Abstract
Free full text

Comprehensive analysis of classroom microclimate in context to health-related national and international indoor air quality standards
Associated Data
Abstract
Indoor air quality (IAQ) and indoor air pollution are critical issues impacting urban environments, significantly affecting the quality of life. Nowadays, poor IAQ is linked to respiratory and cardiovascular diseases, allergic reactions, and cognitive impairments, particularly in settings like classrooms. Thus, this study investigates the impact of indoor environmental quality on student health in a university classroom over a year, using various sensors to measure 19 environmental parameters, including temperature, relative humidity, CO2, CO, volatile organic compounds (VOCs), particulate matter (PM), and other pollutants. Thus, the aim of the study is to analyze the implications of the indoor microclimate for the health of individuals working in the classroom, as well as its implications for educational outcomes. The data revealed frequent exceedances of international standards for formaldehyde (HCHO), VOC, PM2.5, NO, and NO2. HCHO and VOCs levels, often originating from building materials and classroom activities, were notably high. PM2.5 levels exceeded both annual and daily standards, while NO and NO2 levels, possibly influenced by inadequate ventilation, also surpassed recommended limits. Even though there were numerous exceedances of current international standards, the indoor microclimate quality index (IMQI) score indicated a generally good indoor environment, remaining mostly between 0 and 50 for this indicator. Additionally, analyses indicate a high probability that some indicators will exceed the current standards, and their values are expected to trend upwards in the future. The study highlighted the need for better ventilation and pollutant control in classrooms to ensure a healthy learning environment. Frequent exceedances of pollutant standards can suggest a significant impact on student health and academic performance. Thus, the present study underscored the importance of continuous monitoring and proactive measures to maintain optimal indoor air quality.
1 Introduction
Indoor Air Quality (IAQ) and Indoor Air Pollution (IAP) are fundamental interconnected problems that significantly impact the quality of life, particularly in urban environments (1). Poor IAQ is associated with various adverse health outcomes, including increased mortality and morbidity. The presence of indoor pollutants, which can originate from activities such as smoking, cooking, heating, and the use of household products, poses serious health risks. These pollutants contribute to conditions such as respiratory and cardiovascular diseases, allergic reactions, cancers, and specific syndromes like sick building syndrome and building-related illnesses (2–5).
Recent studies have highlighted the importance of effective IAQ monitoring and mitigation strategies to protect public health. Modern IAQ monitoring systems utilize wireless technologies and advanced sensors to provide real-time data on pollutant levels, allowing for timely interventions (6). Additionally, the development of novel materials for sensors and smart home technologies offers promising solutions for improving IAQ (7). For instance, research has shown that indoor pollutants like particulate matter (PM), CO, and VOC are significantly influenced by household activities and the type of fuel used for heating. These pollutants are linked to severe health issues such as chronic obstructive pulmonary disease and lung cancer, particularly in rural areas where coal burning is common, but not only (8).
Children, older adults, and those with pre-existing syndromes are especially vulnerable to the adverse effects of IAP, which can lead to respiratory problems and infections. This vulnerability is exacerbated in households with poor ventilation and high pollutant concentrations (9). Therefore, enhancing IAQ through effective monitoring and innovative mitigation strategies is crucial for reducing health risks and improving overall public health in urban environments.
Nowadays, IAQ in classrooms is a critical factor influencing the health and academic performance of students and teachers. Poor IAQ can lead to a range of adverse health effects, including respiratory and cardiovascular diseases, allergic reactions, and cognitive impairments, which can significantly impact learning outcomes and overall wellbeing (10–12). Studies have shown that classrooms often contain high levels of various air pollutants. These pollutants originate from outdoor sources, such as traffic emissions, and indoor activities, including the use of paints, markers, and cleaning products (13). Good IAQ in schools is essential to provide a safe, healthy, productive, and comfortable environment for students, teachers, and other school staff. Various air pollutants found in classrooms, sometimes even at low concentrations, can impact students' health, particularly respiratory health, classes attendance, and academic performance (14–16). For instance, higher levels of hazardous air pollutants have been associated with decreased concentration, productivity, and overall academic performance among university students (15). A study conducted in university classrooms revealed that inadequate ventilation and high levels of CO2, PM, and other pollutants significantly affect students' ability to focus and perform academic tasks (17). Other studies found that improvements in IAQ, such as through renovations, resulted in better standardized test scores and academic outcomes in a pre-university school, suggesting similar potential benefits in higher education environments (18).
The most common pollutants found in classrooms include PM, VOC, CO, and biological contaminants like mold and bacteria. Particulate matter, including PM10 and PM2.5, originates from outdoor sources such as traffic emissions and indoor sources like dust and classroom activities (19, 20). Various research has shown that high levels of PM in classrooms are associated with adverse health effects, including respiratory symptoms, and reduced cognitive performance (14, 21). VOCs are emitted from sources like paints, cleaning supplies, and building materials, causing health issues such as irritation, headaches, and chronic effects like liver and kidney damage. Poor ventilation exacerbates VOC accumulation, increasing the risk for students and staff (22, 23). CO2 levels, due to inadequate ventilation and high occupancy, indicate poor air quality, leading to symptoms like headaches, dizziness, and reduced cognitive function (24, 25). Biological contaminants like mold, bacteria, and viruses thrive in classrooms with poor humidity control and inadequate cleaning, leading to allergies, respiratory issues, and infections. Low concentrations of H2S cause eye irritation and nausea, and high concentrations can induce fainting and death (26). SO2 is associated with increased respiratory symptoms and premature death (27, 28), at the same time O3, NO, and NO2 irritate the airways and can cause severe cardiopulmonary problems and death (29, 30). Molds can grow on damp surfaces, releasing spores that exacerbate asthma and other respiratory conditions (31).
Based on those mentioned above, the present study aims to monitor and evaluate the internal microclimate of a classroom within the Faculty of Geography, Tourism, and Sport (FGTS) at the University of Oradea, Romania, with the objective of determining the influence of specific microclimate parameters such as temperature (T), relative humidity (RH), CO, and other pollutants on human health, wellbeing, concentration levels, and the academic performance of students. Using various environmental indicators, alongside advanced statistical techniques, this research offers a thorough understanding of the impact of these variables on human health and wellbeing. The outcomes will enrich the discourse on IAQ within the classroom, thereby harmonizing our methodologies with international standards to achieve a balanced approach between the preservation of human health and the need for a clean environment conducive to scientific endeavors.
2 Materials and methods
The monitored classroom is an IT-dedicated room, containing 16 computers and having a volume of 441 m3. The room can simultaneously accommodate 20 students and one teacher, and it is used intermittently during the academic year from Monday to Friday between 8 am and 8 pm. Approximately 302 FGTS students used the classroom over the monitoring period, with an average activity period for a student being between 2 and 4 h per week, and for a teacher between 2 and 10 h.
The selection of this specific classroom was based on its representativeness of modern educational environments, including computers, various usage patterns, and the need to monitor a comprehensive set of environmental parameters over a full academic year. At the same time, IT classrooms are equipped with multiple computers and other electronic equipment that can emit volatile chemicals and thermal radiation, which can influence IAQ, and the classes taught here require a high degree of concentration and cognitive performance, making the learning environment particularly important.
The internal microclimate is controlled by HVAC systems throughout the year, but these may be insufficient or non-compliant when many students are present simultaneously in the space. Oradea, the municipality where the University of Oradea is located, experiences a temperate-continental climate, characterized by hot summers and cold winters. This climatic context can influence indoor air quality, as HVAC systems need to adjust to significant temperature variations and maintain consistent indoor conditions despite external weather fluctuations.
2.1 Analysis of pollutants variations inside the classroom
Pollution monitoring was carried out continuously during 1 calendar year, between September 1, 2022, and August 31, 2023. During this period, a number of 19 indicators were monitored, as follows: T, RH, pressure (P), CO2, CO, HCHO, VOC, H2S, SO2, O2, O3, NO, NO2, CH4, PM2.5, PM5, PM10, the concentration of positive ions (I+), and negative ions (I-). For most of the parameters, the use of datalogger sensors programmed to record and store the data every minute was considered, in order to then realize hourly averages for the targeted indicators. They were distributed throughout the room to cover it as well as possible, determining the values of the analyzed indicators with high accuracy (Figure 1A). For other indicators (I+, I-, and PM in particular), manual measurements were made before classes, during and after them, and for other indicators, additional manual measurements were made (if necessary) during the classes, such as VOC and HCHO. All the sensors used were chosen in such a way as to determine the indicators with the highest possible accuracy (Table 1). The results obtained were reported to the international standards in force for the targeted parameters concerning their influence on human health and wellbeing.
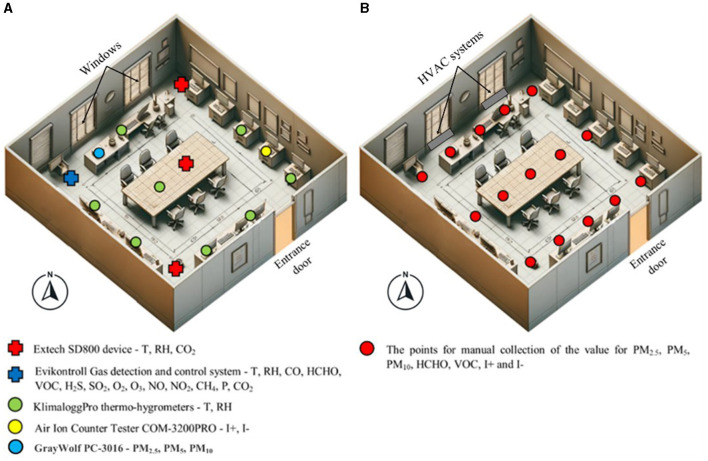
The distribution at the level of the classroom of the sensors for monitoring the indoor microclimate (A) and the manual data collection points (B).
Table 1
Technical information about the sensors used to monitor the indicators inside the classroom.
Sensor | Producer | Pollutants measured | Accuracy | No. of sensors used | Datalogger |
---|---|---|---|---|---|
Extech SD800 devices | Extech Instruments, Nashua, NH, USA | T, RH, CO2 | ±0.8°C (T), ±4% (RH), ±40 ppm (CO2) | 3 | ![]() |
Evikontroll Gas detection and control system devices | Evikontroll Gas, Tartu, Estonia | T, RH, CO2, CO, HCHO, VOC, H2S, SO2, O2, O3, NO, NO2, CH4, P | ±0.5°C (T), ±5% (RH), ±50 ppm (CO2), ±1 ppm (CO), ±0.01 ppm (HCHO, VOC), ±0.1 ppm (H2S, SO2, O3, NO, NO2), ±0.01% (O2, CH4), ±1 hPa (P) | 2 | ![]() |
KlimaloggPro thermo-hygrometers | TFA, Ottersberg, Germany | T, RH | ±1°C (T), ±3% (RH) | 8 | ![]() |
BLATN BR-smart-123s device | BLATN Science and Technology, Beijing, China | HCHO, VOC | ±5% (HCHO, VOC) | 1 | × |
Air Ion Counter Tester COM-3200PRO II | Universal Plan Co., Tokyo, Japan | I+, I- | ±10 ions/cm3 (I+, I-) | 1 | ![]() |
PCE-PCO 2 | PCE Instruments UK, Southampton, UK | PM2.5, PM5, PM10 | up to ±5% (PM2.5, PM5, PM10) | 1 | × |
GrayWolf PC-3016 | GrayWolf Sensing Solutions, Shelton, Connecticut, USA | PM2.5, PM5, PM10 | up to ±5% (PM2.5, PM5, PM10) | 1 | ![]() |
The manually determined parameters (PM, I+, and I-) were measured at 15 points and uniformly distributed in the classroom in order to have the best possible distribution of pollutants at the room level (Figure 1B). The location of the collection points allows spatial analysis by means of cartograms that illustrate the distribution and evolution of these indicators in the analyzed room. The measurements were made at a height of 1.2–1.3 m, equivalent to the height of a sitting person of average height, to allow the analysis of the possible negative impact of these indicators on the health and wellbeing of the students. Regarding the manual PM determinations, the sampling times were 30 s for each collection point, considering the highest value measured for the sampling time.
Sensor calibration was implemented only for certain sensors, as others come pre-calibrated from the factory and do not require further user intervention. For those requiring calibration, it was conducted in controlled environments to minimize external influences, where the exact values of the respective indicators were known. Sensors were validated through cross-referencing and comparison with manual measurements. Additionally, continuous monitoring using dataloggers helped identify anomalies and validate data. Regular checks ensured that real-time data were accurate.
The collected data were analyzed both with reference to individual, hourly, daily, weekly, monthly, and annual values, depending on the need. R version 4.3.2, ArcMap 10.8.2, and MATLAB 9.7 software were used in the data analysis and interpretation process. At the same time, the recorded data were the basis for the realization of risk indices on human health and analyses regarding the probability that the recorded values will be exceeded in the future or not. All the indexes created were based on the thresholds in force accepted by the international bodies regarding the internal microclimate indicators considered.
2.2 Assessing the impact of the internal microclimate on human health
In order to assess the impact of indoor microclimate on human health, a simplified index designed to reflect IAQ was developed. This index aims to quantify internal environmental effects by comparing the average values of various selected indicators with existing international standards. The aim is to provide a clear understanding of internal environmental conditions and to facilitate the assessment of potential health risks. In the specialized literature, there are numerous studies that consider the creation of similar indices for the evaluation of the IAQ and its impact on human health, among which Saad et al. (32), Wagdi et al. (33), or Dionova et al. (34) are worth mentioning. However, in the case of the present study, an adaptation of it was chosen to reflect the needs of the study.
The following section details the mathematical formula of the indoor microclimate quality index (IMQI) (Equation 1), highlighting how these values are integrated and related to international air quality standards:
where n is the number of parameters considered, Scorei is the score for each parameter i, calculated according to the threshold or ideal interval, zi is the weight assigned to each parameter.
The score component represents a parameter that considers the calculation of the ratio between the measured value of an indicator and its international standard in force. If the value is equal to or lower than the standard, the score is 0, indicating an ideal situation and a healthy internal microclimate. If the value exceeds the standard, the score increases proportionally to the exceedance and indicates a low quality of the internal microclimate. The score component (Si) is calculated based on the Equation 2:
where MV represents the measured value of indicator i, and SV represents the standard value of indicator i.
If an indicator does not have a fixed threshold, but falls within a well-established range, as is the case of O2, which according to international standards (35), must fall between a concentration of 19.5 and 23.5%, SO2 (score for the indicator O2) will be assigned as follows (Equations 3–5):
where MVO2 is the measured value for the O2 indicator. If the O2 level is between 19.5 and 23.5%, the score is perfect (0), if the O2 level is below 19.5%, the score increases proportionally with the exceeding of the lower limit, and if the O2 level is above 23.5%, the score increases proportionally with the exceeding of the upper limit.
The zi component represents a significant weight assigned to each indicator. Thus, if a certain pollutant has a greater effect on people's health or comfort, it will be assigned a higher weighting factor in the model. By adjusting these factors, the model can balance the contributions of different variables to reflect their relative risk or importance more accurately to air quality. The specific interval for the zi weight was established to be between 0 and 1 (on four levels), where 0 means no influence, and 1 means maximum influence. The weight was assigned in such a way to reflect the comparative importance of each pollutant in affecting IAQ and human health, considering the importance in the indoor environment, the impact on health, international standards and recommendations, scientific research in the field, the consensus of experts, etc.
A low IMQI score indicates good IAQ, which means that pollutant concentrations are below the thresholds set by international standards, and the indoor environment is considered healthy and safe for occupants. At the same time, a high score indicates a low quality of the indoor microclimate, indicating that one or more pollutants exceed the limits of international standards. Considering that there is no internationally accepted scale to classify IAQ using IMQI, in this model, we have chosen to report the results using the scale proposed by EPA (36) for air evaluation. Within this system of categorizing the degree of risk to human health of the outdoor air, the EPA considers its categorization on six different levels, from a good indoor microclimate quality (0–50) to a hazardous indoor environment for human health (over 301; Figure 2).
The use of the proposed US EPA model for outdoor conditions under indoor environmental conditions may be limited due to significant differences in pollution sources, dispersion patterns, and indoor environmental conditions, as well as limited data collection and confounding factors specific to the indoor environment, such as building materials, furniture, and human activities. However, the application of this model is justified as it provides a standardized and rigorous framework for air quality assessment and the establishment of benchmarks for the protection of human health in indoor environments in the absence of dedicated standards.
2.3 Statistical analysis of probabilities
To identify microclimatic patterns and fluctuations and to obtain a comprehensive understanding of thermal comfort conditions and air quality in the classroom, the determination of the empirical distribution function (EDF) was also considered to calculate the probability that a random value is more or less than or equal to a specific value. This function was calculated for each microclimate variable that exceeded the international standards in force (the standard being regarded as the threshold that will or will not be exceeded in the future). The EDF is used to evaluate the statistical behavior of the internal microclimate, including the determination of the median, percentiles, and variability (37, 38).
The specific formula for calculating the EDF is:
where F(k) is the probability of observing a value ≤ k, a is a constant that adjusts the formula, which must be between 0 and 0.5 and n is the sample size or the number of observations. The formula essentially normalizes the position of the value k within the observed data range, adjusted by a. The choice of a affects the weighting of the tails of the distribution (Equation 6).
The ARIMA model was also applied to analyze and predict the evolution of pollutant levels in the classroom. This was done to determine to what extent the pollution that exceeded the limits imposed by the international standards in force follows a certain trend or not, having the potential to increase in terms of concentrations in the future. The ARIMA model combines three main components: the autoregressive, integration, and moving average processes, each of which has an essential role in modeling and understanding the time series dynamics of pollutant concentrations.
Autoregressive processes of p order are described by the formula:
where Xt is the value of the time series at time t, c is the constant, Ø1,...,Øp are the coefficients of the autoregressive process, and εt represents the error term (39) (Equation 7).
The integration component of d order involves differentiating the time series to ensure its stationarity, being expressed by:
where d denotes the differentiation operator of d order, and L is the delay operator (Equation 8).
The moving average processes of q order are defined as:
where μ is the mean of the series, and θ1,…,θp are the coefficients that measure the influence of previous errors on the current value (40) (Equation 9).
Combining these components, the ARIMA model is expressed by the Equation 10:
In order to ensure the quality of the obtained results, taking into account the fact that for the ARIMA model the data series must be stationary, the null hypothesis of non-stationarity was tested for each data series (where a p-value < 0.05 indicated stationarity). For non-stationary series, differentiation was applied. The order of differentiation (d) was determined by the number of times differentiation to achieve stationarity. The autoregressive order function (p) was determined using the partial autocorrelation function, while the moving average order (q) was determined using the plot autocorrelation function. After model fitting, residuals were analyzed to ensure they resembled white noise. The absence of significant autocorrelations in the plots indicates that the residuals are white noise, suggesting a good model fit.
This approach allows us to analyze how past time series values and recorded errors affect the current value and make predictions about the future evolution of pollutants in the classroom, determining whether they will increase, decrease, or remain constant in the near future. This can be implemented even if the indoor microclimate in the classroom is mostly controlled with HVAC systems, because ARIMA can identify patterns and fluctuations that can be influenced by different factors, including the operation of this systems (41, 42).
3 Results
3.1 Analysis of pollution variations inside the classroom
The values obtained for the analyzed indicators were reported to multi-annual average standards, daily average standards and respectively to short-term exposure limit that can represent an immediate threat to life or health. The results indicate that only five pollutants (HCHO, VOC, NO, NO2, and PM2.5) exceeded the multi-year average values allowed by the international standards in force. At the same time, some exceedances of the daily values were also recorded for other indicators (T, RH, and O3), but these are small and only sporadic. Regarding short-term and very short-term exceeding of the limits, they were outlined only in the case of two indicators (HCHO and PM2.5), the values being still quite small (Table 2).
Table 2
The values obtained for the analyzed indicators, being reported to the international standards in force.
Pollutant | MU | Max | Min | Avg | Std. dev. | MAA | PE—MAA | DA | PE—DA | STEL/IDLH | PE—STEL/IDLH |
---|---|---|---|---|---|---|---|---|---|---|---|
T | °C | 27.1 | 18.1 | 22.9 | 2.1 | 20–24 (43) | 14 | 18–26 (44, 45) | 0.2 | × | × |
RH | % | 60.6 | 17.6 | 38.5 | 10.2 | 30–50 (46) | 28.5 | 30–70 (47) | 22.9 | × | × |
CO2 | Ppm | 2,744 | 450 | 633.8 | 176.4 | 1,000 (48) | 4.1 | 5,000 (49) | 0 | 10.000–50.000 (50) | 0 |
HCHO | mg/m3 | 0.082 | 0 | 0.057 | 0.063 | 0.04 (51) | 58.5 | 0.05 (47) | 49.4 | 0.100 (52) | 5.8 |
VOC | mg/m3 | 4.8 | 0 | 1.4 | 0.65 | 1 (53) | 69.2 | 3 (54) | 0.4 | 590 (54) | 0 |
I+ | no/cm3 | 2,200 | 200 | 799.5 | 358.5 | <1,000 (55, 56) | 25 | × | × | × | × |
I- | no/cm3 | 2,650 | 300 | 1,168.4 | 464.2 | >1,000 (55, 56) | 38.7 | × | × | × | × |
CO | mg/m3 | 5.60 | 0 | 1.79 | 0.74 | × | × | 10 (53) | 0 | 30–40a | 0 |
H2S | mg/m3 | 0.174 | 0.012 | 0.080 | 0.026 | 0.15 (53) | 1 | 1.5 (53) | 0 | 14b | 0 |
SO2 | μg/m3 | 106.7 | 7.9 | 46.2 | 18.6 | 50 (53) | 42.5 | 125 (53) | 0 | 13,000a | 0 |
O2 | % | 22.74 | 20.39 | 21.67 | 0.5 | × | × | 19.5–23.5 (35) | 0 | 10 (55) | 0 |
O3 | μg/m3 | 137.2 | 37.3 | 69 | 18.1 | × | × | 120 | 0.8 | 196a | 0 |
NO | μg/m3 | 172.4 | 10.4 | 84.3 | 39.9 | 40 (53) | 84.7 | 75 (53) | 56.3 | 1,880a | 0 |
NO2 | μg/m3 | 260.9 | 36.9 | 107.4 | 41.2 | 40 (53) | 99.5 | 75 (53) | 78.8 | 1,880a | 0 |
CH4 | % | 0.8 | 0.1 | 0.42 | 0.19 | × | × | 5 (57, 58) | 0 | 15 (57, 58) | 0 |
PM2.5 | μg/m3 | 131.2 | 29.5 | 65.5 | 13.5 | 12 (59) | 100 | 35 (59) | 99.5 | 100 (60) | 0.7 |
PM5 | μg/m3 | 24.4 | 1.1 | 9.6 | 4 | 12 (59) | 27 | 35 (59) | 0 | 100 (60) | 0 |
PM10 | μg/m3 | 18.4 | 0.2 | 5.6 | 2.6 | 12 (59) | 1 | 35 (59) | 0 | 100 (60) | 0 |
P | hPa | 1,011.7 | 992.5 | 1,001.6 | 2.1 | 980–1,050 (61) | 0 | × | × | × | × |
MAA, Multi-annual average standard values; PE—MAA, The percentage of exceeding the MAA; DA, Daily average standard values; PE—DA, The percentage of exceeding the DA; STEL/IDLH, Short-term exposure limit/Immediate threat to life or health standards; PE—STEL/IDLH, The percentage of exceeding the STEL/IDLH; Max, Maximum value during the entire monitoring period; Min, Minimum value during the entire monitoring period; Avg, Average value of the monitorings; Std. dev., Standard deviation; MU, Measure unit.
Does not exceed MMA/Exceed MMA.
ahttps://www.cdc.gov/niosh/index.htm (accessed January 8, 2024).
bhttps://eur-lex.europa.eu/LexUriServ/LexUriServ.do?uri=OJ:L:2009:338:0087:0089:EN:PDF (accessed May 28, 2024).
The average T recorded was 22.9°C, the maximum value being 27.1°C, and the minimum 18.1°C. These values indicate an average located in the range recommended by ASHRAE (20–24°C) for thermal comfort, but with significant deviations, suggesting that in some periods the conditions may be less comfortable (Figure 3). The RH average was 38.5% and varied between 17.60 and 60.60%. The average and most RH values are consistent within the EPA recommended range (30–50%) for minimizing risks related to mold growth and respiratory problems, although there are records exceeding the upper limit (Figure 3). According to the analysis performed on the data set, the average CO2 concentration was about 633.8 ppm. The maximum value recorded was 2,744.38 ppm, significantly above the threshold of 1,000 ppm recommended by the ANSI/ASHRAE standard for maintaining human health. However, ~95.9% of the CO2 records remained below the 1,000 ppm threshold, suggesting that air quality was adequate most of the time (Figure 3).
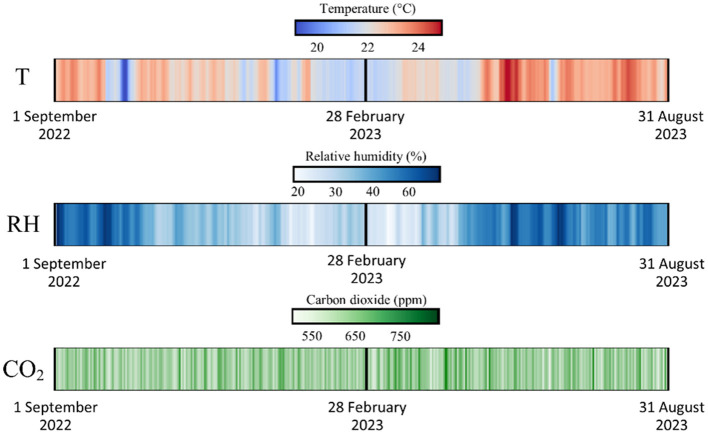
Variations of daily averages of T, RH, and CO2 inside the classroom analyzed during the period 01.09.2022–31.08.2023.
The spatial distribution of T at the classroom level indicates a higher value in the immediate vicinity of the windows (24.09°C) and decreases with the distance from them and the proximity to the opposite wall (21.51°C). Regarding RH, its values are inversely proportional to those of T so that in the immediate vicinity of the windows, the lowest values are recorded (33.3%), while in the opposite area of the room, the values reach over 41% in the wettest points (Figure 4). This is also determined by the HVAC systems being located near the windows.
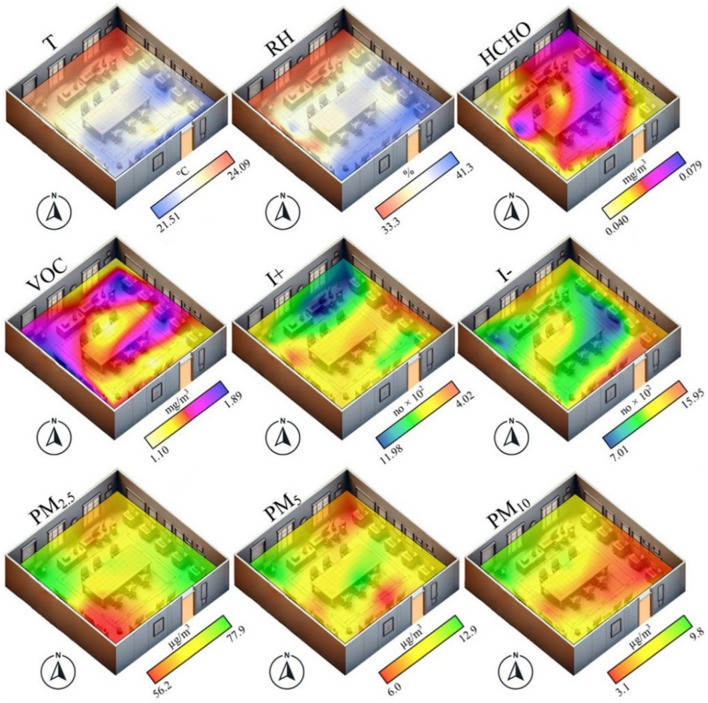
The spatial distribution at the classroom level of the average values of T, RH, HCHO, VOC, I+, I-, PM2.5, PM5, and PM10 (the values represent the multi-year average for each collection point).
The values obtained indicate an average VOC concentration of 1.43 mg/m3, exceeding the annual threshold of 1 mg/m3 (53), suggesting a possible influence on the health of the occupants. The variability of VOC indicates a maximum value of 4.8 mg/m3 and a minimum of 0.0 mg/m3, resulting in the daily limit of 3 mg/m3 being exceeded in only 0.4% of the measurements. In the northern area of the room, the VOC concentrations reached a maximum of 1.89 mg/m3, reflecting the uneven distribution inside the room (Figures 4, ,5).5). The average concentration of HCHO was 0.057 mg/m3, a value above the daily threshold of 0.05 mg/m3, indicating a constant and potentially harmful exposure. Approximately 49.4% of the measurements exceeded the established daily limit, with a maximum value of 0.82 mg/m3. Regarding the spatial variation within the classroom, the high values (up to 0.079 mg/m3) are concentrated in the middle of the classroom, while on the sides (the exception being the window area), the values decrease to a minimum of 0.040 mg/m3 (Figure 4).
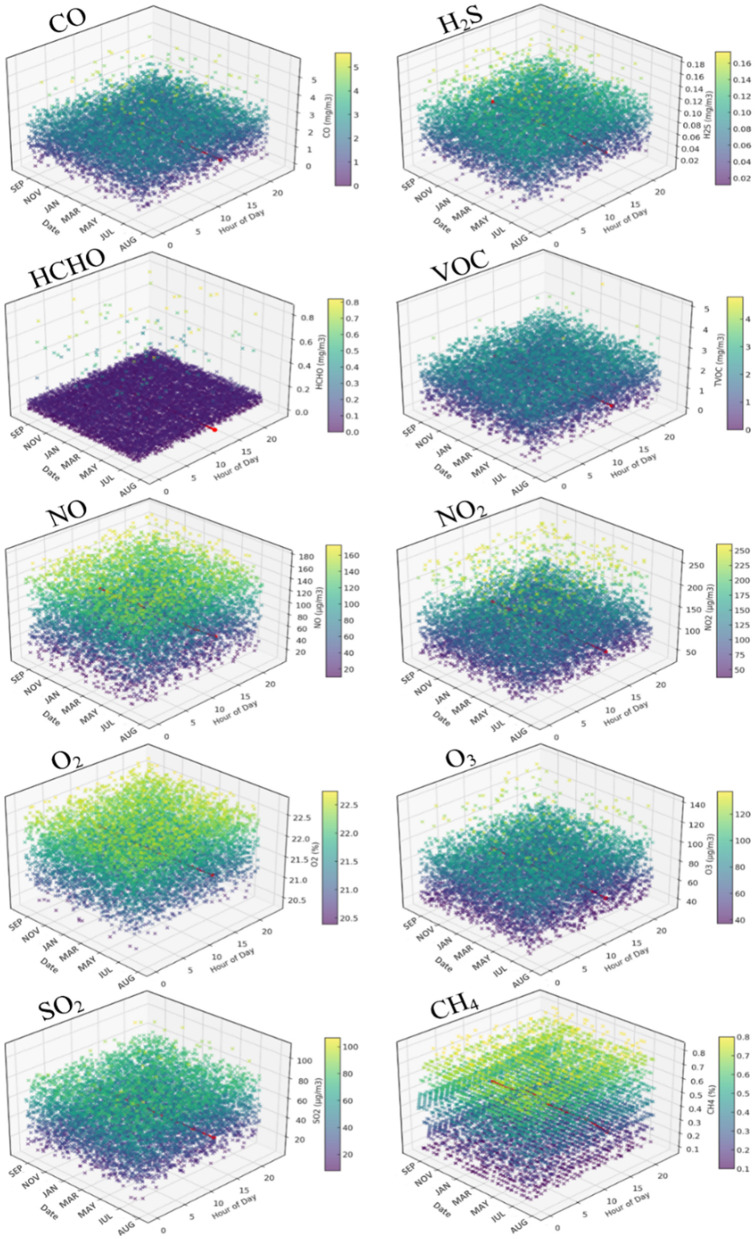
The values obtained by hours and days for the CO, H2S, HCHO, VOC, NO, NO2, O2, O3, SO2, and CH4 indicators in the case of the analyzed classroom.
Average daily CO values were constant, remaining below the safety limit of 10 mg/m3, with a mean of 1.79 mg/m3 and variations between 0.0 and 5.6 mg/m3, indicating safe short- and long-term exposure. H2S concentrations varied daily with an average of 0.075 mg/m3 and daily maximum values of up to 0.174 mg/m3. Although 7% of days exceeded the daily threshold of 0.15 mg/m3, the overall values remained below the prolonged exposure threshold of 1.5 mg/m3. For SO2, average daily concentrations ranged between 12.29 and 103.03 μg/m3, with an annual average of 46.19 μg/m3, below the threshold of 50 μg/m3. Maximum daily values were below the 125 μg/m3 limit, indicating general compliance with international standards. O2 concentrations were maintained between 20.39 and 22.74%, with an average of 21.67%, according to international standards for IAQ. The average value of O3 was 68.98 μg/m3, below the threshold recommended by the World Health Organization (53). The values varied between 37.3 and 137.2 μg/m3, with the threshold of 120 μg/m3 being exceeded in 0.84% of the measurements. The average concentration of NO was 84.27 μg/m3, and that of NO2 was 107.40 μg/m3, with significant variations around the average and high frequencies of exceeding the international limits indicating the value of 75 μg/m3 as the daily average allowed. The average CH4 concentration was 0.57%, below the critical threshold, with values varying between 0.30 and 0.70%, demonstrating continuous compliance with environmental protection regulations (Table 2 and Figure 5).
The average concentration of I+ was 799.5/cm3, with a maximum value of 2,200/cm3 and a minimum value of 200/cm3. Of the total recordings, ~25.4% exceeded the established threshold of 1,000/cm3, indicating a frequently high level of measurements exceeding the recommended threshold. On the other hand, the average concentration of I- was 1,168.4/cm3, with a maximum value of 2,650/cm3 and a minimum of 300/cm3. Of the total measurements, 61.3% exceeded the value of 1,000/cm3, considered to be conducive to the development of human activity, and 5.2% of the time, the values even exceeded 2,000/cm3 (Figure 6). Regarding the analysis of the spatial distribution of the two indicators at the level of the analyzed classroom, two different situations are individualized. In the case of I+, the area in the immediate vicinity of the windows obtained values higher than 1,000/cm3, while in the rest of the rooms the values do not exceed 800/cm3. I- is individualized by low values, up to 701/cm3, in the middle of the room, while the majority neighboring areas obtained values of over 1,000/cm3 (Figure 4). These results highlight, for the most part, a predominance of positive ions above the optimal level and an insufficiency of negative ions in relation to the values considered ideal for a healthy environment.
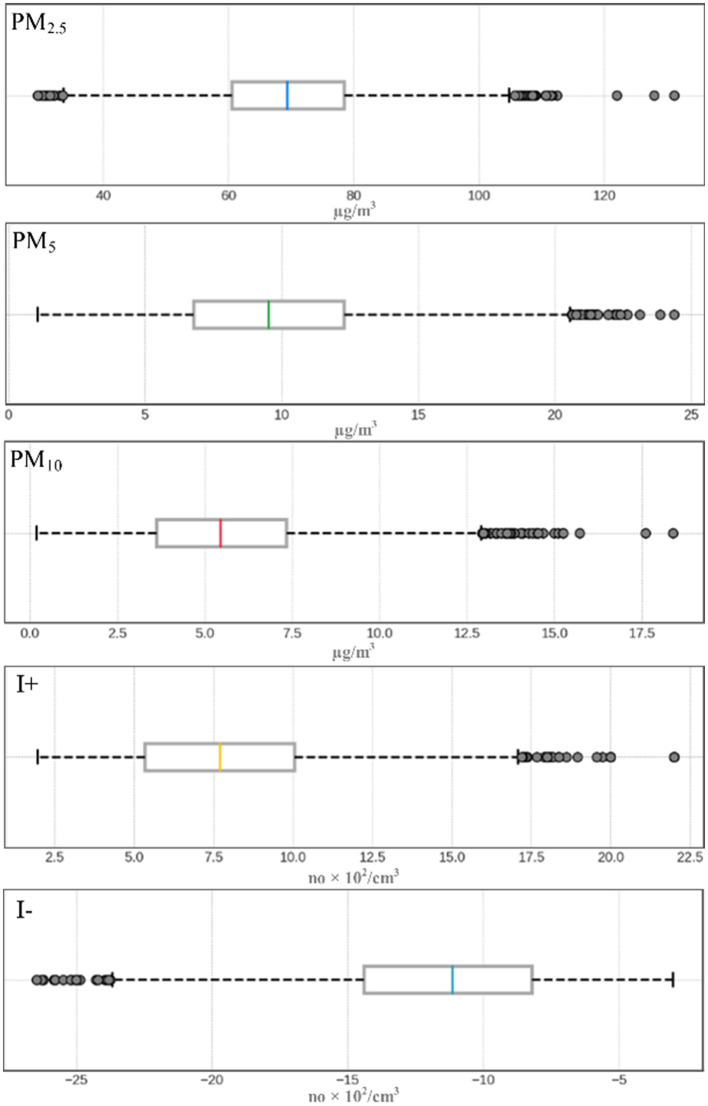
The concentration of PM2.5, PM5, PM10, I+, and I- in the classroom, during the period 01.09.2022–31.08.2023.
Regarding PM, the average concentration of PM2.5 was 69.51 μg/m3, with absolute values between 29.53 and 131.20 μg/m3. PM5 averaged 9.61 μg/m3, ranging between 1.06 and 24.37 μg/m3. PM10 recorded an average of 5.60 μg/m3, with absolute values between 0.18 and 18.40 μg/m3 (Figure 6). Thus, PM2.5 frequently exceeded the EPA annual standard (57) of 12 μg/m3, and 99.4% of the measurements exceeded the daily limit of 35 μg/m3, indicating persistent pollution. PM5 exceeded the annual threshold of 12 μg/m3 in 27.4% of cases without daily exceedances; however, PM10 exceeded the same threshold in only 1.1% of cases without exceeding the daily limit. These results suggest significant PM2.5 pollution, while PM5 and PM10 had rare exceedances of accepted standards.
Significant differences are observed when analyzing the spatial distribution of PM2.5, PM5, and PM10 in the classroom. PM2.5 shows lower values in the southern part (maximum of 56.2 μg/m3), while the rest of the hall registers values above 60 μg/m3, with maxima up to 77.9 μg/m3 in opposite corners of the room. The PM5 indicator has a variable distribution, with higher concentrations in the center and northwest of the hall and values below 8 μg/m3 in the surrounding areas. PM10 shows low values in the eastern and central part (minimum of 3.1 μg/m3), with higher concentrations near the windows (maximum of 9.8 μg/m3; Figure 4). High PM values in the vicinity of windows may be due to their opening for ventilation, allowing PM from outside to enter and increase indoor concentrations.
The results indicate significant PM2.5 pollution and frequent exceedances of acceptable concentrations for VOC, NO, NO2, and HCHO, suggesting a persistent negative influence on occupant health. Also, the spatial distribution of these pollutants reveals higher concentrations in certain classroom areas, especially near windows, highlighting the need to implement strict ventilation and pollution control measures to ensure a safe and healthy learning environment. The results are suggestive even if a US EPA standard designed for the outdoor environment was used to compare the results obtained. This is because there are no universally accepted standards for indoor PM2.5 and PM10 levels. The EPA standards are a benchmark because they are well-established and widely recognized for their health-based limits. The EPA's outdoor standards are based on extensive health research designed to protect public health, including sensitive populations. Using these standards as a reference for indoor air quality helps ensure that indoor environments are safe and healthy. Using the same standards for outdoor and indoor environments allows for easier comparison and understanding of air quality data. It provides a consistent framework for evaluating and managing air quality across different settings.
3.2 Assessing the impact of the internal microclimate on human health (IMQI)
To determine the IMQI, 18 indicators were used, considered to significantly impact human health if they are not mentioned in the accepted standards. Within this, it was considered to give a weight for each pollutant, depending on the impact that the pollutant has on health. Because there is no clear methodology for assigning weights and considering the fact that the WHO does not specify how they assign weights to pollutants, the indicator was assigned based on specialized literature (Table 3). At the same time, because people spend a limited amount of time inside the classroom, the standards to which the pollutant values were reported are those that aim at the daily variations allowed or the maximum values accepted for spending a limited amount of time in a certain indoor microclimate.
Table 3
The average obtained for each pollutant in the analyzed period, the value of the accepted international threshold at which it was reported, the unit of measure, the weight given to each indicator within the IMQI equation, and the scores obtained by the analyzed indicators.
Pollutant | Pollutant average | International standards thresholds | Measure unit | Indicator weight | SLA | Score |
---|---|---|---|---|---|---|
PM2.5 | 69.5 | 35 | μg/m3 | 1 | (62, 63) | 98.6 |
PM5 | 9.6 | 35 | μg/m3 | 1 | (62, 63) | 0 |
PM10 | 5.6 | 35 | μg/m3 | 1 | (62, 63) | 0 |
O3 | 68.9 | 120 | μg/m3 | 1 | (64) | 0 |
NO2 | 107.4 | 75 | μg/m3 | 0.75 | (65–67) | 43.2 |
NO | 84.3 | 75 | μg/m3 | 0.75 | (65–67) | 12.4 |
SO2 | 46.2 | 125 | μg/m3 | 0.75 | (65) | 0 |
CO | 1.8 | 10 | mg/m3 | 0.75 | (62, 68) | 0 |
VOC | 1.4 | 3 | mg/m3 | 0.75 | (69) | 0 |
HCHO | 0.057 | 0.05 | mg/m3 | 0.75 | (69) | 14 |
H2S | 0.075 | 1.5 | mg/m3 | 0.5 | (70) | 0 |
CO2 | 633.8 | 5,000 | ppm | 0.5 | (70, 71) | 0 |
CH4 | 0.42 | 5 | % | 0.25 | (72) | 0 |
O2 | 21.67 | 19.5–23.5 | % | 0.5 | (70, 73) | 0 |
T | 22.88 | 20–24 | °C | 0.25 | (74) | 0 |
RH | 38.5 | 30–50 | % | 0.25 | (74) | 0 |
I+ | 8 × 102 | <10 × 102 | No. | 0.25 | (75, 76) | 0 |
I- | 11.7 × 102 | >10 × 102 | No. | 0.25 | (75, 76) | 0 |
*SLA—the specialized literature analyzed for the conferring of the indicator weights.
IMQI scores exceeding the ideal value (0).
Out of the 18 analyzed indicators, 14 obtained scores of 0, which indicates that the average values obtained for the entire monitoring period are lower than or equal to the international standards to which they were reported. The other four indicators recorded exceedances in a different quantity of the allowed thresholds. Thus, the smallest exceedances were consistent with NO (score 12.4), followed by HCHO (score 14) and NO2, with a score of 43.2. The most significant score was recorded in the case of the PM2.5 indicator, which was individualized by a coefficient of 98.6. Although most of the indicators comply with international standards, which indicates a generally satisfactory level of IMQ, high values in the case of some pollutants (especially PM2.5 and NO2) have the potential to represent a danger to human health, even acting in an individual way.
The final IMQI results considering the pollutant average was 13.4. Such a score indicates that the internal microclimate in the classroom is of good quality, presenting no danger to human health. Analyzing the IMQI index for each hour of the data series, it appears that the scores vary between a minimum of 0.3 and a maximum of 121.4. Thus, these values fall into three different categories in terms of the influence they can have on human health. The best represented score range is between 0 and 50, which is individualized by a weight of 98.8%, followed by the category located between 50.1 and 100 which has 0.8% and the category 100.1–150, with only 0.4% (Figure 7B).
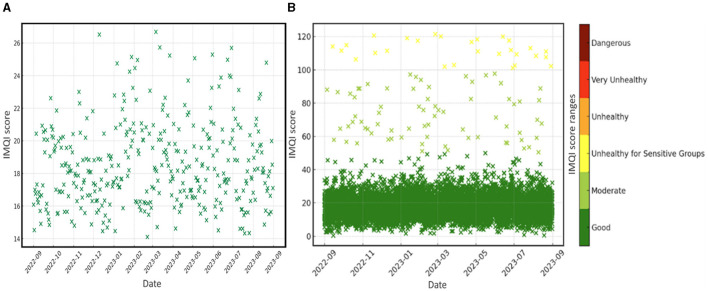
IMQI scores were obtained based on daily (A) and hourly (B) values of pollutants determined in the classroom.
Although some hourly values are above the threshold that defines the ideal, the daily IMQI values are much better. Thus, the results demonstrated that all daily IMQI scores fall within the range of 0–50, with an average of 18.5, a minimum of 14.1, and a maximum of 26.7 (Figure 7A). This consistent categorization indicates that the air quality throughout the period is of a high standard, posing minimal health risks to human health. Thus, staff and students experience minimal exposure to air pollutants, reducing the risk of pollution-related health issues.
3.3 Statistical analysis of probabilities
According to the ARIMA model applied to analyze and predict the evolution of pollutant levels in the classroom, different trends of evolution for pollutants until January 2025 (for the next year) were identified. The model revealed that the levels of CO2, CO, HCHO, TVOC, and O2 will show a stabilization, without significant variations in the near future. These results suggest that, in the absence of major changes in environmental conditions or classroom use, the concentrations of these pollutants will remain constant. On the other hand, the model showed that H2S and NO2 levels tend to decrease slightly. The reduction in the levels of these pollutants can be attributed to an improvement in ventilation and pollution source control measures toward the end of the monitoring period. In contrast, SO2 and NO concentrations tend to increase (Figure 8). The increase in SO2 and NO2 levels can be related to the use of certain materials or equipment that emit these pollutants, suggesting the need for interventions to reduce emissions. It is essential to understand the causes of these trends and take appropriate measures to ensure a healthy classroom environment. Continuous monitoring and adjustment of ventilation and pollution control strategies are vital to maintaining air quality within optimal parameters. These predictions emphasize the importance of continuous monitoring and proactive intervention to control and reduce air pollution in classrooms, thereby ensuring an environment conducive to the teaching-learning process and the health of all occupants.
In addition to creating models for predicting the evolution of indoor pollutant concentrations, it is crucial to determine the probability that these concentrations will exceed current international standards, thus indicating a potentially harmful environment for the health of staff and students. Thus, the EDF analysis indicates that HCHO is a pollutant that registers large and frequent exceedances of the international standards in force. The maximum values per day recorded exceeded 0.070 mg/m3 in all cases, the probability being very high that they will exceed the value of 0.1 mg/m3 (~76%-−0.237 EDF index). The probability that HCHO values exceed 0.5 mg/m3 is ~15% (0.848 EDF index), and that it exceeds 0.8 mg/m3 is 2% (0.979 EDF index). Regarding I+, the international standards in force, which show the maximum acceptability threshold as 1,000, were exceeded daily at least once. The EDF values for I+ indicate that a break above 1,400 is assigned an index of 0.23, while a value of 2,000 is assigned an EDF index of 0.809 and a high of 2,200 is assigned an EDF index of 0.998 (Figure 9).
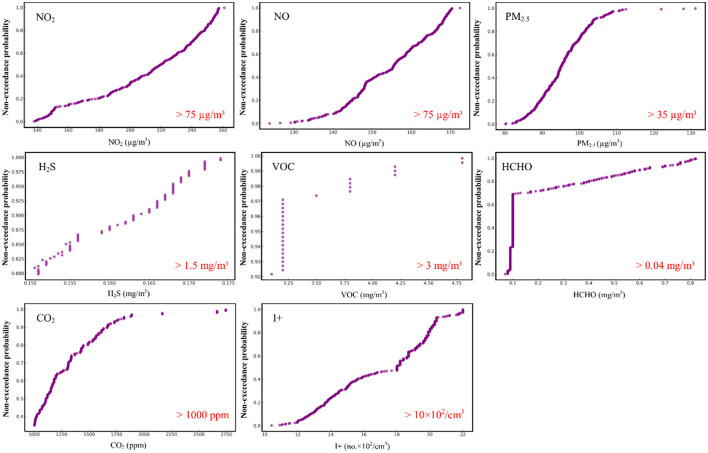
The empirical probability that the NO2, NO, PM2.5, H2S, VOC, HCHO, CO2, and I+ values exceed the limits imposed by the international standards in force.
A pollutant that recorded significant exceedances is PM2.5, for which the maximum daily measured values exceed 80 μq/m3 on all days. Thus, the value of 80.1 μq/m3 was given an EDF index of 0.002 (which indicates the fact that this value is very likely to be exceeded), the value of 100 μq/m3 has an index of 0.74, while 122 μq/m3 has a fairly low probability of exceeding, 0.993. As previously shown, both the NO2 and NO values have significantly exceeded the international standards that regulate the values of these pollutants at 75 μq/m3 in terms of daily variations. The obtained results indicate that in all the analyzed days, the values exceeded this limit at least once. The concentration twice the allowed limit was given an EDF index of 0.095 in the case of NO2 and 0.388 in the case of NO, and the value of 170 μq/m3 has an index of 0.185 in the case of NO2 and 0.979. At the same time, regarding NO2, the value of 200 μq/m3 was assigned an index of 0.333 and the value of 250 μq/m3, 0.837. H2S recorded maximum values above the allowed thresholds only in 74 days of monitoring. The probability that the values exceed the threshold of 0.15 mg/m3 is 20% (0.809 EDF index), and that the values exceed 0.170 mg/m3, the index rises to 0.968 (~3%). Regarding VOC, the allowed thresholds were exceeded in only 29 days by the maximum values of the pollutant. The probability that the values exceed 3.1 mg/m3 is only 7.8% (0.922 EDF index), and the probability that the values are at 4.2 mg/m3 is 1.3% (0.987 EDF index). In the case of CO2, values exceeding the standards of 1,000 ppm were recorded in 238 days. The EDF index for values exceeding 1,000 ppm is 0.349, for exceeding 1,500 is 0.818, while the index for them reacting to 2,167 ppm concentration is 0.974 (Figure 9).
EDF indices reveal significant and frequent exceedances of international standards for various pollutants, including HCHO, PM2.5, NO2, NO, H2S, VOC, and CO2. Given the direct impact on occupant health and comfort, these findings highlight the need for proper IAQ management.
The data also highlights several challenges and critical issues related to the indoor environment that require increased attention and appropriate action; of which it is essential to identify the specific sources of pollution and implement effective strategies to reduce them.
4 Discussion
The results obtained indicate that the pollutants that raise the greatest challenges for maintaining a healthy environment in the analyzed classroom are HCHO, VOC, PM2.5, NO, and NO2, due to concentrations above the thresholds allowed by the international standards in force.
Although the values of the five indicators registered some excesses, the question arises to what extent these exceedances have the characteristic of acting individually to induce health problems, even if all the other indicators are within normal limits. Some studies in the field support this, because it is well-known that HCHO is a respiratory irritant and is classified as a carcinogen, being associated with nasopharyngeal cancer and leukemia (77); PM2.5 can penetrate the lungs, causing cardiovascular and respiratory diseases, and is linked to increased mortality rates (78); NO2 is a respiratory irritant that can aggravate lung diseases and trigger asthma attacks (79); NO can contribute to the formation of ground-level ozone, which has negative health effects (80) and VOCs can cause headaches, dizziness, respiratory irritation and liver and kidney damage, some of which are carcinogenic (8).
Four of the five indicators that recorded high values (HCHO, VOC, NO, and NO2) behave independently of the time of day, showing large or small variations without being influenced by the time. In contrast, PM2.5 values (as well as PM5 and PM10) showed discontinuous maxima between 8 am and 8 pm from Monday to Friday, the period corresponding to the students' course schedule, suggesting the significant influence of human activity on these parameters (81, 82). In addition, human activity causes a slight increase in temperature (T) and a decrease in relative humidity (RH) in certain situations, as well as a considerable increase in CO2 levels and a slight decrease in O2 concentration. These exceeding of the allowed limits are supported by the specialized literature. A study by Ateş and Khameneh (83) demonstrated that classroom temperatures can exceed thermal comfort thresholds, and CO2 concentrations can reach alarming levels in the absence of adequate ventilation. Similarly, research by Kapalo et al. (84) highlighted a significant increase in CO2 levels during intense activities in classrooms, highlighting the crucial need for compliant HVAC systems.
In addition to identifying the pollutants that registered values above the permitted thresholds, a better understanding of the origin of these values is also of great importance. Thus, the evaluation of the way in which the five targeted pollutants interrelate and are determined by the other indoor pollutants through linear regression analysis (LR), indicated a limited ability to explain the variations in the levels of HCHO, VOC, PM2.5, NO2, and NO based-on variables. For the most part, the LP analysis shows that the influence of the large pollutants inside the classroom is quite small on the values of the indicators, even insignificant in some cases. The R2 levels are very low for each pollutant in relation to HCHO (average R2−0.057), VOC (average R2−0.059), and PM2.5 (average R2−0.082). NO had an average R2 coefficient of 0.3315, suggesting that the relationships between the other pollutants and NO are not very predictive and does not explain the model. At the same time, neither the values of other pollutants, such as O3, CO2, or NO2, do not significantly explain the variation in NO levels. The average R2 coefficient for NO2 is only 0.227, showing a modest predictive relationship with other pollutants such as O3, CO, and SO2. This suggests that while there are interactions between various atmospheric pollutants influenced by weather conditions and other external factors, the relationships are relatively weak.
It is well-known that the pollutants inside the classrooms are often influenced by external sources. The studies of Kaewrat et al. (85), Gaffin et al. (86), and Villanueva et al. (87) indicate that HCHO, VOC, PM2.5, NO, and NO2 values in classrooms can be significantly influenced by external sources, suggesting a strong correlation between the outside and the inside. In the case of the current study, based on the data obtained from the national air quality monitoring network, it was identified that there is also a weak correlation between PM2.5 concentrations outside and inside, with an R2 coefficient of ~0.36. This indicates a possible influence of outdoor pollutants on IAQ, suggesting that outdoor sources of PM2.5 may contribute to a certain extent to indoor levels, although the relationship is not very strong and does not explain much of the variation. In contrast, the influences of outdoor NO and NO2 on indoor levels are negligible, with correlation coefficients approaching zero. The authorized bodies of the Municipality of Oradea do not intend to determine the concentration of VOC or HCHO, therefore correlations could not be made between these outdoor and indoor indicators. But analyzing the links between other pollutants (O3, SO2, CO, etc.), the results also indicate weak correlations between outdoor and indoor concentrations. Therefore, we can only deduce the fact that the external concentrations of HCHO and VOC have a low influence on the interior too, especially since the classroom is at a significant distance from the main street; its influence being relatively low.
The aspects presented above indicate that the pollutants most likely have an internal source of emission (with a small exception regarding PM2.5). HCHO and VOC are often emitted by building materials and new furniture, as well as cleaning products and paints (69, 87). At the same time, the two can be emitted by electronic equipment, including computers. Studies show that certain synthetic materials and adhesives used in the manufacture of electronic equipment can emit these chemical substances (88). PM2.5 is determined by the activity of the students, who displace the sedimented particles inside and bring others from outside. High NO and NO2 values are often associated with unventilated heating sources, and studies indicate that NO and NO2 concentrations can be significantly higher in classrooms in modern buildings due to insufficient ventilation and emissions from internal sources (89).
Although the recorded values do not greatly and frequently exceed the standards in force and although students spend a limited amount of time indoors, pollutants can have a significant impact on students' wellbeing and performance. Studies show that continuous exposure to these chemicals can lead to fatigue and decreased ability to concentrate in students (69). They can also cause irritation of the eyes, nose and throat, headaches, and respiratory symptoms, exacerbate asthmatic symptoms in children (90), ultimately leading, according to some studies, to absenteeism (91).
Since the vast majority of pollutants most likely have internal sources, advanced occupancy monitoring and improved system operations could play a crucial role in managing indoor air quality. By integrating real-time occupancy sensors and their integration with HVAC systems, it is possible to optimize ventilation and air purification systems to respond dynamically to changes in room occupancy (92). This approach not only helps maintain pollutant levels within safe limits but also enhances energy efficiency by reducing unnecessary HVAC operation during low-occupancy periods (93). Additionally, investigating the specific activities or sources within classrooms that contribute to pollutant levels can help in developing targeted interventions. For example, reducing the use of certain materials or improving cleaning practices could mitigate the presence of allergens and other pollutants.
5 Limitation of the study
As in the case of any scientific approach, this study also presents some limits that must be considered for a correct interpretation of the results and to guide future research.
The first point concerns the limitations of LR and the ARIMA model. The LR values indicated a limited ability to explain the variability in HCHO, VOC, PM2.5, NO, and NO2 levels based on other variables within the classroom. Consequently, this suggests that there are likely other significant factors influencing these pollutant levels that were not accounted for in the model. The ARIMA model, although useful for short-term forecasting, can suffer from shortcomings related to unexpected changes in classroom use or environmental conditions that are not accounted for by the model. In the specific context of the characteristics of the building and the functionalization of the room analyzed, the quantification of the operating time of the HVAC system was impractical, considering its autonomous operation with respect to the presence of occupants. The occupancy rate varied significantly, which prevented rigorous record keeping. Additionally, the status of windows could not be quantified due to limited control over them. These aspects are complemented by other potential individual indicators, which, being independent of the authors, could not be effectively monitored.
Another critical aspect is the methodology for assigning weights to different indicators in the IMQI calculation. The weights assigned to the pollutants were established based on the literature, but this approach can be subjective and may vary depending on the sources used. At the same time, the influence of IAQ is quite relative and depends a lot on pre-existing conditions and the general condition of each individual.
At the same time, the use of standards (especially the US EPA for PM2.5 and PM10) and reporting basis (US EPA's IMQI scale) created for the outdoors in the indoor environment presents significant limitations due to the differences between the two environments. These standards do not consider the difference in exposure time, different pollution sources (building materials, furniture, and daily indoor activities), ventilation rates, and efficiency of air filtration systems, etc. But considering the fact that for certain pollutants and reporting scales there are no internationally accepted standards, or due to the fact that they are not enough research to represent a benchmark, in some studies it is necessary to use adapted thresholds. But on the other hand, the similar interior-exterior thresholds leave the possibility of a better comparative analysis.
6 Conclusions
Critical indicators for health, such as HCHO, VOC, PM2.5, NO, and NO2, frequently exceeded the limits allowed by the international standards in force, highlighting a significant environmental problem. Thus, HCHO concentrations exceed the daily international standards in force by 49.4%, VOC by 69.2%, PM2.5 by 99.5%, NO2 by 78.8%, and NO by 56.3%. All these parameters have a significant potential to harm human health. Although exceedances of international standards have also been recorded for other pollutants (T, RH, CO2, I+, I-, SO2, and PM5), these exceedances are sporadic and without a high harmful potential. It is worth noting that, despite these excesses, the limited time spent by students and teachers inside the classroom mitigates the impact of the internal microclimate on health, rather causing a decrease in concentration and the ability to perfect certain tasks.
Analysis of forecasts of pollutant levels for the coming year suggests the stabilization of CO2, CO, HCHO, TVOC, and O2 concentrations, while SO2 and NO levels could increase. It is essential to continuously monitor these pollutants to prevent exceeding the thresholds set by international standards. The highest EDFs are recorded for HCHO, PM2.5, NO2, and NO, underlining the need to implement adequate air quality control measures. The IMQI index was used to quantify indoor environmental effects on human health. The obtained values revealed that, although some of the pollutants are above the permitted limits, the general state of the microclimate was kept in the range of 0–50 in most cases, indicating the good quality of the microclimate and low health risks.
To improve IAQ it is crucial to improve ventilation systems and reduce pollution sources in the classroom by using less polluting building materials and furniture and implementing more efficient monitoring and air cleaning equipment. Future studies in this classroom will include assessing the perception of the students working here through a questionnaire, analyzing the bacterial and fungal microflora at the species and genus level, and correlating it with potential conditions determined. A direct relationship between IMQ quality and student academic performance will also be established.
Data availability statement
The raw data supporting the conclusions of this article will be made available by the authors, without undue reservation.
Author contributions
TC: Conceptualization, Formal analysis, Methodology, Supervision, Writing – original draft. AI: Data curation, Investigation, Validation, Writing – original draft. ZB: Funding acquisition, Project administration, Resources, Writing – original draft. HA-H: Software, Visualization, Writing – review & editing. DI: Data curation, Supervision, Writing – original draft. BS: Methodology, Writing – review & editing. TH: Investigation, Validation, Visualization, Writing – review & editing. GH: Conceptualization, Formal analysis, Writing – original draft. NH: Data curation, Investigation, Writing – review & editing. BB: Data curation, Software, Writing – review & editing. AP: Data curation, Writing – review & editing.
Acknowledgments
The research undertaken was made possible by the equal scientific involvement of all the authors concerned.
Funding Statement
The author(s) declare financial support was received for the research, authorship, and/or publication of this article. The research has been funded by the University of Oradea, Romania. This work was partially supported by the Deanship of Scientific Research, Vice Presidency for Graduate Studies and Scientific Research, King Faisal University, Saudi Arabia (Grant No. KFU241371).
Conflict of interest
The authors declare that the research was conducted in the absence of any commercial or financial relationships that could be construed as a potential conflict of interest.
Publisher's note
All claims expressed in this article are solely those of the authors and do not necessarily represent those of their affiliated organizations, or those of the publisher, the editors and the reviewers. Any product that may be evaluated in this article, or claim that may be made by its manufacturer, is not guaranteed or endorsed by the publisher.
References
Articles from Frontiers in Public Health are provided here courtesy of Frontiers Media SA
Citations & impact
This article has not been cited yet.
Impact metrics
Alternative metrics

Discover the attention surrounding your research
https://www.altmetric.com/details/166233898
Similar Articles
To arrive at the top five similar articles we use a word-weighted algorithm to compare words from the Title and Abstract of each citation.
A review of relevant parameters for assessing indoor air quality in educational facilities.
Environ Res, 261:119713, 31 Jul 2024
Cited by: 0 articles | PMID: 39094896
Review
Indoor air quality in green buildings: A case-study in a residential high-rise building in the northeastern United States.
J Environ Sci Health A Tox Hazard Subst Environ Eng, 50(3):225-242, 01 Jan 2015
Cited by: 7 articles | PMID: 25594117
Indoor air quality in the primary school of China-results from CIEHS 2018 study.
Environ Pollut, 291:118094, 01 Sep 2021
Cited by: 4 articles | PMID: 34517175
Indoor Exposure to Selected Air Pollutants in the Home Environment: A Systematic Review.
Int J Environ Res Public Health, 17(23):E8972, 02 Dec 2020
Cited by: 75 articles | PMID: 33276576 | PMCID: PMC7729884
Review Free full text in Europe PMC