Abstract
Free full text

Inhibition of the Early-Stage Cross-Amyloid Aggregation of Amyloid-β and IAPP via EGCG: Insights from Molecular Dynamics Simulations
Abstract
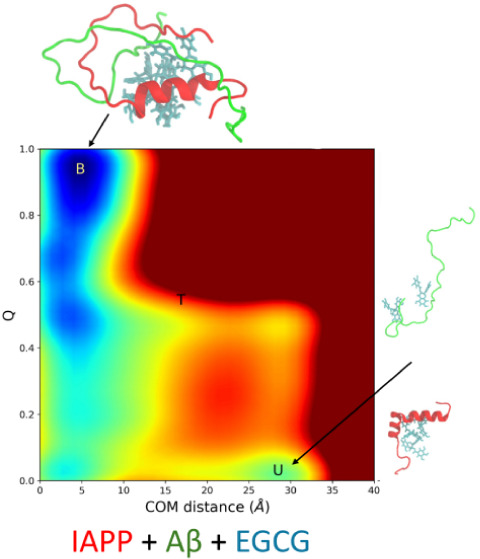
Amyloid-β (Aβ) and islet amyloid polypeptide (IAPP) are small peptides that have the potential to not only self-assemble but also cross-assemble and form cytotoxic amyloid aggregates. Recently, we experimentally investigated the nature of Aβ-IAPP coaggregation and its inhibition by small polyphenolic molecules. Notably, we found that epigallocatechin gallate (EGCG) had the ability to reduce heteroaggregate formation. However, the precise molecular mechanism behind the reduction of heteroaggregates remains unclear. In this study, the dimerization processes of Aβ40 and IAPP peptides with and without EGCG were characterized by the enhanced sampling technique. Our results showed that these amyloid peptides exhibited a tendency to form a stable heterodimer, which represented the first step toward coaggregation. Furthermore, we also found that the EGCG regulated the dimerization process. In the presence of EGCG, well-tempered metadynamics simulation indicated a notable shift in the bound state toward a greater center of mass (COM) distance. Additionally, the presence of EGCG led to a significant increase in the free energy barrier height (~15kBT) along the COM distance, and we observed a transition state between the bound and unbound states. Our findings also unveiled that the EGCG formed a greater number of hydrogen bonds with Aβ40, effectively obstructing the dimer formation. In addition, we carried out microseconds of all-atom conventional molecular dynamics (cMD) simulations to investigate the formation of both hetero- and homo-oligomer states by these peptides. MD simulations illustrated that EGCG played a significant role in preventing oligomer formation by reducing the content of β-sheets in the peptide. Collectively, our results offered valuable insight into the mechanism of cross-amyloid aggregation between Aβ40 and IAPP and the inhibition effect of EGCG on the heteroaggregation process.
Introduction
Misfolded proteins and their aggregation are implicated in more than 30 human diseases, including Alzheimer’s disease (AD) and type 2 diabetes mellitus (T2DM).1,2 AD is the most prevalent form of dementia, and it is projected to impact around 131.5 million global population by 2050.3 Similarly, T2DM, a chronic metabolic syndrome marked by insulin resistance and hyperglycemia, is anticipated to affect the 700 million global population by 2045.4 Both AD and T2DM are classified as protein aggregation diseases. This is due to the amyloid deposition of β-amyloid (Aβ) peptide in the brain in the case of AD, and islet amyloid polypeptide (IAPP) or amylin in the pancreas in the case of T2DM.2,5 Misfolding of Aβ forms oligomers, protofibrils, and mature amyloid fibrils that are cytotoxic.2,5 Moreover, electron microscopy, solid-state nuclear magnetic resonance (NMR), and circular dichroism (CD) show that Aβ1–40 forms fibrils via the conformational change to β-sheets. On the other hand, T2DM involves the amyloid deposition of islet amyloid polypeptide (IAPP) or amylin in the pancreas.2,5 IAPP or amylin is a 37 amino-acid hormone that is cosecreted with insulin from the pancreatic β-cells.6,7 The CD and NMR spectra demonstrate a highly disordered and unfolded structure of monomeric IAPP.8 Epidemiological studies report a strong association between AD and T2DM.9−11 Recent experimental studies suggest that IAPP can access the brain parenchyma from circulation and deposit along with Aβ in the brain tissues of AD patients.12−16
The process of amyloid aggregation follows a nucleation-dependent mechanism characterized by all or none sigmoidal kinetics. It involves an initial nucleation phase followed by rapid elongation and eventual saturation.17,18 Both β-amyloid (Aβ) and IAPP can form amyloid fibrils with similar β-sheets. However, these two peptides exhibit distinct aggregation rates. IAPP tends to aggregate faster with significantly shorter nucleation phases in vitro when compared to Aβ at the same concentration. The lag phase can be shortened or even eliminated by introducing preformed amyloids, a phenomenon known as the seeding effect.
The cross- or coaggregation of Aβ and IAPP has been explored in both in vivo and in vitro studies to uncover the cross-talk between these two devastating diseases. In vivo studies have shown that the injection of IAPP and Aβ fibrils into mice expressing human IAPP resulted in an increased deposition of IAPP in pancreatic tissue, possibly through homologous and heterologous seeding mechanisms.16,19,20 The result also shows a significant increase in pancreatic IAPP and brain Aβ amyloid deposition in a double transgenic mouse model expressing Aβ and IAPP in comparison with mice expressing only human IAPP or only Aβ.19,21In vitro studies have shown that monomeric IAPP and Aβ interact strongly with each other, with binding affinities in the low nanomolar range.22 Recent studies found that the coincubation of IAPP and Aβ at the monomeric and prefibrillar stage leads to the misfolding of both peptides and the formation of cross-amyloid aggregation.23−28 Structural models of both Aβ and IAPP fibrils derived from the experiments, combined with the MD simulations, have provided valuable molecular insights into their cross-interactions.29−31
At the in silico level, a study reported an accelerated coaggregation process of IAPP with Aβ as compared with the aggregation of Aβ alone. Molecular dynamics (MD) simulations showed that binding of IAPP to Aβ resulted in a conformational change of the Aβ segment (16–22) from a helical to an elongated form, which in turn decreased the barrier of aggregation free energy and thus accelerated the coaggregation process.28 In another in silico study, MD simulations were performed on preformed octamer oligomers of IAPP15–35 alone, Aβ15–40 alone, and the heteroassembly of four strands of each peptide (IAPP15–35 and Aβ15–40). Throughout the simulation, all three octamer systems maintained a common structure consisting of a U-shaped structure (β-strand – turn – β-strand) stabilized by the hydrogen bonding network as well as hydrophobic contacts. Within the formed hetero-oligomers, Aβ was found to form a template for the growth of IAPP and vice versa.32 In another in silico study, MD simulations of IAPP-Aβ cross-seeding on cell lipid membranes were performed, indicating possible toxicity mechanisms of IAPP-Aβ coaggregates via the alteration of calcium homeostasis.33 The simulations revealed a strong binding interface between lipids and peptides, driven mainly by electrostatic forces that contribute to the formation of Ca2+ bridges linking peptides to lipids. Conformational changes upon the formation of these heterocomplexes have been observed; however, the precise molecular details and mechanisms underlying the coaggregation of Aβ and IAPP remain elusive. Here, in this study, we address this issue using enhanced sampling techniques (well-tempered metadynamics method) on the dimer level. We find that the system visits a few metastable states when it goes from an unbound state to a bound state.
The discovery of molecules that can inhibit not only the self-aggregation of amyloidogenic proteins but also their coaggregation has been proven to be challenging.34 This is mainly because IAPP and Aβ lack well-defined structures and are highly flexible and dynamic in nature.34 In order for inhibitors to prevent the aggregation of such intrinsically disordered proteins, they should theoretically block the sites of protein–protein interactions.34 Understanding the detailed molecular interactions occurring between these proteins is a prerequisite to the analysis of the inhibitor–peptide interface and to the investigation of how inhibitors can potentially destabilize the binding interfaces and prevent the self-assembly and coassembly processes. Recently, we experimentally studied the nature of IAPP-Aβ40 coaggregation and its inhibition by small molecules. We selected polyphenolic candidates for the inhibition of IAPP or Aβ40 self-aggregation.35 Next, we examined the inhibitory effect of the most potent candidate on IAPP-Aβ40 cross-interaction and aggregation. We found that epigallocatechin gallate (EGCG) reduced heteroaggregate formation. However, the molecular details and mechanism behind the inhibition of heteroaggregate formation remain still unclear. In this study, we performed cMD simulations of IAPP-Aβ40 in the absence and presence of EGCG to elucidate the mechanism by which EGCG inhibits the early stage of IAPP-Aβ40 coaggregation.
Results and Discussion
As we know, the first step of aggregation is the dimer formation. A schematic representation of the simulated heterodimer system in the presence of EGCG is depicted in Figure Figure11. We first analyzed the heterodimer formation of Aβ40 and IAPP in the absence and presence of EGCG. Next, we characterized the hetero-oligomer formation of Aβ40 and IAPP hexamers in the absence and presence of EGCG, with more comprehensive details presented in the forthcoming sections.
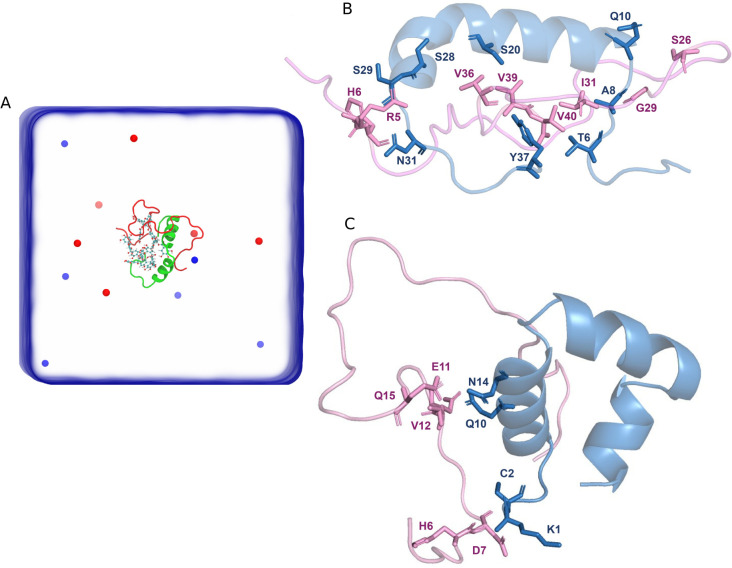
(A) Schematic representation of the heterodimer simulation box containing a monomeric Aβ40 and a monomeric IAPP in the presence of EGCG. EGCG molecules are shown in CPK representation, whereas blue and red spheres represent Na+ and Cl– ions. The heterodimer interface of Aβ40 and IAPP in the absence (B) and presence (C) of EGCG. In both (B) and (C), IAPP (blue) and Aβ40 (pink) are shown as cartoons with the residues of each peptide that form heterodimer interface shown as stick representation.
The Dimerization Process of IAPP-Aβ40 in the Absence and Presence of EGCG
To elucidate the dimerization process of the IAPP-Aβ40, the two-dimensional free energy landscapes (FELs) of the binding process were generated. Figure Figure22 shows the FEL of the binding process projected using the two collective variables (CV1 and CV2). CV1 corresponds to the center of mass (COM) distance of two monomers (Aβ40 and IAPP), and CV2 is the contact number of the residue pairs on the interface of the dimer obtained from our previous equilibrium MD simulations (see Methods section for detail). The two peptide monomers are bound together when they have small COM distances and a large Q value (CV2), whereas these are unbound when they have large COM distances and a small Q value. Based on two CV values, we can divide the FELs into different regions: the bound region, with CV1 < 6 Å and CV2 > 0.7; the unbound region, with CV1 > 25 Å and CV2 < 0.2; and the intermediate region, with 10 Å < CV1 < 20 Å and 0.3 < CV2 < 0.6.
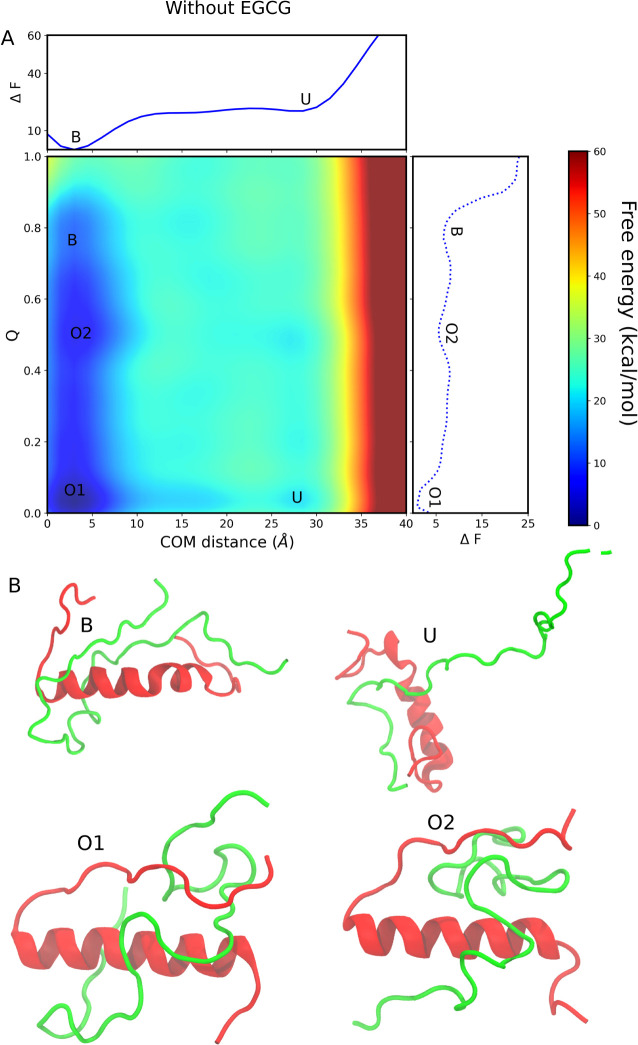
Dimerization process of Aβ40 and IAPP in the absence of EGCG. (A) The binding free energy landscape as a function of center of mass (COM) distance and interchain contacts at the dimer interface. The bound state and unbound state are labeled B and U, respectively. The two off-pathway intermediate states are labeled O1 and O2. The upper abscissa shows the one-dimensional projection of the free energy landscape along the COM distance, whereas the left ordinate shows the one-dimensional projection of the free energy landscape along the Q value. (B) The representative structure of each conformation is observed in the free energy landscape.
As shown in Figure Figure22A, the lowest free energy minimum is located in the bound state, which simply means that both IAPP-Aβ40 monomers prefer to bind with each other. The free energy of the unbound state is higher than the bound state, and we observed no free energy barrier between the bound and unbound states when we project the FEL along the COM distance. The free energy of the unbound state is ~20.55 kcal/mol when the free energy of the bound state is set to zero. The bound state in FEL is observed at a COM distance of ~3.1 Å and a Q value of ~0.76, whereas the unbound state is located at a COM distance of ~29.1 Å and a Q value of 0.1. When projecting the free energy along the COM distance, only two local minima become apparent. However, a two-dimensional reaction coordinate that encompasses both the COM distance and the Q value uncovers multiple metastable states (B, O2, O1, and U). The metastable states observed along the dimerization pathway are named off-pathway states. The orientation of the two monomers (IAPP and Aβ40) in these two states is distinct from those in the bound state.
Figure Figure22B shows the representative structures corresponding to the minimum free energy states observed in the FELs. It can be seen that the orientation of the C-terminal loop is different in both the O1 and O2 states than in the dimer state. In the bound state, a high Q value of 0.76 indicates that most of the residue pairs defined as CV2 (as stated in the Methods section) are present at the heterodimer interface. The dimerization pathway observed in our simulations in the absence of EGCG is defined as follows: first, the system goes to the unbound state, then it goes to the O1 state, after O1 it visits the O2 state, and finally, it reaches the bound state, which is thermodynamically more stable than the other observed states.
The FEL of heterodimer formation in the presence of EGCG was generated using the same CVs (CV1 and CV2) as described previously and is shown in Figure Figure33A. Only CV2, which refers to the contact number of residue pairs at the interface of the dimer, changes in the presence of EGCG (see Methods section for detail). The FEL in the presence of EGCG was also divided into three regions: the bound region, the intermediate region, and the unbound region. We observed a transition state between the bound and unbound regions. The metastable states O1 and O2 observed in the absence of EGCG are absent in the presence of EGCG, but a new intermediate state (high energy state) has emerged. The bound state in FEL is observed at a COM distance of ~5.2 Å and the Q value is ~0.9, while the unbound state is located at a COM distance of ~29.1 Å and the Q value is 0.06. In both systems (with and without EGCG), we found that the minimum free energy state corresponds to the bound state, but the free energy minima corresponding to the COM distance are shifted to a higher value in the presence of EGCG. This observation is probably due to the effect of EGCG in preventing the two monomers from approaching each other. The free energy of the unbound state is higher than that of the bound state, and there is a transition state between the bound state and the unbound state. The free energies of the unbound state and the transition state are 29.4 and 38.9 kcal/mol, respectively, while the free energy of the bound state is set to zero. Based on the free energy landscape, we conclude that the dimerization in the presence of EGCG is a two-step process. From the unbound state, the system must overcome the free energy barrier of the transition state. Once it has overcome the energy barrier, it goes very rapidly into the bound state as indicated by a steep slope. The free energy barrier between the unbound state and the transition state is 9.5 kcal/mol.
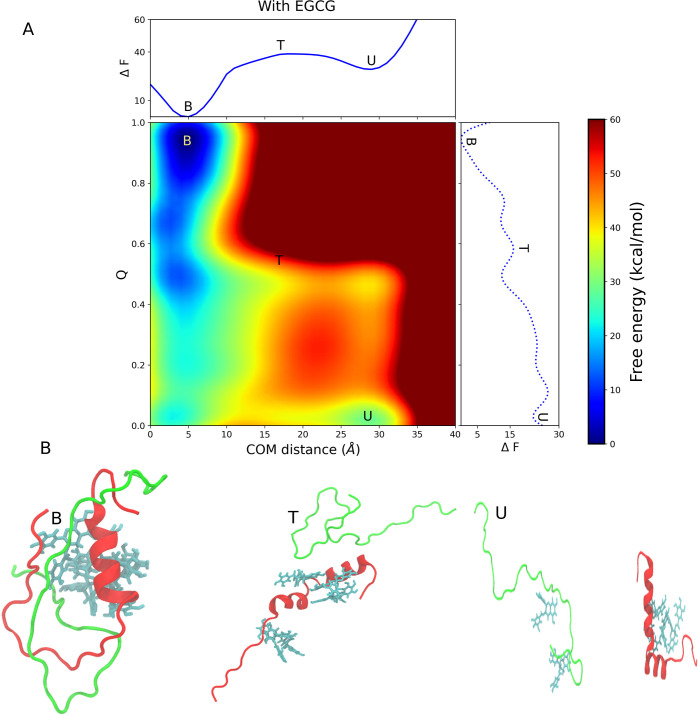
Dimerization process of Aβ40 and IAPP in the presence of EGCG. (A) The binding free energy landscape as a function of center of mass (COM) distance and interchain contacts at the dimer interface. The bound state, unbound state, and transition state are labeled B, U, and T, respectively. The upper abscissa shows the one-dimensional projection of the free energy landscape along the COM distance, whereas the left ordinate shows the one-dimensional projection of the free energy landscape along the Q value. (B) The representative structure of each conformation is observed in the free energy landscape.
The two major minima on the free energy landscape curve indicate that the dimerization of IAPP-Aβ40 in the presence of EGCG is a two-state process, whereas in the absence of EGCG, we observed some metastable states. In the presence of EGCG, the system should overcome a much higher free energy barrier from the unbound state to the bound state than in the absence of the EGCG system (9.5 kcal/mol vs 0 kcal/mol). The result clearly indicates a slower transition between the unbound and bound states in the presence of EGCG. We found that EGCG does not completely block dimer formation but slows the dimerization process. Figure Figure33B shows the representative structures corresponding to the minimum free energy states observed in the FELs. In the bound state, EGCG binds to both monomers and prevents them from approaching each other, whereas in the transition state, all EGCG molecules bind to IAPP. The dimerization pathway observed in our simulations in the presence of EGCG is defined as follows: first, the system enters the unbound state, then it overcomes the free energy barrier and visits the transition state, and finally, it reaches the bound state.
In our simulation, both systems exhibit the bound state. EGCG does not completely inhibit dimer formation but slows down the dimerization process by increasing the free energy barrier between the unbound and bound states. It also shifts the global minima at a higher COM distance. In addition, we experimentally reported in our previous work the inhibition of coaggregation by EGCG.35 Therefore, to explore the precise mechanism of the inhibition of cross-aggregation, we performed the MD simulations for the hexamer system (i.e., at the oligomeric level), which we will discuss in the following sections.
The Inter-residue Distance between IAPP-Aβ40 Dimer Systems in the Absence and Presence of EGCG
To get an overview of how the two peptides are arranged with respect to each other as dimers both with EGCG and without EGCG, we calculated the interpeptide distance on a residue-to-residue basis. The resulting interpeptide distance matrices for both systems are shown in Figure Figure44. Figure Figure44A demonstrates, in the absence of EGCG, IAPP residues, 8–20, 22, 25–30, to have high contact density with Aβ40 residues, 22–37. Our data show that IAPP residues that are in close contact with Aβ40 span different regions of IAPP including the middle region (residues 8–20) and the amyloidogenic region (residues 20–29). As for Aβ40, its identified residues that are in close contact with IAPP are mainly located in its core and C-terminus regions (22–37). These regions, particularly the sequence 29–40 of Aβ40, are previously shown in the literature to be highly aggregation-prone for amyloid formation.36,37 Additionally, the IAPP region (20–29) has been reported in studies as important in controlling amyloid formation.38−40 In fact, the rat IAPP sequence differs from that of humans at six positions, five of which are located in the region (20–29). Three proline substitutions at positions 25, 28, and 29 prevent the rat IAPP from forming a β-sheet amyloid structure.38−40 Our data are in agreement with previous experimental studies that have reported the hot regions of IAPP (8–18 and 22–28) and Aβ40 (19–22, 27–32, and 35–40) to mediate both self-interaction and cross-interaction between the two peptides.22 The IAPP and Aβ40 residues identified in our distance matrices are largely within the reported hot regions. Understanding the detailed molecular interactions between IAPP and Aβ40 in the absence of EGCG is required to analyze the effect of the inhibitor EGCG on the protein–protein interaction interface.34
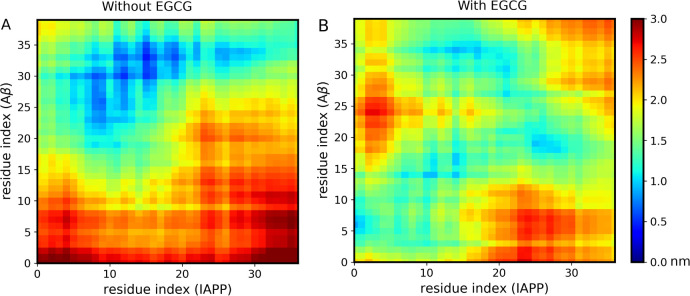
Distance matrices illustrating inter-residue contacts between Aβ40 and IAPP peptides in the absence of EGCG (A) and in the presence of EGCG (B). The inter-residue contacts are shown for Aβ40 and IAPP peptides for the whole simulation time. The color bar on the right indicates average inter-residue distances (in nanometers).
On the other hand, in the presence of EGCG, the interpeptide distance between almost all residues of the two peptides increased (Figure Figure44B). This clearly demonstrates that EGCG disrupts the peptides when they come into close proximity, consistent with our free energy results. In both peptides, the N-termini and a few residues of the core regions are between 0.5 and 1 nm apart from each other, while the amyloidogenic regions of both peptides are not at a distance that permits their interactions. Only IAPP residues (11–17, 21, and 25–28) and Aβ40 residues (14–21, 27, 31, and 34) are between 0.5 and 1 nm apart from each other. These distances are above the required threshold for hydrogen bond formation. Importantly, the bound system in the presence of EGCG does not show the aggregation-prone residues of each peptide to be close enough to each other as they are in the bound state in the absence of EGCG. This finding indicates that residues forming the heterodimer interface in the absence of the inhibitor are unable to interact with each other in the presence of the inhibitor. The bound state presented earlier in the presence of EGCG (Figure Figure33) has a center of mass distance of ~5.2 Å; however, the bound state does not imply that IAPP and Aβ40 residues are at a distance that permits their interactions to form close polar and hydrophobic contacts at the interface. In addition, we analyzed the inhibitor–peptide interactions by computing inter-residue distance maps (Figure S1). These data enable us to further investigate how EGCG can potentially destabilize the binding interface and prevent the cross-interaction process. It can be noticed that four EGCG molecules are in close proximity to Aβ40 residues from the core region (9–15) and C-termini residues (30–35) with distances of around 0.5 nm. While for IAPP, only two EGCG molecules are in close proximity with the IAPP residues from the core (10, 11, and 14 at a distance of around 0.5 nm) and amyloidogenic regions (25–31 at a distance of around 1 nm).
Interpeptide Hydrogen Bonds between IAPP and Aβ40 in the Absence and Presence of EGCG
To investigate the effect of EGCG on modulating IAPP-Aβ40 cross-interaction, we analyzed the interpeptide hydrogen bonds (H-bonds) during dimer simulations. The interpeptide H-bonds are defined as the hydrogen bonds formed between Aβ40 and IAPP monomers. The GROMACS hbond tool41 was used to calculate the presence of the H-bonds using a threshold distance of 3.5 Å and a cutoff angle of 30°. Figure S2 shows the time evolution of interpeptide H-bonds both in the presence and absence of EGCG. Our result showed that the number of interpeptide H-bonds varied throughout the simulation between the two dimer systems. Notably, in the absence of EGCG, the average number of interpeptide H-bonds is 5.40, whereas in the presence of EGCG, the average number of H-bonds is 4.2. The low number of interpeptide H-bonds in the presence of EGCG clearly indicates that EGCG modulates the cross-interaction at the dimer level. To elucidate whether EGCG binds any monomers, we also calculated the H-bonds between the EGCG molecule and Aβ40 or IAPP. The time evolution of H-bond formation between each EGCG molecule and Aβ40 and IAPP is shown in Figure Figure55A,B, respectively. Figure Figure55C,D illustrates the schematic representation of the H-bonds formed between EGCG and Aβ40, as well as the IAPP. The average number of H-bonds formed between each EGCG molecule and Aβ40 peptide is 1.02, whereas for IAPP the average number of H-bonds is 0.415. Our data demonstrate that EGCG forms more H-bonds with Aβ40 than with IAPP throughout the simulation time. Moreover, the interaction with both peptides may indicate an interference effect of EGCG against the formation of IAPP-Aβ40 heterodimer.
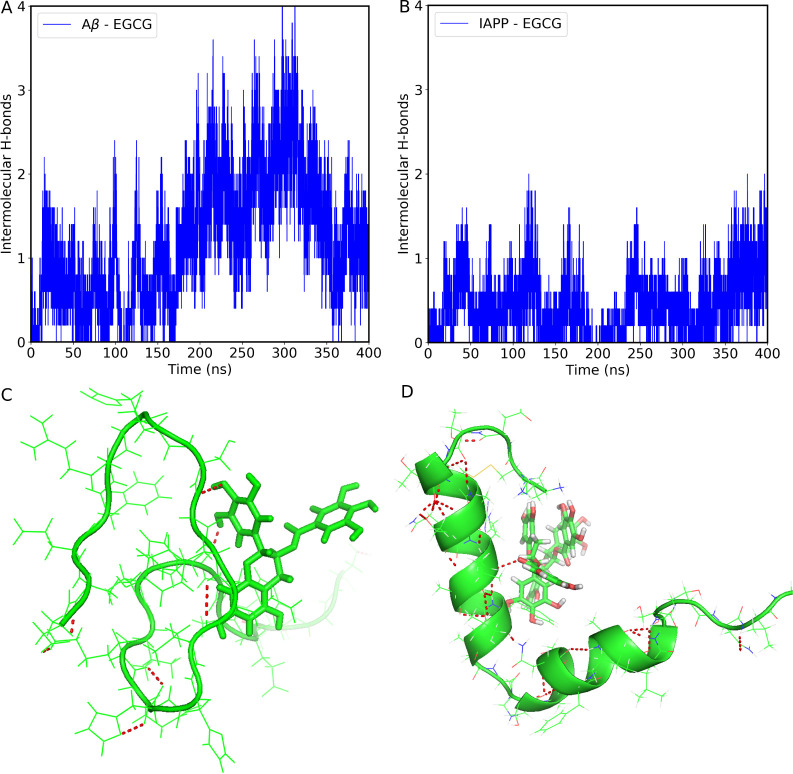
Time evolution of intermolecular hydrogen bonds formed between each EGCG molecule and Aβ40 (A) and IAPP (B) in the heterodimer system. Schematic representation of H-bond between Aβ40-EGCG (C) and IAPP-EGCG (D). The EGCG molecule was shown in stick representation. The red dotted lines show the H-bonds.
Homo- and Hetero-Oligomerization of IAPP and Aβ40 in the Absence and Presence of EGCG
To understand the early steps of IAPP and Aβ40 self- and coaggregation, we performed cMD simulations of homo- and heterohexamer systems. For homosystems, the oligomerization simulation of six IAPP monomers (Figure Figure66A, red dotted line) shows an immediate formation of IAPP tetramers during the first 200 ns, followed by the formation of pentamer oligomers until 580 ns. The stable formation of IAPP homohexamers is observed from 580 ns until the end of the simulation time. As with the Aβ40 homosystem (Figure Figure66A, blue solid line), the Aβ40 monomers form pentamer oligomers after the first 50 ns, which fluctuate slightly until 750 ns, where a stable Aβ40 homohexamer forms. Our MD simulations show that stable homohexamers form earlier for IAPP than for Aβ40. This observation is consistent with previous experimental results showing shorter lag phases and faster self-aggregation kinetics of IAPP compared to Aβ40.24,28,35
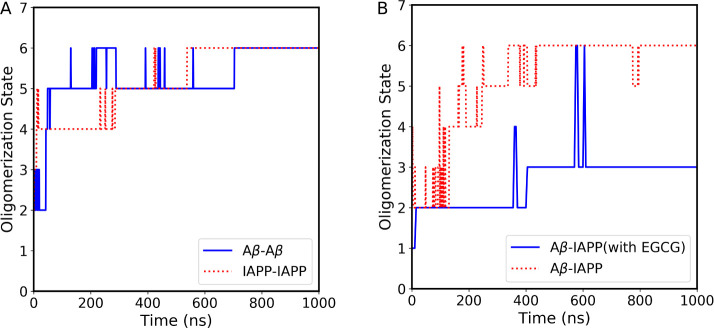
(A) The homo-oligomerization states of the Aβ40 and IAPP systems over simulation time. The blue solid line corresponds to the Aβ40 system, whereas the red dotted lines correspond to the IAPP system. (B) The hetero-oligomerization states of the Aβ40 and IAPP systems in the presence and absence of EGCG over simulation time. The blue solid line corresponds to the heteroaggregate in the presence of EGCG, whereas the red dotted line corresponds to heteroaggregate forms in the absence of EGCG.
In contrast to homo-oligomerization, hetero-oligomerization of three IAPP and three Aβ40 monomers results in a different oligomerization pathway (Figure Figure66B, the red dotted line). The IAPP-Aβ40 heterosystem shows an early formation of heterotrimers lasting about 180 ns, followed by a fluctuation of tetramer and pentamer oligomers until 420 ns, after which stable heterohexamers are formed until the end of the simulation time. Interestingly, in the presence of EGCG (Figure Figure66B, the blue solid line), the IAPP and Aβ40 monomers formed only dimers in the first 400 ns, followed by trimer formation with the transient formation of hexamers. Our MD results decipher the molecular mechanism by which EGCG molecules prevent the early steps of IAPP-Aβ40 co-oligomerization, as indicated by the inability of IAPP and Aβ40 monomers to form stable hexamers in the presence of EGCG.
To further elucidate the inhibition mechanism of EGCG at the early stages of IAPP and Aβ40 coaggregation, the heterohexamer systems were analyzed for the number of atomic contacts between IAPP and Aβ40 residues in the absence and presence of EGCG over the entire simulation time (Figure Figure77). The results of atomic contacts clearly demonstrate the lower number (between 1.3- and 2.5-fold decrease) of atomic contacts between IAPP and Aβ40 in the presence of EGCG as compared to the system simulated in the absence of EGCG. The results suggest the effect of EGCG molecules in interfering with the interactions occurring between the IAPP and Aβ40 heterohexamers throughout the simulation time. Our current MD results support the inhibitory role of EGCG against IAPP-Aβ40 coaggregation, in agreement with our previous experimental data.35 Notably, in our previous work, we investigated the effect of EGCG on the isolated Aβ monomer or IAPP monomer systems as well as the mixed IAPP-Aβ heterosystem. Using thioflavin-T fluorescence assay and transmission electron microscopy, we investigated the role of EGCG (along with 5 potential polyphenolic candidates) in preventing Aβ40 and IAPP self-aggregation. Our results demonstrated that EGCG was highly and similarly effective in reducing the self-aggregation of Aβ40 (by 67.5%) as well as IAPP (77.6%). While the effect of EGCG on Aβ40 is well addressed in the literature, several studies in the literature have also reported the inhibitory effect of EGCG on IAPP aggregation. Studies have shown that EGCG efficiently inhibited IAPP aggregation and protected the rat β-cells from the toxic effect of IAPP fibrils42,43 Given that ECGC was the most potent candidate in our experimental screening, the current study was devoted to elaborate on its mechanistic role in preventing the IAPP-Aβ40 coaggregation systems in silico.
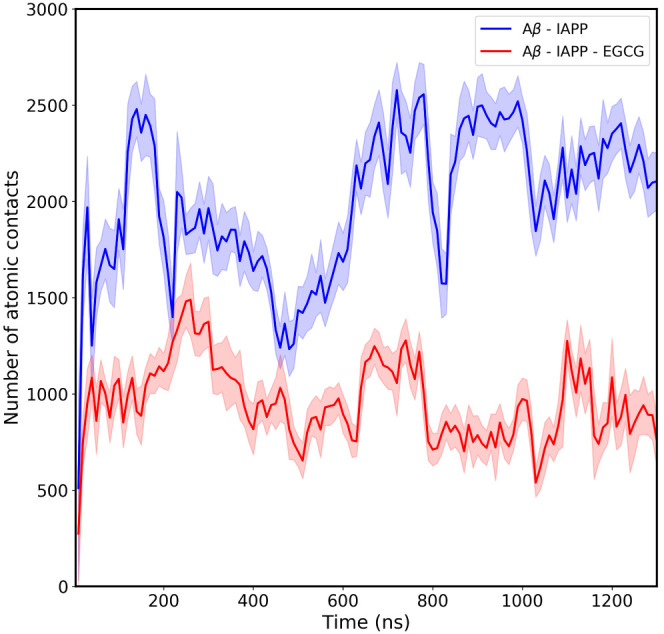
Time evolution of atomic contact between the Aβ40 and IAPP peptides in the absence and the presence of EGCG molecules. The blue solid line corresponds to the atomic contacts between Aβ40 and IAPP in the absence of EGCG, whereas the red solid line corresponds to the atomic contacts between Aβ40 and IAPP in the presence of EGCG. The shaded region shows the error bars.
Secondary Structure Changes during the Processes of Homo- and Hetero-Oligomerization of IAPP and Aβ40 in the Absence and Presence of EGCG
The secondary structural analyses of the homohexamer and heterohexamer systems were performed by calculating the probabilities per residue for each secondary structure element, α-helices, β-sheets, and turns over the entire simulation time (1000 ns) and for all six monomers in each system. Examination of the Aβ40-homohexamer system (Figure Figure88A) reveals that most Aβ40 residues, particularly core-stretch and C-terminus, form predominantly turn structures and β-sheets. These results are consistent with the expected intrinsically disordered nature of Aβ40 monomers and their tendency to form a hairpin structure consisting of turns and β-sheets.44 In fact, Aβ oligomers have been shown to exhibit compact twisted conformations known as beta-hairpins. The crystallographic structures of Aβ oligomers were revealed using β-hairpin mimics, which had the tendency to assemble into trimers and higher-order hexamers and dodecamers via hydrophobic and exposed hydrogen bonds.44 On the other hand, secondary structure analysis of the IAPP homohexamer system shows that most IAPP residues tend to form turns and α-helices and have little probability of forming a β-sheet (Figure Figure88B). Our observation of the involvement of α-helices and turns in the self-oligomerization of IAPP can be explained by previous studies that showed the importance of α-helices over β-sheets in the early stages of IAPP self-assembly and oligomerization.45 Using Raman and infrared spectroscopy, IAPP oligomers were found to have a higher content of α-helices as compared with the mature IAPP fibrils that are rich in β-sheets.46
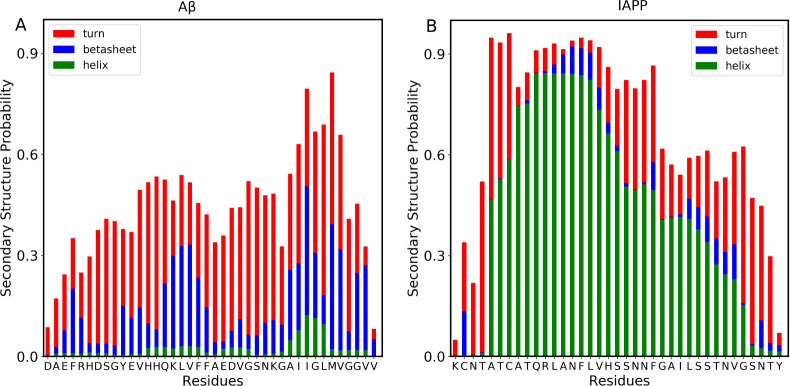
Secondary structural characterization of homo-oligomerization states. Probability of secondary structures to form in each residue of the Aβ40 (A) and IAPP (B) peptides. The bars represent the cumulative secondary structure probabilities consisting of helix (green color), β-strand/bridge (blue color), and turn or bend (red color). The difference from 1.0 presents the probability of the random coil state.
Secondary structure analysis of the heterohexamer system shows that both IAPP and Aβ40 monomers have overall similar secondary structural elements as their homosystems (Figure Figure99). However, IAPP residues in the early core region (7–15) have a higher content of α-helices in the heterosystem than in the homosystem. Also, IAPP residues in the C-termini regions (28–37) exhibit a higher tendency to form turn structures in the heterosystem than in the homosystem. Having higher turn structures in IAPP residues 28–37 may indicate their role in interacting with Aβ40 to form early oligomeric species. Interestingly, in the presence of EGCG (Figure Figure99B), IAPP-Aβ40 heterohexamers exhibit a lower tendency toward β-sheets in the Aβ40 residues and a higher tendency toward α-helices in the IAPP residues (especially in the amyloidogenic region of IAPP). The presence of the key amyloidogenic residues of IAPP in an α-helix rather than in the turn structure in the presence of EGCG may indicate the effect of EGCG in maintaining the structured helical folding of IAPP, protecting its residues from interaction with Aβ40, as we have previously shown in inter-residue distance data in Figure Figure44.
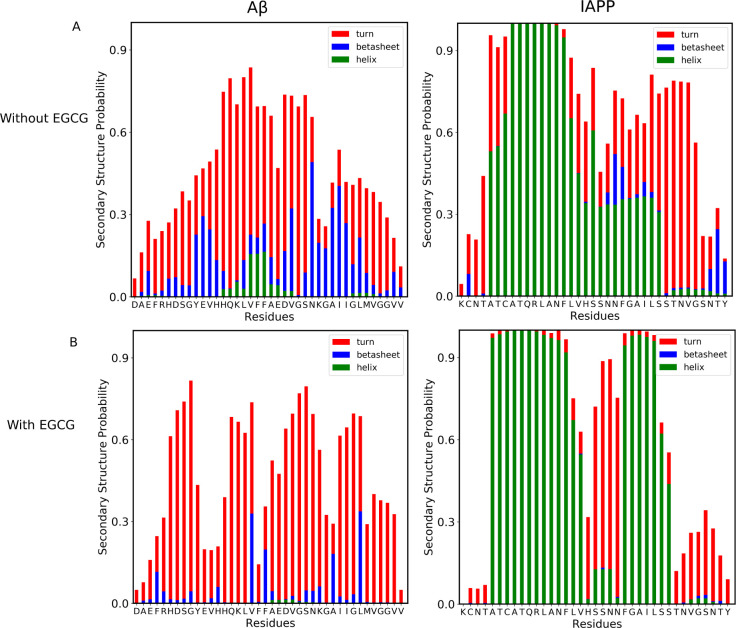
Secondary structural characterization of hetero-oligomerization states. Probability of secondary structures to form in each residue of the Aβ and IAPP peptides in the absence of EGCG (A), and in the presence of EGCG (B). The bars represent the cumulative secondary structure probabilities consisting of helix (green color), β-strand/bridge (blue color), and turn or bend (red color). The difference from 1.0 presents the probability of the random coil state.
Conclusion
In recent years, significant research encompassing in vitro, in vivo, and as well as computational in silico studies unveiled a strong association between AD and T2DM. Notably, individuals diagnosed with AD face an increased risk of developing T2DM, while those with T2DM are prone to developing AD. The precise mechanism behind this association is still ambiguous, but recent studies suggest that peptides central to each of these diseases play a pivotal role in this association. Recently, we experimentally investigated the nature of IAPP-Aβ40 coaggregation and its inhibition by small polyphenolic molecules. Notably, we found that EGCG had the ability to reduce heteroaggregate formation. EGCG is an antioxidant polyphenolic small molecule that has wide preclinical applications in many human diseases including its use as an inhibitor of self-aggregation of different pathogenic proteins such as Aβ and IAPP.35 In our previous work, we also demonstrated the protective effect of EGCG on two cell lines (neuronal and pancreatic cell models) against the cytotoxicity of IAPP–Aβ40 heteroaggregates. The in vitro cell viability rates of PC-12 cells and RIN-m5F cells were reduced when treated with IAPP-Aβ40 heteroaggregates (without EGCG addition), whereas cells treated with IAPP-Aβ40 heteroaggregates in the presence of EGCG had higher viability rates indicating the inhibitory role of EGCG against the cytotoxicity of IAPP-Aβ40 heteroaggregates. Importantly, we found the highest reduction of cytotoxicity of IAPP-Aβ40 heteroaggregates on PC-12 and RIN-m5F cell models, specifically at EGCG concentrations, which are 2.5-fold higher than the mixed peptide concentrations.35
To gain insights into the dimerization process of IAPP and Aβ40, which is the first step of coaggregation, both in the absence and presence of EGCG, we employed advanced sampling techniques, specifically the well-tempered metadynamics method. WTMD simulations enable us to characterize the free energy landscape and identify metastable states involved in the dimerization process with and without EGCG. In both cases, the lowest free energy state on the FEL corresponds to the bound state, indicating a significant interaction between the two peptides. However, there is a notable distinction between the two cases. In the absence of EGCG, the minimum energy state corresponds to a COM distance of 3.1 Å, indicating a close proximity of two peptides. In contrast, in the presence of EGCG, the minima correspond to a COM distance of 5.2 Å, which exceeds the threshold value for polar interaction. In essence, our findings suggest that without EGCG, the system radially forms a stable dimer, while the presence of EGCG prevents the dimerization process, leading to a bound state but not the formation of a stable dimer.
Furthermore, we examined the formation of interpeptide H-bonds and found that in the absence of EGCG, IAPP-Aβ40 exhibited a higher number of H-bonds, which aligns with our argument. However, this raised the question of why, in the presence of EGCG, the system does not form the dimer. To address this, we investigated the intermolecular H-bonds between EGCG and the peptides and observed that EGCG indeed forms H-bonds with both peptides. As a result, when the two peptides come into close proximity, the presence of EGCG obstructs the dimer formation process. Supporting this observation, we provided data on the distances between residues in both cases, indicating that regions of the peptides that were close in the absence of EGCG moved significantly apart in the presence of EGCG. Additionally, we looked at the dimerization pathways, finding multiple metastable states between the bound and unbound states in the absence of EGCG, whereas with EGCG, we observed a high energy transition state between these states.
We also investigated the homo- and hetero-oligomerization of IAPP and Aβ40 in both the absence and presence of EGCG, aiming to elucidate the mechanism of cross-amyloid inhibition using long cMD simulations. Our results revealed that IAPP aggregated at a faster rate compared to Aβ40, which aligned with experimental findings. Without EGCG, Aβ40 and IAPP coaggregated to form hexamers, while in the presence of EGCG, they never coaggregated as hexamers during the whole simulation period. Analysis of the secondary structure revealed that EGCG inhibited coaggregation by modulating the secondary structure of both peptides. In summary, this study offers valuable insights into the mechanism by which EGCG inhibits coaggregation, shedding light on the complex interplay between these peptides.
Methods
Systems
To understand the inhibitory role of EGCG against IAPP-Aβ40 cross-interaction, molecular dynamics simulations have been performed with and without EGCG. Aβ40 and Aβ42 are the two major isoforms of the β-amyloid peptides and are both elevated in the brain of Alzheimer’s disease patients.47 Aβ42 is more amyloidogenic and toxic with respect to Aβ40, and the higher abundance of Aβ40 in body fluids, with a ratio of 9:1 (Aβ40:Aβ42), is the reason for adopting Aβ0 in our MD simulation. The two systems were modeled accordingly: the first system contains one Aβ40 (Residue: 1–40) monomer and one IAPP (Residue: 1–37) monomer, and the second system contains one Aβ monomer, one IAPP monomer, and five EGCG molecules. The initial structures of Aβ40 (PDBID: 2LFM)48 and IAPP (PDBID: 2L86)49 were obtained from the Protein Data Bank. The IAPP structure was resolved for the native amidated IAPP at a physiological pH and with a disulfide bridge between residues Cys2–Cys7. EGCG structure was built from its canonical SMILES obtained from the PubChem database. Since the reported PDB structures were resolved in the multimeric form, therefore, in this study, we have taken one monomeric structure for each peptide. We simulated the monomeric peptides starting from the initial structure and solvated them with a cubic box. We run these simulations for 500 ns. For the heterodimer simulations, the representative Aβ40 and IAPP monomer structures were determined using the GROMACS clustering tool of Daura et al.50 with a cutoff of the root-mean-square deviation (RMSD) of 0.2 nm. All-atom MD simulations were performed using the GROMACS package v5.1.4,41 CHARMM36m force field51 with the TIP3P water model.52 To mimic the experimental condition, we added 100 mM NaCl to the system.
For heterodimer
formation simulations, we placed the two monomers that correspond
to Aβ40 and IAPP peptides randomly using the PACKMOL.53 The two peptides were placed at least 1.2 nm
distance between them in a cubic simulation box with a distance of
10 Å from the box walls. Ions were added to neutralize the system
as well as mimic the experimental conditions. We capped the N- and C-terminals of the peptides with acetyl (ACE) and N-methyl amide (NME) groups, respectively, to mimic the
experimental conditions. Before performing the well-tempered metadynamics
(WTMD) simulations,54 the systems were
energy-minimized through the steepest descent method for the 10000
steps to remove the bad contacts that may arise due to the random
placement of water and ions. Periodic boundary conditions were applied
in all three directions. For neighbor search, a cutoff of 10 Å
was used. The neighbor list for the nonbonded pairs was updated every
40 steps. The particle mesh Ewald summation method55 was used to calculate the long-range electrostatic interactions
with a grid spacing of 0.16 nm and interpolation of order 4. SETTLE56 and LINCS57 algorithms
were used to constrain the covalent bonds of the system to their equilibrium
properties. The temperature was set to 310 K with a V-rescale thermostat.58 The pressure was retained at 1 bar using a Perrinello-Rahman
barostat59 and an isotropic scheme. After
minimization, each system was heated up from 0 to 310 K in the NVT
ensemble for 10 ns, followed by another 10 ns equilibration in the
NPT ensemble.
Well-Tempered Metadynamics Simulations
The WTMD simulations were performed with the GROMACS package v5.1.441 and PLUMED v2.4.60 The first collective variable of our metadynamics simulation was defined as the distance between the center of mass (COM) of the two peptides. The second collective variable (CV2) of our simulations was defined as the contact number of the typical residue pairs that lie on the dimer interface. We used our 700 ns equilibrium simulation presented in our previous work35 to extract the residue pairs located at the dimer interface. Briefly, in the absence of EGCG, the heterodimer interface forms between the Aβ40 residues (R5, H6, S26, G29, I31, V36, V39, V40) and the IAPP residues (T6, A8, Q10, S20, S28, S29, N31, Y37) that form polar interactions at the heterodimer interface. While in the presence of EGCG, the heterodimer interface is formed between Aβ40 residues (H6, D7, E11, V12, Q15) and IAPP residues (K1, C2, Q10, N14).
Figure Figure11B,C shows the residue pairs at the dimer interface in the presence and absence of EGCG. The Gaussian height was set as 0.5 kJ/mol and deposited every 1 ps with a gradually decreasing bias factor of 32.0. The Gaussian widths were set to 0.6 for both CV1 and CV2. WTMD simulations were performed for 400 ns with the time step set to 2 fs. The fluctuations of the CVs were monitored and the convergence of the simulation was assessed by evaluating the magnitude of Gaussian height. The sampling was considered converged when the Gaussian height dropped and reached a threshold value (Figure S3). We used Gaussian height <0.006 kJ/mol as a threshold value.
Hexamer Simulations
To study the underlying mechanism of both the homo- and heteroaggregation (oligomer formation) and how the EGCG inhibits the heteroaggregation, we introduced six peptides into a cubic box with a 10 nm edge length. For the homosystem, all six peptides correspond to the same peptide, whereas for the heterosystem, three peptides correspond to each system. We used the same force field and water model, i.e., CHARMM36m51 and TIP3P,52 to study the aggregation behavior of the peptides. The initial configuration for these simulations was generated with the PACKMOL53 program, using the most populated peptide structure observed in the monomer simulations. We positioned the monomer in such a way that the monomer–monomer distance was smaller than 1.2 nm. We followed the same simulation protocol as discussed in the Well-Tempered Metadynamics Simulations section. Each hexamer simulation was performed for 1 μs with the time step set to 4 fs.
Analysis
Structural analysis The simulation trajectories were analyzed using a combination of GROMACS tools, in-house Python scripts invoking the MDAnalysis,61 and MDTraj libraries.62 To identify the inter- and intrapeptide contacts, we considered two residues to be in contact, if the distance between any pair of atoms of the residues is less than or equal to 0.4 nm. Using the same distance cutoff, we determined the size of oligomers. For the H-bonds, we used the following criteria: the distance between the donor and the acceptor is d ≤ 3.5 Å, and the angle between the donor and the acceptor is <30°. The results were plotted using the matplotlib v3.3.263 The structural images were drawn using the visual molecular dynamics (VMD)64 and PyMOL.65
Free energy landscape The free energy landscapes (FELs) are plotted as a function of the probability distribution.

where kB is the Boltzmann constant and T is the temperature. Pmax is the maximum probability that corresponds to ΔF = 0.
In our WTMD simulations, the probability distribution of conformational states was computed along the two reaction coordinates: the center of mass distance between the Aβ40 and IAPP monomer-formed dimers; and the fraction of the native contacts (Q) calculated using the equation:66

where the sum runs over the N atom pairs, rij(Ω)
is the distance between the i and j atom pair in configuration Ω, is the distance between the
heavy atoms i and j from the experimental
crystal structure.
β is the smoothing parameter taken to be 5 Å–1, and the factor λ accounts for the fluctuations when the contact
is formed, taken to be 1.8. Eight residue pairs were selected to characterize
the binding process in the absence of an inhibitor (EGCG), whereas
six residue pairs were chosen in the presence of an inhibitor. Therefore, N = 6 and 8 were chosen for both cases to calculate the
fraction of native contacts (Q) and plot the free
energy landscape.
Acknowledgments
This work was supported by the Khalifa University of Science and Technology under award No. [CIRA-2020-01] and the Abu Dhabi Award for Research Excellence (AARE) 2019 (Project Contract No. AARE19-202). The MD simulations were carried out using the high-performance computing (HPC) facility supported by the Khalifa University of Science and Technology, Abu Dhabi, UAE.
Supporting Information Available
The Supporting Information is available free of charge at https://pubs.acs.org/doi/10.1021/acsomega.4c00500
Distance matrices between EGCG molecule and Aβ40 and IAPP peptides; time evolution of interpeptide hydrogen bonds formed between Aβ40 and IAPP in the absence and presence of EGCG; convergence test plot. The initial and last frame of both metadynamics and cMD simulations for all systems, the representative structures of all intermediate states, and the input files for the metadynamics simulations and cMD simulations are available at Zenodo data repository (https://zenodo.org/records/10478034) (PDF)
References
- Knowles T. P.; Vendruscolo M.; Dobson C. M. The amyloid state and its association with protein misfolding diseases. Nat. Rev. Mol. Cell Biol. 2014, 15, 384–396. 10.1038/nrm3810. [Abstract] [CrossRef] [Google Scholar]
- Chiti F.; Dobson C. M. Protein misfolding, functional amyloid, and human disease. Annu. Rev. Biochem. 2006, 75, 333–366. 10.1146/annurev.biochem.75.101304.123901. [Abstract] [CrossRef] [Google Scholar]
- Prince M.; Comas-Herrera A.; Knapp M.; Guerchet M.; Karagiannidou M.. World Alzheimer Report: Improving healthcare for people living with dementia: Coverage, Quality and costs now and in the future Doctoral dissertation, 2016. [Google Scholar]
- DeFronzo R. A.; Ferrannini E.; Groop L.; Henry R. R.; Herman W. H.; Holst J. J.; Hu F. B.; Kahn C. R.; Raz I.; Shulman G. I.; Simonson D. C.; et al. Type 2 diabetes mellitus. Nat. Rev. Dis. Primers 2015, 1, 15019.10.1038/nrdp.2015.19. [Abstract] [CrossRef] [Google Scholar]
- Mukherjee A.; Morales-Scheihing D.; Butler P. C.; Soto C. Type 2 diabetes as a protein misfolding disease. Trends Mol. Med. 2015, 21, 439–449. 10.1016/j.molmed.2015.04.005. [Europe PMC free article] [Abstract] [CrossRef] [Google Scholar]
- Toshimori H.; Narita R.; Nakazato M.; Asai J.; Mitsukawa T.; Kangawa K.; Matsuo H.; Takahashi K.; Matsukura S. Islet amyloid polypeptide in insulinoma and in the islets of the pancreas of non-diabetic and diabetic subjects. Virchows Archiv A 1991, 418, 411–417. 10.1007/BF01605927. [Abstract] [CrossRef] [Google Scholar]
- Lukinius A.; Wilander E.; Westermark G.; Engström U.; Westermark P. Co-localization of islet amyloid polypeptide and insulin in the B cell secretory granules of the human pancreatic islets. Diabetologia 1989, 32, 240–244. 10.1007/BF00285291. [Abstract] [CrossRef] [Google Scholar]
- Akter R.; Cao P.; Noor H.; Ridgway Z.; Tu L.-H.; Wang H.; Wong A. G.; Zhang X.; Abedini A.; Schmidt A. M.; Raleigh D. P.; et al. Islet amyloid polypeptide: Structure, function, and pathophysiology. J. Diabetes Res. 2016, 2016, 2798269.10.1155/2016/2798269. [Europe PMC free article] [Abstract] [CrossRef] [Google Scholar]
- Cukierman T.; Gerstein H.; Williamson J. Cognitive decline and dementia in diabetes—systematic overview of prospective observational studies. Diabetologia 2005, 48, 2460–2469. 10.1007/s00125-005-0023-4. [Abstract] [CrossRef] [Google Scholar]
- Biessels G. J.; Staekenborg S.; Brunner E.; Brayne C.; Scheltens P. Risk of dementia in diabetes mellitus: A systematic review. Lancet Neurol. 2006, 5, 64–74. 10.1016/S1474-4422(05)70284-2. [Abstract] [CrossRef] [Google Scholar]
- Janson J.; Laedtke T.; Parisi J. E.; O’Brien P.; Petersen R. C.; Butler P. C. Increased risk of type 2 diabetes in Alzheimer disease. Diabetes 2004, 53, 474–481. 10.2337/diabetes.53.2.474. [Abstract] [CrossRef] [Google Scholar]
- Jackson K.; Barisone G. A.; Diaz E.; Jin L.-W.; DeCarli C.; Despa F. Amylin deposition in the brain: A second amyloid in Alzheimer disease?. Ann. Neurol. 2013, 74, 517–526. 10.1002/ana.23956. [Europe PMC free article] [Abstract] [CrossRef] [Google Scholar]
- Martinez-Valbuena I.; Valenti-Azcarate R.; Amat-Villegas I.; Riverol M.; Marcilla I.; de Andrea C. E.; Sánchez-Arias J. A.; Marti G.; Erro M.-E.; et al. Amylin as a potential link between type 2 diabetes and alzheimer disease. Ann. Neurol. 2019, 86, 539–551. 10.1002/ana.25570. [Abstract] [CrossRef] [Google Scholar]
- Banks W. A.; Kastin A. J.; Maness L. M.; Huang W.; Jaspan J. B. Permeability of the blood-brain barrier to amylin. Life Sci. 1995, 57, 1993–2001. 10.1016/0024-3205(95)02197-Q. [Abstract] [CrossRef] [Google Scholar]
- Banks W. A.; Kastin A. J. Differential permeability of the blood–brain barrier to two pancreatic peptides: Insulin and amylin. Peptides 1998, 19, 883–889. 10.1016/S0196-9781(98)00018-7. [Abstract] [CrossRef] [Google Scholar]
- Oskarsson M. E.; Paulsson J. F.; Schultz S. W.; Ingelsson M.; Westermark P.; Westermark G. T. In vivo seeding and cross-seeding of localized amyloidosis: A molecular link between type 2 diabetes and Alzheimer disease. Am. J. Pathol. 2015, 185, 834–846. 10.1016/j.ajpath.2014.11.016. [Abstract] [CrossRef] [Google Scholar]
- Chen S.; Ferrone F. A.; Wetzel R. Huntington’s disease age-of-onset linked to polyglutamine aggregation nucleation. Proc. Natl. Acad. Sci. U. S. A. 2002, 99, 11884–11889. 10.1073/pnas.182276099. [Europe PMC free article] [Abstract] [CrossRef] [Google Scholar]
- Ferrone F. Analysis of protein aggregation kinetics. Methods Enzymol. 1999, 309, 256–274. 10.1016/S0076-6879(99)09019-9. [Abstract] [CrossRef] [Google Scholar]
- Wijesekara N.; Ahrens R.; Sabale M.; Wu L.; Ha K.; Verdile G.; Fraser P. E. Amyloid-β and islet amyloid pathologies link Alzheimer’s disease and type 2 diabetes in a transgenic model. Faseb J. 2017, 31, 5409–5418. 10.1096/fj.201700431R. [Abstract] [CrossRef] [Google Scholar]
- Ly H.; Verma N.; Sharma S.; Kotiya D.; Despa S.; Abner E. L.; Nelson P. T.; Jicha G. A.; Wilcock D. M.; Goldstein L. B.; et al. The association of circulating amylin with β-amyloid in familial Alzheimer’s disease. Alzheimer’s Dementia 2021, 7, e1213010.1002/trc2.12130. [Europe PMC free article] [Abstract] [CrossRef] [Google Scholar]
- Moreno-Gonzalez I.; Edwards Iii G.; Salvadores N.; Shahnawaz M.; Diaz-Espinoza R.; Soto C. Molecular interaction between type 2 diabetes and Alzheimer’s disease through cross-seeding of protein misfolding. Mol. Psychiatry 2017, 22, 1327–1334. 10.1038/mp.2016.230. [Europe PMC free article] [Abstract] [CrossRef] [Google Scholar]
- Andreetto E.; Yan L.-M.; Tatarek-Nossol M.; Velkova A.; Frank R.; Kapurniotu A. Identification of Hot Regions of the Aβ–IAPP Interaction Interface as High-Affinity Binding Sites in both Cross-and Self-Association. Angew. Chem., Int. Ed. 2010, 49, 3081–3085. 10.1002/anie.200904902. [Abstract] [CrossRef] [Google Scholar]
- Yan L.-M.; Velkova A.; Tatarek-Nossol M.; Andreetto E.; Kapurniotu A. IAPP Mimic Blocks Aβ Cytotoxic Self-Assembly: Cross-Suppression of Amyloid Toxicity of Aβ and IAPP Suggests a Molecular Link between Alzheimer’s Disease and Type II Diabetes. Angew. Chem., Int. Ed. 2007, 46, 1246–1252. 10.1002/anie.200604056. [Abstract] [CrossRef] [Google Scholar]
- Yan L.-M.; Velkova A.; Kapurniotu A. Molecular characterization of the hetero-assembly of β-amyloid peptide with islet amyloid polypeptide. Curr. Pharm. Des. 2014, 20, 1182–1191. 10.2174/13816128113199990064. [Abstract] [CrossRef] [Google Scholar]
- Young L. M.; Mahood R. A.; Saunders J. C.; Tu L.-H.; Raleigh D. P.; Radford S. E.; Ashcroft A. E. Insights into the consequences of co-polymerisation in the early stages of IAPP and Aβ peptide assembly from mass spectrometry. Analyst 2015, 140, 6990–6999. 10.1039/C5AN00865D. [Europe PMC free article] [Abstract] [CrossRef] [Google Scholar]
- Hu R.; Zhang M.; Chen H.; Jiang B.; Zheng J. Cross-seeding interaction between β-amyloid and human islet amyloid polypeptide. ACS Chem. Neurosci. 2015, 6, 1759–1768. 10.1021/acschemneuro.5b00192. [Abstract] [CrossRef] [Google Scholar]
- Bharadwaj P.; Solomon T.; Sahoo B. R.; Ignasiak K.; Gaskin S.; Rowles J.; Verdile G.; Howard M. J.; Bond C. S.; Ramamoorthy A.; Martins R. N.; et al. Amylin and beta amyloid proteins interact to form amorphous heterocomplexes with enhanced toxicity in neuronal cells. Sci. Rep. 2020, 10 (1), 10356.10.1038/s41598-020-66602-9. [Europe PMC free article] [Abstract] [CrossRef] [Google Scholar]
- Ge X.; Yang Y.; Sun Y.; Cao W.; Ding F. Islet amyloid polypeptide promotes amyloid-beta aggregation by binding-induced helix-unfolding of the amyloidogenic core. ACS Chem. Neurosci. 2018, 9, 967–975. 10.1021/acschemneuro.7b00396. [Europe PMC free article] [Abstract] [CrossRef] [Google Scholar]
- Qian Z.; Saikia N.; Sun Y. Oligomerization and fibrillation of amyloid peptides: Mechanism, toxicity and inhibition. Front. Mol. Biosci. 2022, 9, 1023047.10.3389/fmolb.2022.1023047. [Europe PMC free article] [Abstract] [CrossRef] [Google Scholar]
- Li X.; Lao Z.; Zou Y.; Dong X.; Li L.; Wei G. Mechanistic insights into the co-aggregation of Aβ and hIAPP: an all-atom molecular dynamic study. J. Phys. Chem. B 2021, 125, 2050.10.1021/acs.jpcb.0c11132. [Abstract] [CrossRef] [Google Scholar]
- Baram M.; Atsmon-Raz Y.; Ma B.; Nussinov R.; Miller Y. Amylin–Aβ oligomers at atomic resolution using molecular dynamics simulations: a link between Type 2 diabetes and Alzheimer’s disease. Phys. Chem. Chem. Phys. 2016, 18, 2330–2338. 10.1039/C5CP03338A. [Europe PMC free article] [Abstract] [CrossRef] [Google Scholar]
- Berhanu W. M.; Yasar F.; Hansmann U. H. In silico cross seeding of Aβ and amylin fibril-like oligomers. ACS Chem. Neurosci. 2013, 4, 1488–1500. 10.1021/cn400141x. [Europe PMC free article] [Abstract] [CrossRef] [Google Scholar]
- Zhang M.; Hu R.; Ren B.; Chen H.; Jiang B.; Ma J.; Zheng J. Molecular understanding of Aβ-hIAPP cross-seeding assemblies on lipid membranes. ACS Chem. Neurosci. 2017, 8, 524–537. 10.1021/acschemneuro.6b00247. [Abstract] [CrossRef] [Google Scholar]
- Longhena F.; Spano P.; Bellucci A. Targeting of disordered proteins by small molecules in neurodegenerative diseases. Targeting Trafficking Drug Dev. 2017, 245, 85–110. 10.1007/164_2017_60. [Abstract] [CrossRef] [Google Scholar]
- Al Adem K.; Shanti A.; Srivastava A.; Homouz D.; Thomas S. A.; Khair M.; Stefanini C.; Chan V.; Kim T.-Y.; Lee S. Linking Alzheimer’s disease and type 2 diabetes: characterization and inhibition of cytotoxic Aβ and IAPP hetero-aggregates. Front. Mol. Biosci. 2022, 9, 842582.10.3389/fmolb.2022.842582. [Europe PMC free article] [Abstract] [CrossRef] [Google Scholar]
- Seuma M.; Lehner B.; Bolognesi B. An atlas of amyloid aggregation: the impact of substitutions, insertions, deletions and truncations on amyloid beta fibril nucleation. Nat. Commun. 2022, 13, 7084.10.1038/s41467-022-34742-3. [Europe PMC free article] [Abstract] [CrossRef] [Google Scholar]
- Fernandez-Escamilla A.-M.; Rousseau F.; Schymkowitz J.; Serrano L. Prediction of sequence-dependent and mutational effects on the aggregation of peptides and proteins. Nat. Biotechnol. 2004, 22, 1302–1306. 10.1038/nbt1012. [Abstract] [CrossRef] [Google Scholar]
- Betsholtz C.; Christmansson L.; Engström U.; Rorsman F.; Svensson V.; Johnson K. H.; Westermark P. Sequence divergence in a specific region of islet amyloid polypeptide (IAPP) explains differences in islet amyloid formation between species. FEBS Lett. 1989, 251, 261–264. 10.1016/0014-5793(89)81467-X. [Abstract] [CrossRef] [Google Scholar]
- Westermark P.; Engström U.; Johnson K. H.; Westermark G. T.; Betsholtz C. Islet amyloid polypeptide: pinpointing amino acid residues linked to amyloid fibril formation. Proc. Natl. Acad. Sci. U. S. A. 1990, 87, 5036–5040. 10.1073/pnas.87.13.5036. [Europe PMC free article] [Abstract] [CrossRef] [Google Scholar]
- Ashburn T. T.; Lansbury P. T. Jr Interspecies sequence variations affect the kinetics and thermodynamics of amyloid formation: Peptide models of pancreatic amyloid. J. Am. Chem. Soc. 1993, 115, 11012–11013. 10.1021/ja00076a078. [CrossRef] [Google Scholar]
- Hess B.; Kutzner C.; Van Der Spoel D.; Lindahl E. GROMACS 4: algorithms for highly efficient, load-balanced, and scalable molecular simulation. J. Chem. Theory Comput. 2008, 4, 435–447. 10.1021/ct700301q. [Abstract] [CrossRef] [Google Scholar]
- Meng F.; Abedini A.; Plesner A.; Verchere C. B.; Raleigh D. P. The flavanol (−)-epigallocatechin 3-gallate inhibits amyloid formation by islet amyloid polypeptide, disaggregates amyloid fibrils, and protects cultured cells against IAPP-induced toxicity. Biochemistry 2010, 49, 8127–8133. 10.1021/bi100939a. [Europe PMC free article] [Abstract] [CrossRef] [Google Scholar]
- Cao P.; Raleigh D. P. Analysis of the inhibition and remodeling of islet amyloid polypeptide amyloid fibers by flavanols. Biochemistry 2012, 51, 2670–2683. 10.1021/bi2015162. [Europe PMC free article] [Abstract] [CrossRef] [Google Scholar]
- Kreutzer A. G.; Nowick J. S. Elucidating the structures of amyloid oligomers with macrocyclic β-hairpin peptides: Insights into Alzheimer’s disease and other amyloid diseases. Acc. Chem. Res. 2018, 51, 706–718. 10.1021/acs.accounts.7b00554. [Europe PMC free article] [Abstract] [CrossRef] [Google Scholar]
- Pannuzzo M.; Raudino A.; Milardi D.; La Rosa C.; Karttunen M. α-helical structures drive early stages of self-assembly of amyloidogenic amyloid polypeptide aggregate formation in membranes. Sci. Rep. 2013, 3, 2781.10.1038/srep02781. [Europe PMC free article] [Abstract] [CrossRef] [Google Scholar]
- Rawat A.; Maity B. K.; Chandra B.; Maiti S. Aggregation-induced conformation changes dictate islet amyloid polypeptide (IAPP) membrane affinity. Biochimica Et Biophysica Acta (BBA)-Biomembranes 2018, 1860, 1734–1740. 10.1016/j.bbamem.2018.03.027. [Abstract] [CrossRef] [Google Scholar]
- Qiu T.; Liu Q.; Chen Y.-X.; Zhao Y.-F.; Li Y.-M. Aβ42 and Aβ40: similarities and differences. J. Pept. Sci. 2015, 21, 522–529. 10.1002/psc.2789. [Abstract] [CrossRef] [Google Scholar]
- Vivekanandan S.; Brender J. R.; Lee S. Y.; Ramamoorthy A. A partially folded structure of amyloid-beta (1–40) in an aqueous environment. Biochem. Biophys. Res. Commun. 2011, 411, 312–316. 10.1016/j.bbrc.2011.06.133. [Europe PMC free article] [Abstract] [CrossRef] [Google Scholar]
- Nanga R. P. R.; Brender J. R.; Vivekanandan S.; Ramamoorthy A. Structure and membrane orientation of IAPP in its natively amidated form at physiological pH in a membrane environment. Biochimica Et Biophysica Acta (BBA)-Biomembranes 2011, 1808, 2337–2342. 10.1016/j.bbamem.2011.06.012. [Europe PMC free article] [Abstract] [CrossRef] [Google Scholar]
- Daura X.; Gademann K.; Jaun B.; Seebach D.; Van Gunsteren W. F.; Mark A. E. Peptide folding: when simulation meets experiment. Angew. Chem., Int. Ed. 1999, 38, 236–240. 10.1002/(SICI)1521-3773(19990115)38:1/2<236:AID-ANIE236>3.0.CO;2-M. [CrossRef] [Google Scholar]
- Huang J.; Rauscher S.; Nawrocki G.; Ran T.; Feig M.; de Groot B. L.; Grubmüller H.; MacKerell A. D. Jr CHARMM36m: an improved force field for folded and intrinsically disordered proteins. Nat. Methods 2017, 14, 71–73. 10.1038/nmeth.4067. [Europe PMC free article] [Abstract] [CrossRef] [Google Scholar]
- Jorgensen W. L.; Chandrasekhar J.; Madura J. D.; Impey R. W.; Klein M. L. Comparison of simple potential functions for simulating liquid water. J. Chem. Phys. 1983, 79, 926–935. 10.1063/1.445869. [CrossRef] [Google Scholar]
- Martínez L.; Andrade R.; Birgin E. G.; Martínez J. M. PACKMOL: A package for building initial configurations for molecular dynamics simulations. J. Comput. Chem. 2009, 30, 2157–2164. 10.1002/jcc.21224. [Abstract] [CrossRef] [Google Scholar]
- Barducci A.; Bussi G.; Parrinello M. Well-tempered metadynamics: a smoothly converging and tunable free-energy method. Phys. Rev. Lett. 2008, 100, 020603.10.1103/PhysRevLett.100.020603. [Abstract] [CrossRef] [Google Scholar]
- Darden T.; York D.; Pedersen L. Particle mesh Ewald: An N ·log(N) method for Ewald sums in large systems. J. Chem. Phys. 1993, 98, 10089.10.1063/1.464397. [CrossRef] [Google Scholar]
- Miyamoto S.; Kollman P. A. Settle: An analytical version of the SHAKE and RATTLE algorithm for rigid water models. J. Comput. Chem. 1992, 13, 952–962. 10.1002/jcc.540130805. [CrossRef] [Google Scholar]
- Hess B.; Bekker H.; Berendsen H. J.; Fraaije J. G. LINCS: A linear constraint solver for molecular simulations. J. Comput. Chem. 1997, 18, 1463–1472. 10.1002/(SICI)1096-987X(199709)18:12<1463::AID-JCC4>3.0.CO;2-H. [CrossRef] [Google Scholar]
- Bussi G.; Donadio D.; Parrinello M. Canonical sampling through velocity rescaling. J. Chem. Phys. 2007, 126, 014101.10.1063/1.2408420. [Abstract] [CrossRef] [Google Scholar]
- Parrinello M.; Rahman A. Polymorphic transitions in single crystals: A new molecular dynamics method. J. Appl. Phys. 1981, 52, 7182–7190. 10.1063/1.328693. [CrossRef] [Google Scholar]
- Tribello G. A.; Bonomi M.; Branduardi D.; Camilloni C.; Bussi G. PLUMED 2: New feathers for an old bird. Comput. Phys. Commun. 2014, 185, 604–613. 10.1016/j.cpc.2013.09.018. [CrossRef] [Google Scholar]
- Michaud-Agrawal N.; Denning E. J.; Woolf T. B.; Beckstein O. MDAnalysis: A toolkit for the analysis of molecular dynamics simulations. J. Comput. Chem. 2011, 32, 2319–2327. 10.1002/jcc.21787. [Europe PMC free article] [Abstract] [CrossRef] [Google Scholar]
- McGibbon R. T.; Beauchamp K. A.; Harrigan M. P.; Klein C.; Swails J. M.; Hernández C. X.; Schwantes C. R.; Wang L.-P.; Lane T. J.; Pande V. S. MDTraj: A modern open library for the analysis of molecular dynamics trajectories. Biophys. J. 2015, 109, 1528–1532. 10.1016/j.bpj.2015.08.015. [Europe PMC free article] [Abstract] [CrossRef] [Google Scholar]
- Hunter J. D. Matplotlib: A 2D graphics environment. Comput. Sci. Eng. 2007, 9, 90–95. 10.1109/MCSE.2007.55. [CrossRef] [Google Scholar]
- Humphrey W.; Dalke A.; Schulten K. VMD: Visual molecular dynamics. J. Mol. Graphics 1996, 14, 33–38. 10.1016/0263-7855(96)00018-5. [Abstract] [CrossRef] [Google Scholar]
- Schrödinger L.; DeLano W.. PyMOL http://www.pymol.org/pymol.
- Best R. B.; Hummer G.; Eaton W. A. Native contacts determine protein folding mechanisms in atomistic simulations. Proc. Natl. Acad. Sci. U. S. A. 2013, 110, 17874–17879. 10.1073/pnas.1311599110. [Europe PMC free article] [Abstract] [CrossRef] [Google Scholar]
Articles from ACS Omega are provided here courtesy of American Chemical Society
Citations & impact
This article has not been cited yet.
Impact metrics
Alternative metrics

Discover the attention surrounding your research
https://www.altmetric.com/details/165889128
Data
Data behind the article
This data has been text mined from the article, or deposited into data resources.
BioStudies: supplemental material and supporting data
Protein structures in PDBe (2)
-
(1 citation)
PDBe - 2LFMView structure
-
(1 citation)
PDBe - 2L86View structure
Similar Articles
To arrive at the top five similar articles we use a word-weighted algorithm to compare words from the Title and Abstract of each citation.
Linking Alzheimer's Disease and Type 2 Diabetes: Characterization and Inhibition of Cytotoxic Aβ and IAPP Hetero-Aggregates.
Front Mol Biosci, 9:842582, 17 Mar 2022
Cited by: 5 articles | PMID: 35372522 | PMCID: PMC8968156
Islet Amyloid Polypeptide Promotes Amyloid-Beta Aggregation by Binding-Induced Helix-Unfolding of the Amyloidogenic Core.
ACS Chem Neurosci, 9(5):967-975, 09 Feb 2018
Cited by: 24 articles | PMID: 29378116 | PMCID: PMC5955824
Simulations of cross-amyloid aggregation of amyloid-β and islet amyloid polypeptide fragments.
Biophys J, 121(11):2002-2013, 10 May 2022
Cited by: 4 articles | PMID: 35538665 | PMCID: PMC9247468
Causative factors for formation of toxic islet amyloid polypeptide oligomer in type 2 diabetes mellitus.
Clin Interv Aging, 10:1873-1879, 19 Nov 2015
Cited by: 14 articles | PMID: 26604727 | PMCID: PMC4655906
Review Free full text in Europe PMC