Abstract
Free full text

Exploring the human genome with functional maps
Abstract
Human genomic data of many types are readily available, but the complexity and scale of human molecular biology make it difficult to integrate this body of data, understand it from a systems level, and apply it to the study of specific pathways or genetic disorders. An investigator could best explore a particular protein, pathway, or disease if given a functional map summarizing the data and interactions most relevant to his or her area of interest. Using a regularized Bayesian integration system, we provide maps of functional activity and interaction networks in over 200 areas of human cellular biology, each including information from ~30,000 genome-scale experiments pertaining to ~25,000 human genes. Key to these analyses is the ability to efficiently summarize this large data collection from a variety of biologically informative perspectives: prediction of protein function and functional modules, cross-talk among biological processes, and association of novel genes and pathways with known genetic disorders. In addition to providing maps of each of these areas, we also identify biological processes active in each data set. Experimental investigation of five specific genes, AP3B1, ATP6AP1, BLOC1S1, LAMP2, and RAB11A, has confirmed novel roles for these proteins in the proper initiation of macroautophagy in amino acid-starved human fibroblasts. Our functional maps can be explored using HEFalMp (Human Experimental/Functional Mapper), a web interface allowing interactive visualization and investigation of this large body of information.
The completion of the Human Genome Project and the subsequent flood of genomic data and analyses have provided a wealth of information regarding the entire catalog of human genes. Comprehensive assays of gene expression, protein binding, genetic interactions, and regulatory relationships all provide snapshots of molecular activity in specific cell types and environments, but turning these biomolecular parts lists into an understanding of pathways, processes, and systems biology has proven to be a challenging task. This abundance of data can sometimes obscure biological truths: The size of the human genome, the complexity of human tissue types and regulatory mechanisms, and the sheer amount of available data all contribute to the analytical complexity of understanding human functional genomics.
In order to take advantage of large collections of genomic data, they must be integrated, summarized, and presented in a biologically informative manner. We provide a means of mining tens of thousands of whole-genome experiments by way of functional maps. Each map represents a body of data, probabilistically weighted and integrated, focused on a particular biological question. These questions can include, for example, the function of a gene, the relationship between two pathways, or the processes disrupted in a genetic disorder. Functional integrations investigating individual genes' relationships have been successful with smaller data collections in less complex organisms (Lee et al. 2004; Date and Stoeckert Jr. 2006; Myers and Troyanskaya 2007), although (as discussed below) it is particularly challenging to scale these techniques up to the size and complexity of the human genome. Each functional map, based on an underlying predicted interaction network, summarizes an entire collection of genomic experimental results in a biologically meaningful way.
While functional maps can readily predict functions for uncharacterized genes (Murali et al. 2006), it is important to take advantage of the scale of available data to understand entire pathways and processes. Cross-talk and coregulation among pathways, processes, and genetic disorders can be mapped by analyzing the structure of underlying functional relationship networks. This includes the association of disease genes with (potentially causative) pathways; for example, many known breast cancer genes are involved in aspects of the cell cycle and DNA repair, and novel associations of this type can be mined from high-throughput data. Similarly, associations between distinct but interacting biological processes (e.g., mitosis and DNA replication) can be quantified by examining functional relationships between groups of genes, allowing the identification of proteins key to interprocess regulation.
The functional maps we provide for the human genome include information on protein function, associations between diseases, genes, and pathways, and cross-talk between biological processes. These are all based on probabilistic data integration using regularized naïve Bayesian classifiers. Naïve Bayesian systems have been used successfully to analyze protein–protein interaction (PPI) data (Rhodes et al. 2005; von Mering et al. 2007), whereas our focus is on functional relationships and the biological roles of gene products. Prior work performing functional integration in simpler organisms with smaller data collections (Date and Stoeckert Jr. 2006; Myers and Troyanskaya 2007) has been similarly successful; see Supplemental Text 1 for a complete discussion. Such integrations have not previously been scaled biologically (i.e., to complex metazoans) or computationally (over very large genomic data collections) to provide a functional view of the human genome driven purely by experimental results. In addition to challenges of computational efficiency in the presence of hundreds of genome-scale data sets, naïve classifiers assume that all input data sets are independent; this becomes increasingly untrue and problematic as more data sets are analyzed, resulting in a paradox of decreasing performance with increasing training data. To address this, we use Bayesian regularization (Steck and Jaakkola 2002), a process by which an observed distribution of data can be combined with a prior belief in a principled manner. Intuitively, this results in groups of data sets containing similar information making a more modest contribution to the integration process, up-weights unique data sets, and prevents overconfident predictions. Our regularization of the naïve classifier parameters using a score based on mutual information up- and down-weighted appropriate subsets of data, maintaining both efficiency and accuracy.
We applied our functional maps to a specific biological question in the area of autophagy, the process by which a cell can recycle its own biomass under conditions of starvation or stress (Klionsky 2007). Among many proteins predicted to participate in this biological process by our maps, we chose to investigate AP3B1, ATP6AP1, BLOC1S1, LAMP2, and RAB11A in the laboratory. We demonstrated through multiple lines of experimental evidence that these proteins are indeed involved in macroautophagy in amino acid-starved human fibroblasts, a specific type of autophagy in which bulk cytoplasm is lysosomally degraded. The results of our integration are available through a web-based interface, HEFalMp (Human Experimental/Functional Mapper), at http://function.princeton.edu/hefalmp. This tool allows a user to interactively explore functional maps integrating evidence from thousands of genomic experiments, focusing as desired on specific genes, processes, or diseases of interest.
Results
Using the system outlined in Figure 1A, we generate functional maps of predicted gene functions, pathway and process associations, and genetic disorders focused on 229 biological processes, incorporating information from ~30,000 genome-scale experiments. Within each biological area, maps are derived from a functional relationship network predicted using regularized Bayesian integration of the genomic data. The features and contents of the resulting interaction networks are analyzed to produce gene-, process-, and disease-centric functional maps specific to each biological area. We have experimentally confirmed five genes newly predicted to be active in the area of macroautophagy, AP3B1, ATP6AP1, BLOC1S1, LAMP2, and RAB11A.
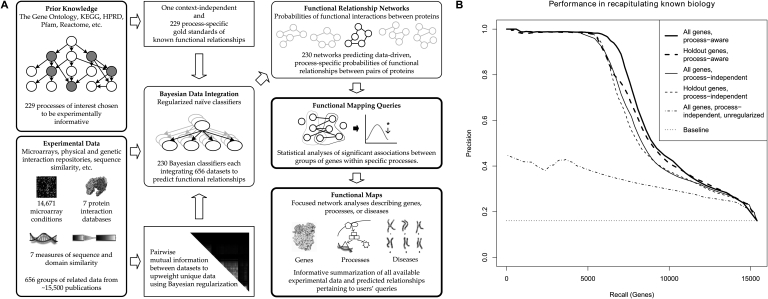
Overview and performance of genomic data integration for functional mapping. (A) Data from ~30,000 genome-scale experiments (~15,000 microarray conditions and ~15,000 interaction and sequence-based assays) were organized into 656 related data sets (Supplemental Table 1). These data sets were used as inputs for 229 process-specific naïve Bayesian classifiers each trained to predict functional relationships specific to a particular biological area and one process-independent global classifier. Mutual information was calculated between each pair of data sets and used to regularize these classifiers and prevent overconfident predictions. Each classifier was used to infer a predicted functional relationship network for a particular biological process. These networks were then analyzed to find statistically significant sets of functional relationships spanning gene groups of interest. This results in functional maps focusing on individual genes, groups of genes, biological processes, or genetic disorders. Each map provides an informative summarization of the genomic data collection focused on the current biological entity of interest. (B) Performance of predicted functional relationship networks in recapitulating known biology. To confirm that the predicted functional relationships underlying our functional maps were accurate, we scored their ability to recover information from a held-out portion (25% of genes) of our gold standard. This evaluation includes the global process-independent network tested on all genes and the holdout set, a process-aware global mean of the process-specific networks tested on all genes and the hold-out set, and an unregularized global process-independent network tested on all genes. Ranking of functionally related gene pairs is performed by comparing predicted probabilities based on data integration with the known relationships in the held-out test set. Results for individual process-specific networks appear in Supplemental Figure 1 and Supplemental Table 3. Precision is well above baseline, and since naïve classifiers are generally robust to overfitting, performance of the hold-out set is only slightly below that of the entire genome. Bayesian regularization provides a large performance increase at low recall by preventing overconfident predictions.
Data integration for functional mapping
A functional map is a view of genomic data focused on a particular area of interest: genes, processes, diseases, and their associations and interrelationships. To derive these maps, we analyze functional relationship networks predicted based on Bayesian integration of ~30,000 genome-scale experiments (Supplemental Table 1). These are organized into 656 data sets (grouped by related microarray experiments, individual interaction databases, and so forth) and probabilistically weighted based on their functional activity in 229 biological processes of interest (e.g., autophagy, mitotic cell cycle, protein processing, etc.). As summarized in Table 1 and Supplemental Table 2, one product of this integration process is an estimate of the biological processes active in each data set. Further, as highlighted in Table 2, over 25% of our predicted functional relationships are supported by at least 100 data sets, and many genes' predictions include information from over 500 genome-scale data sets.
Table 1.
Summary of integrated genomic data
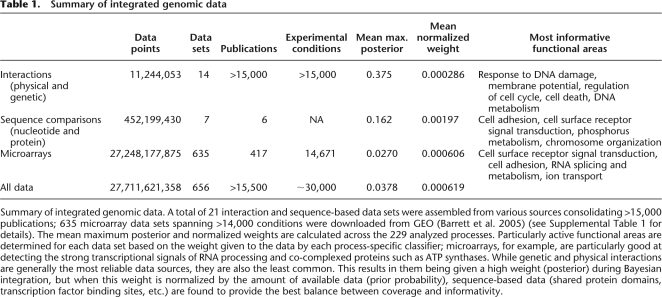
Summary of integrated genomic data. A total of 21 interaction and sequence-based data sets were assembled from various sources consolidating >15,000 publications; 635 microarray data sets spanning >14,000 conditions were downloaded from GEO (Barrett et al. 2005) (see Supplemental Table 1 for details). The mean maximum posterior and normalized weights are calculated across the 229 analyzed processes. Particularly active functional areas are determined for each data set based on the weight given to the data by each process-specific classifier; microarrays, for example, are particularly good at detecting the strong transcriptional signals of RNA processing and co-complexed proteins such as ATP synthases. While genetic and physical interactions are generally the most reliable data sources, they are also the least common. This results in them being given a high weight (posterior) during Bayesian integration, but when this weight is normalized by the amount of available data (prior probability), sequence-based data (shared protein domains, transcription factor binding sites, etc.) are found to provide the best balance between coverage and informativity.
Table 2.
Features of functional relationship networks predicted from data integration
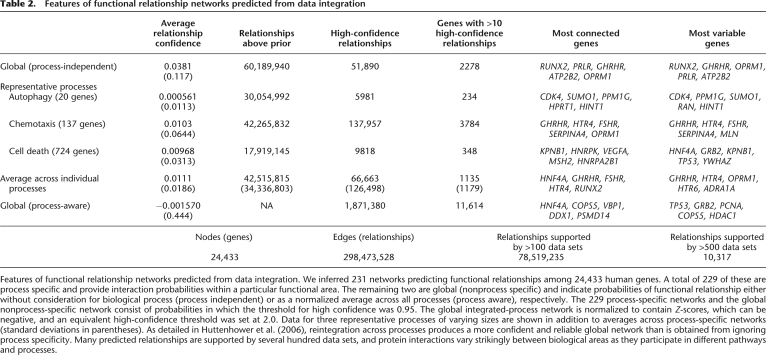
Features of functional relationship networks predicted from data integration. We inferred 231 networks predicting functional relationships among 24,433 human genes. A total of 229 of these are process specific and provide interaction probabilities within a particular functional area. The remaining two are global (nonprocess specific) and indicate probabilities of functional relationship either without consideration for biological process (process independent) or as a normalized average across all processes (process aware), respectively. The 229 process-specific networks and the global nonprocess-specific network consist of probabilities in which the threshold for high confidence was 0.95. The global integrated-process network is normalized to contain Z-scores, which can be negative, and an equivalent high-confidence threshold was set at 2.0. Data for three representative processes of varying sizes are shown in addition to averages across process-specific networks (standard deviations in parentheses). As detailed in Huttenhower et al. (2006), reintegration across processes produces a more confident and reliable global network than is obtained from ignoring process specificity. Many predicted relationships are supported by several hundred data sets, and protein interactions vary strikingly between biological areas as they participate in different pathways and processes.
Using only the information in these predicted functional relationship networks before they have been further processed into functional maps, we can accurately recapitulate known biology from catalogs such as the Gene Ontology (GO). Specifically, Figure 1B quantifies the performance of the data integration process in probabilistically ranking related gene pairs, and Supplemental Figure 1 and Supplemental Table 3 decompose this performance on a per-GO-term basis. As observed in Myers and Troyanskaya (2007), functional integration benefits substantially from context awareness, a fact we take advantage of in our use of process-specific functional maps. Performance differs only slightly between an evaluation of the entire genome and of a held-out test set, demonstrating naïve classifiers' robustness to overfitting. Most significantly, Bayesian regularization provides a dramatic increase in performance by down-weighting groups of similar data sets and up-weighting unique, informative data sets in each biological process.
Features of the functional relationship networks
Functional maps are generated by analysis of functional relationship networks, and each network is based on probabilistic integration of genomic data within a particular biological area. In addition to providing maps of higher-order associations among processes and diseases, these functional relationship networks can be examined directly to provide insights into protein function, functional modules, and characteristics of the integrated experimental data. Table 2 presents summary statistics for several of the networks we analyzed. A substantial fraction (26%) of the networks' edges are supported by evidence from more than 100 data sets, and ~10,000 edges are supported by over 500 data sets. There is strong variation in probabilities and data set weighting between biological processes, with the most confident coverage offered by reintegration across all available processes. While different genes tend to be highly connected in each process-specific network, commonalities emerge in the global networks and interprocess averages. These proteins (HNF4A, RUNX2, GHRHR, and others from the rightmost table column) tend to be components of complexes or receptors; they are thus predicted to have a relatively small number of extremely confident relationships with their other complex members or ligands. This is confirmed by the fact that these genes are also among the most variable, although their predictions are not generally supported by the most data sets. Instead, to find these particular relationships, subsets of appropriately reliable data are up-weighted by our integration system in a process-specific manner.
Individual functional relationship networks can also be used to predict protein function using “guilt by association,” as diagrammed in Figure 2A. If a gene has many strong, specific predicted relationships with genes in a particular biological process, it is itself likely to participate in that process (Supplemental Table 4). ALOX5AP, for example, is a membrane protein required to activate ALOX5 for leukotriene synthesis; this pathway is a clinical target for the treatment of asthma, heart disease, and obesity (Peters-Golden and Brock 2003; Mehrabian et al. 2005). Our integration system predicts it to have many specific functional relationships with other membrane proteins involved in the inflammatory chemotaxis response in leukocytes (among other predicted relationships). While neither ALOX5AP nor ALOX5 are annotated to a chemotactic pathway in the Gene Ontology, one of their immediate biosynthetic products, LTB4, is a known activator of chemotaxis (Peters-Golden and Brock 2003). This is an example of uncovering an uncataloged protein function by functional mapping, and we provide details below of our experimental confirmation of novel predicted functions for LAMP2 and RAB11A in autophagy.
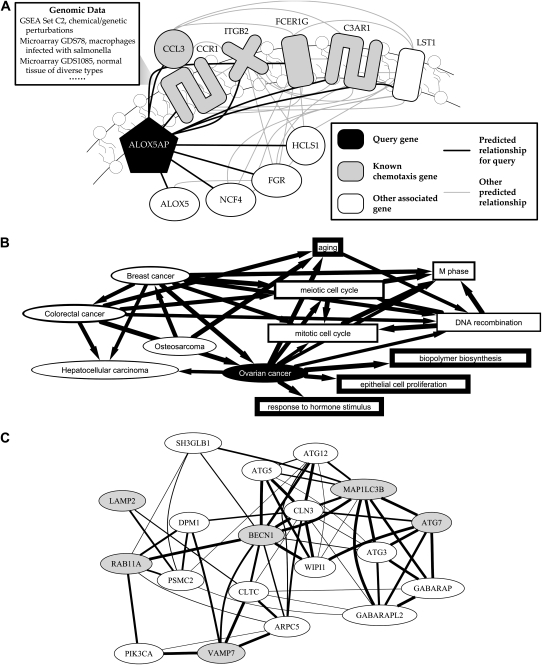
Results of functional mapping from data integration. Functional maps derived from experimental data integration provide information on groups of genes, including cross-talk between pathways, processes, and genes associated with genetic disorders. In all figure parts, thicker edges indicate stronger associations. (A) The process-specific functional relationship networks underlying functional maps can themselves provide information on individual genes' and modules' behavior in the underlying genomic data. Focusing on ALOX5AP, a membrane protein participating in leukotriene synthesis highlights a predicted association with the process of chemotaxis in leukocytes, driven by multiple predicted relationships with known chemotaxis proteins. This represents an instance of functional under-annotation; while ALOX5AP has not been formally cataloged as participating in chemotaxis, its immediate biosynthetic product LTB4 is a known activator of chemotaxis (Peters-Golden and Brock 2003). (B) Associations between genetic disorders and biological processes. To validate functional mapping's ability to discover disease/process associations from data, a focus on ovarian cancer—known to be influenced by at least seven genes (Online Mendelian Inheritance in Man 2008)—we predict associations with the cell cycle, cell proliferation, and hormone stimulus, as well as with several other cancers. These associations are each based on relationships among individual genes predicted from integrated genomic data; directed arrows point to the gene group in which the background connectivity was calculated. As above, additional novel predictions can be explored online using HEFalMp. (C) Visualization of a functional map generated by querying a custom gene set. We chose to focus on the known autophagy proteins ATG7, BECN1, and MAP1LC3B, in addition to genes of interest LAMP2, RAB11A, and VAMP7, in the context of autophagy. This extracts two clear clusters of predicted autophagy-specific functional relationships, one consisting mainly of known autophagy proteins and one enriched for ER/Golgi and vesicular trafficking proteins (including the three test genes). This led us to experimentally test and confirm the hypothesis that LAMP2 and RAB11A (as well as AP3B1, ATP6AP1, and BLOC1S1) are involved in macroautophagy in amino acid-starved human fibroblasts.
By extracting highly connected clusters from functional relationship networks, we can also discover putative functional modules showing high similarity in experimental data without being directly associated with preannotated gene sets or processes. These modules may represent novel pathways, complexes, or other groups of proteins interacting to carry out cellular tasks. The modules can be merged to create a hierarchical structure reminiscent of catalogs such as the Gene Ontology; a small subset of our predicted functional modules appears in Supplemental Figure 2. We have automatically mined and hierarchically organized ~17,000 functional modules from our integrated data, spanning all ~25,000 genes for which we have data and ranging in size from three to 5600 genes (Supplemental Table 5; Supplemental Fig. 3).
Functional associations: Genetic disorders and biological processes
By examining the behavior of entire pathways in integrated genomic data, we derive functional maps of cross-talk between related biological processes (Supplemental Fig. 4). Just as functional relationships between genes are predicted by finding significant agreement among many integrated data sets, functional associations between processes are discovered by observing strong relationships among many of their constituent genes, based on similar behavior of the processes' genes in many genomic data sources and not on prior knowledge of genes shared between processes. Maps associating interrelated biological processes (and detailing the proteins predicted to drive those associations) can be derived from high-throughput data for any biological area of interest. This provides a way of exploring pathway cross-talk in genomic data and quickly identifying potential regulatory hubs.
In a similar manner, groups of known disease-related genes can be associated with each other or with (potentially causative) pathways and processes. An example in Figure 2B focuses on ovarian cancer, currently recorded in OMIM (Online Mendelian Inheritance in Man 2008) as being influenced by at least seven genes. While known shared genes drive some of these associations (e.g., MSH6 in aging or ERBB2 in epithelial cell proliferation), others are more surprising. For example, AKT1, a protein known to contribute to ovarian cancer, is predicted to be related to B3GNTL1 and PHKG2 in biopolymer biosynthesis (i.e., DNA synthesis) due mainly to high microarray correlation across a wide variety of conditions; these proteins are also involved in the estrogen and insulin pathways, respectively, signaling systems that have been observed to interact (Hamelers and Steenbergh 2003). This is an example in which functional mapping provides a small set of specific proteins that may serve as regulatory hubs joining two or more interacting pathways. Similarly, while there is a growing understanding of the link between breast and ovarian cancer and hormone stimulus (Dumeaux et al. 2005), we predict explicit molecular connections driven by LYN, EIF2B5, and MMS19L. We also observe links between ovarian cancer and other cancers, including breast cancer, osteosarcoma, colorectal cancer, and hepatocellular carcinoma, mainly due to interactions or high microarray correlation with BRCA1, MSH6, and other known cancer-related proteins. Functional mapping can thus call out potentially overlooked associations between diseases as well as posit new molecular connections between biological processes and genetic disorders.
Finally, if an investigator has a specific biological hypothesis in mind, it can be explored using functional mapping of user-provided gene sets. Figure 2C demonstrates a query of known autophagy genes, ATG7, BECN1, and MAP1LC3B, with test genes, LAMP2, RAB11A, and VAMP7, in the context of autophagy. This produces two clear clusters, a group of known autophagy genes related to a group of vesicular and transport genes (including the three test genes). These two clusters are associated primarily by RAB11A/BECN1, CLTC/BECN1, ARPC5/CLN3, and SH3GLB1/MAP1LC3B relationships, as well as less heavily weighted links through DPM1 and PSMC2. The four primary relationships are driven by a wide variety of microarray correlations, led by data sets investigating retinal pigment epithelium (Tian et al. 2004), macrophage infection (Detweiler et al. 2001), bone marrow (Graf et al. 2002), and DNA damage (Rieger and Chu 2004). The secondary relationships are also predicted based on diverse microarray data and information from the GSEA gene sets (Subramanian et al. 2005). All of these genes are known to be involved in ER/Golgi trafficking, the secretory and vesicular system, and protein degradation; these associations led us to investigate whether LAMP2, RAB11A, and VAMP7 play roles in the specific activation of macroautophagy. Our experimental confirmation of two of these predictions is detailed below.
AP3B1, ATP6AP1, BLOC1S1, LAMP2, and RAB11A are required for macroautophagy in human fibroblasts
Autophagy is the process by which cells can consume their own biomass in order to survive when starved or otherwise stressed. Particularly in human biology, it is an area of active research, with recent work discovering links to tumorigenesis and bacterial infection (Klionsky 2007). Specifically, macroautophagy is the process of engulfing and degrading the contents of bulk cytoplasm, while chaperone-mediated autophagy and microautophagy use different mechanisms to target specific proteins to the lysosome (Yorimitsu and Klionsky 2005). We will use the terms autophagy and macroautophagy interchangeably, as we focus here only on macroautophagy. We chose to experimentally investigate six proteins predicted to function in autophagy: three from an early version of our maps, LAMP2, RAB11A, and VAMP7, and three from the final version, AP3B1, ATP6AP1, and BLOC1S1. Previous work has shown these proteins to be involved in the lysosome and vesicular trafficking (Chen et al. 1985; Prekeris et al. 2000; Ward et al. 2000; Starcevic and Dell'Angelica 2004; Chapuy et al. 2008), with LAMP2 playing a known role in chaperone-mediated autophagy (Cuervo and Dice 1996), but they have not been specifically associated with macroautophagy. Punctate localization of the MAP1LC3 protein to autophagy-specific vesicles known as autophagosomes and its cleavage from the MAP1LC3-I to the MAP1LC3-II isoform are common markers for cells undergoing autophagy; both of these markers are obviated by the inhibition of proteins necessary for autophagy, e.g., ATG5 (Kabeya et al. 2000; Mizushima et al. 2004). We found these markers to be decreased in primary human fibroblasts, in which five of these six proteins (AP3B1, ATP6AP1, BLOC1S1, LAMP2, or RAB11A) have been knocked down by siRNA, suggesting that these proteins are required for successful autophagy (Fig. 3).
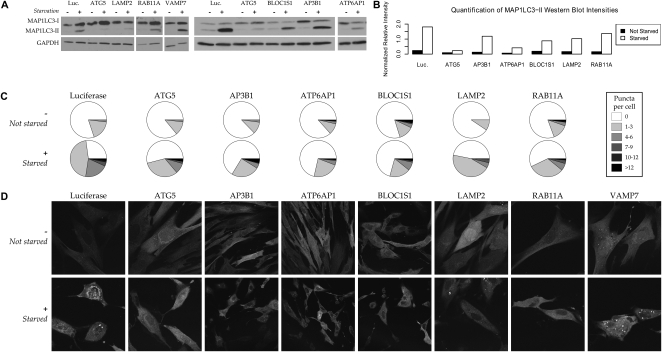
Impaired autophagosome formation confirms the predicted involvement of AP3B1, ATP6AP1, BLOC1S1, LAMP2, and RAB11A in human macroautophagy. Our functional maps predict AP3B1, ATP6AP1, and BLOC1S1 to be involved in autophagy; an early version also predicted the involvement of LAMP2, RAB11A, and VAMP7 in the process, which recycles cellular biomass in order to survive under conditions of starvation or stress. While VAMP7 knockdowns showed no effect (see Discussion), siRNA knockdowns of the other five proteins inhibited normal autophagy. (A) Measurement of the MAP1LC3-I and autophagosome-bound MAP1LC3-II isoforms by immunoblotting. Under a control condition (luciferase siRNA), starvation (+) induces autophagy in human fibroblasts and up-regulates the autophagy marker MAP1LC3-II; this up-regulation is generally inhibited by knockdown of proteins required for autophagy, e.g., ATG5. (B) Quantification of MAP1LC3-II band intensities. Intensities for each condition are calculated relative to GAPDH using the ImageJ software. Replicates (e.g., controls run on multiple gels) have been averaged when available. (C) Quantification of punctate autophagosome formation. The numbers of fluorescent puncta (MAP1LC3-II-labeled autophagosomes) per cell were averaged over counts from three independent investigators in 10 images per normal (−) or starvation (+) condition, unlabeled and randomized (80 images total; see Supplemental Fig. 5 for standard errors). The resulting distribution of puncta frequencies is low under all nonstarved conditions and significantly increased under a negative control (luciferase) condition. It is only slightly increased for the ATG5 positive control and for the AP3B1, ATP6AP1, BLOC1S1, LAMP2, and RAB11A predictions. (D) Punctate localization of fluorescent GFP-LC3 to the autophagosome during autophagy. Under normal conditions (−), MAP1LC3-I is localized diffusely through the cytoplasm; starvation (+) induces autophagy and localization to the autophagosome membrane. Knockdowns of ATG5 (positive control) or the five validated genes abrogate this localization, indicating that these proteins are required for successful macroautophagy.
AP3B1, ATP6AP1, BLOC1S1, LAMP2, and RAB11A depletions all significantly diminish autophagy as measured by immunoblotting and quantification of fluorescent GFP-tagged MAP1LC3 (Fig. 3; Supplemental Fig. 5). These proteins' siRNA depletions specifically abrogate the processing of MAP1LC3 to the MAP1LC3-II isoform (Fig. 3A,B), which is incorporated into autophagosome membranes during normal macroautophagy. In knockdowns of these five predictions, we also detect a reduction in autophagy by localization of MAP1LC3 to autophagosomes, as quantified by the number of fluorescent MAP1LC3-labeled puncta in a collection of 80 microscopic images (Fig. 3C,D; Supplemental Fig. 5B); this manual quantification is also supported by automated image analysis using CellProfiler (Carpenter et al. 2006; Supplemental Fig. 5A). A VAMP7 knockdown showed no effect in any assay, which is possibly due to known variation in its behavior in different cell types; this is discussed in more detail below. The modest decrease in MAP1LC3-II incurred by the RAB11A knockdown (see Fig. 3A,B), as opposed to its strong fluorescence and localization effect (Fig. 3C,D), raises the interesting possibility that it participates in the formation of autophagosomal membranes containing MAP1LC3 after it has been processed by ATG3 and ATG7 to the MAP1LC3-II isoform (Kabeya et al. 2004). Further investigation is necessary to determine the specific roles of AP3B1, ATP6AP1, BLOC1S1, LAMP2, and RAB11A in mammalian autophagy, but these assays provide strong evidence for their involvement as predicted by functional mapping.
HEFalMp: A web-based interface for interactive functional mapping
Our functional maps can be explored interactively using the HEFalMp (Human Experimental/Functional Mapper) tool at http://function.princeton.edu/hefalmp. As shown in Figure 4, HEFalMp provides an interface through which a user can focus on a particular subject of interest—a gene, group of genes, biological process, or disease—and examine its predicted associations. For example, this can predict gene function (gene/process associations), cross-talk between pathways (process/process associations), or processes associated with genetic diseases, and all predictions can be made in any of the >200 biological areas for which we have constructed functional maps. A variety of visualizations are used for different query types, and all results can be downloaded for offline analysis. All predictions between groups of genes can be expanded into the specific functional relationships driving the analysis, and individual functional relationships can always be traced to the genomic data sets on which they are based. HEFalMp provides a convenient and informative way to explore functional maps summarizing data from ~30,000 genome-scale experiments.
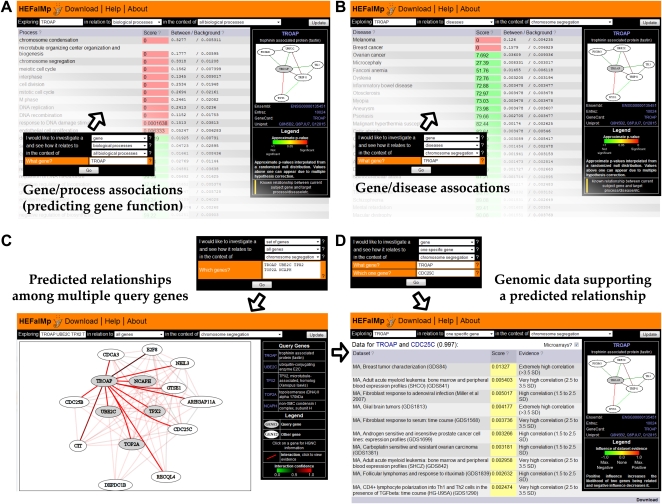
The HEFalMp tool for functional mapping. We have provided a web interface, the Human Experimental/Functional Map (HEFalMp), at http://function.princeton.edu/hefalmp for interactively exploring our predicted functional maps. A user can focus on a gene, gene set, biological process, or genetic disorder of interest and investigate its predicted associations with other genes, processes, or diseases. These predictions are presented using a variety of visualizations, and all data is downloadable for further analysis. (A) Associating a gene with biological processes. An investigator wishes to study which biological processes the TROAP protein is predicted to participate in. (B) Associating a gene with genetic disorders. In the context of one of TROAP's most likely biological processes, chromosome segregation, it is predicted to be particularly associated with genes causing melanomas and breast cancer. (C) Visualizing a predicted functional relationship network for specific genes. Focusing on a gene set consisting of TROAP, two of its most likely relationship partners (UBE2C and TPX2), and two of its most likely partners in chromosome segregation (TOP2A and NCAPH) retrieves a predicted functional relationship network specific to the area of chromosome segregation. (D) Viewing genomic data contributing to a prediction. Clicking on a predicted functional relationship or specifically focusing on TROAP's relationship with CDC25C displays the genomic data used to generate the prediction. Here, TROAP is predicted to relate to CDC25C, a highly conserved mitotic regulator, due to very high correlation between the genes' expression in a variety of microarray conditions. Taken together, this evidence suggests that TROAP is strongly cell cycle regulated and may play an as-yet-uncharacterized role in mitosis.
Discussion
While the growing amount of publicly available genomic data can answer a wide variety of biological questions, usefully integrating, mining, and summarizing these data is an ongoing challenge. Using information from over 650 genome-scale data sets drawn from thousands of publications, we produce functional maps that provide specific information focused on an investigator's area of interest. This can include gene function, functional modules, cross-talk between pathways and processes, or interactions among genetic disorders. We have experimentally confirmed predicted involvements of AP3B1, ATP6AP1, BLOC1S1, RAB11A, and LAMP2 in human macroautophagy, and we provide the HEFalMp web-based interface for biologists to explore our results and to generate new functional maps in their areas of interest.
Applications of functional mapping
Functional mapping can guide further laboratory and computational investigations by taking advantage of large collections of genomic data in a biologically meaningful way. As demonstrated by our confirmation of the participation of five specific proteins in autophagy, functional associations of individual genes with pathways and processes can be used to suggest directed laboratory experiments. In the area of human disease, this can be even more significant, since functional mapping predicts associations of genetic disorders with potentially causative processes and with specific individual genes. It is key that computational methods take advantage of modern high-throughput biology to guide researchers to novel disease genes based on information from thousands of experimental results.
Functional mapping can further leverage high-throughput data to better inform functional cataloging and annotation efforts. As seen above with ALOX5AP, many human proteins have ample literature evidence to link them to established pathways and processes, but have not yet been fully annotated in catalogs such as GO or KEGG. Functional mapping can rapidly direct annotators to such under-annotated genes, providing an opportunity to substantially improve functional catalogs based on existing literature evidence.
Bayesian regularization enables very large-scale data integration
It is notable that previous data integration techniques do not scale adequately to the size of the human genome and the amount of currently available genomic data. Bayesian structure learning has been applied successfully to very small groups of genes with focused datasets (Sachs et al. 2005), but its computational complexity makes it inapplicable on a whole-genome scale. Even TAN classifiers, which are only minimally more complex than naïve networks, can be inefficient to learn from very large, incomplete data collections (Tian et al. 2005). While naïve Bayesian classifiers can perform rapid data integration and can be learned and evaluated very quickly, their inherent independence assumption can produce overly confident predictions in the presence of many data sets (Supplemental Fig. 6). In order to maintain accuracy when dealing with very large data collections, we use Bayesian parameter regularization (Steck and Jaakkola 2002) to assign a uniform prior to each data set with belief inversely proportional to the amount of unique data in the data set. This allows particularly diverse, informative data sets to efficiently provide a stronger contribution to the integration and mapping process.
Mutual information, which we use to evaluate similarities between data sets when performing regularization, also reveals surprising large-scale structure in our collection of genomic data (Fig. 5; Supplemental Table 6). While most data sets share very little information by an absolute measure, small but consistent patterns emerge when considering hundreds of data sets spanning thousands of experimental conditions. Since most available genome-scale data is expression based, microarray platform is one of the broadest factors by which data sets cluster. Within these large platform-based groups, other similarities are detectable based on a variety of factors ranging from tissue type to array normalization algorithm. It is striking that a straightforward data mining measure such as mutual information, when applied to a sufficiently large collection of genome-scale data, can discover various underlying classes of data sets. Even though the amount of information shared based on factors such as array platform is small, its ubiquity violates the independence assumption of naïve classifiers, and it thus provides the basis for the performance improvement that we observe when using regularized parameters.
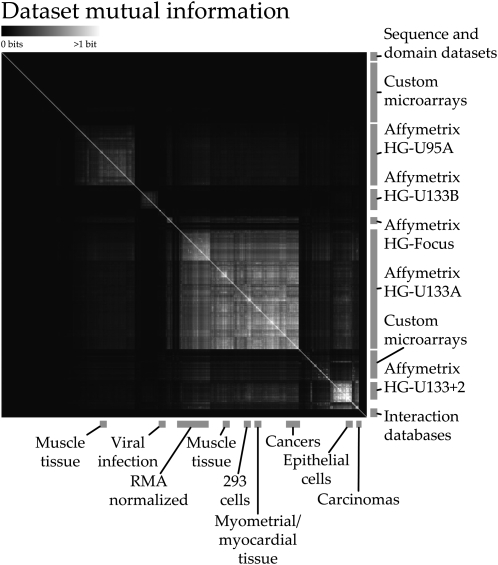
Overview of hierarchically clustered mutual information (MI) between genomic data sets. We used MI among 656 genomic data sets to perform regularization of the parameters of our 230 process-specific Bayesian classifiers. Data sets with a greater proportion of shared information were more heavily mixed with a uniform prior, resulting in the overall up-weighting of particularly unique and informative data. Additionally, a global view of the mutual information scores reveals structure in the data. Primarily platform-based effects can be observed among the expression data sets we obtained from GEO (Barrett et al. 2005), most of which use Affymetrix arrays; tissue type, cell type, and array normalization algorithms can all cause small amounts of information to be shared between many data sets. For example, Robust MultiArray (RMA) normalization causes a noticeable shift in the information shared among HG-U133A arrays. While the amount of MI between any two data sets is generally low (this figure saturates at one bit of shared information), an accumulation of many small overlaps can result in overconfidence during Bayesian data integration, accounting for the success of parameter regularization.
Next steps: Tissue specificity and temporal resolution
A variety of biological features and prior knowledge could be added to further improve functional mapping's integration of genomic data. Most significantly, tissue and cell type are key aspects of metazoan biology that are not currently taken advantage of by our functional maps. This is perhaps evident in our investigation of VAMP7, a vesicle-association membrane protein known to show widely varying behaviors in different tissue types (Advani et al. 1999; Siddiqi et al. 2006). It has characterized roles in the late endosome/lysosome, and our functional maps predict extensive relationships with other synaptosomal proteins, in agreement with VAMP7's function in neuronal morphogenesis (Rossi et al. 2004). While we found that decreasing the expression of VAMP7 in human fibroblasts did not detectably influence their induction of autophagy, it is possible that VAMP7 participates in autophagy in other cell or tissue types.
Similarly, just as many functional associations are cell-type specific, others are dependent on subcellular localization or on temporal characteristics (e.g., cell cycle phase). Our results, as well as previous work (Myers and Troyanskaya 2007), show that explicitly modeling functional relationships within individual biological processes significantly improves accuracy. Differences in cell type, localization, and temporal character represent equally significant cases in which the same proteins can carry out different functions. Incorporating information such as cell and tissue types is thus an important way in which the mapping process can be further developed in the future.
The features, diversity, and amount of genomic data will certainly continue to increase, and functional maps provide a flexible means by which this data can be informatively summarized and explored. By integrating over 650 data sets spanning thousands of experimental conditions, we have predicted functional relationship networks specific to a variety of individual biological processes. Mapping these networks allows an investigator to mine this data from several different perspectives, focusing on associations between genes, pathways, processes, or genetic disorders of interest. We have experimentally confirmed predicted participation of AP3B1, ATP6AP1, BLOC1S1, LAMP2, and RAB11A in the process of macroautophagy, demonstrating that functional mapping can accurately direct experiments to specific genes and functional areas. These predicted associations can be extended to any group of genes, e.g., allowing an experimenter to investigate novel associations among genes linked to genetic disorders. Our results and functional maps have been made available to the community through the interactive HEFalMp tool at http://function.princeton.edu/hefalmp.
Methods
We integrated 656 genome-scale data sets, comprising ~15,000 microarray conditions and ~15,000 interaction and sequence-based results, to predict process-specific functional relationship networks in 229 biological processes. Data integration was performed using naïve Bayesian classifiers, with parameters regularized using a mutual information score between data sets. The resulting functional relationship networks were analyzed to generate functional maps for genes, processes, and diseases within each biological area. Evidence from immunoblotting and fluorescent microscopy was used to confirm novel predictions of the involvement of the AP3B1, ATP6AP1, BLOC1S1, LAMP2, and RAB11A proteins in macroautophagy.
Briefly, functional mapping relies on the construction of process-specific functional relationship networks. These are interaction networks in which each node represents a gene, each edge a functional relationship, and an edge between two genes is probabilistically weighted based on experimental evidence relating to those genes. We integrate evidence from many data sets, with each data set weighted in a process-specific manner. To generate functional maps, these networks are mined for functional associations between groups of genes, which might represent individual genes, pathways, processes, or diseases. A functional association summarizes the overall strength of predicted association between the two groups, and it takes four features into account: relationships between genes spanning the two groups, relationships within the groups, each group's background strength of relationship to the entire genome, and the baseline probability of relationship for all genes. These four features are converted into a P-value by comparing their ratio with a randomized null distribution.
Data preparation
We collected 635 human microarray data sets from the NCBI Gene Expression Omnibus (GEO) repository (Barrett et al. 2005) comprising 14,671 conditions; see Supplemental Table 1 for a complete list. These were processed largely as in Huttenhower and Troyanskaya (2008), with additional manipulation to handle single-channel data and the ambiguity of human probe mapping. Within each data set, negative and very small (less than two) single-channel values were removed, genes with missing values in >30% of the conditions were removed, and the remaining missing values were imputed using KNNImpute (Troyanskaya et al. 2001) with k = 10 (cutoff values recommended by Hibbs et al. [2007] and Troyanskaya et al. [2001], respectively).
Probe IDs were mapped to HGNC symbols using the appropriate GEO platform files. When multiple probes mapped to a single HGNC symbol, a consensus set of probes was generated by finding pairwise Euclidean distances more likely to have been generated from the data set's distribution of intragene probe pairs than from the distribution of intergene probe pairs. If this consensus set contained at least half of the probes mapping to a gene symbol, the consensus set's average value became the expression vector for that gene.
Within each data set, a similarity score for each pair of genes was generated by first calculating the Pearson correlation ρ between the vectors. These correlations were normalized using Fisher's z-transform, shifted by the mean, and divided by the data set standard deviation, yielding a collection of pairwise scores with distribution N(0, 1). Finally, these were binned into one of seven discrete values in the ranges (−∞, −1.5], (−1.5, −0.5], (−0.5, 0.5], (0.5, 1.5], (1.5, 2.5], (2.5, 3.5], (3.5, ∞).
Nonmicroarray pairwise data sets were, for the most part, discretized into two bins: interaction and no interaction/no data. In some cases, negative interactions were explicitly recorded by a third bin. Pairwise data was generated from sequence information (transcription factor binding sites, protein domains, etc.) by calculating either the inner product or the Euclidean distance of the occurrence vectors for each gene pair; see Supplemental Table 1 for details.
Gold standard construction
Biological processes of interest were selected from the Gene Ontology (Ashburner et al. 2000) by polling a panel of six biologists as described in Huttenhower and Troyanskaya (2008). Of the 433 GO terms selected to be experimentally informative, 229 had at least 10 human gene annotations, becoming our processes of interest (see Supplemental Tables 2–4).
An answer set of known functionally related and unrelated proteins was derived by combining these gene sets with information from KEGG (Kanehisa et al. 2008), HPRD (Mishra et al. 2006), Pfam (Finn et al. 2006), Reactome (Vastrik et al. 2007), the Pathway Interaction Database (PID) (Schaefer 2006), and the curated GSEA pathways (Subramanian et al. 2005), all of which represent manually curated databases of functional interactions. A gene pair was considered functionally related if annotated as such in any of these databases and unrelated if annotated to two different terms in GO, KEGG, or PID (the other databases not providing explicit negatives). Genes pairs annotated to terms overlapping with a hypergeometric P-value below 0.05 were excluded from unrelated pair generation (i.e., they were neither related nor unrelated for training and evaluation purposes). This resulted in a gold standard containing 16,184 genes, 8,692,471 functionally related pairs, and 45,712,399 unrelated pairs.
To train and evaluate process-specific classifiers, this answer set was decomposed into subsets related to each biological area of interest. A gene pair was used for training/evaluation in a particular biological process if either (1) both genes were annotated to the process in GO or (2) one of the two genes was annotated to the process and the pair was unrelated in the standard (i.e., not coannotated to another process).
Evaluation was performed using a randomly selected holdout set of 6129 genes (~25% of the genome). Any gene pair including at least one of these genes was withheld from training and used for evaluation of precision/recall and AUPRCs (e.g., Supplemental Fig. 1).
Data integration
One naïve Bayesian classifier was trained per biological area of interest, using the appropriate subset of the gold standard as described above in addition to one global process-unaware classifier trained using the complete gold standard. Each classifier f consisted of a class node predicting the binary presence or absence of a functional relationship (FR) between two genes and n nodes conditioned on FR, each representing the value of a data set Dk.
Parameter regularization was performed as described in Steck and Jaakkola (2002) using mutual information between data sets to estimate a strength of prior belief for each data set. While a large amount of shared information does not guarantee a redundant data set, since the same subset of information could be shared many times, it provides a valuable quantitative estimate of data set uniqueness. For each data set Dk, we calculated a heuristic sum of shared information Uk relative to the data set's entropy:

We then used this value to weight the strength of prior belief in a uniform distribution for the data set, based on the technique in Steck and Jaakkola (2002). This exponentially decreased the weight of a data set as its shared information increased. Let us notate |Dk| as the number of possible observations in data set Dk (discretization levels). For some gene pair (gi, gj), supporting data {d1(gi, gj), d2(gi, gj), …, dn(gi, gj)}, and an effective document count of two, the probability of a FR in function f is thus:

When fewer than 25 gene pairs were available for a particular data set/relationship combination, the global probability distribution was used for that condition. Remaining zero counts were Laplace smoothed.
An additional global process-aware FR network was generated by transforming each set of process-specific probabilities into Z-scores and averaging the results for each gene pair across all processes. Specifically:

We used the C++ implementations of naïve Bayesian learning and inference provided in Huttenhower et al. (2008), relying on the SMILE library and GeNIe modeling environment (Druzdzel 1999) from the University of Pittsburgh Decision Systems Library for Bayesian network manipulation.
Process-specific analysis
The parameters learned by the naïve classifiers in this manner yield a functional activity score (FAS) indicating the strength of the contribution of each data set within each biological process of interest. A data set's FAS is the sum of the change each of its possible values makes in the classifier's posterior times the prior probability of observing that value; this yields high scores for data that are both frequent and accurate. The score for data set D within function f was thus calculated as:

Functional modules
Novel functional modules (FMs) are defined within the global process-aware FR network using an algorithm based on Charikar (2000). We begin with a minimum initial score σ and a minimum final ratio ρ and fill a set of genes Gk and a set of excluded edges E. We repeatedly selected the most related pair of genes not being excluded. To this set, we repeatedly add the gene most related, on average, until this average relationship probability reaches some fraction ρ of the seed pair's original score. If no such gene can be added, the seed pair is marked as excluded; otherwise, each edge weight in the resulting set is reduced by the average connection weight, and the current Gk is output as a functional module.
Each FM is generated with two parameters: the input ratio ρ and a final average edge weight score S(Gk). ρ is akin to a depth within GO. FMs generated at low ρ are larger, more general, and “higher” in the functional hierarchy; FMs generated at high ρ are smaller, more specific, and “lower” in the hierarchy. The score S(Gk) is an estimated confidence in the FM such that a higher value indicates a more self-contained, certain module. In pseudocode, the algorithm is:
Input minimum initial score σ and minimum final ratio ρ
Define:
Let E = {}
Let:
If Zi,j(FR) < σ, stop
Let Gk = {gs1, gs2}
Begin loop
Let:
If S(gt, Gk)/Zs1,s2(FR) < ρ, break
Gk = Gk
{gt}
If |Gk| = 2
E = E
{(gs1, gs2)}
Go to step 4
Output module Gk with parameters ρ, S(Gk)
For all gi,gj
Gk
Zi,j(FR) = max({Zi,j(FR) − S(Gk), 0})
Go to step 4.
To generate novel FMs, we ran this algorithm on the global process-aware human FR network with σ = 0.95 and ρ 0.01, 0.025, 0.05, 0.075, 0.1, 0.2, …, 0.5}, generating a set of preliminary FMs
. To remove redundant FMs, we merged by union any pair with Jaccard index at least 0.5, with the newly formed FM occupying the more specific depth. Specifically, for all pairs of modules Mi and Mj within module sets
and
(ρ depths x and y):
Until no changes occur
For all
For all
If J(Mi, Mj) ≥ 0.5
To form the resulting merged FMs into a DAG similar to the structure of GO, parent/child relationships were established only from higher to lower depths when (1) an indirect descendant relationship did not already exist and (2) the higher FM contained at least two-thirds of the lower FM's genes. This generated parent/child relationships p(Mp, Mc):
For x from 0.5 to 0.01
For y from x to 0.01
For all
If Mj is not a descendant of Mi and |Mi ∩ Mj|/|Mj| ≥ 2/3
p(Mi, Mj) = 1
This process resulted in 17,759 FMs across the nine depth levels, 11,674 parent/child relationships, and 10 connected components in the DAG (nine singletons). A functional evaluation of the FMs is shown in Supplemental Figure 3, and their contents and hierarchical structure are provided in Supplemental Table 5.
Functional mapping associations and P-values
The functional association of two gene sets quantifies the degree of specific overall relationship between their constituent genes. This score is made up of four parts. The score between two gene sets within a process is the average probability of all edges between them. Their background score in a process is the average probability of all edges incident to either set. The baseline score is the average probability of an edge in the process-independent network. The score within a single gene set is the average edge probability assuming nodes are self-connected with baseline strength, and the score within two gene sets is their unweighted average. The between and baseline scores are divided by the background and within scores to calculate two gene sets' functional association, which is thus increased if they are more interconnected and decreased if they are more self-connected.
This score was designed to mitigate several sources of variation and potential false positives in the networks. Known disease genes tend to be well-studied, providing them with more data and increasing their overall probability of functional relationship. Sets of genes representing genetic disorders can thus be very small and highly connected, which is normalized by the within-score and its unweighted average. This and the baseline are calculated in the process-independent network, which also has lower variability than the process-specific networks. Normalizing by the baseline guarantees an expected value of one, and assuming self-connections with baseline weight allows the functional association score to extend seamlessly to arbitrarily small sets.
Thus, within any functional relationship network f, two gene sets G1 and G2 were assigned a functional association score as follows. For f0 the global process-independent network and n genes in the genome, let:





All averages are Winsorized by 10% of their length to mitigate outliers; Winsorization is a standard robust averaging process in which the n largest and smallest values are replaced by copies of the n-first largest and n-first smallest value, respectively. This defines the functional association between two gene sets as:

This score was converted into a P-value by interpolating over a bootstrapped null distribution. For each combination of sizes 1, 2, 5, 10, 15, 20, 25, 50, 100, and 500, pairs of sets were generated randomly 62,500 times within each process, and the resulting functional association score calculated. The distributions of these scores were approximately normal, and the standard deviations were asymptotic in the sizes of the two gene sets (Supplemental Table 7). Fitting these empirical curves with a ratio of linear polynomials allowed real-time computation of an approximate standard deviation for any pair of gene set sizes, which then allowed the conversion of functional association scores into P-values using a normal distribution function.
Web-based interface
HEFalMp was implemented in two parts, combining a web-based front end using Ruby on Rails (37 signals) with a C++ back-end for rapid data processing using the Sleipnir library (Huttenhower et al. 2008). For details, see http://function.princeton.edu/hefalmp.
Experimental validation
Human dermal fibroblasts were cultured in subconfluent conditions in fibroblast basal medium supplemented with FBS, insulin, and fibroblast growth factor (Lonza Group Ltd.). Cells received fresh medium every 2 d.
For siRNA transfection, 1.2 × 105 fibroblasts were transiently transfected with 100 nM duplex siRNA designed by the Rosetta algorithm (Sigma) against control targets (ATG5 or luciferase) or experimental targets (AP3B1, ATP6AP1, BLOC1S1, RAB11A, LAMP2, VAMP7) using Oligofectamine transfection reagent (Invitrogen). On the day of experimentation, cells were either supplied with fresh medium (not starved), or starved for amino acids for 4 h in Kreb's Ringer Bicarbonate (KRB) solution (Sigma) at 37°C.
Western blots were performed using cell lysates collected on ice by scraping each plate into RIPA buffer (50 mM Tris-Cl at pH 7.4, 150 mM NaCl, 1% Triton X-100, 1% sodium deoxycholate, and 0.1% SDS) supplemented with a protease inhibitor cocktail tablet consisting of chymotrypsin (1.5 μg/mL), thermolysin (0.8 μg/mL), papain (1 mg/mL), pronase (1.5 μg/mL), pancreatic extract (1.5 μg/mL), and trypsin (0.002 μg/mL) (Roche Diagnostics) at either 48 h (default) or 72 h (LAMP2 and VAMP7) post-transfection. Freeze-thawing of lysates was avoided whenever possible, and freshly denatured samples were run on appropriate percentage SDS–polyacrylamide gels and transferred onto PVDF membranes (Perkin Elmer) using BioRad electrophoresis equipment (BioRad). Antibodies for Western blot analysis were used at the following concentrations in PBS plus BSA: rabbit anti-LC3 at 2 μg/mL (Novus Biologicals), rabbit anti-RAB11A at 1 mg/mL (Sigma), rabbit anti-LAMP2 at 1 mg/mL (Sigma), rabbit anti-VAMP7 at 1 μg/mL (Abcam Inc.).
A GFP-LC3 fusion was used as a fluorescent marker for autopaghy. We generated fibroblasts stably expressing a GFP-LC3 fusion protein by infecting subconfluent fibroblasts with a retroviral construct encoding GFP and the rat LC3 sequence (C. Thompson, University of Pennsylvania). GFP-LC3 fibroblasts transfected with siRNA against control or experimental targets were cultured in uncoated glass bottom culture dishes (MatTek Corp.) and visualized either 48 h (default) or 72 h (LAMP2 and VAMP7) post-transfection. Transfected GFP-LC3 fibroblasts were imaged using a Zeiss LSM510 confocal microscope.
Acknowledgments
We thank Florian Markowetz, Edo Airoldi, Chad Myers, Aster Legesse-Miller, Josh Forman, Eric Suh, Sarah Pfau, Leonid Kruglyak, and Dannie Durand for insightful conversations, and the laboratory of Craig Thompson for their generous gift of the GFP-LC3 construct. This research was partially supported by NIH grant R01 GM071966, NSF CAREER award DBI-0546275, NSF grant IIS-0513552, PhRMA Foundation grant 2007RSGl9572, a New Jersey Commission on Cancer Research fellowship, NIH grant T32 HG003284, and NIGMS Center of Excellence grant P50 GM071508. H.A.C. is the Milton E. Cassel scholar of the Rita Allen Foundation. O.G.T. is an Alfred P. Sloan Research Fellow.
Footnotes
[Supplemental material is available online at www.genome.org; results from this study and the interactive HEFalMp tool are available at http://function.princeton.edu/hefalmp.]
Article published online before print. Article and publication date are at http://www.genome.org/cgi/doi/10.1101/gr.082214.108.
References
- Advani R.J., Yang B., Prekeris R., Lee K.C., Klumperman J., Scheller R.H. VAMP-7 mediates vesicular transport from endosomes to lysosomes. J. Cell Biol. 1999;146:765–776. [Europe PMC free article] [Abstract] [Google Scholar]
- Ashburner M., Ball C.A., Blake J.A., Botstein D., Butler H., Cherry J.M., Davis A.P., Dolinski K., Dwight S.S., Eppig J.T., et al. Gene ontology: Tool for the unification of biology. The Gene Ontology Consortium. Nat. Genet. 2000;25:25–29. [Europe PMC free article] [Abstract] [Google Scholar]
- Barrett T., Suzek T.O., Troup D.B., Wilhite S.E., Ngau W.C., Ledoux P., Rudnev D., Lash A.E., Fujibuchi W., Edgar R. NCBI GEO: Mining millions of expression profiles–database and tools. Nucleic Acids Res. 2005;33:D562–D566. [Europe PMC free article] [Abstract] [Google Scholar]
- Carpenter A.E., Jones T.R., Lamprecht M.R., Clarke C., Kang I.H., Friman O., Guertin D.A., Chang J.H., Lindquist R.A., Moffat J., et al. CellProfiler: Image analysis software for identifying and quantifying cell phenotypes. Genome Biol. 2006;7:R100. 10.1186/gb-2006-7-10-r100. [Europe PMC free article] [Abstract] [CrossRef] [Google Scholar]
- Chapuy B., Tikkanen R., Muhlhausen C., Wenzel D., von Figura K., Honing S. AP-1 and AP-3 mediate sorting of melanosomal and lysosomal membrane proteins into distinct post-Golgi trafficking pathways. Traffic. 2008;9:1157–1172. [Abstract] [Google Scholar]
- Charikar M. Lecture notes in computer science. Proceedings of the third international workshop on approximation algorithms for combinatorial optimization. Vol. 1913. Springer-Verlag; London, UK: 2000. Greedy approximation algorithms for finding dense components in a graph; pp. 84–95. [Google Scholar]
- Chen J.W., Pan W., D'Souza M.P., August J.T. Lysosome-associated membrane proteins: Characterization of LAMP-1 of macrophage P388 and mouse embryo 3T3 cultured cells. Arch. Biochem. Biophys. 1985;239:574–586. [Abstract] [Google Scholar]
- Cuervo A.M., Dice J.F. A receptor for the selective uptake and degradation of proteins by lysosomes. Science. 1996;273:501–503. [Abstract] [Google Scholar]
- Date S.V., Stoeckert C.J., Jr Computational modeling of the Plasmodium falciparum interactome reveals protein function on a genome-wide scale. Genome Res. 2006;16:542–549. [Europe PMC free article] [Abstract] [Google Scholar]
- Detweiler C.S., Cunanan D.B., Falkow S. Host microarray analysis reveals a role for the Salmonella response regulator phoP in human macrophage cell death. Proc. Natl. Acad. Sci. 2001;98:5850–5855. [Europe PMC free article] [Abstract] [Google Scholar]
- Druzdzel M.J. SMILE: Structural modeling, inference, and learning engine and GeNIe: A development environment for graphical decision-theoretic models. Sixteenth national conference on artificial intelligence; Menlo Park, CA: AAAI Press/The MIT Press; 1999. pp. 902–903. [Google Scholar]
- Dumeaux V., Fournier A., Lund E., Clavel-Chapelon F. Previous oral contraceptive use and breast cancer risk according to hormone replacement therapy use among postmenopausal women. Cancer Causes Control. 2005;16:537–544. [Europe PMC free article] [Abstract] [Google Scholar]
- Finn R.D., Mistry J., Schuster-Bockler B., Griffiths-Jones S., Hollich V., Lassmann T., Moxon S., Marshall M., Khanna A., Durbin R., et al. Pfam: Clans, web tools and services. Nucleic Acids Res. 2006;34:D247–D251. [Europe PMC free article] [Abstract] [Google Scholar]
- Graf L., Iwata M., Torok-Storb B. Gene expression profiling of the functionally distinct human bone marrow stromal cell lines HS-5 and HS-27a. Blood. 2002;100:1509–1511. [Abstract] [Google Scholar]
- Hamelers I.H., Steenbergh P.H. Interactions between estrogen and insulin-like growth factor signaling pathways in human breast tumor cells. Endocr. Relat. Cancer. 2003;10:331–345. [Abstract] [Google Scholar]
- Hibbs M.A., Hess D.C., Myers C.L., Huttenhower C., Li K., Troyanskaya O.G. Exploring the functional landscape of gene expression: Directed search of large microarray compendia. Bioinformatics. 2007;23:2692–2699. [Abstract] [Google Scholar]
- Huttenhower C., Troyanskaya O.G. Assessing the functional structure of genomic data. Bioinformatics. 2008;24:i330–i338. [Europe PMC free article] [Abstract] [Google Scholar]
- Huttenhower C., Hibbs M., Myers C., Troyanskaya O.G. A scalable method for integration and functional analysis of multiple microarray data sets. Bioinformatics. 2006;22:2890–2897. [Abstract] [Google Scholar]
- Huttenhower C., Schroeder M., Chikina M.D., Troyanskaya O.G. The Sleipnir library for computational functional genomics. Bioinformatics. 2008;24:1559–1561. [Europe PMC free article] [Abstract] [Google Scholar]
- Kabeya Y., Mizushima N., Ueno T., Yamamoto A., Kirisako T., Noda T., Kominami E., Ohsumi Y., Yoshimori T. LC3, a mammalian homologue of yeast Apg8p, is localized in autophagosome membranes after processing. EMBO J. 2000;19:5720–5728. [Europe PMC free article] [Abstract] [Google Scholar]
- Kabeya Y., Mizushima N., Yamamoto A., Oshitani-Okamoto S., Ohsumi Y., Yoshimori T. LC3, GABARAP and GATE16 localize to autophagosomal membrane depending on form-II formation. J. Cell Sci. 2004;117:2805–2812. [Abstract] [Google Scholar]
- Kanehisa M., Araki M., Goto S., Hattori M., Hirakawa M., Itoh M., Katayama T., Kawashima S., Okuda S., Tokimatsu T., et al. KEGG for linking genomes to life and the environment. Nucleic Acids Res. 2008;36:D480–D484. [Europe PMC free article] [Abstract] [Google Scholar]
- Klionsky D.J. Autophagy: From phenomenology to molecular understanding in less than a decade. Nat. Rev. Mol. Cell Biol. 2007;8:931–937. [Abstract] [Google Scholar]
- Lee I., Date S.V., Adai A.T., Marcotte E.M. A probabilistic functional network of yeast genes. Science. 2004;306:1555–1558. [Abstract] [Google Scholar]
- Mehrabian M., Allayee H., Stockton J., Lum P.Y., Drake T.A., Castellani L.W., Suh M., Armour C., Edwards S., Lamb J., et al. Integrating genotypic and expression data in a segregating mouse population to identify 5-lipoxygenase as a susceptibility gene for obesity and bone traits. Nat. Genet. 2005;37:1224–1233. [Abstract] [Google Scholar]
- Mishra G.R., Suresh M., Kumaran K., Kannabiran N., Suresh S., Bala P., Shivakumar K., Anuradha N., Reddy R., Raghavan T.M., et al. Human protein reference database–2006 update. Nucleic Acids Res. 2006;34:D411–D414. [Europe PMC free article] [Abstract] [Google Scholar]
- Mizushima N., Yamamoto A., Matsui M., Yoshimori T., Ohsumi Y. In vivo analysis of autophagy in response to nutrient starvation using transgenic mice expressing a fluorescent autophagosome marker. Mol. Biol. Cell. 2004;15:1101–1111. [Europe PMC free article] [Abstract] [Google Scholar]
- Murali T.M., Wu C.J., Kasif S. The art of gene function prediction. Nat. Biotechnol. 2006;24:1474–1475. author reply 1475–1476. [Abstract] [Google Scholar]
- Myers C.L., Troyanskaya O.G. Context-sensitive data integration and prediction of biological networks. Bioinformatics. 2007;23:2322–2330. [Abstract] [Google Scholar]
- Online Mendelian Inheritance in Man, OMIM. 2008. McKusick-Nathans Institute of Genetic Medicine, Johns Hopkins University, Baltimore, MD, and National Center for Biotechnology Information, National Library of Medicine, Bethesda, MD. http://www.ncbi.nlm.nih.gov/omim/.
- Peters-Golden M., Brock T.G. 5-lipoxygenase and FLAP. Prostaglandins Leukot. Essent. Fatty Acids. 2003;69:99–109. [Abstract] [Google Scholar]
- Prekeris R., Klumperman J., Scheller R.H. A Rab11/Rip11 protein complex regulates apical membrane trafficking via recycling endosomes. Mol. Cell. 2000;6:1437–1448. [Abstract] [Google Scholar]
- Rhodes D.R., Tomlins S.A., Varambally S., Mahavisno V., Barrette T., Kalyana-Sundaram S., Ghosh D., Pandey A., Chinnaiyan A.M. Probabilistic model of the human protein–protein interaction network. Nat. Biotechnol. 2005;23:951–959. [Abstract] [Google Scholar]
- Rieger K.E., Chu G. Portrait of transcriptional responses to ultraviolet and ionizing radiation in human cells. Nucleic Acids Res. 2004;32:4786–4803. [Europe PMC free article] [Abstract] [Google Scholar]
- Rossi V., Banfield D.K., Vacca M., Dietrich L.E., Ungermann C., D'Esposito M., Galli T., Filippini F. Longins and their longin domains: Regulated SNAREs and multifunctional SNARE regulators. Trends Biochem. Sci. 2004;29:682–688. [Abstract] [Google Scholar]
- Sachs K., Perez O., Pe'er D., Lauffenburger D.A., Nolan G.P. Causal protein-signaling networks derived from multiparameter single-cell data. Science. 2005;308:523–529. [Abstract] [Google Scholar]
- Schaefer C. An Introduction to the NCI Pathway Interaction Database. NCI-Nature Pathway Interaction Database. 2006 10.1038/PID.2006.001. [CrossRef] [Google Scholar]
- Siddiqi S.A., Mahan J., Siddiqi S., Gorelick F.S., Mansbach C.M., 2nd Vesicle-associated membrane protein 7 is expressed in intestinal ER. J. Cell Sci. 2006;119:943–950. [Europe PMC free article] [Abstract] [Google Scholar]
- Starcevic M., Dell'Angelica E.C. Identification of snapin and three novel proteins (BLOS1, BLOS2, and BLOS3/reduced pigmentation) as subunits of biogenesis of lysosome-related organelles complex-1 (BLOC-1) J. Biol. Chem. 2004;279:28393–28401. [Abstract] [Google Scholar]
- Steck H., Jaakkola T.S. On the Dirichlet prior and Bayesian regularization. Massachusetts Institute of Technology; Cambridge, MA: 2002. [Google Scholar]
- Subramanian A., Tamayo P., Mootha V.K., Mukherjee S., Ebert B.L., Gillette M.A., Paulovich A., Pomeroy S.L., Golub T.R., Lander E.S., et al. Gene set enrichment analysis: A knowledge-based approach for interpreting genome-wide expression profiles. Proc. Natl. Acad. Sci. 2005;102:15545–15550. [Europe PMC free article] [Abstract] [Google Scholar]
- Tian J., Ishibashi K., Handa J.T. The expression of native and cultured RPE grown on different matrices. Physiol. Genomics. 2004;17:170–182. [Abstract] [Google Scholar]
- Tian F., Wang Z., Yu J., Huang H. Advances in intelligent computing. Springer; Berlin, Germany: 2005. Learning TAN from incomplete data; pp. 495–504. [Google Scholar]
- Troyanskaya O., Cantor M., Sherlock G., Brown P., Hastie T., Tibshirani R., Botstein D., Altman R.B. Missing value estimation methods for DNA microarrays. Bioinformatics. 2001;17:520–525. [Abstract] [Google Scholar]
- Vastrik I., D'Eustachio P., Schmidt E., Joshi-Tope G., Gopinath G., Croft D., de Bono B., Gillespie M., Jassal B., Lewis S., et al. Reactome: A knowledge base of biologic pathways and processes. Genome Biol. 2007;8:R39. 10.1186/gb-2007-8-3-r39. [Europe PMC free article] [Abstract] [CrossRef] [Google Scholar]
- von Mering C., Jensen L.J., Kuhn M., Chaffron S., Doerks T., Kruger B., Snel B., Bork P. STRING 7–recent developments in the integration and prediction of protein interactions. Nucleic Acids Res. 2007;35:D358–D362. [Europe PMC free article] [Abstract] [Google Scholar]
- Ward D.M., Pevsner J., Scullion M.A., Vaughn M., Kaplan J. Syntaxin 7 and VAMP-7 are soluble N-ethylmaleimide-sensitive factor attachment protein receptors required for late endosome-lysosome and homotypic lysosome fusion in alveolar macrophages. Mol. Biol. Cell. 2000;11:2327–2333. [Europe PMC free article] [Abstract] [Google Scholar]
- Yorimitsu T., Klionsky D.J. Autophagy: Molecular machinery for self-eating. Cell Death Differ. 2005;12(Suppl. 2):1542–1552. [Europe PMC free article] [Abstract] [Google Scholar]
Articles from Genome Research are provided here courtesy of Cold Spring Harbor Laboratory Press
Full text links
Read article at publisher's site: https://doi.org/10.1101/gr.082214.108
Read article for free, from open access legal sources, via Unpaywall:
http://genome.cshlp.org/content/19/6/1093.full.pdf
Free after 6 months at www.genome.org
http://www.genome.org/cgi/content/full/19/6/1093
Free after 6 months at www.genome.org
http://www.genome.org/cgi/reprint/19/6/1093
Free to read at www.genome.org
http://www.genome.org/cgi/content/abstract/19/6/1093
Citations & impact
Impact metrics
Citations of article over time
Alternative metrics
Smart citations by scite.ai
Explore citation contexts and check if this article has been
supported or disputed.
https://scite.ai/reports/10.1101/gr.082214.108
Article citations
Noncanonical usage of stop codons in ciliates expands proteins with structurally flexible Q-rich motifs.
Elife, 12:RP91405, 23 Feb 2024
Cited by: 0 articles | PMID: 38393970 | PMCID: PMC10942620
Decoding disease: from genomes to networks to phenotypes.
Nat Rev Genet, 22(12):774-790, 02 Aug 2021
Cited by: 29 articles | PMID: 34341555
Review
Comparing Host Module Activation Patterns and Temporal Dynamics in Infection by Influenza H1N1 Viruses.
Front Immunol, 12:691758, 14 Jul 2021
Cited by: 0 articles | PMID: 34335598 | PMCID: PMC8317020
GEOlimma: differential expression analysis and feature selection using pre-existing microarray data.
BMC Bioinformatics, 22(1):44, 03 Feb 2021
Cited by: 5 articles | PMID: 33535967 | PMCID: PMC7860207
Responsible, practical genomic data sharing that accelerates research.
Nat Rev Genet, 21(10):615-629, 21 Jul 2020
Cited by: 54 articles | PMID: 32694666 | PMCID: PMC7974070
Review Free full text in Europe PMC
Go to all (132) article citations
Data
Similar Articles
To arrive at the top five similar articles we use a word-weighted algorithm to compare words from the Title and Abstract of each citation.
Disruption of AP3B1 by a chromosome 5 inversion: a new disease mechanism in Hermansky-Pudlak syndrome type 2.
BMC Med Genet, 14:42, 04 Apr 2013
Cited by: 18 articles | PMID: 23557002 | PMCID: PMC3663694
Characterization of in vitro genotoxic, cytotoxic and transcriptomic responses following exposures to amorphous silica of different sizes.
Mutat Res Genet Toxicol Environ Mutagen, 796:8-22, 25 Nov 2015
Cited by: 22 articles | PMID: 26778505
Lipofuscin is formed independently of macroautophagy and lysosomal activity in stress-induced prematurely senescent human fibroblasts.
Free Radic Biol Med, 53(9):1760-1769, 01 Sep 2012
Cited by: 44 articles | PMID: 22982048
Engineering Aspects of Olfaction
CRC Press/Taylor & Francis, Boca Raton (FL), 05 Jun 2015
Cited by: 0 articles | PMID: 26042329
ReviewBooks & documents Free full text in Europe PMC
Funding
Funders who supported this work.
NHGRI NIH HHS (1)
Grant ID: T32 HG003284
NIGMS NIH HHS (2)
Grant ID: P50 GM071508
Grant ID: R01 GM071966