Abstract
Free full text

TaxMan: a server to trim rRNA reference databases and inspect taxonomic coverage
Abstract
Amplicon sequencing of the hypervariable regions of the small subunit ribosomal RNA gene is a widely accepted method for identifying the members of complex bacterial communities. Several rRNA gene sequence reference databases can be used to assign taxonomic names to the sequencing reads using BLAST, USEARCH, GAST or the RDP classifier. Next-generation sequencing methods produce ample reads, but they are short, currently ~100–450nt (depending on the technology), as compared to the full rRNA gene of ~1550
nt. It is important, therefore, to select the right rRNA gene region for sequencing. The primers should amplify the species of interest and the hypervariable regions should differentiate their taxonomy. Here, we introduce TaxMan: a web-based tool that trims reference sequences based on user-selected primer pairs and returns an assessment of the primer specificity by taxa. It allows interactive plotting of taxa, both amplified and missed in silico by the primers used. Additionally, using the trimmed sequences improves the speed of sequence matching algorithms. The smaller database greatly improves run times (up to 98%) and memory usage, not only of similarity searching (BLAST), but also of chimera checking (UCHIME) and of clustering the reads (UCLUST). TaxMan is available at http://www.ibi.vu.nl/programs/taxmanwww/.
INTRODUCTION
The bacterial small subunit of the ribosomal gene, the 16S rRNA gene, is the most common housekeeping genetic marker used in bacterial phylogeny and taxonomy. The reasons for this are its presence in almost all bacteria, relative stability over time and its size that is large enough for informatics purposes (1). Cloning of the (nearly complete) 16S rRNA gene in Escherichia coli and sequencing, although highly elaborate and costly, became a standard method in determining microbial community composition (2,3). With the advent of high throughput next-generation sequencing (NGS) technology, the cloning bias could be circumvented and the costs per nucleotide substantially reduced. Now, the standard method of assessing the taxonomic composition of microbial communities is to sequence the 16S rRNA gene, using PCR amplification and NGS technology. The bacterial 16S rRNA gene consists of conserved sequences interspersed with variable sequences that include nine hypervariable regions (4). These regions are flanked by conserved parts of the 16S rRNA gene, which are used in primer designs to target as diverse a bacterial community as possible. The sequences of the hypervariable regions themselves are used to discriminate among bacterial taxa.
Different hypervariable regions evolve at different rates and different species of the same genus (or e.g. genera of the same family) may be similar in some hypervariable regions and more divergent in others (5,6). Primer bias occurs when the selected primers do not anneal to the DNA from all members of the community equally, but preferentially amplify certain taxonomic groups. For instance, Verrucomicrobia, a bacterial phylum previously thought to occur in soil at a low abundance, was shown to be highly abundant in different soil samples by simply replacing commonly used primer set 27F/338R (V1–V2), obviously biased against Verrucomicrobia, by the primer set 515F/806R targeting hypervariable region V4 (7). Assessing the nature and extent of primer bias is an important first step whenever primers are selected. In silico testing for the most effective regions for discerning taxa from a particular environment or for finer resolution of particular taxa would have a large impact on experimental costs and outcomes. This has recently been demonstrated within the Human Microbiome Project (8), where both the V1–V3 and the V3–V5 sections of the rRNA gene were sequenced, trimmed and clustered into 3% operational taxonomic units (OTUs) (9). The V1–V3 data showed three dominant Lactobacillus OTUs, which appear to differentiate L. crispatus, L. iners and L. gasseri (10). These OTUs correspond to the three primary vaginal biome types identified by Zhou et al. (11) and Ravel et al. (12). The V3–V5 sequence data, however, was dominated by only one OTU, which included over six different Lactobacillus species. Conversely, the V3–V5 sequence data identified a Bifidobacteriaceae OTU that was not detected as such with the V1–V3 sequences.
The data resulting from PCR amplification and NGS sequencing requires processing through a bioinformatics pipeline. This pipeline should assure that low quality sequences are discarded and meaningful groups or clusters of sequences, OTUs, are created. The representative sequence of each OTU is then compared with sequences found in publicly available 16S rRNA gene databases and, when possible, a consensus taxonomic lineage (genus, family or higher taxon) is given to the OTU. For these downstream analyses of the sequences, only the amplified part of the 16S rRNA gene is required. The use of the short amplicon sequences instead of the full-length rRNA gene as reference sets in computational pipelines, reduces the run times considerably. Some programs such as GAST (13), used to assign taxonomy based on the best match in a Global Alignment for Sequence Taxonomy, require a trimmed database that matches the length of the amplicons. An additional advantage of using a trimmed database is that it can serve as a quality check for accurate trimming of (the sequenced) amplicons.
Programs already exist that test which sequences match a given oligonucleotide probe. For the different rRNA gene databases, these are SILVA’s TestProbe (14), Greengenes’ Probes (15) or RDP’s Probe Match (16). Probes can be designed using stand-alone software, such as Primrose (17) and PrimerProspector (18). The latter provides a probe/primer design pipeline that supports de novo barcoded primer design and includes command-line scripts to analyze taxonomic coverage. Most programs, however, do not return trimmed reference sequences matching the probes.
We have developed TaxMan, a straightforward web-tool, to trim the reference sequences of several rRNA gene databases to the hypervariable regions used, based on pre-selected primers, and to interactively analyze taxonomic coverage. We show that the use of the provided trimmed sequences in computations increases analysis speed. Additionally, by assessing the ability of amplification products to differentiate specific taxa from a particular environment, thus by analyzing the taxonomic coverage using several rRNA gene databases, before performing the sequencing, researchers will be able to better target their experiments to resolve the taxa of greatest interest to their research question. To this end, TaxMan also provides graphical analysis of the taxa that are selected for or against with the selected primer set(s).
MATERIALS AND METHODS
Database construction
Several rRNA gene databases are provided, including two oral microbiome-specific databases: CORE (16S rDNA database of the core human oral microbiome) (19) and HOMD (Human Oral Microbiome Database) (20), the vaginal 16S reference package (21), as well as more inclusive databases such as Greengenes (15) and the SILVA comprehensive ribosomal RNA databases (small subunit, small subunit with human skin and mouse wound microbiome, and large subunit) (14). Other databases can be added upon user’s request.
TaxMan uses the sequences in FASTA format and includes the taxonomic lineage as FASTA description. The different taxonomic categories are separated by a semi-colon. For example, ‘Bacteria;Bacteroidetes;Bacteroidia;Bacteroidales;Porphyromonadaceae;Porphyromonas’.
The taxonomy is taken from the source databases and is not changed. For all databases, missing categories in the taxonomic lineage are represented by an ‘empty string’. This can occur if, for example, no order or family, but a genus was supplied by the respective taxonomy. The ‘empty string’ is replaced with ‘noname’ in the tree. If the database has classified a sequence as unclassified explicitly, this will remain as such.
All databases are made non-redundant. The databases, apart from SILVA, have been preprocessed to include the lineage in the FASTA records.
CORE
The Excel file was downloaded [http://microbiome.osu.edu/ (19)] and the taxonomic categories were concatenated. The CORE accession id and the lineage form the FASTA header line.
HOMD
The 16S rRNA RefSeq and taxon table [http://www.homd.org/ (20)] data were combined based on the HOT identifier. The constructed FASTA headers start with the HOT id merged with the strain synonym followed by the lineage.
Greengenes
The Greengenes PROKMSA_id and GenBank accession in the Greengenes FASTA file [current_GREENGENES_gg16S_unaligned.fasta; http://greengenes.lbl.gov/ (15)] were merged with an underscore and the lineage (Greengenes/Hugenholtz) format was changed.
SILVA
Files were downloaded from http://www.arb-silva.de/ (14).
Vaginal 16S reference
The sequences were taken from the alignment file and gaps were removed (vaginal_aln.fasta; http://microbiome.fhcrc.org/apps/refpkg/). The lineages, based on the taxtable.txt file, contain the following levels: species, genus, family, order, class, phylum and superkingdom. The word ‘unclassified’ was appended to the lineage at the level from which all sub-classifications are absent.
Web server
Input
The web site takes forward and reverse PCR primer sequence(s) as input. Primers may contain ambiguity codes. The reverse primer needs to be in the reverse complement orientation, as is common for PCR primers. The user can further select a target rRNA reference database. Options include setting a mismatch percentage for the primers, removing forward and/or reverse primer(s) from the amplicons and two options related to treatment of (redundant) lineages (cf. online documentation).
Processing
The FASTA sequences of the rRNA gene databases have been preprocessed to contain the taxonomic lineage in the FASTA header. In silico PCR is performed with an adapted version of primersearch from EMBOSS (v6.4.0) (22) to find the positions of the primers in the sequences and with Perl code to extract the corresponding sub-sequences. The adaptation of primersearch changes the expansion of the IUPAC ambiguity codes. For example, R now expands to GAR instead of GA. In cases where more amplicons are produced for a single reference sequence, the longest amplicon is kept. Then, the set of produced amplicon sequences is made non-redundant. Next, a taxonomic tree is built of the amplicons and combined with the tree of the original reference sequences. In cases where different species have identical amplicons, the taxonomic lineages are optionally summarized to the first non-common level, similar to microarray probes, for example, Bacteria;Bacteroidetes;(Sphingobacteria/Flavobacteria). This tree data is used for the HTML Tree viewer, pie-chart plotting (using jqPlot, an open source project by Chris Leonello; http://www.jqplot.com/) and for the FASTA headers in the downloadable file.
RESULTS AND DISCUSSION
Overview
For NGS amplicon sequencing of bacterial communities, hypervariable regions of the rRNA genes are amplified with PCR. The TaxMan server provides in silico PCR against several rRNA reference databases and interactive analysis of the resulting taxonomic coverage. If more than one forward and reverse primer is provided, a multiplex PCR is performed: all forward primers are combined with all reverse primers. The ambiguity codes, possibly present in a primer, are expanded to include subsets of ambiguity codes, since the rRNA reference sequences can themselves contain ambiguity codes.
The selection of a (few) hypervariable region(s) of the rRNA gene, resulting in shorter sequences, has two implications:
the reference database can be trimmed to correspond with the used rRNA gene region. This can increase the analysis speed considerably both by reducing the length of the sequences to search against and because shorter sequences can be more redundant, the number of non-redundant sequences to search against is also reduced and
the ability to differentiate taxa is reduced, because the targeted hypervariable region(s) can have identical sequences for different species.
Improvements in speed and memory usage
The difference in run time between using the trimmed versus the original reference data set was assessed for several programs. We measured the run times of BLAST (23) to find the taxonomy of the reads, of UCLUST (24) to cluster the reads (using default and reference optimal) and of UCHIME (25) to chimera check the reads. The test data consisted of reads from pyrosequenced amplicons from oral samples (V5–V7 region, Kraneveld,E.A. et al., submitted for publication). This data was either only denoised (722943 reads) for UCHIME chimera checking or denoised and chimera-checked (644
797 reads) for BLAST and UCLUST clustering. For BLAST, the denoised and chimera-checked set was also made non-redundant, leaving 2806 reads.
Table 1 shows the computer run times, memory usage and improvements therein when the different programs were run with the original 16S rRNA gene reference data as compared to the trimmed 16S rRNA gene sequence data (primers removed). As can be seen from Table 1, the use of these trimmed sequences that correspond with the amplicons can result in considerable improvements in both run time and memory usage of 25% up to 98%.
Table 1.
Data on CPU time, run time (hr:mm:ss format), physical memory (mem) and virtual memory (vmem) usage (in kb) as reported by the cluster software (PBS). BLAST was run on eight cores, the other programs on one core. Percentage improvement is calculated as the relative difference (original-trimmed)/original
Program | Measure | Original set | Trimmed set | % Improvement | Fold improvement |
---|---|---|---|---|---|
BLAST | CPU time | 9:17:27 | 4:05:52 | 56 | 2.3 |
run time | 1:12:48 | 0:41:26 | 43 | 1.8 | |
mem | 396![]() | 189![]() | 52 | 2.1 | |
vmem | 1![]() ![]() | 974![]() | 25 | 1.3 | |
UCLUST refa | CPU time | 0:05:17 | 0:00:47 | 85 | 6.7 |
run time | 0:05:25 | 0:00:56 | 83 | 5.8 | |
mem | 9![]() ![]() | 699![]() | 93 | 14 | |
vmem | 12![]() ![]() | 869![]() | 93 | 14 | |
UCLUST ref optb | CPU time | 73:46:16 | 1:14:17 | 98 | 60 |
run time | 73:54:41 | 1:14:35 | 98 | 59 | |
mem | 9![]() ![]() | 1![]() ![]() | 85 | 6.8 | |
vmem | 12![]() ![]() | 1![]() ![]() | 86 | 7.0 | |
UCHIMEc | CPU time | 29:57:17 | 3:13:00 | 89 | 9.3 |
run time | 30:00:50 | 3:13:26 | 89 | 9.3 | |
mem | 1![]() ![]() | 164![]() | 84 | 6.1 | |
vmem | 1![]() ![]() | 267![]() | 76 | 4.2 |
aUCLUST reference mode.
bUCLUST reference optimal mode.
cThe concordance is 93.5%. The fold improvement is the ratio (original/trimmed)
Server output files, taxonomic coverage and visualization
In addition to producing trimmed versions of a reference database, TaxMan can be used to analyze the taxonomic coverage of the trimmed sequences (amplicons), and the original reference database sequences. We illustrate the use of TaxMan with a primer set used in our previous studies on the oral microbiota of children and oral health (26). The primers target the V5–V6 hypervariable region of the 16S rRNA gene. This example is present on the server.
The output provides an overview of the run: the number of non-redundant sequences in the selected database and number of total and non-redundant sequences that the primers formed. In addition, the percentage of sequences (based on the number of total or non-redundant amplicons) in the entire reference database targeted by the primers is stated. Not all database sequences are full-length rRNA gene sequences. Therefore, especially when primers target the ends of the rRNA gene sequence, the coverage may appear to be lower than expected. Last, links are shown to three different sections of the output page: the download, tree and pie chart sections.
Under ‘Download amplicon and lineage data’, three files can be downloaded: the taxonomic lineage coverage and two FASTA files with the amplicon sequences. The lineage file contains counts for all taxa in the amplicon set and in the reference database. The FASTA files contain the same sequence data, but with different headers for redundant sequences: either the taxonomic lineage is summarized (to the identical part or to the first non-common level) or all original FASTA headers are concatenated.
The tree and especially the pie chart sections provide interactive analysis and visualization. The tree is expandable and searchable (Figure 1). The pie charts provide a different view on the taxonomic coverage to facilitate the analysis of taxonomic distributions (Figure 2). By clicking on a slice of the Root pie, a pie for the next taxonomic level is plotted. For this plot, a percentage threshold can be applied. This threshold filters the data that is plotted at the percentage that the respective taxa occur in their taxonomic parent level. For example, a threshold of 14% for Bacteria will only show those bacterial phyla that occur at least 14% (relative to their counts in the reference database), which would be the phylum Firmicutes in this example.
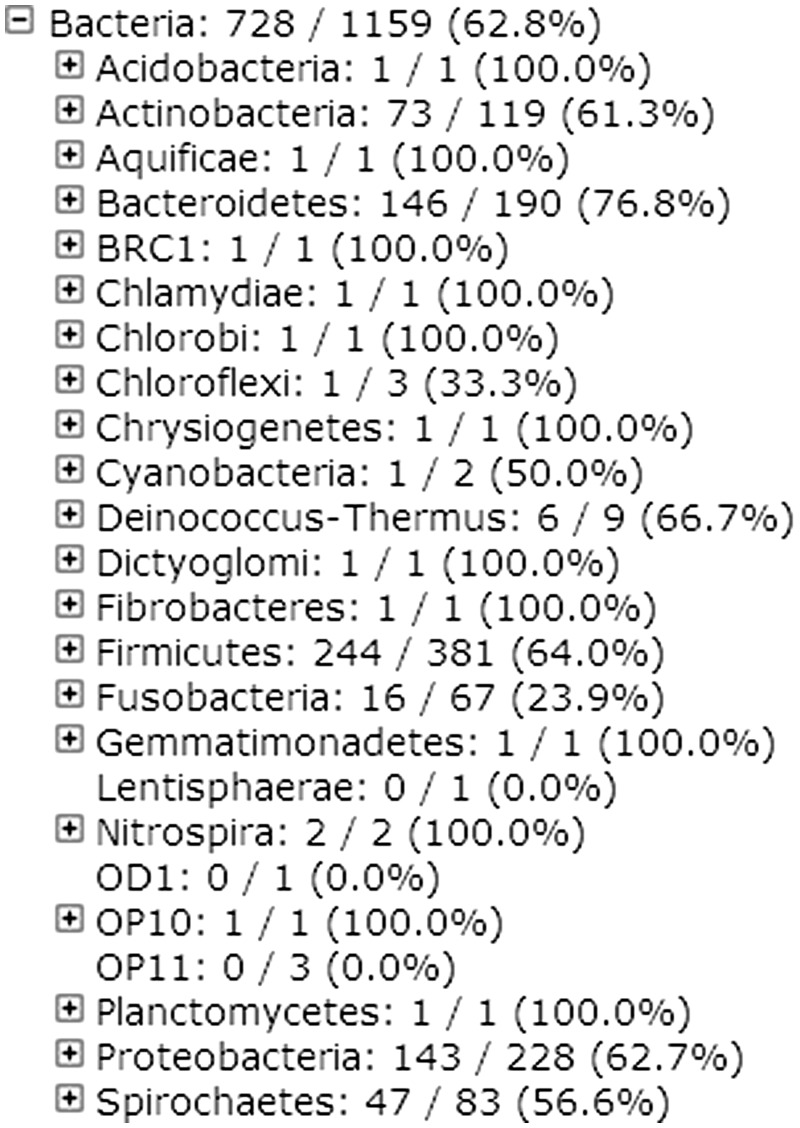
Partial tree view of the amplicons based on the CORE database. For each node, it shows the number of sequences targeted by the given primers, followed by number in the original reference as well as the percentage. The data used for the tree (except the percentages) is downloadable as the tab-delimited lineage file.
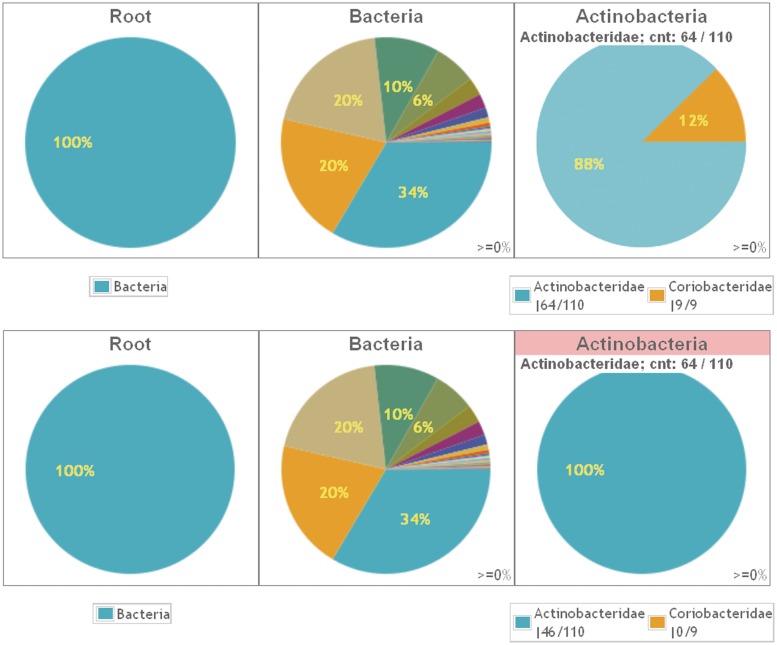
An example of pie plots for the amplicons (CORE database). The distribution of sub-categories within three taxonomic levels, shown as the chart titles, is plotted. The percentage threshold is 0 for all plots. The top panel series is obtained by clicking on Bacteria (Root pie) and Actinobacteria (Bacteria pie). Clicking a pie slice or legend label will produce the next chart and hide the legend of the previous one (except the legend of the Root pie). The bottom panel series of charts is similar, but for the phylum Actinobacteria a plot of differences, indicated by the pink header, is shown. Here, the data refers to the number of sequences missed by the amplicons as compared with the reference data. For the class Actinobacteridae, 46 out of 110 sequences are missing (see legend). The ‘100%’ in the Actinobacteridae pie slice illustrates that all missed sequences in the phylum Actinobacteria belong to the Actinobacteridae class. For Coriobacteridae, no sequences are missing (indicated by 0/9 in the legend). When hovering over a ‘legend’ label, always the number of sequences that are targeted is displayed in the pie (Actinobacteridae; cnt: 64/110). Therefore, this information is the same for both types of pies for Actinobacteria.
For the amplicon sequences, the differences between the taxa targeted by the amplicons compared with the reference can also be plotted. Now, the size of the pie slice relates to the number of sequences missing for this taxonomic level. The percentage threshold here filters on the percentage of missing sequences at the selected taxonomic level. This offers a detailed view on what taxa are absent. For example, with the threshold set to ≥70%, the pie only shows taxa for which at least 70% of the reference sequences are missing. At this threshold, relatively most sequences, not targeted by the primers, are from the phylum Fusobacteria (51 out of 67 in this example). Clearly, these numbers depend on the selected database. However, replacing the V5–V6 primers with V5–V7 primers provided better coverage of the Fusobacteria occurring in the oral cavity.
The pie charts are highly flexible: each pie can be set to plot the differences and each pie can have a different threshold. The selected thresholds are shown in the pie and a pink header indicates the ‘difference plots’.
CONCLUSION
The Taxman server provides a user-friendly way to carry out (multiplex) in silico PCR to produce trimmed versions of rRNA gene reference databases. Both the trimmed sequences and the distribution of targeted taxa can be downloaded for local use. TaxMan also supports interactive analysis of the taxonomic coverage including pie charts which can quickly illustrate, with taxonomic trees, which taxa, according to the selected rRNA database, are targeted by the primer set(s) and which are not. The use of the trimmed sequences instead of the full-length rRNA gene sequences in computational pipelines results in significant improvements in the use of computational resources.
FUNDING
University of Amsterdam under the research priority area ‘Oral Infections and Inflammation’ (to B.W.B.); National Science Foundation [NSF/BDI 0960626 to S.M.H.]; the European Union Seventh Framework Programme (FP7/2007-2013) under ANTIRESDEV grant agreement no 241446 (to E.Z.). Funding for open access charge: ANTIRESDEV.
Conflict of interest statement. None declared.
ACKNOWLEDGEMENTS
We would like to thank the teams who produce the databases used in TaxMan. We are also thankful to the SILVA team for providing the multiple sequence alignment of the SILVA SSU Ref NR data set.
REFERENCES
Articles from Nucleic Acids Research are provided here courtesy of Oxford University Press
Full text links
Read article at publisher's site: https://doi.org/10.1093/nar/gks418
Read article for free, from open access legal sources, via Unpaywall:
https://europepmc.org/articles/pmc3394339?pdf=render
Citations & impact
Impact metrics
Citations of article over time
Alternative metrics
Smart citations by scite.ai
Explore citation contexts and check if this article has been
supported or disputed.
https://scite.ai/reports/10.1093/nar/gks418
Article citations
Diet and the Gut Microbiome as Determinants Modulating Metabolic Outcomes in Young Obese Adults.
Biomedicines, 12(7):1601, 18 Jul 2024
Cited by: 1 article | PMID: 39062174 | PMCID: PMC11275099
VESPA: an optimized protocol for accurate metabarcoding-based characterization of vertebrate eukaryotic endosymbiont and parasite assemblages.
Nat Commun, 15(1):402, 09 Jan 2024
Cited by: 1 article | PMID: 38195557 | PMCID: PMC10776621
The hallmarks of dietary intervention-resilient gut microbiome.
NPJ Biofilms Microbiomes, 8(1):77, 08 Oct 2022
Cited by: 12 articles | PMID: 36209276 | PMCID: PMC9547895
The Evaluation of the Effects of Two Probiotic Strains on the Oral Ecosystem: A Randomized Clinical Trial.
Front Oral Health, 3:825017, 30 Mar 2022
Cited by: 3 articles | PMID: 35434705 | PMCID: PMC9007728
Gut Microbiota as Early Predictor of Infectious Complications before Cardiac Surgery: A Prospective Pilot Study.
J Pers Med, 11(11):1113, 29 Oct 2021
Cited by: 5 articles | PMID: 34834465 | PMCID: PMC8622065
Go to all (26) article citations
Similar Articles
To arrive at the top five similar articles we use a word-weighted algorithm to compare words from the Title and Abstract of each citation.
Design of 16S rRNA gene primers for 454 pyrosequencing of the human foregut microbiome.
World J Gastroenterol, 16(33):4135-4144, 01 Sep 2010
Cited by: 239 articles | PMID: 20806429 | PMCID: PMC2932916
Review Free full text in Europe PMC
TaxMan: a taxonomic database manager.
BMC Bioinformatics, 7:536, 18 Dec 2006
Cited by: 3 articles | PMID: 17176465 | PMCID: PMC1766369
Review Free full text in Europe PMC
Construction & assessment of a unified curated reference database for improving the taxonomic classification of bacteria using 16S rRNA sequence data.
Indian J Med Res, 151(1):93-103, 01 Jan 2020
Cited by: 6 articles | PMID: 32134020 | PMCID: PMC7055167
Exploring microbial diversity and taxonomy using SSU rRNA hypervariable tag sequencing.
PLoS Genet, 4(11):e1000255, 21 Nov 2008
Cited by: 519 articles | PMID: 19023400 | PMCID: PMC2577301