Abstract
Free full text

A comprehensive analysis of the prognostic value and immune microenvironment of lysosome-dependent cell death in glioma: Including glioblastoma and low-grade glioma
Abstract
Lysosome-dependent cell death (LCD) plays a significant role in overcoming cancer apoptosis and drug resistance. However, the relationship between LCD-associated genes (LCDGs) and glioma, including glioblastoma (GBM) and low-grade glioma (LGG), remains unclear. In this study, an LCDGs risk signature was constructed for glioma patients by utilizing 4 algorithms (Extreme Gradient Boosting, Support Vector Machine, Random Forest, and Generalized Linear Models) to identify core LCDGs. Their correlation with clinical features and the immune microenvironment was also determined in glioma, GBM, and LGG. Additionally, the role of hub LCDGs in various cancers was elucidated via pan-cancer analyses. Validation of the core gene in glioma was performed using qRT-qPCR and immunofluorescence staining analysis. The results showed that the LCDGs risk signature was strongly associated with the prognosis, cancer grades, histological types, and primary therapy outcomes of glioma patients. Furthermore, it was closely linked to the overall survival of LGG patients. Mechanistic analyses revealed a significant association between the risk signature and the immune microenvironment in glioma. Based on differential expression analysis, receiver operating characteristic analysis, and interacted model algorithms, LAPTM4A was identified as a hub LCDG in glioma. It exhibited significant upregulation in glioma, GBM, and LGG samples. Moreover, LAPTM4A expression correlated with the prognosis of glioma and LGG patients, as well as age, grades, histological types, and primary therapy outcomes in glioma. Pan-cancer analysis confirmed that LAPTM4A expression was modulated in the majority of cancers and was associated with the prognosis of various cancers. Mechanistic analyses suggested a strong relationship between LAPTM4A and immune cell infiltration, as well as several drug sensitivities. In conclusion, our findings suggest that LAPTM4A may serve as a potential oncogene associated with LCD in pan-cancer, particularly in glioma, GBM, and LGG. These findings provide important insights for individualized treatment of glioma.
1. Introduction
Glioma, the most prevalent primary malignant brain tumor, accounts for 81% of malignancies in the central nervous system.[1] It is a heterogeneous neoplasm that ranges from surgically curable low-grade glioma (LGG) to glioblastoma (GBM).[2] GBM constitutes over 50% of all gliomas and is considered the most invasive and virtually incurable type, with a 5-year survival probability of <3%.[3] Despite significant progress in surgical operation treatment, chemotherapy, and radiotherapy, the overall survival (OS) of patients with glioma remains very unfavorable. Immunotherapy offers promise as a treatment for malignant cancers.[4] However, due to the presence of an immunosuppressive tumor microenvironment, glioma patients are usually resistant to immune checkpoint inhibitors, ultimately leading to a poor prognosis.[5,6] Therefore, urgently needed are the identification and screening of novel treatment approaches and immunotherapy molecular biomarkers, as well as the construction of prognostic signatures capable of accurately predicting a patient’s condition.
Lysosomes, acidic compartments containing various hydrolytic enzymes and an acidic environment, are important membrane-bound cellular organelles that play a vital role in substrate degradation during the terminal step of autophagy.[7] In the process of cancer development, tumor cells increasingly rely on enhanced lysosomal function to obtain nutrients, activating the lysosome-dependent pathway. Recent studies have also demonstrated significant connections between lysosomes and cancer metabolism, angiogenesis, and immune escape.[8] Lysosome-dependent cell death (LCD), a regulated form of cell death, is primarily characterized by lysosomal membrane permeabilization.[9] Given cancer cells’ dependence on lysosomal function, LCD has emerged as a promising therapeutic target for treating cancers.[10,11] Various lysosome-targeted agents used alone or in combination have shown significant antitumor effects and effectively reduced cancer cell resistance to radiotherapy and chemotherapy.[11–13] Additionally, lysosome-targeted therapies can counteract the compensatory effects of autophagy in cancers.[14] Nonetheless, the precise mechanisms responsible for the potential role of LCD in tumor modulation remain unclear, and the association between LCD and glioma has yet to be explored.
In this study, we screened a risk signature of LCD-associated genes (LCDGs) significantly associated with glioma and LGG patients’ prognosis and immune microenvironment. By employing comprehensive bioinformatic methods, including Extreme Gradient Boosting, Support Vector Machine, Random Forest, Generalized Linear Models, receiver operating characteristic (ROC), as well as differential expression analysis, we determined that LAPTM4A is a hub LCDG in glioma. Furthermore, we validated the clinical significance and prognostic role of the LAPTM4A gene using patients with glioma, GBM, and LGG. Additionally, we conducted pan-cancer analysis to investigate the connections between LAPTM4A and various cancer types. In summary, we have first identified the role of LCDGs in glioma, presenting a promising target for predicting prognosis and clinical outcomes of glioma, GBM, and LGG in this study.
2. Materials and methods
2.1. Acquisition of raw data
RNA sequencing datasets of 704 glioma patients, including 170 GBM samples and 534 LGG samples, were collected from The Cancer Genome Atlas (TCGA, https://portal.gdc.cancer.gov/) database. Additionally, due to the absence of normal controls in the TCGA database, 1152 normal brain tissues were obtained from the Genotype-Tissue Expression (GTEx, http://commonfund.nih.gov/GTEx/) database. For external validation, additional glioma samples were collected from the GSE4290 (23 normal, 76 LGG, and 77 GBM samples) and GSE22866 (6 normal and 40 GBM samples) GEO microarray datasets (https://www.ncbi.nlm.nih.gov/geo/). To perform pan-cancer analysis, RNA-seq expression data from 33 different types of tumors were obtained from the TCGA database, along with CNV data. The identification of LCDGs was based on prior research,[15] and a total of 220 LCDGs were identified through screening. The search of all public databases in this study adhered to relevant guidelines. Since no human or animal subjects were involved, ethical approval was not required.
2.2. Construction of the LCDGs risk signature
To identify the hub LCDGs, differential expression analysis, univariate Cox regression analysis, weighted gene co-expression network analysis (WGCNA), and least absolute shrinkage and selection operator (LASSO) analysis were performed on TCGA-glioma samples. The univariate Cox regression analysis utilized a cutoff criterion of P < .001. Differential expression analysis was conducted using the R package “limma,” with criteria of |log fold change (FC)| > 1 and P < .05. The “WGCNA” package was employed to establish a co-expression network linking LCDGs with sample modules, determining the best soft threshold based on the scale-free topological criterion (>0.86) and a minimum power value. Hierarchical clustering was then used to construct dendrograms based on the topological overlap matrix (TOM). The number of generated modules was controlled by defining main parameters with a minModuleSize of 10. LCDGs displaying significant interconnections were assigned to various patterns. Gene significance and module eigengenes were calculated for all modules. The “VennDiagram” package facilitated visualization of the overlap of LCDGs among WGCNA, differential expression, and univariate Cox regression analyses. These genes were further integrated into the LASSO analysis to identify the hub LCDGs in glioma for further investigation.
LCDGs risk signatures were generated based on the expression of 14 identified hub LCDGs. The risk score for the signatures was calculated using the formula: risk score = Σexpi × βi (expi represents the relative expression of LCDGs i, and β is the regression coefficient). Glioma patients were then divided into low- and high-risk subgroups based on the median value of the calculated risk score. Cox regression and Kaplan–Meier (KM) survival analyses were conducted to assess and compare the prognostic ability using the “survival” package. The accuracy of the risk signature for prediction was calculated using the “timeROC” and “cliROC” packages. Finally, we utilized the “rms” package to construct a nomogram that incorporated the risk scores of the gene signature for predicting the outcomes of glioma patients.
2.3. Immune infiltration analysis
Firstly, CIBERSORT analysis was conducted to assess immune cell infiltration in glioma and normal samples. Additionally, the relationship between the expression of hub LCDGs and immune cell infiltration was also assessed by CIBERSORT analysis. Subsequently, single-sample gene set enrichment analysis (GSEA) was performed to explore discernible differences in immune cell infiltration and immune functions among different risk subgroups of glioma. The relationship between the scores of immune-associated factors (including stromal and immune scores) and the risk signature was evaluated through Spearman correlation analysis. The connection between the risk signature and immune-associated genes was also investigated by referencing previously identified potential immune checkpoints.[16]
2.4. Machine learning model construction
The LCDGs identified from LASSO analysis were used to construct 4 different machine learning models: Extreme Gradient Boosting, Support Vector Machine, Random Forest, and Generalized Linear Models. These models were constructed using R packages such as “xgboost,” “randomForest,” “dalex,” and “caret,” respectively. Residual analysis was applied to the samples to evaluate the accuracy of these models, followed by plotting the reverse cumulative distribution of residuals. Receiver operating characteristic (ROC) curves for the 4 machine learning models were plotted using the “pROC” R package. Each LCDG in the models was assigned an importance score to filter out the key genes for glioma patients. Additionally, differential expression analysis and ROC analysis were conducted for each LCDG in glioma. Based on the results of the machine learning models, differential expression analysis, and ROC curves, the best feature among the LCDGs was selected for further analyses.
2.5. Clinical value of LAPTM4A in glioma, GBM, and LGG
Differential expression analysis was performed to evaluate the expression level of the LAPTM4A gene in glioma, GBM, and LGG samples. To compare the overall survival (OS) of LAPTM4A, KM survival analysis, and Cox regression analysis were conducted. The predictive accuracy of LAPTM4A was verified using the R package “timeROC.” A nomogram was constructed to predict the OS of glioma patients based on the expression level of LAPTM4A and other clinical features, using the “rms” package in R. Furthermore, GSEA and GSVA analyses were carried out to investigate the potential mechanism of LAPTM4A. GSEA was performed on the gene expression matrix using the “c2.cp.kegg.symbols.gmt” sets to detect Kyoto Encyclopedia of Genes and Genomes pathways. The potential biological functions of LAPTM4A were evaluated using the GSVA score matrix and the “GSVA” R package. Statistical significance was defined as P values < .05.
2.6. Pan-cancer analysis of LAPTM4A
RNA sequencing data from the TCGA database, comprising 33 distinct types of cancers, were utilized to visualize the differential gene expression of LAPTM4A between normal and cancerous tissues. KM analysis was employed to evaluate the prognostic value of LAPTM4A in different cancer types.
2.7. Chemotherapy sensitivity analysis
Chemotherapy drugs certified by the FDA or verified by clinical laboratory were obtained from the CellMiner database (https://discover.nci.nih.gov/cellminer). Pearson correlation analyses were conducted to assess the sensitivity of LAPTM4A to these drugs. The results were visualized using R packages such as “ggplot2,” “limma,” “ggpubr,” and “impute.”
2.8. Cell culture
The glioma cell line U373 and the normal control NHA cells were purchased from BNCC. They were cultured in Dulbecco Modified Eagle’s medium (Gibco, Thermo Fisher Scientific, Waltham, MA) supplemented with 10% fetal bovine serum (Hyclone, Logan, UT) and 1% penicillin/streptomycin (Solarbio, Beijing, China). The cells were maintained at 37°C in a 5% CO2 incubator.
2.9. Quantitative real-time PCR (qRT-PCR)
RNA isolation was performed using Trizol reagent (Invitrogen, CA), followed by cDNA synthesis using a Transcriptor First-strand cDNA synthesis kit (Roche, Basel, Switzerland). Subsequently, qRT-PCR was conducted using SYBR green master mix. Each sample was analyzed in triplicate, and gene expression was quantified using the 2−ΔΔCT method. The primer sequences are listed in Supplementary Table 1, http://links.lww.com/MD/L392.
2.10. Immunofluorescence analysis
Cells on plate slides were fixed with 4% paraformaldehyde for 15 minutes and permeabilized with 0.5% Triton X for 15 minutes at room temperature. Next, the cells were incubated with 1% BSA to block nonspecific binding sites of antibodies. After overnight incubation at 4°C with the primary anti-LAPTM4A antibody (1:50; Invitrogen, CA), the cells were incubated with secondary antibodies for 1 hour. Finally, DAPI solution was used to stain the nuclei, and immunofluorescent images were observed under a fluorescence microscope (Olympus, Japan).
3. Results
3.1. Risk signature of LCDGs for glioma patients
Through univariate Cox regression and differential expression analyses, we identified 145 prognosis-associated LCDGs (Supplementary Table 2, http://links.lww.com/MD/L390) and 171 differentially expressed LCDGs (Supplementary Table 3, http://links.lww.com/MD/L392). To gain deeper insights into the functional clusters associated with glioma patients, we extended the WGCNA analysis to include the LCDGs (Fig. (Fig.1A-B).1A-B). Amongst the various modules, the turquoise module showed the strongest association (R = 0.95) between the 2 clusters, leading us to choose LCDGs from this module for further analysis (Fig. (Fig.1C).1C). Based on these analyses, we selected 28 overlapping LCDGs as candidate genes (Fig. (Fig.1D).1D). Subsequently, LASSO analysis was employed to reduce the number of genes, resulting in the identification of 14 hub LCDGs: ACP2, AGA, AP3S2, BORCS6, CTNS, GATA2, GNS, HPS6, KIF1B, LAPTM4A, NCOA4, PSAP, SNAPIN, and SPAG9, which were further analyzed for their prognostic significance (Fig. (Fig.11E-F).
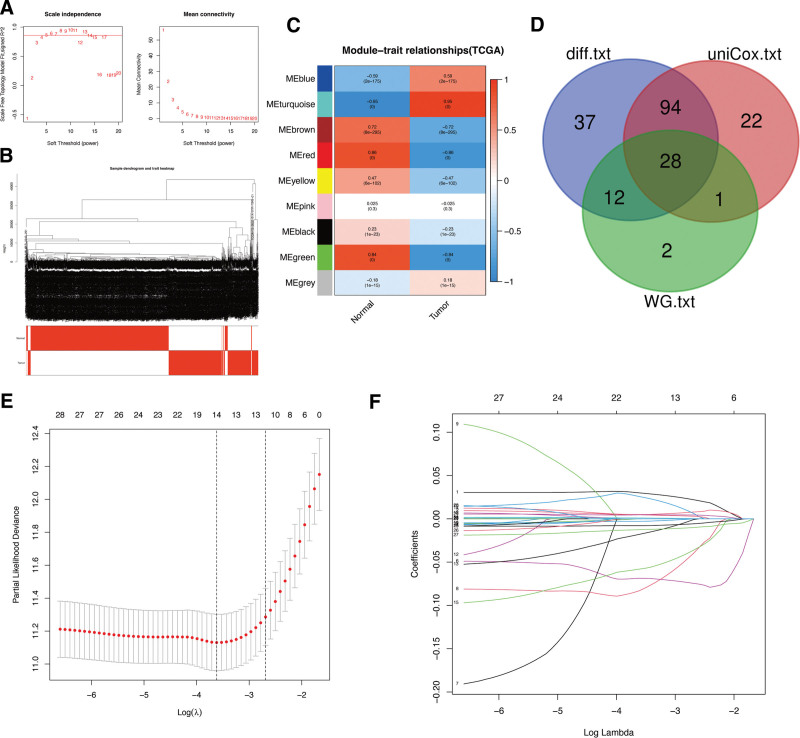
Identification of hub LCDGs in glioma. (A-B) Soft-thresholding powers scale-free fit index. (C) Heatmap showing the correlation between clinical traits and gene module. (D) The Venn diagram. (E-F) LASSO analysis to determine factors and construct the model.
Investigation of somatic copy number variations (CNVs) in the identified hub LCDGs in glioma revealed a higher prevalence of CNVs in the genes AP3S2, SNAPIN, SPAG9, GNS, NCOA4, and PSAP. Specifically, these genes exhibited increased CNV changes (Fig. (Fig.2A).2A). The alterations of CNVs across the respective chromosomes of the hub LCDGs are depicted in Figure Figure2B.2B. Notably, network visualization of the correlation between the hub LCDGs demonstrated significant associations among all the genes (Fig. (Fig.2C,2C, D). Furthermore, to explore the relationship of LCDGs with immune infiltration in glioma, Figure Figure2E2E indicated that all 14 identified LCDGs exhibited significant correlations with the proportions of immune cells, providing evidence for their potential as prognostic targets for glioma immunotherapy.
3.2. Clinical value of risk signature in glioma and LGG
Based on the calculated median value of risk scores, glioma patients from the TCGA database were classified into high- or low-risk subgroups (Fig. (Fig.3A).3A). Investigating the association between LCDGs and glioma risk subgroups revealed higher expression of genes AP3S2, BORCS6, GATA2, HPS6, KIF1B, NCOA4, PSAP, and SPAG9 in the high-risk subgroup, while ACP2, AGA, CTNS, GNS, LAPTM4A, and SNAPIN showed lower expression (Fig. (Fig.3B).3B). Furthermore, the risk signature of LCDGs independently predicted outcomes for glioma patients (Fig. (Fig.3C-D).3C-D). KM survival analysis demonstrated a negative correlation between risk scores and OS of glioma patients (Fig. (Fig.3E).3E). The risk signature exhibited high predictive accuracy with AUC values of 0.867, 0.875, 0.845, 0.867, and 0.875 at 1-year, 3-year, 5-year, 7-year, and 9-year OS follow-up, respectively (Fig. (Fig.3F).3F). Compared to other clinical features such as gender, age, and histological type, the risk signature showed higher predictive accuracy for the OS of glioma patients (Fig. (Fig.3G).3G). Notably, patients aged > 60 (Fig. (Fig.3H),3H), with G3 tumor grade (Fig. (Fig.3I),3I), GBM histological type (Fig. (Fig.3J),3J), or progressive disease (Fig. (Fig.3K)3K) exhibited significantly higher risk scores compared to patients aged ≤ 60, G2 tumor grade, LGG histological types, or primary therapies. Evaluation of the risk signature’s predictive role in LGG prognosis revealed a significant negative correlation between risk scores and OS of LGG patients (Fig. (Fig.3L).3L). The LCDGs risk signature exhibited moderate predictive accuracy at 1-year, 3-year, 5-year, and 7-year follow-up with AUC values all above 0.7 (Fig. (Fig.3M).3M). Additionally, LGG patients aged > 60 showed significantly higher risk scores compared to those aged ≤ 60 (Fig. (Fig.3N).3N). However, due to the separation of all GBM patients into the high-risk subgroup in our LCDGs risk signature, this model could not be applied to analyze the prognosis of GBM patients separately. Finally, we created a nomogram plot for glioma patients incorporating clinical characteristics and the risk score (Fig. (Fig.4A),4A), demonstrating substantial agreement (Fig. (Fig.44B).
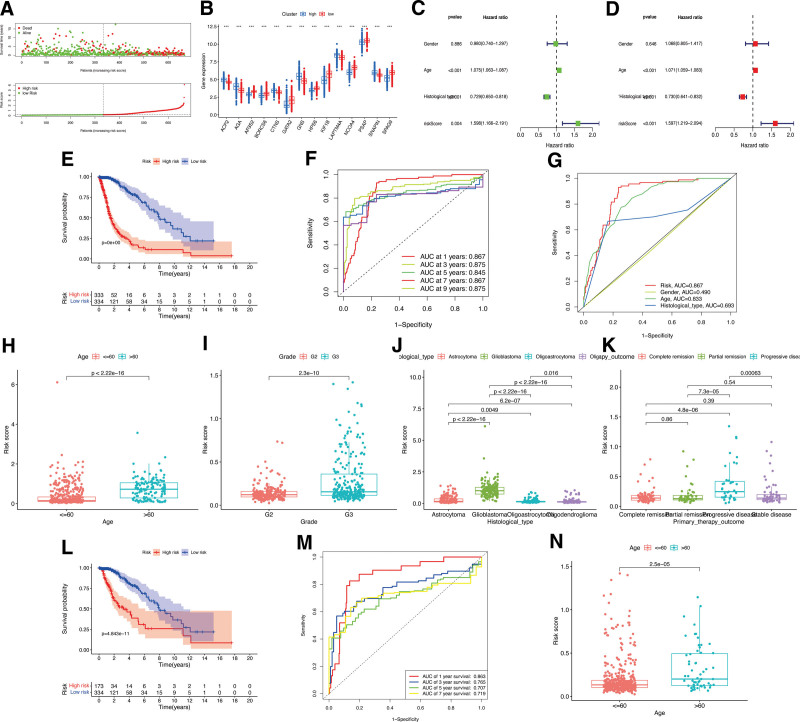
The Role of LCDGs risk signature. (A) The risk score distributions in the TCGA-glioma patients. (B) The differential expression of LCDGs in the risk subgroups of TCGA-glioma patients. (C-E) The survival status of risk signature in the TCGA-glioma patients. Survival (F) and clinical (G) ROC analysis of risk signature in the TCGA-glioma patients. Correlations between risk scores and ages (H), tumor grades (I), histological types (J), and primary therapy outcomes (K) in glioma patients. (L-M) The survival status of risk signature in the GSE4290-LGG patients. (N) Correlations between risk scores and ages in LGG patients.
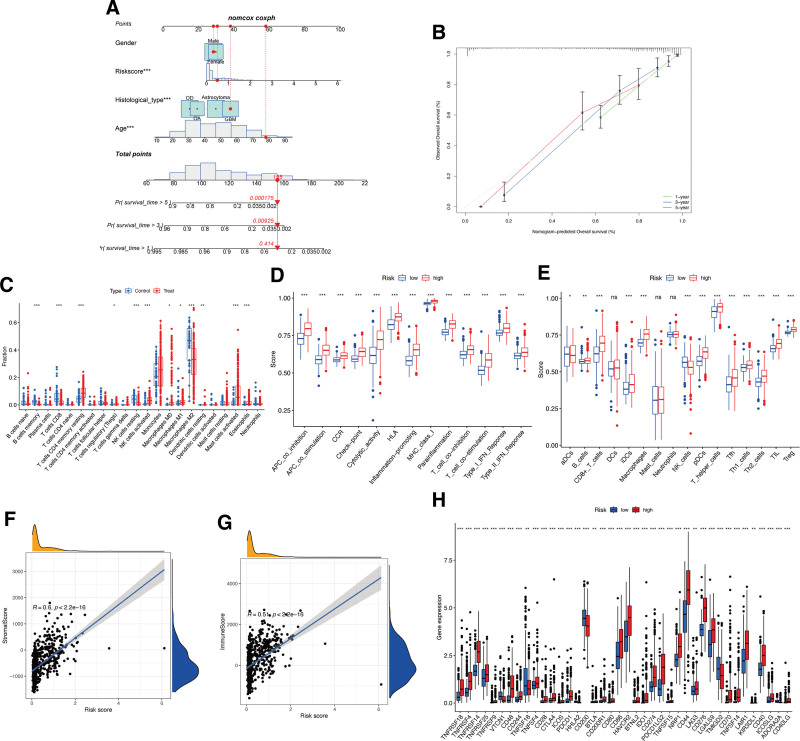
Associations between risk signature and immune characteristics. (A-B) Nomogram for predicting the OS of risk scores. (C) The proportion of immune cells between normal control and glioma samples. Boxplots of scores of immune-associated functions (D) and immune cells (E) in risk signature. Associations between risk signature, stromal scores (F) and immune scores (G). (H) Expression of immune checkpoints among 2 risk subgroups in glioma patients.
To investigate immune infiltration in glioma, a CIBERSORT analysis was conducted. Figure Figure4C4C shows significant differences in the infiltration levels of several immune cell types between glioma and control samples, including memory B cells, CD8 T cells, CD4 memory resting T cells, regulatory T cells, resting/activated NK cells, M0/M1/M2 macrophages, resting dendritic cells, activated mast cells, and eosinophils. Patients with high-risk scores exhibited significantly increased immune cell functions and subpopulations, except for aDCs, DCs, mast cells, neutrophils, and NK cells (Fig. (Fig.4D-E).4D-E). Moreover, the risk signature showed a positive correlation with stromal (Fig. (Fig.4F)4F) and immune (Fig. (Fig.4G)4G) scores, indicating its association with the immune microenvironment. Differential expression of various immune checkpoints was observed within the 2 risk subgroups. Patients with high-risk scores showed elevated expression of almost all immune biomarkers, such as TNFRSF18, TNFRSF4, and TNFRSF14, except for VTCN1, TNFSF18, HHLA2, CD200, TMIGD2, and ADORA2A (Fig. (Fig.44H).
3.3. Identification the core LCDG LAPTM4A in glioma, GBM, and LGG
The analyses of 4 different algorithms consistently demonstrated small residuals ranging from 0 to 0.1 for all 4 algorithms employed, as represented by box plots (Fig. (Fig.5A)5A) and reverse cumulative distribution (Fig. (Fig.5B).5B). The diagnostic models’ high accuracy was confirmed by the results of ROC curves, with all 4 algorithms exhibiting AUC values close to 1 (Fig. (Fig.5C).5C). Evaluating the importance scores of the 4 algorithms, LCDGs LAPTM4A and HPS6 demonstrated the highest score (Fig. (Fig.5D).5D). To further validate if the hub LCDGs significantly predict glioma occurrence, we conducted a disease-specific association ROC curve for 14 identified LCDGs. The AUC values for LAPTM4A and HPS6 were 1.000 and 0.999, respectively, indicating their specific predictive ability for glioma (Fig. (Fig.5E).5E). Through differential expression analysis, both HPS6 (Fig. (Fig.5F)5F) and LAPTM4A (Fig. (Fig.5G)5G) showed significant upregulation in TCGA-glioma patients. However, in the GSE4290 validation cohort, only LAPTM4A exhibited significant differential expression in glioma tissues (Fig. (Fig.5I),5I), whereas there was no significant value for gene HPS6 (Fig. (Fig.5H).5H). The significant upregulation of LAPTM4A gene and protein expression levels were also confirmed in U373 glioma cells through qRT-PCR (Fig. (Fig.5J)5J) and immunofluorescence analysis (Fig. (Fig.5K).5K). Consequently, LAPTM4A was identified as the core LCDG in glioma and employed for subsequent prognostic analysis.
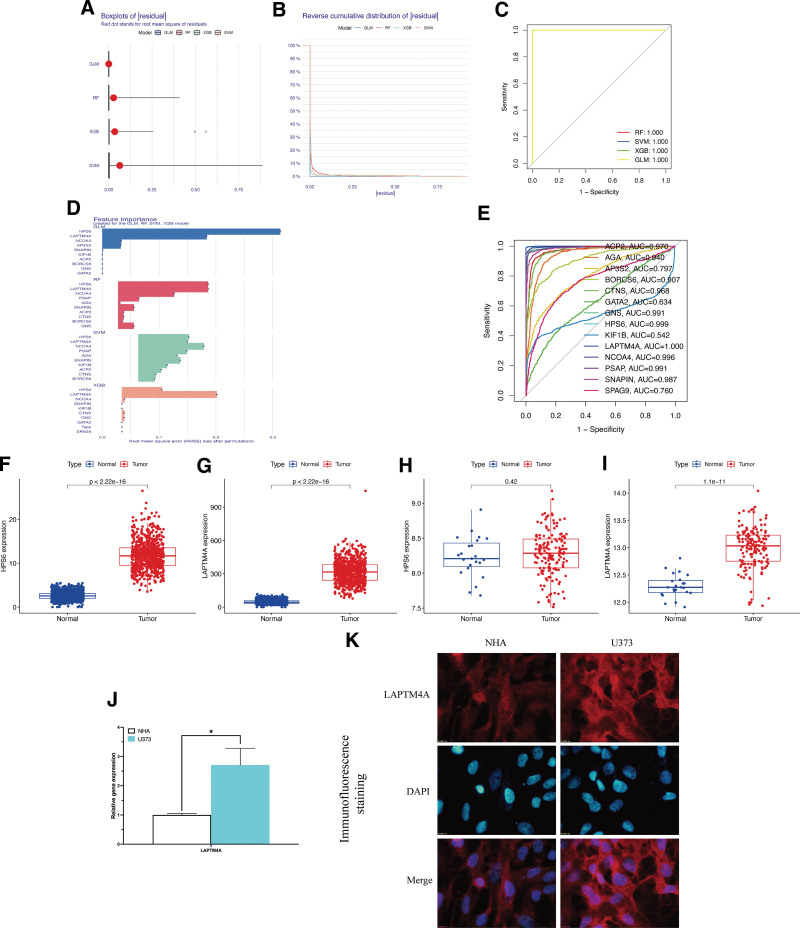
Identification of LAPTM4A in glioma. (A) Box plots of sample residuals of the 4 algorithms. (B) The reverse cumulative distribution map of model residuals. (C) ROC analysis of algorithms. (D) Importance score of feature genes in the model. (E) The ROC curve of LCDGs in glioma patients. Gene expression level of HPS6 (F) and LAPTM4A (G) in TCGA-glioma patients. Gene expression level of HPS6 (H) and LAPTM4A (I) in GSE4290-glioma patients. qRT-PCR (J) and immunofluorescence (K) analysis of LAPTM4A.
To further confirm the role of LAPTM4A in glioma prognosis, KM analysis indicated a significant negative association between LAPTM4A expression and OS of glioma (Fig. (Fig.6A)6A) and LGG (Fig. (Fig.6Q)6Q) patients, while no significant relationship was observed for GBM patients’ OS (Fig. (Fig.6M).6M). The AUC values for LAPTM4A in glioma (Fig. (Fig.6B)6B) and LGG (Fig. (Fig.6R)6R) OS ranged from 0.60 to 0.75, indicating moderate accuracy in predicting the prognosis of glioma and LGG. Figure Figure6C6C and D demonstrated that LAPTM4A served as an independent prognostic factor for OS in glioma patients. Interestingly, we observed significantly higher levels of LAPTM4A expression among glioma patients aged > 60 compared to those aged ≤60 (Fig. (Fig.6E).6E). Additionally, patients with G3 tumor grade (Fig. (Fig.6F),6F), GBM histological type (Fig. (Fig.6G),6G), or progressive tumor status (Fig. (Fig.6H)6H) also exhibited significantly higher LAPTM4A expression, suggesting a potential correlation between LAPTM4A and glioma progression. When exploring LAPTM4A gene expression level in GBM, TCGA (Fig. (Fig.6I),6I), GSE4290 (Fig. (Fig.6J),6J), and GSE22866 (Fig. (Fig.6K)6K) GBM patients all showed a significant upregulation compared to normal controls. LAPTM4A exhibited perfect predictive accuracy for the occurrence of GBM (Fig. (Fig.6L).6L). Similar results were found in LGG patients, with significant upregulation of LAPTM4A expression in TCGA (Fig. (Fig.6N)6N) and GSE4290 (Fig. (Fig.6O)6O) tumor samples. In LGG, LAPTM4A achieved an AUC of 1.00 (Fig. (Fig.6P).6P). To predict glioma patients’ OS, a nomogram plot incorporating LAPTM4A expression and relevant clinical characteristics was developed (Fig. (Fig.7A-B).7A-B). Moreover, tight associations were observed between LAPTM4A and several pathways, such as allograft rejection, asthma, primary immunodeficiency, and natural killer cell-mediated cytotoxicity, as revealed by GSVA and GSEA enrichment analyses (Fig. (Fig.7C,7C, D). Finally, LAPTM4A exhibited significant and distinct correlations with various chemotherapy drugs, indicating a negative association between elevated LAPTM4A expression and sensitivity to drugs like 6-mercaptopurine, tamoxifen, and imatinib (Fig. (Fig.77E).
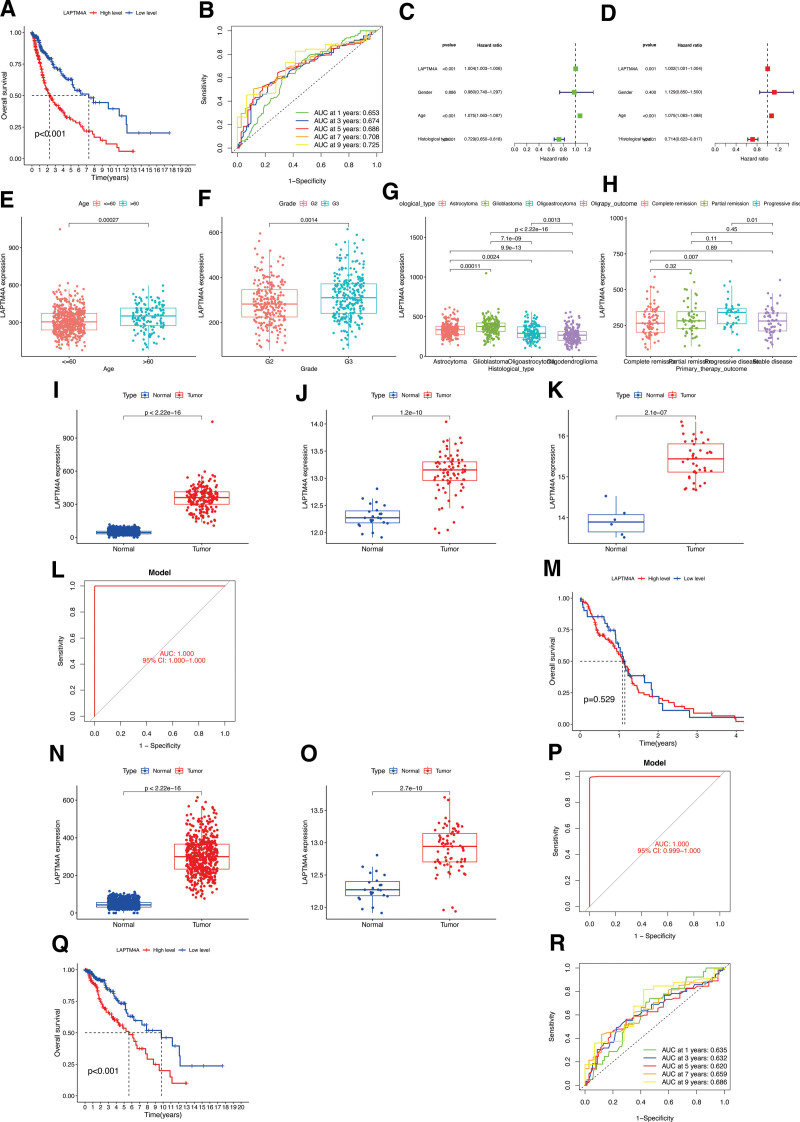
The role of LAPTM4A in glioma, LGG, and GBM. The OS of LAPTM4A in glioma were analyzed by KM (A), timeROC curve (B), univariate (C) and multivariate Cox (D) regression analyses. Correlations between LAPTM4A expression level and ages (E), tumor grades (F), histological types (G), and primary therapy outcomes (H) in glioma patients. Gene expression level of LAPTM4A in TCGA (I), GSE4290 (J), and GSE22866 (K) GBM patients. ROC curve (L) and KM (M) analyses of LAPTM4A in GBM. Gene expression level of LAPTM4A in TCGA (N) and GSE4290 (O) LGG patients. ROC curve (P), KM (Q), and time survival ROC curve (R) of LAPTM4A in LGG.
3.4. Pan-cancer analysis of LAPTM4A
KM and Cox regression analyses were conducted to investigate the correlation between LAPTM4A expression and the prognosis of pan-cancer patients. Figure Figure8A8A demonstrated that LAPTM4A was significantly upregulated in 6 types of tumors, namely CHOL, ESCA, HNSC, KIRP, LIHC, and STAD (with the exception of GBM), while it was downregulated in CESC, KICH, LUAD, LUSC, READ, and UCEC. KM survival analysis of 32 different cancer types revealed a significant association between higher LAPTM4A expression and improved OS in patients with PCPG (Fig. (Fig.8B)8B) and KIRC (Fig. (Fig.8E).8E). Conversely, LAPTM4A expression was negatively associated with OS in PAAD (Fig. (Fig.8C),8C), LIHC (Fig. (Fig.8D),8D), and HNSC (Fig. (Fig.88F).
4. Discussion
The application of advanced sequencing technologies in biology has led to the discovery of an increasing number of biomarkers associated with glioma. However, there is a pressing need to identify biomarkers that have the potential for early detection or prognostic prediction in patients with glioma. LCD, a recently identified mechanism for cell death, is believed to play a crucial role in cancer cell death.[17] Nevertheless, the precise involvement of LCD in cancer pathogenesis and clinical behavior, including its contribution to tumorigenesis, disease progression, and metastatic spread, remains largely unknown and requires further investigation. In this study, we developed an LCDGs risk signature and identified LAPTM4A as the central LCDG in glioma, highlighting the potential utility and clinical advantages of LCDGs.
To elucidate the relationships between LCD and OS in glioma patients, we conducted a comprehensive analysis of LCDGs. As a result, the following genes were identified as hub LCDGs in glioma: ACP2, AGA, AP3S2, BORCS6, CTNS, GATA2, GNS, HPS6, KIF1B, LAPTM4A, NCOA4, PSAP, SNAPIN, and SPAG9. The LCDGs risk signature demonstrated high predictive accuracy and significant prognostic value for both glioma and LGG patients. Through the utilization of 4 machine learning algorithms, ROC analysis, and differential expression analysis, LAPTM4A emerged as a highly important gene in predicting glioma compared to other LCDGs, exhibiting significant differential expression in both TCGA and external validation cohorts. Based on these findings, LAPTM4A was identified as the core gene associated with LCD in glioma.
LAPTM4A is a protein characterized by 4 transmembrane spans and was initially identified as a mouse transporter protein.[18] Previous reports have shown that LAPTM4B is widely expressed in human heart, skeletal muscle, and testis tissues, while its expression is limited in the lung and peripheral leukocytes.[19] Additionally, LAPTM4B is significantly overexpressed in HCC tissues and correlates with the differentiation status of HCC samples.[20] However, the specific role of LAPTM4A in other cancers remains unclear, and there is limited research on its mechanism in cancer cells. This study contributes to the field by conducting a pan-cancer analysis of LAPTM4A, revealing differential expression in various cancer types and its substantial contribution to cancer prognosis. Various analytical approaches were employed to verify the prognostic value of LAPTM4A in predicting the clinical outcomes of glioma, LGG, and GBM patients. Our results demonstrate a strong association between LAPTM4A and key clinical parameters, including OS in glioma and LGG, age, tumor grade, histological type, and primary therapy outcome. Furthermore, the efficacy of LAPTM4A in predicting glioma OS was validated using a nomogram.
Currently, immunotherapy is widely recognized as a promising therapeutic approach for improving outcomes in various malignant tumors.[21] In this study, we observed enrichments of LAPTM4A in immune-associated pathways, such as the intestinal immune network for IgA production, autoimmune thyroid disease, primary immunodeficiency, natural killer cell-mediated cytotoxicity, and antigen processing and presentation. Furthermore, we observed significant positive correlations between the LCDGs risk signature and stromal and immune scores. The risk signature in glioma exhibited positive correlations with the infiltration of immune cells, including B cells and CD8 + T cells, as well as with immune cell functions such as T cell co-stimulation and Type I IFN response. Considering the pivotal role of immune cells in regulating immune responses against tumors,[22] these observed correlations with immune infiltration hold significant value in predicting the OS of glioma patients. Additionally, the correlations with various chemotherapy drugs confirmed the potential application prospects of LAPTM4A in the immunotherapy of glioma. However, further investigation is necessary to elucidate the functional mechanism of LAPTM4A with immune cells.
5. Conclusions
In conclusion, this study has contributed to a better understanding of the functions of LCDGs and LAPTM4A in the pathogenesis of glioma, LGG, and GBM. LAPTM4A holds potential as a biomarker for predicting the prognosis of glioma patients and reflecting their immune conditions. To our knowledge, this study represents the first bioinformatic analysis examining the role of LCDGs in glioma, providing a unique perspective for advancing therapeutic strategies for glioma patients.
Author contributions
Conceptualization: Wei Li.
Data curation: Wei Li.
Formal analysis: Wei Li, Chao Tang.
Supervision: Wei Li.
Visualization: Wei Li.
Writing – original draft: Wei Li.
Investigation: Jun Wan.
Methodology: Jun Wang.
Validation: Jun Wang.
Writing, review and editing: Chao Tang.
Abbreviations:
- FC
- fold change
- GBM
- glioblastoma
- GTEx
- Genotype-Tissue Expression
- LASSO
- least absolute shrinkage and selection operator
- LCD
- lysosome-dependent cell death
- LCDGs
- LCD-associated genes
- LGG
- low-grade glioma
- OS
- overall survival
- ROC
- receiver operating characteristic
- TCGA
- The Cancer Genome Atlas
The authors have no funding and conflicts of interest to disclose.
The datasets generated during and/or analyzed during the current study are available from the corresponding author on reasonable request.
The datasets analyzed during the current study are available sourced from the publicly available TCGA database (https://portal.gdc.cancer.gov), GEO (https://www.ncbi.nlm.nih.gov/geo/) and GTEx database (https://gtexportal.org/home/datasets).
Supplemental Digital Content is available for this article.
How to cite this article: Li W, Wang J, Tang C. A comprehensive analysis of the prognostic value and immune microenvironment of lysosome-dependent cell death in glioma: Including glioblastoma and low-grade glioma. Medicine 2024;103:6(e36960).
References
Articles from Medicine are provided here courtesy of Wolters Kluwer Health
Data
Data behind the article
This data has been text mined from the article, or deposited into data resources.
BioStudies: supplemental material and supporting data
Similar Articles
To arrive at the top five similar articles we use a word-weighted algorithm to compare words from the Title and Abstract of each citation.
Integrative Analysis of Neuregulin Family Members-Related Tumor Microenvironment for Predicting the Prognosis in Gliomas.
Front Immunol, 12:682415, 13 May 2021
Cited by: 20 articles | PMID: 34054873 | PMCID: PMC8155525
Identification of potential biomarkers related to glioma survival by gene expression profile analysis.
BMC Med Genomics, 11(suppl 7):34, 20 Mar 2019
Cited by: 34 articles | PMID: 30894197 | PMCID: PMC7402580
SLC10A3 Is a Prognostic Biomarker and Involved in Immune Infiltration and Programmed Cell Death in Lower Grade Glioma.
World Neurosurg, 178:e595-e640, 04 Aug 2023
Cited by: 2 articles | PMID: 37543196
Identification of a novel cuproptosis-related gene signature and integrative analyses in patients with lower-grade gliomas.
Front Immunol, 13:933973, 15 Aug 2022
Cited by: 44 articles | PMID: 36045691 | PMCID: PMC9420977
Review Free full text in Europe PMC