM. Transmission of tuberculosis from smear negative patients: a molecular epidemiology study. Thorax. 2004;59:286–290. 10.1136/thx.2003.011759. [Europe PMC free article] [Abstract] [CrossRef] [Google Scholar]
- Hoa NB, Sy DN, Nhung NV, Tiemersma EW, Borgdorff MW, Cobelens FGJ. National survey of tuberculosis prevalence in Viet Nam. Bulletin of the World Health Organization. 2010;88:273–280. 10.2471/BLT.09.067801. [Europe PMC free article] [Abstract] [CrossRef] [Google Scholar]
- Houben RMGJ, Esmail H, Emery JC, Joslyn LR, McQuaid CF, Menzies NA, Sanz J, Shrestha S, White RG, Yang C, Cobelens F. Spotting the old foe-revisiting the case definition for TB. The Lancet. Respiratory Medicine. 2019;7:199–201. 10.1016/S2213-2600(19)30038-4. [Abstract] [CrossRef] [Google Scholar]
- Johansson MA, Quandelacy TM, Kada S, Prasad PV, Steele M, Brooks JT, Slayton RB, Biggerstaff M, Butler JC. SARS-CoV-2 Transmission From People Without COVID-19 Symptoms. JAMA Network Open. 2021;4:e2035057. 10.1001/jamanetworkopen.2020.35057. [Europe PMC free article] [Abstract] [CrossRef] [Google Scholar]
- Kendall EA. When infections don’t reflect infectiousness: interpreting contact investigation data with care. Clinical Infectious Diseases. 2021;73:e3456–e3458. 10.1093/cid/ciaa1144. [Europe PMC free article] [Abstract] [CrossRef] [Google Scholar]
- Kendall EA, Shrestha S, Dowdy DW. The Epidemiological Importance of Subclinical Tuberculosis. A Critical Reappraisal. American Journal of Respiratory and Critical Care Medicine. 2021;203:168–174. 10.1164/rccm.202006-2394PP. [Europe PMC free article] [Abstract] [CrossRef] [Google Scholar]
- Krivinka R, Drápela J, Kubík A, Danková D, Krivánek J, Ruzha J, Miková Z, Hejdová E. Epidemiological and clinical study of tuberculosis in the district of Kolín, Czechoslovakia. Second report (1965-1972) Bulletin of the World Health Organization. 1974;51:59–69. [Europe PMC free article] [Abstract] [Google Scholar]
- Ku C-C, MacPherson P, Khundi M, Nzawa Soko RH, Feasey HRA, Nliwasa M, Horton KC, Corbett EL, Dodd PJ. Durations of asymptomatic, symptomatic, and care-seeking phases of tuberculosis disease with a Bayesian analysis of prevalence survey and notification data. BMC Medicine. 2021;19:298. 10.1186/s12916-021-02128-9. [Europe PMC free article] [Abstract] [CrossRef] [Google Scholar]
- Law I, Sylavanh P, Bounmala S, Nzabintwali F, Paboriboune P, Iem V, Somphavong S, Kim SJ, Sismanidis C, Onozaki I, Sebert J. The first national tuberculosis prevalence survey of Lao PDR (2010-2011) Tropical Medicine & International Health. 2015;20:1146–1154. 10.1111/tmi.12536. [Abstract] [CrossRef] [Google Scholar]
- Law I, Floyd K, Group ATPS. National tuberculosis prevalence surveys in Africa, 2008-2016: an overview of results and lessons learned. Tropical Medicine & International Health. 2020;25:1308–1327. 10.1111/tmi.13485. [Europe PMC free article] [Abstract] [CrossRef] [Google Scholar]
- Leung NHL, Chu DKW, Shiu EYC, Chan K-H, McDevitt JJ, Hau BJP, Yen H-L, Li Y, Ip DKM, Peiris JSM, Seto W-H, Leung GM, Milton DK, Cowling BJ. Author Correction: Respiratory virus shedding in exhaled breath and efficacy of face masks. Nature Medicine. 2020;26:981. 10.1038/s41591-020-0946-9. [Europe PMC free article] [Abstract] [CrossRef] [Google Scholar]
- Liu Y, Funk S, Flasche S, Centre for Mathematical Modelling of Infectious Diseases nCoV Working Group The contribution of pre-symptomatic infection to the transmission dynamics of COVID-2019. Wellcome Open Research. 2020;5:58. 10.12688/wellcomeopenres.15788.1. [Europe PMC free article] [Abstract] [CrossRef] [Google Scholar]
- Madhani F, Maniar RA, Burfat A, Ahmed M, Farooq S, Sabir A, Domki AK, Page-Shipp L, Khowaja S, Safdar N, Khan AJ, Khan PY. Automated chest radiography and mass systematic screening for tuberculosis. The International Journal of Tuberculosis and Lung Disease. 2020;24:665–673. 10.5588/ijtld.19.0501. [Abstract] [CrossRef] [Google Scholar]
- Mandal S, Chadha VK, Laxminarayan R, Arinaminpathy N. Counting the lives saved by DOTS in India: a model-based approach. BMC Medicine. 2017;15:47. 10.1186/s12916-017-0809-5. [Europe PMC free article] [Abstract] [CrossRef] [Google Scholar]
- Marks GB, Nguyen NV, Nguyen PTB, Nguyen T-A, Nguyen HB, Tran KH, Nguyen SV, Luu KB, Tran DTT, Vo QTN, Le OTT, Nguyen YH, Do VQ, Mason PH, Nguyen V-AT, Ho J, Sintchenko V, Nguyen LN, Britton WJ, Fox GJ. Community-wide screening for tuberculosis in a high-prevalence setting. The New England Journal of Medicine. 2019;381:1347–1357. 10.1056/NEJMoa1902129. [Abstract] [CrossRef] [Google Scholar]
- Ministry of Health - Cambodia . Report of the Second National Tuberculosis Prevalence Survey. WHO; 2011. [Google Scholar]
- Ministry of Health - Mongolia . Report of the First National Tuberculosis Prevalence Survey in Mongolia 2014-2015. WHO; 2016. [Google Scholar]
- Ministry of Health - Myanmar . Report on National TB Prevalence Survey 2009–2010. WHO; 2010. [Google Scholar]
- Ministry of Health, Republic of Indonesia . Indonesia Tuberculosis Prevalence Survey 2013-2014. National Institute of Health Research and Development in collaboration with Directorate General of Disease Control and Environmental Health; 2015. [Google Scholar]
- Ministry of Health - Rwanda . Report of the First National Pulmonary Tuberculosis Prevalence Survey in Rwanda. WHO; 2014. [Google Scholar]
- Ministry of Health - Vietnam . National Tuberculosis Programme Report: National TB Prevalence Survey in Vietnam 2006-2007. WHO; 2008. [Google Scholar]
- Ministry of Health and Child Care – Zimbabwe . Report: the zimbabwe national population based tuberculosis prevalence survey. Ministry of Health and Child Care; 2014. [Google Scholar]
- Ministry of Health and Social Welfare - The Gambia . First Ethiopian National Population Based Tuberculosis Prevalence Survey. WHO; 2011. [Google Scholar]
- Nathavitharana RR, Garcia-Basteiro AL, Ruhwald M, Cobelens F, Theron G. Reimagining the status quo: How close are we to rapid sputum-free tuberculosis diagnostics for all? EBioMedicine. 2022;78:103939. 10.1016/j.ebiom.2022.103939. [Europe PMC free article] [Abstract] [CrossRef] [Google Scholar]
- National Department of Health - South Africa The First National TB Prevalence Survey. 2021. [February 5, 2021]. https://www.knowledgehub.org.za/system/files/elibdownloads/2021-02/A4_SA_TPS%20Short%20Report_10June20_Final_highres.pdf
- Nguyen HV, Tiemersma EW, Nguyen HB, Cobelens FGJ, Finlay A, Glaziou P, Dao CH, Mirtskhulava V, Nguyen HV, Pham HTT, Khieu NTT, de Haas P, Do NH, Nguyen PD, Cung CV, Nguyen NV. The second national tuberculosis prevalence survey in Vietnam. PLOS ONE. 2020;15:e0232142. 10.1371/journal.pone.0232142. [Europe PMC free article] [Abstract] [CrossRef] [Google Scholar]
- Nguyen HV, Tiemersma E, Nguyen NV, Nguyen HB, Cobelens F. Disease transmission by patients with subclinical tuberculosis. Clinical Infectious Diseases. 2023;76:2000–2006. 10.1093/cid/ciad027. [Europe PMC free article] [Abstract] [CrossRef] [Google Scholar]
- Onozaki I, Law I, Sismanidis C, Zignol M, Glaziou P, Floyd K. National tuberculosis prevalence surveys in Asia, 1990-2012: an overview of results and lessons learned. Tropical Medicine & International Health. 2015;20:1128–1145. 10.1111/tmi.12534. [Abstract] [CrossRef] [Google Scholar]
- Patterson B, Wood R. Is cough really necessary for TB transmission? Tuberculosis. 2019;117:31–35. 10.1016/j.tube.2019.05.003. [Europe PMC free article] [Abstract] [CrossRef] [Google Scholar]
- Pedrazzoli D, Carter DJ, Borghi J, Laokri S, Boccia D, Houben RM. Does Ghana’s National Health Insurance Scheme provide financial protection to tuberculosis patients and their households? Social Science & Medicine. 2021;277:113875. 10.1016/j.socscimed.2021.113875. [Abstract] [CrossRef] [Google Scholar]
- Ragonnet R, Flegg JA, Brilleman SL, Tiemersma EW, Melsew YA, McBryde ES, Trauer JM. Revisiting the natural history of pulmonary tuberculosis: a bayesian estimation of natural recovery and mortality rates. Clinical Infectious Diseases. 2021;73:e88–e96. 10.1093/cid/ciaa602. [Abstract] [CrossRef] [Google Scholar]
- R Development Core Team . Vienna, Austria: R Foundation for Statistical Computing; 2014. https://www.r-project.org [Google Scholar]
- Richards AS, Sossen B, Emery JC, Horton KC, Heinsohn T, Frascella B, Balzarini F, Oradini-Alacreu A, Hacker B, Odone A, McCreesh N, Grant AD, Kranzer K, Cobelens F, Esmail H, Houben RM. The Natural History of TB Disease-a Synthesis of Data to Quantify Progression and Regression across the Spectrum. bioRxiv. 2021 10.1101/2021.09.13.21263499. [CrossRef]
- Riley RD, Higgins JPT, Deeks JJ. Interpretation of random effects meta-analyses. BMJ. 2011;342:d549. 10.1136/bmj.d549. [Abstract] [CrossRef] [Google Scholar]
- Scriba TJ, Fiore-Gartland A, Penn-Nicholson A, Mulenga H, Kimbung Mbandi S, Borate B, Mendelsohn SC, Hadley K, Hikuam C, Kaskar M, Musvosvi M, Bilek N, Self S, Sumner T, White RG, Erasmus M, Jaxa L, Raphela R, Innes C, Brumskine W, Hiemstra A, Malherbe ST, Hassan-Moosa R, Tameris M, Walzl G, Naidoo K, Churchyard G, Hatherill M, CORTIS-01 Study Team Biomarker-guided tuberculosis preventive therapy (CORTIS): a randomised controlled trial. The Lancet. Infectious Diseases. 2021;21:354–365. 10.1016/S1473-3099(20)30914-2. [Europe PMC free article] [Abstract] [CrossRef] [Google Scholar]
- Silva S, Arinaminpathy N, Atun R, Goosby E, Reid M. Economic impact of tuberculosis mortality in 120 countries and the cost of not achieving the Sustainable Development Goals tuberculosis targets: a full-income analysis. The Lancet. Global Health. 2021;9:e1372–e1379. 10.1016/S2214-109X(21)00299-0. [Europe PMC free article] [Abstract] [CrossRef] [Google Scholar]
- Slater HC, Ross A, Felger I, Hofmann NE, Robinson L, Cook J, Gonçalves BP, Björkman A, Ouedraogo AL, Morris U, Msellem M, Koepfli C, Mueller I, Tadesse F, Gadisa E, Das S, Domingo G, Kapulu M, Midega J, Owusu-Agyei S, Nabet C, Piarroux R, Doumbo O, Doumbo SN, Koram K, Lucchi N, Udhayakumar V, Mosha J, Tiono A, Chandramohan D, Gosling R, Mwingira F, Sauerwein R, Paul R, Riley EM, White NJ, Nosten F, Imwong M, Bousema T, Drakeley C, Okell LC. The temporal dynamics and infectiousness of subpatent Plasmodium falciparum infections in relation to parasite density. Nature Communications. 2019;10:1433. 10.1038/s41467-019-09441-1. [Europe PMC free article] [Abstract] [CrossRef] [Google Scholar]
- Stan Development Team Rstan: the R interface to Stan. 2.21.2R Package Version. 2020 http://mc-stan.org/
- Stan Development Team Stan modeling language users guide and reference manual. 2.28Stan. 2021 https://mc-stan.org
- The Republic of Uganda . The Uganda National Tuberculosis Prevalence Survey, 2014-2015 Survey Report. WHO; 2015. [Google Scholar]
- Theron G, Limberis J, Venter R, Smith L, Pietersen E, Esmail A, Calligaro G, Te Riele J, de Kock M, van Helden P, Gumbo T, Clark TG, Fennelly K, Warren R, Dheda K. Bacterial and host determinants of cough aerosol culture positivity in patients with drug-resistant versus drug-susceptible tuberculosis. Nature Medicine. 2020;26:1435–1443. 10.1038/s41591-020-0940-2. [Europe PMC free article] [Abstract] [CrossRef] [Google Scholar]
- Tostmann A, Kik SV, Kalisvaart NA, Sebek MM, Verver S, Boeree MJ, van Soolingen D. Tuberculosis transmission by patients with smear-negative pulmonary tuberculosis in a large cohort in the Netherlands. Clinical Infectious Diseases. 2008;47:1135–1142. 10.1086/591974. [Abstract] [CrossRef] [Google Scholar]
- Tupasi TE, Radhakrishna S, Rivera AB, Pascual ML, Quelapio MI, Co VM, Villa ML, Beltran G, Legaspi JD, Mangubat NV, Sarol JN, Jr, Reyes AC, Sarmiento A, Solon M, Solon FS, Mantala MJ. The 1997 nationwide tuberculosis prevalence survey in the philippines. The International Journal of Tuberculosis and Lung Disease. 1999;3:471–477. [Abstract] [Google Scholar]
- UNAIDS Core epidemiology slides. 2021. [November 30, 2021]. https://www.unaids.org/en/resources/documents/2021/core-epidemiology-slides
- Williams CML, Cheah ESG, Malkin J, Patel H, Otu J, Mlaga K, Sutherland JS, Antonio M, Perera N, Woltmann G, Haldar P, Garton NJ, Barer MR. Face mask sampling for the detection of Mycobacterium tuberculosis in expelled aerosols. PLOS ONE. 2014;9:e104921. 10.1371/journal.pone.0104921. [Europe PMC free article] [Abstract] [CrossRef] [Google Scholar]
- Williams CM, Abdulwhhab M, Birring SS, De Kock E, Garton NJ, Townsend E, Pareek M, Al-Taie A, Pan J, Ganatra R, Stoltz AC, Haldar P, Barer MR. Exhaled Mycobacterium tuberculosis output and detection of subclinical disease by face-mask sampling: prospective observational studies. The Lancet. Infectious Diseases. 2020;20:607–617. 10.1016/S1473-3099(19)30707-8. [Europe PMC free article] [Abstract] [CrossRef] [Google Scholar]
- World Health Organisation WHO Expert Committee on Tuberculosis - Ninth Report Geneva. 1974. [September 17, 2019]. https://apps.who.int/iris/bitstream/handle/10665/41095/WHO_TRS_552_eng.pdf?sequence=1&isAllowed=y [Abstract]
- World Health Organisation . Tuberculosis Prevalence Surveys: A Handbook. Geneva: WHO; 2011. [Google Scholar]
- World Health Organisation Global tuberculosis report 2021. 2021. [October 17, 2021]. https://www.who.int/publications/i/item/9789240037021
- World Health Organisation End TB Strategy. 2022. [June 9, 2022]. https://www.who.int/teams/global-tuberculosis-programme/the-end-tb-strategy
- World Health Organization Tuberculosis fact sheet. 2020. [December 14, 2020]. https://www.who.int/news-room/fact-sheets/detail/tuberculosis
- World Health Organization WHO consolidated guidelines on tuberculosis - Module 2: Systematic screening for tuberculosis disease Genève: World Health Organisation 2021. 2021. [September 9, 2021]. https://apps.who.int/iris/handle/10665/341426 [Abstract]
- Xu Y, Cancino-Muñoz I, Torres-Puente M, Villamayor LM, Borrás R, Borrás-Máñez M, Bosque M, Camarena JJ, Colomer-Roig E, Colomina J, Escribano I, Esparcia-Rodríguez O, Gil-Brusola A, Gimeno C, Gimeno-Gascón A, Gomila-Sard B, González-Granda D, Gonzalo-Jiménez N, Guna-Serrano MR, López-Hontangas JL, Martín-González C, Moreno-Muñoz R, Navarro D, Navarro M, Orta N, Pérez E, Prat J, Rodríguez JC, Ruiz-García MM, Vanaclocha H, Colijn C, Comas I. High-resolution mapping of tuberculosis transmission: Whole genome sequencing and phylogenetic modelling of a cohort from Valencia Region, Spain. PLOS Medicine. 2019;16:e1002961. 10.1371/journal.pmed.1002961. [Europe PMC free article] [Abstract] [CrossRef] [Google Scholar]
- Yoon C, Chaisson LH, Patel SM, Allen IE, Drain PK, Wilson D, Cattamanchi A. Diagnostic accuracy of C-reactive protein for active pulmonary tuberculosis: a meta-analysis. The International Journal of Tuberculosis and Lung Disease. 2017;21:1013–1019. 10.5588/ijtld.17.0078. [Europe PMC free article] [Abstract] [CrossRef] [Google Scholar]
- Zaman K, Hossain S, Banu S, Quaiyum MA, Barua PC, Salim MAH, Begum V, Islam MA, Ahmed J, Rifat M, Cooreman E, Van Der Werf MJ, Borgdorff M, Van Leth F. Prevalence of smear-positive tuberculosis in persons aged ≥ 15 years in Bangladesh: results from a national survey, 2007-2009. Epidemiology and Infection. 2012;140:1018–1027. 10.1017/S0950268811001609. [Abstract] [CrossRef] [Google Scholar]
This important study estimates the amount of tuberculosis transmission attributable to subclinical (asymptomatic) cases at the population level. The authors rely on existing survey data on household contacts of index cases with tuberculosis, and their respective symptomatic and bacteriological status. A solid, novel approach is proposed to incorporate this existing data to produce meaningful indicators of the relative importance of subclinical tuberculosis in sustaining the global tuberculosis epidemic. In addition to being of interest to tuberculosis epidemiologists, these results will be an important resource for policymakers in the ongoing debate about tuberculosis case finding and the role of symptom screening algorithms.
Decision letter
Decision letter after peer review:
Thank you for submitting your article "Estimating the contribution of subclinical tuberculosis disease to transmission: an individual patient data analysis from prevalence surveys" for consideration by eLife. Your article has been reviewed by 3 peer reviewers, and the evaluation has been overseen by a Reviewing Editor and Bavesh Kana as the Senior Editor. The reviewers have opted to remain anonymous.
The reviewers have discussed their reviews with one another, and the Reviewing Editor has drafted this to help you prepare a revised submission.
Essential revisions:
1. The authors should run a sensitivity analysis on:
– The assumption that smear and symptom status are constant over time since this is almost certainly not the case. This could be accomplished, for example, by using the model described in sensitivity analysis #2 – by assigning estimates of per–unit–time relative infectiousness to each state in that model and then calibrating those estimates to the cumulative hazard ratios that are estimated in this study.
– The assumption that the background hazard of infection is the same across all households in a given survey/study. For example, do results change much if households with an index case have a 50% higher background hazard of infection?
– The durations of smear–positive and smear–negative TB are the same. For example, do results change much if smear–positive TB lasts twice as long as smear–negative TB?
2. Did the authors consider fitting a single cumulative hazard model for all 5 countries, that would allow only Λ_B (background cumulative hazard) to vary by country, rather than fitting 5 separate models and then pooling the estimates for the other lambdas after fitting? Does the former approach allow for estimation of the other λ's with more precision – unless the authors have reason to believe the λ's will vary by setting?
3. How were households with multiple TB cases treated in the analysis? If they were excluded, what effect is this likely to have on the analysis? This should be described somewhere (at least in the supplement) and the likely impact on results discussed.
4. Given how much the Philippines 1997 prevalence survey seems to be driving results (in terms of the larger sample size of household contacts), it would be useful to show how the percent of transmission results in Figure 3 look when applied to the Philippines 1997 survey data. Currently only one of the four surveys/studies used to derive the relative infectiousness estimates (Vietnam 2007) is used in the percent of transmission estimates.
Other issues that should be addressed:
1. The finding that subclinical TB is likely to be more transmissible than symptomatic TB is counterintuitive and warrants much more discussion.
2. It may be useful to clarify to readers how Mtb infection differs from subclinical TB (or TB more generally).
3. Figure 1 presents the odds ratios for infection of smear–positive vs. smear–negative (on the left) and clinical vs. subclinical TB (on the right). Figure 2 presents relative per–unit–time infectiousness estimates in the exact opposite way (smear–negative vs. smear–positive and subclinical vs. clinical, and in the opposite order). It would be clearer for readers if the presentation was more consistent across figures (and accompanying text).
4. Presenting figure 1 in terms of the relative cumulative hazard rather than (or in addition to) odds ratios would also help with the continuity between figures 1 and 2 (since the estimates of per–unit–time relative infectiousness used the relative cumulative hazards, not odds ratios).
5. Methods text should contain a bit more information on how the estimated durations of subclinical and clinical TB were used to convert the infection odds ratios into per–unit–time relative infectiousness estimates – this is clear in the appendix but not in the main text.
6. In the abstract, the term "individual population data" is a bit unclear.
7. Supplementary figure 2 may be mislabeled – for Bangladesh, it seemed that only smear–positive cases were included but the graph makes it look like only subclinical cases were included. Are the other panels similarly mislabeled?
8. p5 (line 7–9) Sentence could be made clearer. What does it mean there is a "disconnect"? Is this a pathological link? or causality? lack of evidence?
9. Results: The estimations of per unit time infectiousness of subclinical TB should be brought up front in the results, before the meta–analysis.
10. The discussion around the limitations of household contact and transmission assessment is very limited. This study inherits the limitations of the source studies, a more robust discussion around this issue would be beneficial.
11. Sensitivity of symptom screening algorithms is not discussed: TB disease, although split it into two categories is more likely a continuum.
12. Discussion page 15 (L11–L12): Is it relevant to mention SARS–CoV and Malaria here? Bringing asymptomatic transmission as a transversal subject across diseases without providing more epidemiological insights seems gratuitous. This should be removed.
13. The implications on policy and directly on recommendations around symptom screening in WHO plans are not commented on enough, this is where these results could be more useful.
14. page 16, line 32: The sentence "This is in keeping with increasing data showing that symptoms, in particular the classic TB symptom of cough, are not closely correlated to the amount of Mtb exhaled [28,29] " This is not completely consistent with Ref [28] by Theron et al. Nature Med 2020. The authors actually found that cough alone was a strong indicator for the measured transmissibility supplementary table 8. OR for CASS positivity for cough: 3.38 (1.90–5.99) despite the overall symptom score being negatively associated with transmissibility.
15. Supplementary Table 1: would be useful to add information on the definition of symptoms used to define subclinical against clinical disease.
16. Is there evidence from the literature regarding the subclinical transmission using results from whole genome sequencing? For example, Xu et al. (plos medicine) found in a study from Spain that 5/14 (35.7%) cases likely transmitted TB well before symptom onset. This can be discussed.
Reviewer #1 (Recommendations for the authors):
Slow declines in the global burden of TB are driven by a large gap between TB prevalence and the number of people with TB that are diagnosed and treated. Subclinical TB contributes to this "prevalence–notification gap"; a substantial (around 50%) of people with TB disease do not present with TB symptoms (or have fewer or milder symptoms that would not screen positive on a typical symptom screening questionnaire). The extent to which detecting and curing people with subclinical TB is important to reducing population–level TB transmission (and thus TB burden) is unclear, and depends both on infectiousness (how infectious people with subclinical TB are at any given point in time) and duration (how long they are expected to have TB before being detected or resolving on their own; and their associated future trajectory of infectiousness). Shedding light on this question could have a substantial impact on the global strategy for ending TB, including whether it is important for mass screening efforts (and other strategies to reduce the prevalence–notification gap) to detect those with subclinical TB and, if so, how to prioritize future diagnostics that best accomplish this (as the authors discuss in the last paragraph of the Discussion section).
In this study, the authors aimed to assess the infectiousness of people with subclinical TB (relative to symptomatic TB) at any given point in time; i.e., adjusting for the duration. To do this, they used individual– and household–level data from 4 cross–sectional surveys/studies that collected information on the prevalence of symptomatic TB, subclinical TB, and TB infection in households. The cumulative relative infectiousness of subclinical vs. symptomatic TB can be estimated by assessing how much higher the prevalence of TB infection is in households with a subclinical vs. symptomatic TB case. However, this estimate of cumulative relative infectiousness combines both pre–unit–time infectiousness and duration. To adjust for the duration and estimate per–unit–time relative infectiousness, the authors employed a cumulative hazard model that they then adjusted for estimates of the duration of symptomatic and subclinical TB; these duration estimates came from a mathematical model of TB natural history that is described in a different paper by Ku et al.
Strengths of the analysis include the separation of the duration of different forms of TB to estimate the per–unit–time relative infectiousness, as well as the Bayesian framework used to infer the cumulative relative hazards of infection from different forms of TB. Weaknesses include a number of assumptions made in the analysis that are unlikely to hold true and could bias results: the assumption that household index cases have continually had the same form of TB that they had when they were detected in a prevalence survey (or active case finding study), that smear–positive and smear–negative forms of TB are of the same duration, and that the background hazard of infection is the same for all households in a given survey/study. It would be useful for the authors to conduct a sensitivity analysis on these three assumptions. For example, in sensitivity analysis they estimate the durations of subclinical and symptomatic TB using a model that allows populations to transition between these states – could that model not be used to infer the per–unit–time relative infectiousness estimates? Additionally, historical data from the pre–antibiotic era (and a recent modeling study based on this data https://doi.org/10.1073/pnas.2211045119) suggests differential disease duration by smear status. In countries where a lot of TB is still diagnosed using smears (or was at the time of these prevalence surveys), differential detection/treatment probabilities could also lead to differential disease duration. This could have important implications for the analysis, given that symptom and smear status seem to be somewhat correlated in prevalence surveys (subclinical cases are much more likely to be smear–negative than smear–positive) and that smear status is more strongly predictive of infectiousness.
In all three cases, it is difficult to predict the direction and magnitude of potential bias on results, which is why sensitivity analysis is important and could strengthen the extent to which the authors' conclusions are justified by the analysis (currently this is somewhat unclear). Furthermore, the conclusion that subclinical TB is actually more transmissible than symptomatic TB is counterintuitive and warrants more discussion than is currently included in the study.
Despite these limitations, the broad conclusion that a substantial portion of TB transmission comes from subclinical cases will probably continue to hold even with adjustments to the analysis and additional sensitivity analyses. Even at the lower bound of the wide current estimated prediction interval, 27% of transmission coming from TB is substantial. This is an important finding, and thus additional analysis/revisions that can strengthen the robustness of this finding would be valuable.
Suggestions on analysis
– Suggest running sensitivity analysis on the assumption that smear and symptom status are constant over time since this is almost certainly not the case. This could be accomplished, for example, by using the model described in sensitivity analysis #2 – by assigning estimates of per–unit–time relative infectiousness to each state in that model and then calibrating those estimates to the cumulative hazard ratios that are estimated in this study.
– Suggest running sensitivity analysis on the assumption that the background hazard of infection is the same across all households in a given survey/study. For example, do results change much if households with an index case have a 50% higher background hazard of infection?
– Suggest running sensitivity analysis on the assumption that the durations of smear–positive and smear–negative TB are the same. For example, do results change much if smear–positive TB lasts twice as long as smear–negative TB?
– Did the authors consider fitting a single cumulative hazard model for all 5 countries, which would allow only Λ_B (background cumulative hazard) to vary by country, rather than fitting 5 separate models and then pooling the estimates for the other lambdas after fitting? I wonder if the former approach could allow for the estimation of the other lambdas with more precision – unless the authors have reason to believe the lambdas will vary by setting.
– How were households with multiple TB cases treated in the analysis? If they were excluded, what effect is this likely to have on the analysis? The effect could be to exclude those households that had the highest hazard of infection. This should be described somewhere (at least in the supplement) and the likely impact on results discussed.
– Given how much the Philippines 1997 prevalence survey seems to be driving results (in terms of the larger sample size of household contacts), it would be useful to show how the percent of transmission results in Figure 3 look when applied to the Philippines 1997 survey data. Currently only one of the four surveys/studies used to derive the relative infectiousness estimates (Vietnam 2007) is used in the percent of transmission estimates.
Suggestions on presentation
– The finding that subclinical TB is likely to be more transmissible than symptomatic TB is counterintuitive and warrants much more discussion (I don't believe this is currently discussed at all)
– Depending on the intended audience, it may be useful to clarify to readers how Mtb infection differs from subclinical TB (or TB more generally).
– Figure 1 presents the odds ratios for infection of smear–positive vs. smear–negative (on the left) and clinical vs. subclinical TB (on the right). Figure 2 presents relative per–unit–time infectiousness estimates in the exact opposite way (smear–negative vs. smear–positive and subclinical vs. clinical, and in the opposite order). It would be clearer for readers if the presentation was more consistent across figures (and accompanying text).
– Presenting figure 1 in terms of the relative cumulative hazard rather than (or in addition to) odds ratios would also help with the continuity between figures 1 and 2 (since the estimates of per–unit–time relative infectiousness used the relative cumulative hazards, not odds ratios).
– Methods text should contain a bit more information (like 1 sentence) on how the estimated durations of subclinical and clinical TB were used to convert the infection odds ratios into per–unit–time relative infectiousness estimates – this is clear in the appendix but not in the main text.
Reviewer #2 (Recommendations for the authors):
Emery et al., present for the first time quantitative estimates of the relative infectiousness of subclinical TB cases and the overall contribution of these to the global and country–specific (selected countries) ongoing TB transmission. The authors review and aggregate individual–level data from studies where household contacts and their index cases were assessed for their bacteriological and symptomatic status in order to reclassify and reanalyse household transmission accordingly. They produce aggregated estimates of the Odds Ratio of TB infection for households of subclinical TB index cases vs clinical index cases, and more importantly, they use a novel approach that uses cumulative hazard models to estimate the per unit infectiousness of subclinical TB. This approach accounts for important considerations like background TB transmission and duration of the infectious period. Their results (for ORs and infectiousness) show no significant advantage in the clinical index cases for infection. In light of these results, one can conclude that subclinical TB cases have no transmission disadvantage compared to symptomatic TB cases, and given its high prevalence in high–burden settings might be silently contributing disproportionately to the overall burden of TB. The implications of these results are that current approaches to active case finding and household investigation interventions put most of their efforts to identify symptomatic TB cases, potentially missing important sources of infection.
The main observable limitations of this work are "inherited" from the sources of data, namely:
1. Household contacts of index cases are a very indirect (although useful) way of assessing TB transmission and infectiousness: The direction of transmission (index case to contact) can never truly be asserted, not even can it be ruled out to have occurred from external sources. The authors only briefly mention this but it is the main source of uncertainty when discussing TB household transmission investigation. The approach here proposed does not address these points, and thus it remains a gap and a challenge in the research field.
2. Assessment of TB symptoms is in itself a type of diagnostic tool with a measurable level of performance (sensitivity/specificity). This can drastically alter the estimation of relative infectiousness by clinical/subclinical status. The authors don't mention this and it is not immediately clear how this was done in the source studies.
I have enjoyed having the opportunity of reviewing this manuscript, which I think can be a widely used resource for policymakers, mathematical modellers, and epidemiologists working on TB. The main advantages and solid points I found are summarised in my public review and assessment. Here I add some points that I think need to be addressed to improve the presentation and conveyance of the message.
– p5 (line 7–9) Sentence could be made clearer. What does it mean there is a "disconnect"? Is this a pathological link? or causality? lack of evidence?
– Results: In my view, the estimations of per unit time infectiousness of subclinical TB should be brought up front in the results, before the meta–analysis.
– The discussion around the limitations of household contact and transmission assessment is very limited. As I said before, this study inherits the limitations of the source studies but a more robust discussion around this issue would feel healthier (Kendall editorial note, etc..)
– Sensitivity of symptom screening algorithms is not discussed: TB disease, although we split it into two categories is more likely a continuum, and how good we get at identifying those signs determines how much we will trust these results in the future.
– Discussion page 15 (L11–L12): I wonder how relevant is to mention SARS–CoV and Malaria here. Bringing asymptomatic transmission as a transversal subject across diseases without providing more epidemiological insights seems gratuitous. I would suggest either removing or expanding.
– The implications on policy and directly on recommendations around symptom screening in WHO plans are not commented on enough, and I believe this is where these results could be more useful.
Reviewer #3 (Recommendations for the authors):
The investigators applied a statistical framework to synthesize information from prevalence surveys of active TB disease (regardless of symptoms) and latent tuberculosis infection among household contacts of index cases from tuberculosis surveys. They estimated an odds ratio of 1.2 (95% CI: 0.6–2.3) for infection in a household with a clinical versus subclinical index case (not considering the duration of the disease). They estimated that 68% (27–92%) of global tuberculosis transmission was from subclinical TB.
The major strength of the study is the ability to collect relevant information from prevalence surveys and integrate it using a sophisticated statistical framework. The result is novel in the sense that no such estimation has been provided before.
One major limitation of the study, as acknowledged by the authors, is the very wide confidence interval (27–92%) for the estimated global proportion of transmission contributed by subclinical disease. The wide 95% CI was consistent with a range from modest to very large impact of subclinical TB and therefore has different policy implications for tuberculosis control. It will be useful if the authors could indicate the major source of uncertainty behind this confidence interval. In other words, where and how should we collect more data to improve the precision of this estimation procedure?
Another limitation of the study is the lack of consideration of the conversion between subclinical and clinical TB status (i.e., not a one–way street) in the cumulative hazard model. Its impact on the main finding (the transmission contribution from subclinical disease) remains unclear. Considering the natural history of TB is usually from subclinical to clinical (with conversion and reversion along the way), it is anticipated that the cumulative latent infection among household contacts of a clinical TB index case would likely be contributed by both the clinical period as well as the subclinical period. This "misclassification" of clinical vs. subclinical TB seems to bias the relative infectiousness (clinical vs. subclinical) towards the null value of OR=1, under the hypothesis that clinical TB is more infectious than subclinical TB. In other words, it might therefore underestimate the relative transmissibility of clinical TB.
One of the major results of the study was that an estimated 68% (27–92%, 95% PrI) of global transmission was from subclinical TB. Given that there is substantial between–country variation in the % of subclinical TB among prevalent cases (Figure 3A) and variation in the duration of subclinical TB (see. Ku C–C et al. 2021, citation #32 of this paper), the country–level heterogeneity of the % transmission from subclinical cases should be acknowledged when making inference on the global estimate, accounting for the burden of disease in each geography. The current global estimate was based on the pooled parameter values from 14 countries (not including major countries like India and China) without formal consideration of the burden of disease. I, therefore, found the claim of global estimate a bit too strong.
Despite all these limitations, the present research is considered novel and will contribute to the field of TB epidemiology. It will generate more discussion (and debate) on the importance of detecting and treating subclinical TB. Better data and further refinement of the analytic framework will generate more precise information which will prove to be useful for the revision of TB control strategies.
Author response
Essential revisions:
1. The authors should run a sensitivity analysis on:
– The assumption that smear and symptom status are constant over time since this is almost certainly not the case. This could be accomplished, for example, by using the model described in sensitivity analysis #2 – by assigning estimates of per–unit–time relative infectiousness to each state in that model and then calibrating those estimates to the cumulative hazard ratios that are estimated in this study.
The cumulative hazard approach we have used in this work was chosen to suit the available data and corresponding study designs. We agree that the assumption of a single disease phase (e.g. just subclinical, smear–negative) is a limitation of this approach and acknowledge this in our discussion (see Page 18, Line 11).
We appreciate the suggestion of changing the approach to a more dynamic model. This would ultimately change the methodology of the paper however and, in the absence of additional data with which to fit the more complex model, would rely on additional assumptions. While an interesting approach, we prefer to retain the current balance between data, assumptions and model complexity.
See Page 18, Line 11 for an expanded discussion of this limitation:
“An important limitation of our cumulative hazard model is the assumption that index cases only ever had the disease type they were diagnosed with during screening (e.g. sputum smear–positive, subclinical). Instead, it is more likely that individuals will fluctuate between being, for example, subclinical and clinical [31]. The impact such additional dynamics would have on our results remains uncertain, since they would depend on the detailed model of tuberculosis natural history assumed. Such a model would require additional data to prevent the need for additional assumptions.”
– The assumption that the background hazard of infection is the same across all households in a given survey/study. For example, do results change much if households with an index case have a 50% higher background hazard of infection?
Thank you for the suggestion, we have included this as ‘Sensitivity analysis 3’.
See Page 10, Line 29 for a description of the methods:
“Sensitivity analysis 3: To explore the impact of a differential background risk of infection amongst households with and without index cases, the analysis was repeated assuming a 50% increase in the background risk of infection for those households with an index case.”
See Page 15, Line 31 for a description of the results:
Sensitivity analysis 3: The above analysis was repeated assuming that households with an index case have a 50% greater background risk of infection than households with no index case. Affected results are shown in Supplementary Figure 8. The infectiousness of subclinical TB per unit time relative to clinical TB increased to 2.44 (0.60–10.06, 95% PrI), with corresponding values of 74% (29–95%, 95% PrI) of global transmission from subclinical TB, ranging from 53% (20–85%, 95% PrI) in Nigeria to 88% (61–97%, 95% PrI) in Mongolia.
See Page 17 in the Supplementary Materials for plots of the results.
– The durations of smear–positive and smear–negative TB are the same. For example, do results change much if smear–positive TB lasts twice as long as smear–negative TB?
We have also run this sensitivity analysis as ‘Sensitivity analysis 4’.
Please see Page 11, Line 1 for a description of the methods:
“Sensitivity analysis 4: Instead of assuming equal durations for sputum smear–positive and smear–negative TB, the above analysis was repeated assuming that sputum smear–positive TB has twice the duration of smear–negative TB.”
And page 16, Line 6 for a description of the results:
“Sensitivity analysis 4: The above analysis was repeated assuming sputum smear–positive TB has twice the duration of smear–negative TB. Affected results are shown in Supplementary Figure 9. The infectiousness of subclinical TB per unit time relative to clinical TB was largely unchanged at 1.94 (0.63–6.16, 95% PrI), with corresponding values of 70% (29–93%, 95% PrI) of global transmission from subclinical TB, ranging from 49% (21–79%, 95% PrI) in Nigeria to 86% (63–96%, 95% PrI) in Mongolia.”
See Page 18 in the Supplementary Materials for plots of the results.
2. Did the authors consider fitting a single cumulative hazard model for all 5 countries, that would allow only Λ_B (background cumulative hazard) to vary by country, rather than fitting 5 separate models and then pooling the estimates for the other lambdas after fitting? Does the former approach allow for estimation of the other λ's with more precision – unless the authors have reason to believe the λ's will vary by setting?
An interesting suggestion, which we have included as ‘Sensitivity analysis 5’.
Methods are described on Page 11, Line 5:
“Sensitivity analysis 5: In the main analysis each study was modelled separately, with the results combined using meta–analyses. As a sensitivity we model all studies simultaneously, assuming local background risks of infection for each study and global values across all studies for the remaining cumulative hazards.”
And see Page 16, Line 14 for a description of the results:
“Sensitivity analysis 5: The above analysis was repeated with all studies modelled simultaneously, assuming local background risks of infection for each study and global values across all studies for the remaining cumulative hazards. Affected results are shown in Supplementary Figure 10. The infectiousness of subclinical TB per unit time relative to clinical TB decreased to 1.39 (0.50–4.02, 95% PrI), with corresponding values of 58% (20–88%, 95% PrI) of global transmission from subclinical TB, ranging from 34% (15–62%, 95% PrI) in Nigeria to 76% (52–91%, 95% PrI) in Mongolia.”
See Page 19 in the Supplementary Materials for plots of the results.
We have also added some comments on all of the sensitivities in the discussion (see Page 16, Line 33):
“Our results were relatively robust to the sensitivities that were performed. In two cases, that is the removal of two studies (sensitivity analysis 1) and the use of a single model to account for all studies (sensitivity analysis 5), our estimates for the relative infectiousness of subclinical TB relative to clinical TB and the proportion of transmission from subclinical TB were lower than in the primary analysis. Our qualitative results and conclusions remain unchanged however. All other sensitivities resulted in higher estimates.”
3. How were households with multiple TB cases treated in the analysis? If they were excluded, what effect is this likely to have on the analysis? This should be described somewhere (at least in the supplement) and the likely impact on results discussed.
Households with multiple index cases were excluded from the analysis, as the premise of the household contact approach is to ascribe the incremental infections to the household index case. This assumption becomes difficult to interpret if there are multiple household index cases (e.g. in terms of unknown differences in disease onset), resulting in an inability to ‘divide’ the incremental infections in the household between the index cases. Excluding these households results in a slight loss of power (co–prevalent household index cases were below 10% across studies), but we feel this is worth the resulting clarity of the association.
We have added the following in the text (Page 3, Line 4 of the Supplementary Materials):
“Households with multiple co–prevalent index cases were excluded from the analysis, to retain the premise of the analysis which links infections above the community level to the characteristics of the single index case. Co–prevalent cases were absent in one study (ACT3 [5]) and below 10% in other studies, limiting the impact on power or introduction of bias.”
4. Given how much the Philippines 1997 prevalence survey seems to be driving results (in terms of the larger sample size of household contacts), it would be useful to show how the percent of transmission results in Figure 3 look when applied to the Philippines 1997 survey data. Currently only one of the four surveys/studies used to derive the relative infectiousness estimates (Vietnam 2007) is used in the percent of transmission estimates.
Thank you for the suggestion. Whilst we agree that this would be an interesting addition, given the number of surveys used and their range of proportions subclinical and sputum smear–positive (see Figure 3), the impact on the proportion of global transmission from subclinical TB is likely to be marginal, leaving our qualitative results and conclusions unchanged. We have instead focused on the three additional sensitivity analyses detailed above.
Other issues that should be addressed:
1. The finding that subclinical TB is likely to be more transmissible than symptomatic TB is counterintuitive and warrants much more discussion.
We agree that subclinical TB is unlikely to be more transmissible than clinical TB and have added further text to the discussion to address this point.
See Page 17, Line 21:
“Indeed, the paucity of the data provides an estimate that is consistent with subclinical TB being more infectious than clinical TB. Whilst we consider this to be implausible, we have avoided introducing priors that rule out this possibility. Instead we would emphasise that our results reflect the uncertainty of the data. The lower bound of our estimate precludes subclinical TB being significantly less infectious than clinical TB, while there is no evidence against subclinical TB being as infectious as clinical TB.”
2. It may be useful to clarify to readers how Mtb infection differs from subclinical TB (or TB more generally).
Thank you. We have added a line in the introduction to this effect.
See Page 4, Line: 31:
“We distinguish both disease states from Mtb infection, whereby individuals may test positive on a Tuberculin Skin Test (TST) or Interferon–Γ Release Assay (IGRA) but do not have bacteriologically–confirmed disease.”
3. Figure 1 presents the odds ratios for infection of smear–positive vs. smear–negative (on the left) and clinical vs. subclinical TB (on the right). Figure 2 presents relative per–unit–time infectiousness estimates in the exact opposite way (smear–negative vs. smear–positive and subclinical vs. clinical, and in the opposite order). It would be clearer for readers if the presentation was more consistent across figures (and accompanying text).
Thank you for your comment. We agree this was inconsistent. Figure 1 has been altered accordingly to show subclinical vs. clinical on the left and sputum smear–negative vs. smear–positive on the right (please see Page 12).
4. Presenting figure 1 in terms of the relative cumulative hazard rather than (or in addition to) odds ratios would also help with the continuity between figures 1 and 2 (since the estimates of per–unit–time relative infectiousness used the relative cumulative hazards, not odds ratios).
Whilst we agree that presenting cumulative hazards would be closer to the modelling framework, we chose odds ratios to complement the modelling results, since they are more intuitive for a wide range of potential readers. We feel this helps to broaden the readership and understanding of the work and would therefore prefer to keep this metric.
5. Methods text should contain a bit more information on how the estimated durations of subclinical and clinical TB were used to convert the infection odds ratios into per–unit–time relative infectiousness estimates – this is clear in the appendix but not in the main text.
Further details have been added to the methods.
See Page 8, Line 10:
“To infer the infectiousness of subclinical TB per unit time relative to clinical TB from our posterior estimate for the subclinical relative cumulative hazard rs we note that, assuming constant hazards, the relative cumulative hazards from index cases will depend on the product of the relative per unit time infectiousness and relative durations of infectiousness. We assume that per unit time infectiousness depends on symptom status and sputum smear–status, whilst durations of infectiousness depends on symptom status only. It follows then that:
Finally, we sampled from the posterior estimate for the subclinical relative cumulative hazard and an assumed duration of disease for subclinical index cases relative to clinical index cases, providing a median and 95% equal tailed posterior estimate for the relative infectiousness of subclinical index cases relative to clinical index cases for each study separately. Finally, we provide a summary estimate by mixed–effects meta–analysing the individual estimates across the separate studies. Analogous results are presented for the relative infectiousness per unit time of sputum smear–negative TB relative to smear–positive TB.”
6. In the abstract, the term "individual population data" is a bit unclear.
This has been edited.
See Page 2, Line 28:
“We collated individual–level data on representative populations for analysis…”
7. Supplementary figure 2 may be mislabeled – for Bangladesh, it seemed that only smear–positive cases were included but the graph makes it look like only subclinical cases were included. Are the other panels similarly mislabeled?
Thank you for the comment – this was indeed mislabelled and has been edited. All other panels are correct.
See Page 11 of the Supplementary Materials.
8. p5 (line 7–9) Sentence could be made clearer. What does it mean there is a "disconnect"? Is this a pathological link? or causality? lack of evidence?
This has been edited.
See Page 5, Line 9:
“Indeed, whilst recent empirical studies have suggested that tidal breathing may contribute significantly to Mtb transmission [27], exhalation of infectious aerosols appears unrelated to the presence of symptoms [28] or cough frequency [29] in TB patients.”
9. Results: The estimations of per unit time infectiousness of subclinical TB should be brought up front in the results, before the meta–analysis.
We agree that the results for the per unit time infectiousness of subclinical TB could be made more prominent. However, moving Figure 2 (estimates of per unit time infectiousness) above Figure 1 (odds ratios of the underlying data) would be inconsistent with the order of the methods. We instead have edited the text by introducing subsections ‘Data’ and ‘Estimating the relative infectiousness of subclinical TB’ to make the results more easily identifiable whilst preserving the order of the methods.
See Page 11, Line 12 for the first new subsection:
“Data”.
See Page 12, Line 10 for the second new subsection:
‘Estimating the relative infectiousness of subclinical TB’.
10. The discussion around the limitations of household contact and transmission assessment is very limited. This study inherits the limitations of the source studies, a more robust discussion around this issue would be beneficial.
We have added additional text to the discussion of this limitation to acknowledge that our work necessarily inherits all such limitations. Given other additions to the discussion as a result of peer review, we feel any further comment would make the Discussion section prohibitively long.
See Page 18, Line 4:
“Such household contact studies are therefore liable to biases and our study necessarily inherits such limitations. For example…”
11. Sensitivity of symptom screening algorithms is not discussed: TB disease, although split it into two categories is more likely a continuum.
A paragraph has been added to the discussion to this effect.
See Page 18, Line 26:
“We defined subclinical and clinical TB as being culture and/or NAAT positive and responding negatively or positively to an initial symptom screen, respectively. In practice subclinical and clinical TB are part of a continuous spectrum and alternative definitions could be defined according to different criteria. Here we have used the definition most closely aligned with the methodology of the majority of prevalence surveys, which is consistent with other studies of subclinical TB [18] and pragmatic for inclusion of future surveys.”
12. Discussion page 15 (L11–L12): Is it relevant to mention SARS–CoV and Malaria here? Bringing asymptomatic transmission as a transversal subject across diseases without providing more epidemiological insights seems gratuitous. This should be removed.
We agree that whilst evidence for asymptomatic transmission in SARS–CoV–2 and Malaria does not provide evidence for such transmission in TB, we mention them in the discussion to make the broader point that asymptomatic transmission would not be unique to TB. We have edited the text to clarify this point.
See Page 17, Line 17:
“More broadly, recent work on SARS–CoV–2 and malaria have similarly shown how ‘asymptomatic’ or ‘subpatent’ infections can be important drivers of transmission [45–47], meaning a role for asymptomatic transmission would not be unique to TB.”
13. The implications on policy and directly on recommendations around symptom screening in WHO plans are not commented on enough, this is where these results could be more useful.
We have added a reference to the WHO screening guidelines [1] in the discussion (see Page 19, Line 31):
“As our results show that subclinical TB likely contributes substantially to transmission, an increased emphasis on symptom agnostic screening in, for example, the TB screening guidelines [58] should be considered, as should the inclusion of subclinical TB in the planned update of WHO case definitions. Target Product Profiles for diagnostic tools should consider all infectious TB, regardless of whether individuals are experiencing or aware of symptoms, and interventions using such tools should be critically evaluated for their impact on Mtb transmission and cost–effectiveness.”
14. page 16, line 32: The sentence "This is in keeping with increasing data showing that symptoms, in particular the classic TB symptom of cough, are not closely correlated to the amount of Mtb exhaled [28,29] " This is not completely consistent with Ref [28] by Theron et al. Nature Med 2020. The authors actually found that cough alone was a strong indicator for the measured transmissibility supplementary table 8. OR for CASS positivity for cough: 3.38 (1.90–5.99) despite the overall symptom score being negatively associated with transmissibility.
We have removed the reference to Theron et al. Nature Med 2020 (Ref [28] in the above).
See Page 19, Line 19:
“This is in keeping with increasing data showing that symptoms, in particular the classic TB symptom of cough, are not closely correlated to the amount of Mtb exhaled [29]…”
15. Supplementary Table 1: would be useful to add information on the definition of symptoms used to define subclinical against clinical disease.
These have been added to Supplementary Table 1.
See Page 4 in the Supplementary Materials.
16. Is there evidence from the literature regarding the subclinical transmission using results from whole genome sequencing? For example, Xu et al. (plos medicine) found in a study from Spain that 5/14 (35.7%) cases likely transmitted TB well before symptom onset. This can be discussed.
We have added this study [2] to the discussion.
See Page 17, Line 13:
“Our results are also in keeping with recent results from whole genome sequencing [45], in which 36% of individuals likely transmitted Mtb before symptom onset, assuming a linear SNP mutation rate.”
We have also added another study to the discussion that was published whilst this paper was under review [3].
See Page 17, Line 7:
“Using data from the 2007 Viet Nam prevalence survey however, [43] find that among children aged 6–10 years, those living with clinical, smear–positive tuberculosis patients, and those living with subclinical, smear–positive tuberculosis patients, had similarly increased risks of TST positivity compared with those living without tuberculosis patients.”
References
[1] World Health Organization. WHO consolidated guidelines on tuberculosis – Module 2: Systematic screening for tuberculosis disease. Genève: World Health Organisation 2021. https://apps.who.int/iris/handle/10665/341426 (accessed 9 Sep 2021).
[2] Xu Y, Cancino–Muñoz I, Torres–Puente M, et al. High–resolution mapping of tuberculosis transmission: Whole genome sequencing and phylogenetic modelling of a cohort from Valencia Region, Spain. PLoS Med 2019;16:e1002961. 10.1371/journal.pmed.1002961
[3] Nguyen HV, Tiemersma E, Nguyen NV, et al. Disease Transmission by Patients With Subclinical Tuberculosis. Clin Infect Dis 2023;76:2000–6. 10.1093/cid/ciad027
Articles from eLife are provided here courtesy of eLife Sciences Publications, Ltd
Citations & impact
Impact metrics
Citations of article over time
Alternative metrics

Discover the attention surrounding your research
https://www.altmetric.com/details/157695012
Article citations
Estimating the Impact of Tuberculosis Pathways on Transmission-What Is the Gap Left by Passive Case Finding?
J Infect Dis, 230(5):e1158-e1161, 01 Nov 2024
Cited by: 1 article | PMID: 39106422 | PMCID: PMC11566222
Community-Wide Active Case Finding for Tuberculosis: Time to Use the Evidence We Have.
Trop Med Infect Dis, 9(9):214, 14 Sep 2024
Cited by: 0 articles | PMID: 39330903 | PMCID: PMC11436250
Review Free full text in Europe PMC
Implications of subclinical tuberculosis for vaccine trial design and global effect.
Lancet Microbe, 5(10):100895, 01 Jul 2024
Cited by: 1 article | PMID: 38964359 | PMCID: PMC11464400
Review Free full text in Europe PMC
One Half of the Pair: Prioritizing Tuberculosis Transmitters for Early Detection.
Am J Respir Crit Care Med, 210(2):143-144, 01 Jul 2024
Cited by: 0 articles | PMID: 38687502 | PMCID: PMC11273311
Evaluating the diagnostic accuracy of QIAreach QuantiFERON-TB compared to QuantiFERON-TB Gold Plus for tuberculosis: a systematic review and meta-analysis.
Sci Rep, 14(1):14455, 24 Jun 2024
Cited by: 0 articles | PMID: 38914731 | PMCID: PMC11196697
Review Free full text in Europe PMC
Go to all (19) article citations
Similar Articles
To arrive at the top five similar articles we use a word-weighted algorithm to compare words from the Title and Abstract of each citation.
The impact of HIV on infectiousness of pulmonary tuberculosis: a community study in Zambia.
AIDS, 7(7):981-987, 01 Jul 1993
Cited by: 52 articles | PMID: 8357557
Tuberculosis
The International Bank for Reconstruction and Development / The World Bank, Washington (DC), 14 Sep 2018
Cited by: 0 articles | PMID: 30212088
ReviewBooks & documents Free full text in Europe PMC
Prevalence of subclinical pulmonary tuberculosis in adults in community settings: an individual participant data meta-analysis.
Lancet Infect Dis, 24(7):726-736, 12 Mar 2024
Cited by: 2 articles | PMID: 38490237
Folic acid supplementation and malaria susceptibility and severity among people taking antifolate antimalarial drugs in endemic areas.
Cochrane Database Syst Rev, 2(2022), 01 Feb 2022
Cited by: 12 articles | PMID: 36321557 | PMCID: PMC8805585
Review Free full text in Europe PMC
Funding
Funders who supported this work.
Bill & Melinda Gates Foundation (3)
Grant ID: OPP1135288
Grant ID: INV-001754
Grant ID: OPP1084276
Bill & Melinda Gates Foundation (3)
Grant ID: INV-001754
Grant ID: OPP1135288
Grant ID: OPP1084276
Economic and Social Research Council (1)
Grant ID: ES/P008011/1
European & Developing Countries Clinical Trials Partnership (EDCTP) (1)
Grant ID: RIA208D-2505B
European Research Council (2)
Grant ID: ERC Starting Grant No. 757699
Grant ID: 757699
Foreign, Commonwealth and Development Office
Medical Research Council (2)
A mathematical modeling framework for tuberculosis burden estimation and economic evaluation of pharmaceutical interventions
Professor Peter Dodd, University of Sheffield
Grant ID: MR/P022081/1
Grant ID: CCF17-7779
NIAID NIH HHS (1)
Grant ID: R01 AI147321
NIH HHS (1)
Grant ID: 1R01AI147321-01
National Institutes of Health (1)
Grant ID: 1R01AI147321-01
UK Government
Wellcome Trust (1)
Grant ID: 218261/Z/19/Z
World Health Organization (2)
WHO generic grant number for open-access policy
World Organization
Grant ID: 001
Grant ID: 2020/985800-0