Abstract
Free full text

Exploring indoor and outdoor dust as a potential tool for detection and monitoring of COVID-19 transmission
Summary
This study investigated the potential of using SARS-CoV-2 viral concentrations in dust as an additional surveillance tool for early detection and monitoring of COVID-19 transmission. Dust samples were collected from 8 public locations in 16 districts of Bangkok, Thailand, from June to August 2021. SARS-CoV-2 RNA concentrations in dust were quantified, and their correlation with community case incidence was assessed. Our findings revealed a positive correlation between viral concentrations detected in dust and the relative risk of COVID-19. The highest risk was observed with no delay (0-day lag), and this risk gradually decreased as the lag time increased. We observed an overall decline in viral concentrations in public places during lockdown, closely associated with reduced human mobility. The effective reproduction number for COVID-19 transmission remained above one throughout the study period, suggesting that transmission may persist in locations beyond public areas even after the lockdown measures were in place.
Introduction
The global impact of the COVID-19 pandemic on health, economy, and society has been profound. Despite the World Health Organization (WHO) declaring an end to COVID-19 as a public health emergency of international concern (PHEIC) on May 4, 2023,1 it is important to recognize that COVID-19 continues to pose a global threat. The virus has become endemic and remains a persistent health issue in numerous countries.1
While clinical diagnostic testing for COVID-19 plays a vital role in identifying and managing individual cases, relying solely on such testing may not provide a comprehensive understanding of community health risks, particularly given the presence of asymptomatic carriers.2,3,4,5,6 To effectively monitor the transmission of SARS-CoV-2 in communities, it is essential to incorporate additional surveillance tools alongside clinical testing data. This combined approach becomes even more crucial now as COVID-19 has shifted from a pandemic to an endemic state.
During the COVID-19 pandemic, wastewater-based epidemiology (WBE) emerged as a crucial aspect of public health surveillance.2,5,7,8,9,10,11 This approach is non-invasive, cost-effective, and can be implemented in public spaces, making it a potent tool for detecting community-level infection and disease outbreaks more efficiently than traditional testing methods.8,12 WBE can help pinpoint the source of infection, whether within a building or a large community. It can also be utilized for ongoing monitoring of COVID-19 prevalence in communities, assessing the impact of lockdowns and intervention programs, and providing early warnings of COVID-19 transmission.2,13,14,15,16
Recent studies have revealed that the levels of SARS-CoV-2 viral load in wastewater correlate with the incidence of COVID-19 cases in the community.14,17,18 As infected individuals move from place to place, they release the virus through fecal and bodily fluids, leaving behind a genomic footprint of SARS-CoV-2 in the environment. Depending on the environmental and meteorological conditions, the genomic footprint can persist in the environment for hours to days.19,20,21,22 However, the association between SARS-CoV-2 viral loads in wastewater and COVID-19 cases may differ depending on the monitoring scale, the time lag of COVID-19 case reporting, the outbreak stage, and the wastewater management system.23,24,25 While wastewater surveillance can provide early indications of community-wide infections, it can be challenging to identify the exact locations of cases without specific monitoring of wastewater in individual buildings.26,27 Furthermore, factors such as dilution, wastewater flow rate, the distance between the source and sampling site, and the area of the catchments can all influence the concentration of SARS-CoV-2 in wastewater.25,28
Indoor dust monitoring in environments such as hospital wards, public indoor spaces, and public transport vehicles has the potential to complement wastewater surveillance, especially in areas with a small number of infected individuals, where it is crucial to identify specific outbreaks.14,20,29,30,31,32,33 Recent studies have shown that indoor dust in rooms where infected individuals were present contains detectable levels of SARS-CoV-2, suggesting that monitoring SARS-CoV-2 in indoor dust could effectively detect COVID-19 outbreaks.14,30,31 However, the relationship between the concentration of SARS-CoV-2 RNA found in dust and the incidence of COVID-19 cases in the community is still inadequately explored. This lack of understanding may obscure the potential of dust monitoring as a complementary method of COVID-19 surveillance. Therefore, the objective of this study is to investigate the dynamics of SARS-CoV-2 RNA concentration in dust within public locations and its correlation with the incidence of COVID-19 cases in the community.
Results
SARS-CoV-2 viral concentrations in dust collected from public locations
We collected dust particles from various public locations in 16 districts of Bangkok, Thailand, between June 1, 2021, and August 29, 2021 and quantified their SARS-CoV-2 RNA concentrations (Figure S1). Our analysis revealed that in most of the districts (14 out of 16), the overall viral concentrations, including all variants, peaked in June and began to decline in July following the implementation of the nationwide lockdown (or stay-at-home) measure on July 21, 2021 (Figures 1 and and2A).2A). Throughout the study period, the three most prevalent SARS-CoV-2 variants circulating in Bangkok were the ancestral, Alpha (B.1.1.7), and Delta (B.1.617.1). The viral concentrations of the ancestral variant were found to be very low (0–7.90 × 105 copies/mg) compared to Alpha, B.1.1.7 (0–1.00 × 107 copies/mg) and Delta, B.1.617.1 (1.80 × 106–1.23 × 107 copies/mg) variants.
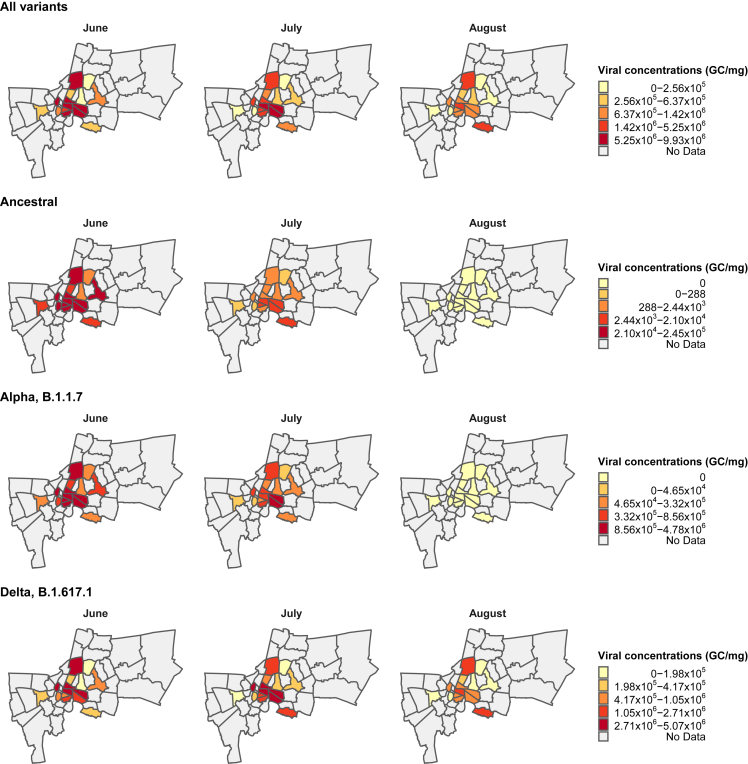
Monthly average viral concentrations detected in dust from 16 districts of Bangkok classified by variants (Ancestral, Alpha, B.1.1.7, and Delta, B.1.617.1)
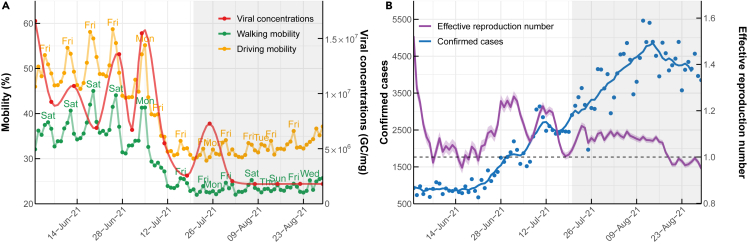
SARS-CoV-2 viral concentrations detected in dust, confirmed cases, and mobility changes in Bangkok
(A) SARS-CoV-2 viral concentrations (all variants) detected in public places and changes in mobility (%), as indicated by the ratio of trips from homes to other locations classified by transportation type (driving and walking) compared to before the pandemic (January 13, 2020), which was defined as 100%.
(B) Number of confirmed COVID-19 cases and estimated Rt. The gray area on the graph indicates the lockdown period, which lasted from July 21th until the end of the study period.
Viral concentrations detected in public areas post-lockdown were markedly lower than those recorded before the lockdown (Figure 2A). Despite the decreasing viral concentrations detected in public places, the number of daily confirmed COVID-19 cases in Bangkok showed a near-continuous rise from June 1 to August 29, 2021, even after implementing the lockdown measure (Figure 2). The effective reproduction number, Rt, representing the average number of secondary cases caused by a primary infected individual, remained above the critical threshold of 1 for nearly a month after the lockdown. This observation suggests the possibility of COVID-19 transmission persisting in locations beyond public areas even after the enforcement of lockdown measures.
The reduction in viral concentrations detected in public places aligns with the decline in human mobility in Bangkok (Figures 2A and Table 1). Before the lockdown, walking and driving mobility varied between 23%– 45% and 30%–59%, respectively, in comparison to the mobility levels before the pandemic, which was defined as 100%. After the implementation of the lockdown, these values decreased to approximately 22–26% and 30–37%, respectively, and persisted at low levels throughout the study period. The decrease in human mobility was observed around 10 days prior to the enforcement of the lockdown control measure, coinciding with the reduction in SARS-CoV-2 viral concentrations in public places (Figure 2A).
Table 1
Summary of viral concentrations detected in dust, time-varying reproduction number (Rt), daily cases, and human mobility parameter in Bangkok
Mean | SD | Min | P25 | Median | P75 | Max | |
---|---|---|---|---|---|---|---|
Viral loads detected in dust during the pre-lockdown period (from Jun 1 to July 20, 2021) | |||||||
Total | 9.58×106 | 4.61×106 | 2.56×106 | 6.71×106 | 9.25×106 | 1.36×107 | 1.58×107 |
Ancestral | 1.31×105 | 2.51×105 | <LODa | <LODa | 6.13×104 | 1.00×105 | 7.90×105 |
Alpha, B.1.1.7 | 3.95×106 | 3.04×106 | 7.80×104 | 1.87×106 | 3.66×106 | 5.59×106 | 1.00×107 |
Delta, B.1.617.1 | 5.61×106 | 3.11×106 | 2.48×106 | 3.53×106 | 4.75×106 | 5.77×106 | 1.23×107 |
Daily records | |||||||
Cases | 1496 | 725 | 675 | 903 | 1158 | 2020 | 3137 |
Rt | 1.11 | 0.10 | 0.96 | 1.04 | 1.09 | 1.19 | 1.41 |
Human mobility | |||||||
Driving | 45 | 8 | 30 | 42 | 47 | 50 | 59 |
Walking | 33 | 6 | 23 | 30 | 34 | 37 | 45 |
Viral loads detected in dust during the lockdown period (from July 21 to August 29, 2021) | |||||||
Total | 3.35×106 | 2.46×106 | 1.80×106 | 1.80×106 | 1.81×106 | 4.67×106 | 7.28×106 |
Ancestral | <LODa | <LODa | <LODa | <LODa | <LODa | <LODa | <LODa |
Alpha, B.1.1.7 | 8.25×103 | 2.02×104 | <LODa | <LODa | <LODa | <LODa | 4.95×104 |
Delta, B.1.617.1 | 2.75×106 | 2.20×106 | 1.80×106 | 1.80×106 | 1.81×106 | 1.99×106 | 7.23×106 |
Daily records | |||||||
Cases | 4068 | 716 | 1967 | 3614 | 4136 | 4552 | 5463 |
Rt | 1.05 | 0.06 | 0.95 | 0.99 | 1.07 | 1.09 | 1.13 |
Human mobility | |||||||
Driving | 33 | 2 | 30 | 31 | 32 | 33 | 37 |
Walking | 23 | 1 | 22 | 23 | 23 | 24 | 26 |
P25 and P75 is the 25th, and 75th percentile, respectively. SD is the standard deviation.
Association between SARS-CoV-2 viral concentrations detected in dust and the relative risk of COVID-19
We estimated the association between SARS-CoV-2 viral concentrations found in dust and the relative risk of COVID-19, compared to a baseline with no viral concentration in dust and no reported cases of COVID-19 (Figure 3). We found that the viral concentrations seen in dust are positively associated with the relative risk of COVID-19, with the highest risk occurring at a 0-day lag and decreasing as the lag time increased. We considered the viral concentrations in the dust as a leading indicator of the relative risk of COVID-19, and our results indicated that the association between the number of confirmed COVID-19 cases in a specific location was strongest when the dust viral concentration in that location was highest. The association weakened as the time lag increased.
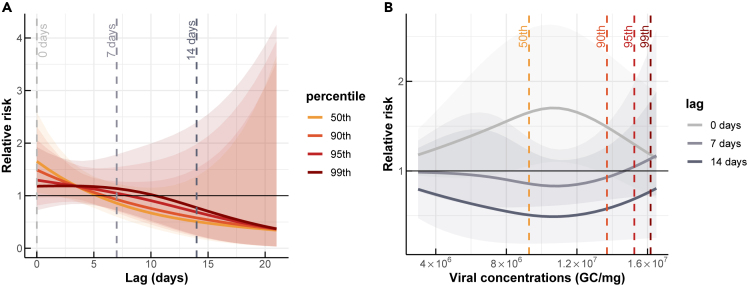
Associations between SARS-CoV-2 viral concentrations detected in the dust and COVID-19 risks over different lags
(A) The COVID-19 risks at the 50th, 90th, 95th, and 99th percentiles of detected viral concentrations.
(B) The COVID-19 risks for lags of 0, 7, and 14 days.
At the 50th percentile of viral concentrations, the relative risk (RR) was estimated at 1.65 (95% confidence interval (CI): 1.06, 2.59) at a 0-day lag, and decreased to less than 1 with lags longer than 5 days (Figure 3A). At a 0-day lag, the relative risk of COVID-19 was consistently higher than 1 across all detected viral concentrations, indicating a greater risk of COVID-19 transmission with higher viral concentrations (Figure 3B). Conversely, at 7- and 14-day lags, the risk of COVID-19 was less than 1, except in cases of very high viral concentrations. This suggests that at these longer time lags, there was no significant association between viral concentrations and the risk of COVID-19.
Association between human mobility and the relative risk of COVID-19
Since walking and driving mobility showed a similar trend and high correlation with each other (Figure 2A), the analysis here focused on the association between the relative risk of COVID-19 and human mobility represented by walking. We found that human mobility, as measured by walking, was most strongly associated with COVID-19 risk with a 5-day lag (Figure 4A). We evaluated the relative risk of COVID-19 at different percentiles of mobility, including the 25th, 50th, 90th, and 99th percentiles, compared to pre-pandemic mobility levels. The highest relative risks of COVID-19 were observed at the 99th percentile with a value of 1.17 (95% CI: 0.83, 1.67), followed by the 90th percentile with a value of 1.14 (95% CI: 0.80, 1.61), and the 25th and 50th percentiles with values of 1.18 (95% CI: 0.86, 1.57) and 1.11 (95% CI: 0.78, 1.58), respectively. Despite an increase in the number of COVID-19 infections over time (Figure 2B), we found no significant difference in COVID-19 infection risk for varying levels of human mobility (Figure 4B).
District-level SARS-CoV-2 viral concentrations and the relative risk of COVID-19 infection
We examined the district-level association between SARS-CoV-2 viral concentrations in dust and COVID-19 risk, with a focus on the 50th, 90th, 95th, and 99th percentiles of viral concentrations at 0-day lag (Figure 5 and Table 2). Results showed that the Chatu Chak district had the highest COVID-19 risk at the 50th percentile of viral concentrations, while the Klong Toei district had the lowest risk. At this percentile, seven out of 16 districts had a relative risk of less than 1. However, at the 90th, 95th, and 99th percentiles, the number of districts with a relative risk greater than 1 increased to six. The relative risk decreased with an increase in viral concentration percentile for only three districts (Bang Kapi, Chatu Chak, and Vadhana), while the other 13 districts had a higher relative risk with a higher percentile of viral concentrations.
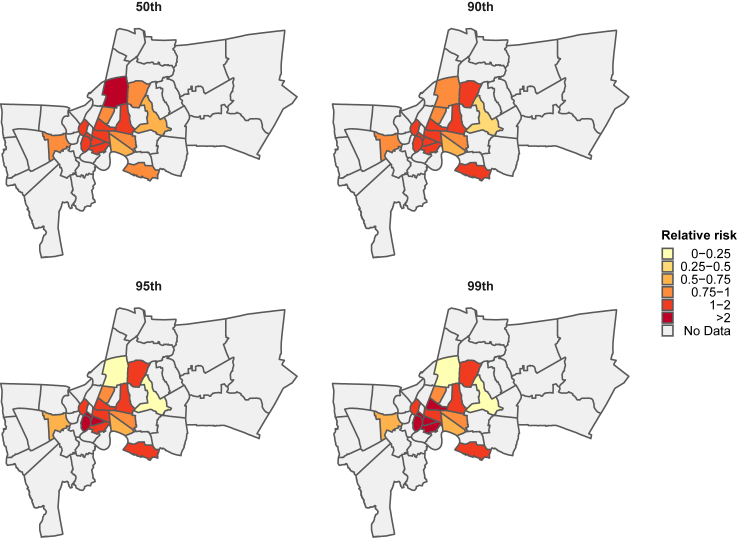
District-level association between SARS-CoV-2 viral concentrations in dust and relative risk of COVID-19 at 0-day lag
The relative risk of COVID-19 associated with district-level viral concentrations at the 50th, 90th, 95th, and 99th percentiles are shown.
Table 2
Relative risk (RR) for 16 districts at 0-day lag
Districts | RR at 50th | RR at 90 th | RR at 95 th | RR at 99 th |
---|---|---|---|---|
Bang Kapi | 0.667 (0.433, 1.028) | 0.251 (0.045, 1.389) | 0.153 (0.013, 1.756) | 0.003 (0, 11.764) |
Bang Na | 0.999 (0.954, 1.046) | 1.212 (1.075, 1.366) | 1.204 (1.073, 1.35) | 1.133 (0.994, 1.29) |
Bang Rak | 1.645 (1.241, 2.18) | 1.458 (1.084, 1.96) | 2.286 (1.263, 4.138) | 2.876 (1.360, 6.083) |
Chatu Chak | 2.065 (0.824, 5.176) | 0.824 (0.232, 2.926) | 0.217 (0.047, 0.994) | 0.002 (0, 0.029) |
Huai Khwang | 1.025 (0.979, 1.073) | 1.573 (1.108, 2.234) | 1.512 (1.052, 2.172) | 1.410 (0.938, 2.120) |
Khlong San | 1.166 (0.881, 1.543) | 1.310 (0.983, 1.747) | 19.501 (1.809, 210.239) | 1531.457 (4.715, 497375.152) |
Khlong Toei | 0.644 (0.496, 0.836) | 0.682 (0.565, 0.822) | 0.663 (0.548, 0.803) | 0.656 (0.540, 0.797) |
Lat Phrao | 0.986 (0.948, 1.025) | 1.102 (0.964, 1.259) | 1.289 (0.962, 1.727) | 1.367 (0.957, 1.954) |
Parthum Wan | 1.482 (0.632, 3.476) | 1.544 (0.674, 3.537) | 1.637 (0.703, 3.814) | 1.713 (0.724, 4.051) |
Phasi Charoen | 0.952 (0.879, 1.030) | 0.786 (0.682, 0.905) | 0.672 (0.562, 0.804) | 0.630 (0.517, 0.767) |
Phaya Thai | 0.994 (0.892, 1.108) | 0.963 (0.877, 1.057) | 0.963 (0.868, 1.068) | 0.962 (0.826, 1.120) |
Phra Nakhon | 1.315 (0.965, 1.793) | 1.270 (0.982, 1.641) | 1.478 (1.162, 1.880) | 1.566 (1.231, 1.993) |
Ratchathewi | 1.126 (1.016, 1.248) | 1.174 (0.972, 1.417) | 1.427 (1.034, 1.967) | 5.296 (1.304, 21.511) |
Samphanthawong | 1.448 (0.370, 5.661) | 1.806 (0.076, 43.158) | 9.096 (0.008, 9885.893) | 19.218 (0.003, 123238.900) |
Sathon | 1.586 (0.939, 2.678) | 1.522 (1.036, 2.235) | 1.870 (1.280, 2.733) | 2.318 (1.549, 3.469) |
Vadhana | 0.948 (0.653, 1.375) | 0.918 (0.672, 1.255) | 0.848 (0.557, 1.29) | 0.835 (0.535, 1.302) |
Discussion
This study provides important insights into the relationship between the concentration of SARS-CoV-2 RNA in dust and the incidence of COVID-19 cases in the community. By monitoring the viral concentrations of SARS-CoV-2 in dust particles in public areas of Bangkok, Thailand, we discovered a positive association between viral concentrations in dust and the relative risk of COVID-19. Notably, the highest relative risk was observed immediately (0-day lag) and gradually decreased with increasing lag times (Figure 3). These findings suggest that measuring viral concentrations in dust can serve as an indicator to assess the risk of COVID-19 transmission, which is consistent with prior research.14,34 Furthermore, the presence of higher viral concentrations in the dust could indicate a greater likelihood of COVID-19 incidence in the community.
Viable SARS-CoV-2 RNA can persist on surfaces ranging from 4 h to 7 days, depending on the surface and weather conditions.21,22 Thus, the transmission of the virus could be through contaminated intermediate objects such as fomites (fomite transmission).35,36,37 Besides the fomite transmission, the spread of viruses could be from airborne transmission via droplets and aerosols.38,39 Compared to fomite transmission, the viability of aerosolized SARS-CoV-2 is relatively short, with a half-life of approximately 1–3 h.40 In this study, we collected indoor dust particles as our samples using a vacuum cleaner that could capture aerosols, droplets, and fomites, leading to a wide range of viral concentrations across different particle sizes. We found that high viral concentrations in dust particles were associated with a longer period of COVID-19 risk (RR > 1), suggesting that cleaning surfaces more often than usual during disease outbreaks in public locations might be necessary.
We found heterogeneity in the association between SARS-CoV-2 viral concentrations and COVID-19 risk at the district level. Some districts had a COVID-19 risk of less than 1, while others had a COVID-19 risk of more than 1 for all ranges of viral concentrations (Figure 5). This heterogeneity can possibly be attributed to the presence of mobile individuals who reside and work in specific areas within the city of Bangkok. As dust particles were collected from public areas with high foot traffic, infected individuals may have spread the virus as they moved between locations. If confirmed by further research, this finding would have implications for district-level policies aimed at controlling the spread of COVID-19 and future infectious disease outbreaks.
To enhance our understanding of the link between SARS-CoV-2 viral concentrations in public spaces and the effectiveness of lockdown measures, we conducted an investigation to explore the relationship between human mobility and COVID-19 risk. Our findings demonstrate a close correlation between the decrease in human mobility and the decline in viral concentrations detected in public areas (Figure 2A). This observation underscores the significance of reducing human mobility as a crucial strategy for controlling the spread of the virus, as it effectively limits opportunities for person-to-person transmission in public spaces.41,42,43,44
However, our analysis also revealed that, despite the decline in viral concentrations detected in public places following the implementation of lockdown measures, the effective reproduction number, Rt, remained above the critical threshold of 1 for nearly a month after the lockdown. This suggests that COVID-19 transmission might persist in non-public settings even after the enforcement of lockdown measures. Notably, a study examining data from Singapore and Vo’, Italy, has highlighted the potential for household infections to play a more significant role in the spread of SARS-CoV-2 when community mobility decreases during lockdowns.45 Similarly, research conducted in Thailand has demonstrated the high transmissibility of SARS-CoV-2 within Thai households, with asymptomatic index cases effectively transmitting the virus to their household contacts.46 This compelling evidence suggests that, alongside mobility-restricting lockdown measures, isolating infected individuals within households may be essential for effectively curtailing the transmission of SARS-CoV-2.
Our study has some limitations that must be acknowledged. Firstly, we only collected dust particles bi-weekly for three months, between June 1, 2021, and August 29, 2021, in 16 out of 50 districts in Bangkok. Collecting samples for more extended periods in more districts might be necessary to draw more robust conclusions. However, our study is the first to investigate the association between SARS-CoV-2 RNA concentration found in dust in public areas and the incidence of COVID-19 cases, providing valuable insights into dust surveillance. Secondly, we did not monitor the frequency and method of cleaning in each location, which may have caused variation in the detected viral concentration. Finally, some locations, such as community markets, are open-air, while others, such as community centers and public elevators, are closed spaces with air conditioners. Differences in air circulation in each location may have affected the viral concentrations.
In summary, this study provides insights into the relationship between SARS-CoV-2 RNA concentration in dust and the prevalence of COVID-19 cases within the community. Through the surveillance of viral concentrations in dust particles found in public spaces in Bangkok, Thailand, we have identified a positive correlation between these concentrations and the relative risk of COVID-19. Higher viral concentrations in dust appear to be indicative of an increased likelihood of COVID-19 cases within the community. Furthermore, our research has revealed a strong correlation between the reduction in human mobility and the decrease in viral concentrations detected in public areas. This finding underscores the critical importance of reducing human mobility as a key strategy in controlling virus spread, effectively limiting opportunities for person-to-person transmission in public settings.
STAR
Methods
Key resources table
REAGENT or RESOURCE | SOURCE | IDENTIFIER |
---|---|---|
Bacterial and virus strains | ||
Murine hepatitis virus; Strain: MHV-A59 | ATCC | ATC.VR-764 |
Chemicals, peptides, and recombinant proteins | ||
RNase-Free Water | Qiagen | 129112 |
TaqPath™ qPCR Master Mix, CG | Thermo Fisher Scientific | A15297 |
Critical commercial assays | ||
RNeasy PowerSoil Total RNA Kit | Qiagen | 12866–25 |
iTaq Universal Probes One-Step Kit | Bio-Rad | 1725141 |
Deposited data | ||
Confirmed COVID-19 cases in Thailand | Department of Disease Control, Ministry of Public Health, Thailand | https://data.go.th/dataset/covid-19-daily |
Daily human mobility in Bangkok Metropolis | Apple mobility report | https://covid19.apple.com/mobility |
Oligonucleotides | ||
2019-nCoV_N1-F 5′-GACCCCAAAATCAGCGAAAT-3′ 3 | U.S. Centers for Disease Control and Prevention (CDC) N1, N2, E and human RP gene primers sets | https://www.cdc.gov/coronavirus/2019-ncov/lab/multiplex.html |
2019-nCoV_N1-R 5′-TCTGGTTACTGCCAGTTGAATCTG-3′ 3 | U.S. Centers for Disease Control and Prevention (CDC) N1, N2, E and human RP gene primers sets | https://www.cdc.gov/coronavirus/2019-ncov/lab/multiplex.html |
2019-nCoV_N1-P 5′-FAM-ACCCCGCATTACGTTTGG TGGACC-ZEN/Iowa Black-3′ 3 | U.S. Centers for Disease Control and Prevention (CDC) N1, N2, E and human RP gene primers sets | https://www.cdc.gov/coronavirus/2019-ncov/lab/multiplex.html |
2019-nCoV_N2-F 5′-TTACAAACATTGGCCGCAAA-3′ | U.S. Centers for Disease Control and Prevention (CDC) N1, N2, E and human RP gene primers sets | https://www.cdc.gov/coronavirus/2019-ncov/lab/multiplex.html |
2019-nCoV_N2-R 5′-GCGCGACATTCCGAAGAA-3′ | U.S. Centers for Disease Control and Prevention (CDC) N1, N2, E and human RP gene primers sets | https://www.cdc.gov/coronavirus/2019-ncov/lab/multiplex.html |
2019-nCoV_N2-P 5′-FAM-ACAATTTGCCCCCAG CGCTTCAG- ZEN/Iowa Black-3′ | U.S. Centers for Disease Control and Prevention (CDC) N1, N2, E and human RP gene primers sets | https://www.cdc.gov/coronavirus/2019-ncov/lab/multiplex.html |
2019-nCoV_N3-F 5′-GGGAGCCTTGAATACACCAAAA-3′ | U.S. Centers for Disease Control and Prevention (CDC) N1, N2, E and human RP gene primers sets | https://www.cdc.gov/coronavirus/2019-ncov/lab/multiplex.html |
2019-nCoV_N3-R 5′-TGTAGCACGATTGCAGCATTG-3′ | U.S. Centers for Disease Control and Prevention (CDC) N1, N2, E and human RP gene primers sets | https://www.cdc.gov/coronavirus/2019-ncov/lab/multiplex.html |
2019-nCoV_N3-P 5′-FAM-AYCACATTGGCACCC GCAATCCTG- ZEN/Iowa Black-3′ | U.S. Centers for Disease Control and Prevention (CDC) N1, N2, E and human RP gene primers sets | https://www.cdc.gov/coronavirus/2019-ncov/lab/multiplex.html |
E_Sarbeco_F 5′-ACAGGTACGTTAATAGTTAATAGCGT-3′ | U.S. Centers for Disease Control and Prevention (CDC) N1, N2, E and human RP gene primers sets | https://www.cdc.gov/coronavirus/2019-ncov/lab/multiplex.html |
E_Sarbeco_R 5′-ATATTGCAGCAGTACGCACACA-3′ | U.S. Centers for Disease Control and Prevention (CDC) N1, N2, E and human RP gene primers sets | https://www.cdc.gov/coronavirus/2019-ncov/lab/multiplex.html |
E_Sarbeco_P1 5′-FAM-ACACTAGCCATCCTTACT GCGCTTCG-ZEN/Iowa Black-3′ | U.S. Centers for Disease Control and Prevention (CDC) N1, N2, E and human RP gene primers sets | https://www.cdc.gov/coronavirus/2019-ncov/lab/multiplex.html |
RP-F- 5′-AGA TTT GGA CCT GCG AGC G -3′ | U.S. Centers for Disease Control and Prevention (CDC) N1, N2, E and human RP gene primers sets | https://www.cdc.gov/coronavirus/2019-ncov/lab/multiplex.html |
RP-R- 5′-GAG CGG CTG TCT CCA CAA GT -3′ | U.S. Centers for Disease Control and Prevention (CDC) N1, N2, E and human RP gene primers sets | https://www.cdc.gov/coronavirus/2019-ncov/lab/multiplex.html |
RP-P- 5′- FAM – TTC TGA CCT GAA GGC TCT GCG CG – BHQ-1 -3′ | U.S. Centers for Disease Control and Prevention (CDC) N1, N2, E and human RP gene primers sets | https://www.cdc.gov/coronavirus/2019-ncov/lab/multiplex.html |
RP-P- 5′- FAM-TTC TGA CCT/ZEN/GAA GGC TCT GCG CG-3 - IABkFQ -3′ | U.S. Centers for Disease Control and Prevention (CDC) N1, N2, E and human RP gene primers sets | https://www.cdc.gov/coronavirus/2019-ncov/lab/multiplex.html |
Ancestral ACAATTTGGCAGAGACATCGC | Li et al. and Wannigama et al.25,47 | N/A |
Ancestral AGAACATGGTGTAATGTCAAGAATC | Li et al. and Wannigama et al.25,47 | N/A |
Ancestral /56- FAM/ACTGATGCTGTCCGTGATCCA CAG/3BHQ_1/ | Li et al. and Wannigama et al.25,47 | N/A |
Alpha (B.1.1.7) ACAATTTGGCAGAGACATCGA | Li et al. and Wannigama et al.25,47 | N/A |
Alpha (B.1.1.7) AGAACATGGTGTAATGTCAAGAATC | Li et al. and Wannigama et al.25,47 | N/A |
Alpha (B.1.1.7) /56- FAM/ACTGATGCTGTCCGTGATCCA CAG/3BHQ_1/ | Li et al. and Wannigama et al.25,47 | N/A |
Delta (B.1.617.2) 5′ GGTTGGTGGTAATTATAATTCCCG | Li et al. and Wannigama et al.25,47 | N/A |
Delta (B.1.617.2) 5′ CCTTCAACACCATTACAACGTT | Li et al. and Wannigama et al.25,47 | N/A |
Delta (B.1.617.2) 5′ FAM-TCTCTCAAAAGGTTTGAGATTAGACTTCC-BHQ | Li et al. and Wannigama et al.25,47 | N/A |
Recombinant DNA | ||
Synthetic full-length SARS-CoV- 2 RNA | USA-WA1/2020 | ATCC-VR-1986D |
Software and algorithms | ||
Infection incidences estimate code | Huisman et al.20 | https://github.com/JSHuisman/wastewaterRe |
mgcv | R software package | https://www.r-project.org |
dplyr 1.0.7 | R software package | https://www.r-project.org |
tidyverse 1.3.2 | R software package | https://www.r-project.org |
splines 4.1.0 | R software package | https://www.r-project.org |
zoo 1.8–9 | R software package | https://www.r-project.org |
astsa 1.14 | R software package | https://www.r-project.org |
lubridate 1.9.0 | R software package | https://www.r-project.org |
patchwork 1.1.1 | R software package | https://www.r-project.org |
ggplot2 3.3.5 | R software package | https://www.r-project.org |
dslabs 0.7.4 | R software package | https://www.r-project.org |
scales 1.2.1 | R software package | https://www.r-project.org |
ggalt 0.4.0 | R software package | https://www.r-project.org |
ggpubr 0.4.0 | R software package | https://www.r-project.org |
Other | ||
Centricon® Plus-70 centrifugal ultrafilters | Merck Millipore | UFC710008 |
BLACK+DECKER dustbuster- CHV1410L | BLACK+DECKER | CHV1410L |
Resource availability
Lead contact
Further information and requests for resources, raw data, and code should be directed to and will be fulfilled by the lead contact, Dhammika Leshan Wannigama ([email protected]).
Materials availability
The authors confirm that no new reagents were generated in the study.
Data and code availability
- • Data used for the analysis are included in this published article and its supplemental data. As this study is ongoing, additional data will be available upon reasonable request from the corresponding author DLW.
- • The cumulative number of confirmed COVID-19 cases in Thailand attributed to each province was obtained from the Department of Disease Control, Ministry of Public Health, Thailand (https://data.go.th/dataset/covid-19-daily). The data on daily human mobility in Bangkok Metropolis were obtained from the Apple mobility report. The study is presented according to STROBE guidelines.
- • This paper does not report the original code.
- • Any additional information required to reanalyze the data reported in this paper is available from the lead contact upon request.
- • Data visualization For the maps and the data visualization, we used R program version 4.2.2 with tidyverse 1.3.2, lubridate 1.9.0, scales 1.2.1, zoo 1.8.11, ggplot2 3.4.1, gridExtra 2.3, ggpmisc 0.5.2, ggpubr 0.5.0, and sf 1.0.12 packages.
Experimental model and study participant details
Dust surveillance
Bulk dust samples were collected weekly for 3 months at 49 sampling points in Bangkok province between June 2021 and August 2021. Sampling locations were selected to represent residents and public spaces based on access to the transportation system, population density, and popularity (Arifwidodo and Chandrasiri, 2020) (supplementary data). Bulk dust samples from lobby areas at residential buildings (n = 4), cafeterias (n = 6), shopping centers (n = 4), community centers (n = 2), community markets (n = 6), office buildings (n = 5), public elevators (n = 9), and public toilets (n = 12) were sampled.
COVID-19 and human mobility data
We gathered information on the number of confirmed COVID-19 cases in the Bangkok metropolis between June 1 and August 29, 2021, by extracting data from the daily report of the Department of Disease Control, Ministry of Public Health of Thailand.48 The data was pre-processed to count the daily confirmed cases and filter out cases outside the Bangkok metropolis.
We obtained data on daily human mobility in Bangkok Metropolis by accessing information from the Apple mobility report.49 The data was collected from users' devices using the Apple Maps app, which tracked their movements by walking or driving. The mobility report shows the percentage change in mobility compared to the pre-pandemic period (January 13th, 2020) and is categorized by walking and driving.
Method details
Sample collection
In total, 588 samples were collected using mini handheld vacuum cleaners (BLACK+DECKER dustbuster- CHV1410L). A separate vacuum cleaner was used for each sampling site, and samples were collected from surfaces walls, handrails, door handles, bench surfaces, window shields, toilet seats, table, or chair surfaces, to cover the maximum surface areas where will have interactions with people. Multiple samples were collected for large spaces and combined as a single sample (e.g., lobby areas at residential buildings, cafeterias, shopping centers, community centers, community markets, and office buildings).
RNA extraction
Viral RNA was extracted from bulk dust samples using a Qiagen RNeasy Power microbiome extraction kit procedure (Qiagen, Hilden, Germany), as described previously.9 Triplicates of approximately 50 mg of dust were removed from bulk dust samples using a sterilized spatula, and each replicate was extracted individually in a laminar flow biosafety cabinet. All extraction sets included a blank to detect potential contamination. To quantify SARS-CoV-2 RNA concentration, 2.5 mL of well-mixed centricon concentrates were added directly to a commercial kit optimized for isolation of total RNA from environmental samples according to the manufacturers protocol (RNeasy PowerSoil Total RNA Kit, Qiagen).2,9,10 Two replicate RNA extractions and analyses were performed for each sample. Isolated RNA pellets were dissolved in 50 μL of ribonuclease-free water, and total RNA was measured by spectrophotometry (NanoDrop, Thermo Fisher Scientific) as previously described.2,9,10 RNA was extracted, and SARS-CoV-2 gene markers (N1, N2, N3, and E) were quantified by Real-time qPCR immediately or within one week after RNA extraction (storage at −80°C) following the same procedure described in our previous study.2
SARS-CoV-2 quantification by real-time qPCR
SARS-CoV-2 RNA was quantified by one-step qRT–PCR using the U.S. Centers for Disease Control and Prevention (CDC) primer N1, N2, and N3 sets that each target a different region of the nucleocapsid (N)gene2,9,10 and the set targeting the envelope protein (E) gene from Medema et al. to include targets against two separate SARS-CoV-2 genes (Table S1).2,9,10 The specificity of these primer/probe sets against other respiratory viruses, including human coronaviruses, had been confirmed by several other studies.2,9,10 For control and in accordance with the CDC protocol, analysis was also conducted for the human RP gene,2,9,10 and SARS-CoV-2 results were reported only if RP gene detection was positive. Samples were analyzed using the Bio-Rad iTaq Universal Probes One-Step Kit in 20-μL reactions run at 50°C for 10min and 95°C for 1 min, followed by 40 cycles of 95°C for 10
s and 60°C for 30
s per the manufacturer’s recommendations. SARS-CoV-2 RNA concentrations were determined using a standard curve as previously described and presented as virus RNA copies.2,9,10 For the standard curve, complementary DNA synthesized from full-length SARS-CoV-2 RNA (WA1-USA strain) was used as a template to generate SARS-CoV-2 N gene transcripts as previously described.2,9,10 To validate our N1, N2, N3 and E primers sets, standard curves using the 10-fold series dilution of the N and E gene transcripts were analyzed as previously described.2,9,10 The primer sets generated a standard curve with N1 primer values of R2 0.99, efficiency: 97.1%, N2 primer R2: 0.99, efficiency: 98.4%, N3 primer R2: 0.99, efficiency: 96.1%, and E primer R2: 0.98, efficiency: 94.3%, wild-type R2: 0.99, efficiency: 96.7%, Alpha (B.1.1.7) R2: 0.99, efficiency 99.4%, Delta R2: 0.99, efficiency 98.6%. The SARS-CoV-2 concentration results were adjusted to the total RNA extracted by multiplying sample concentrations by the ratio of the maximum RNA concentration to the sample RNA concentration. This accounts for week-to-week variations in dust and RNA extraction efficiency. SARS-CoV-2 variant concentrations were measured using the primers given in the key resources table.
Estimation of the effective reproduction number
We utilized a statistical method devised by Cori et al. to compute the effective reproduction number, Rt, using the "EpiEstim" package in R software (Version 4.2.2).50,51,52 Rt represents the mean number of secondary cases generated by an infected individual.3,51,53 An Rt value exceeding the critical threshold of 1 signifies an increasing epidemic size, indicating the possibility of spreading infection within the population. Conversely, an Rt value that falls below 1 suggests a decreasing epidemic size at time t. The Rt estimation only requires the count of daily new confirmed cases and the serial interval distribution, which was assumed to be a discretized Gamma distribution with an average and standard deviation of 3.96 days and 4.75 days, respectively.54
Quantification and statistical analysis
To investigate the association between the relative risk of COVID-19 infection (RR) and viral concentrations found in dust, a generalized additive modeling (GAM) framework with a quasi-Poisson function, combined with a distributed lag non-linear model (DLNM), was employed to determine the exposure-response relationship through a cross-basis function, and to explore the lag distribution.55
DLNM has demonstrated its efficacy in the analysis and interpretation of relationships that exhibit temporal spread and potential non-linearity. This modeling approach holds particular utility in fields such as environmental and epidemiological research. For example, Yuan et al. applied DLNM to investigate the correlation between temperature and daily new COVID-19 cases across 188 countries,56 while Nottmeye et al. employed DLNM to explore the influence of temperature and humidity on COVID-19 incidence in England.57
In our study, we employed DLNM to investigate the intricate non-linear and lagged associations between viral concentration in dust and confirmed COVID-19 cases. To facilitate this analysis, we constructed a cross-basis matrix for viral concentrations in dust and utilized a normal cubic spline function with 3° of freedom (DF) for estimation. The model used in determining the relationship between COVID-19 confirmed cases and viral concentrations is given by
where
We also explored the association between COVID-19-confirmed cases and human mobility using the same model as shown in Equation 1, but with a different cross-basis function. A cubic regression spline function was applied with knots in the range of 25 (minimum) to 45 (maximum) for the mobility trend and 0, 7, and 14 days for the lag. We conducted the analysis using the “dlnm” 2.4.758 and “splines” 4.2.259 packages in R software.
Acknowledgments
We thank all the volunteers who kindly supported with sample collection. Also, thanks to Dr. Ong-orn Prasarnphanich at the United States Centers for Disease Control and Prevention (CDC) Thailand for technical support and the previous Chargé d'Affaires of the United States of America to Thailand (U.S. Embassy and Consulate in Thailand), Mr. Michael Heath, for facilitating collaboration with the CDC and Armed Forces Research Institute of Medical Sciences (AFRIMS). We also thank the LGBTQIA+ community in Thailand for generous support with sample collection, TEDxChiangMai team and Martin Venzky-Stalling for facilitating platform for collaboration, and marginalized, vulnerable indigenous communities in Thailand for support with sample collection. Special thanks to Nuttawut Kietchaiyakorn for helping with the illustrations. We, the authors of this paper, embrace inclusive, diverse, and equitable conduct of research. Our team comprises individuals who self-identify as underrepresented ethnic minorities, gender minorities, members of the LGBTQIA+ community, and individuals living with disabilities. We actively promote gender balance in our reference list while maintaining scientific relevance.
Dhammika Leshan Wannigama was supported by Balvi Filantropic Fund and Chulalongkorn University (Second Century Fund-C2F Postdoctoral Fellowship), University of Western Australia (Overseas Research Experience Fellowship) and Yamagata Prefectural Central Hospital, Yamagata, Japan (Clinical Residency Fellowship). Charin Modchang was supported by the Centre of Excellence in Mathematics, Ministry of Higher Education, Science, Research and Innovation, Thailand, Center of Excellence on Medical Biotechnology (CEMB), and Thailand Center of Excellence in Physics (ThEP). Sudarat Chadsuthi was supported by Naresuan University and National Science, Research and Innovation Fund (NSRF). Anthony Kicic is a Rothwell Family Fellow. The sponsor(s) had no role in study design; in the collection, analysis, and interpretation of data; in the writing of the report; or in the decision to submit the article for publication.
Author contributions
Conception, S.C., P.H., C.H., M.A., C.M., and D.L.W.; Data collection, R.K.; Data curation, S. Anupong ., P.H., C.H., P.V., T.S., M.A., and D.L.W.; Formal analysis, S. Anupong ., S.C., P.H., C.H., P.V., T.S., M.A., C.M., and D.L.W.; Statistical analysis, S. Anupong and S.C.; Supervision, S. Anupong, S.C., P.H., C.H., P.P., A.H.R.S.M., S.F., A.T.H., S.L., R.J.S., P.O., N.K.D.R., K.M., L.C., A.N., K. Sei, D.S., T.F., K. Shibuya, P.G.H., A.K., A.C.S., T.C., S.T., S. Abe, H.I., M.A., C.M., and D.L.W.; Writing- original draft, S. Anupong, S.C., P.H., C.H., M.A, C.M., and D.L.W.; Critical review and editing, S. Anupong, S.C., P.P., A.H.R.S.M., S.F., A.T.H., S.L., R.J.S., P.O., N.K.D.R., P.K., N.N., V.N.B., W.T., A.L., T.K., K.M., L.C., A.N., K. Shibuya, R.K., D.S., T.F., K. Sei, P.G.H., A.K., A.C.S., T.C., S.T., S. Abe , H.I., and C.M.; Investigation, P.H., C.H.,M.A.,C.M., and D.L.W.; Funding acquisition, P.H., C.H., C.M., and D.L.W.
Declaration of interests
The authors declare no competing interests.
Footnotes
Supplemental information can be found online at https://doi.org/10.1016/j.isci.2024.109043.
Supplemental information
References
Articles from iScience are provided here courtesy of Elsevier
Citations & impact
Impact metrics
Alternative metrics

Discover the attention surrounding your research
https://www.altmetric.com/details/158803354
Article citations
Early treatment with fluvoxamine, bromhexine, cyproheptadine, and niclosamide to prevent clinical deterioration in patients with symptomatic COVID-19: a randomized clinical trial.
EClinicalMedicine, 70:102517, 14 Mar 2024
Cited by: 1 article | PMID: 38516100 | PMCID: PMC10955208
Lay summaries
Plain language description
Similar Articles
To arrive at the top five similar articles we use a word-weighted algorithm to compare words from the Title and Abstract of each citation.
Indoor Dust as a Matrix for Surveillance of COVID-19.
mSystems, 6(2):e01350-20, 13 Apr 2021
Cited by: 24 articles | PMID: 33850045 | PMCID: PMC8547012
SARS-CoV-2 incidence in secondary schools; the role of national and school-initiated COVID-19 measures.
BMC Public Health, 23(1):1243, 27 Jun 2023
Cited by: 1 article | PMID: 37370045 | PMCID: PMC10294357
Distribution of SARS-CoV-2 RNA signal in a home with COVID-19 positive occupants.
Sci Total Environ, 778:146201, 09 Mar 2021
Cited by: 24 articles | PMID: 34030356 | PMCID: PMC7942153
The role of outdoor and indoor air quality in the spread of SARS-CoV-2: Overview and recommendations by the research group on COVID-19 and particulate matter (RESCOP commission).
Environ Res, 211:113038, 26 Feb 2022
Cited by: 19 articles | PMID: 35231456 | PMCID: PMC8881809
Review Free full text in Europe PMC