Abstract
Free full text

Repelled from the wound, or randomly dispersed? Reverse migration behaviour of neutrophils characterized by dynamic modelling
Abstract
Following neutralization of infectious threats, neutrophils must be removed from inflammatory sites for normal tissue function to be restored. Recently, a new paradigm has emerged, in which viable neutrophils migrate away from inflammatory sites by a process best described as reverse migration. It has generally been assumed that this process is the mirror image of chemotaxis, where neutrophils are drawn into the areas of infection or tissue damage by gradients of chemotactic cues. Indeed, efforts are underway to identify cues that drive neutrophils away by the reverse process, fugetaxis. By using photoconvertible pigments expressed in neutrophils in transparent zebrafish larvae, we were able to image the position of each neutrophil during inflammation resolution in vivo. These neutrophil coordinates were analysed within a dynamic modelling framework, using different forms of the drift–diffusion equation with model selection and parameter estimation based on approximate Bayesian computation. This analysis revealed the experimental data were best fitted by a model incorporating a diffusion term but no drift term—where the presence of drift would indicate fugetaxis. This result, for the first time, provides rigorous data-driven evidence that reverse migration of neutrophils in vivo is not a form of fugetaxis, but rather a stochastic redistribution.
1. Introduction
Neutrophils are critical immune cells, absolutely required for host defence against bacterial and fungal pathogens. They respond rapidly to tissue damage and possess a potent array of anti-microbial strategies [1]. Neutrophils are recruited early in inflammation, and in response to chemotactic cues, they polarize and are guided to the sites of wounding and infection [2–5]. These responses have evolved over hundreds of millions of years to deal vigorously with invading micro-organisms, but a consequence of this is that unchecked neutrophil activity can damage host tissues. There is, therefore, considerable interest in specifically understanding the resolution phase of the inflammatory process [6] and how this might fail in inflammatory disease [7].
Current mammalian models now enable visualization of neutrophil recruitment in vivo with striking results [8,9]. However, during resolution of inflammation, it is much more difficult to define the fates of cells as they are removed from inflammatory sites. In addition, genetic approaches allowing in vivo distinction of neutrophils from other myeloid cells are still uncommon. The transgenic zebrafish model, in contrast, allows in vivo visualization of individual immune cells during inflammation resolution [10–12]. By using the zebrafish model, fluorescently tagged neutrophils can be directly observed in transparent larvae by video-microscopy, and their behaviour analysed to provide insights into the underlying patterns governing their movement. Neutrophils have been observed to undergo apoptosis and to be engulfed by macrophages during inflammation in the zebrafish model [13,14]. There is increasing evidence that in addition to apoptosis, movement of neutrophils away from inflammatory sites is a significant event during inflammation [9,15–17], and may be a key regulatory step in inflammation resolution in the zebrafish [18]. Because this process cannot be easily visualized in mammalian systems, definitive proof of its importance is lacking, but considerable evidence points to its potential significance in mammalian biology [9,15]. In order to understand how this reverse migration is regulated, it is necessary to better understand what this process represents physiologically.
It has been widely assumed that the phenomenon of reverse migration represents neutrophils being driven away from sites of wounding by chemorepellents at the resolution stage of inflammation [16], with clear mechanistic implications. Indeed, this late-stage response is known variously as retrograde chemotaxis, chemorepulsion and fugetaxis [19,20] all of which imply a motivating force on the neutrophils. However, there is very limited analysis of neutrophil migratory patterns during the resolution phase of inflammation, which might confirm or refute this hypothesis.
To investigate neutrophil migratory patterns during the resolution phase of inflammation, we made use of the fluorescent protein Kaede, which fluoresces green, but which can be photoconverted to red fluorescence on exposure to light of certain wavelength [21]. This permits specific labelling of neutrophils recruited to a site of tissue injury at a defined timepoint, in a similar way to the Dendra2 system used by Yoo et al. [22,23]. Those cells can then be followed throughout the resolution phases of inflammation, without confounding them with newly recruited cells. While tracking of individual cells is possible in this model, for these analyses there are advantages to examining the X–Y positions of each neutrophil at each timepoint and treating them as a population with a probability density function, rather than a series of tracks. Specifically, we were able to include data from every identified cell and avoid any possibility of undue influence by long tracks.
In order to elucidate the type of process that best describes neutrophil reverse migration, we used mathematical techniques to model and then compare competing hypotheses of neutrophil movement patterns. Specifically, we sought to answer the question of whether neutrophils are driven away from the wound by chemorepellents (fugetaxis), or whether they simply cease responding to chemotactic cues after a period of time and instead begin a stochastic migration process that disperses the neutrophils away from the wound. To account for each separate hypothesis of cell migration, we used two distinct but related dynamic models: (i) the drift–diffusion model, which describes a directed-stochastic movement away from the wound, supporting the fugetaxis hypothesis, and (ii) the pure-diffusion model, which describes an undirected stochastic movement, supporting the hypothesis that the neutrophils disperse randomly from the wound site, possibly as part of a natural search pattern.
The drift–diffusion description of neutrophil migration used here is based on the random walk model, which has often been used to describe population migration dynamics [24] and cell behaviour in particular [25–27]. We have previously carried out an analysis of neutrophil movements during inflammation resolution using a drift–diffusion model of neutrophil migration based on regression analysis [28]. This study suggested that reverse migration was governed by a stochastic redistribution process, but was inconclusive for definitively determining cell behaviour in the resolution phase. In order to resolve this question definitively, we used the approximate Bayesian computation (ABC) framework [29–31] as a more rigorous approach to identifying the cell migration dynamics. The ABC method is well suited to dynamic modelling of cell migration because it is simple to simulate cell behaviour and then compare with the observations of cell movement [27]. This simulation approach to identification also facilitates the extension of the model with parameters that have potential significance for influencing migration: here, we exploit this feature by augmenting the drift–diffusion model with a term that describes cell movement along preferred spatial channels. This parameter additionally captures components of tissue anisotropy. A key advantage of our approach is that the output is not dependent on a complete understanding of factors that might influence the behaviour of the cell population. In particular, it includes the ultimate effects of phenomenon such as receptor–ligand binding–unbinding events, and the net effects of chemical or mechanical interaction between cells.
Our estimation framework is able to discriminate between different types of drift–diffusion migration process on synthetic examples, and when applied to observational data of neutrophil migration in vivo shows that this process is best fitted by models in which neutrophils randomly redistribute, rather than models in which neutrophils are driven away by fugetactic gradients. This has important implications for our understanding of the mechanisms of inflammation resolution.
2. Results
2.1. Experimental data
We photoconverted Kaede-expressing neutrophils in the vicinity of a wound 4 h post tailfin transection in transgenic zebrafish (figure 1a,b). These cells were initially in the range of 0–100 µm from the wound. We observed the evolving positions of these cells at 5 min intervals, using time-lapse videomicroscopy. Throughout the progression of the experiment, the cells remained most densely clustered near the wound, but their distribution widened over time as cells migrated away (figure 1c). Cells were not individually tracked but rather the distribution at each timepoint was considered as a population. Individual apoptotic events are not monitored with this approach because Kaede fluorescence is lost during neutrophil apoptosis (unpublished observation 2011) in the same way as it is for green fluorescent protein [13].
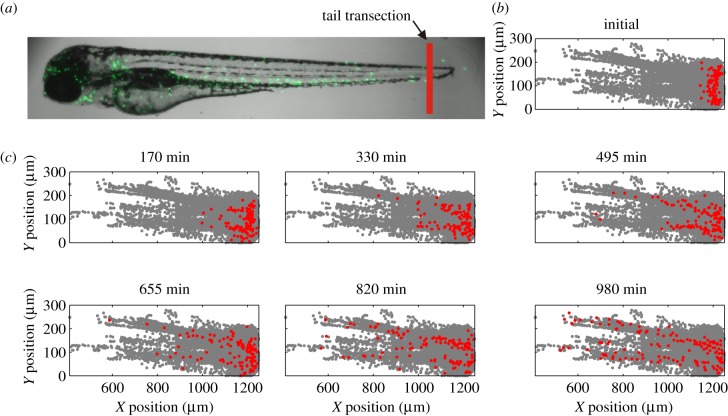
Experimental data: migration of photoconverted neutrophils away from a wound in zebrafish. (a) Image of a zebrafish larva showing the neutrophils expressing a green fluorescent protein and the approximate location for the tailfin transection. (b) Initial observed position of photoconverted neutrophils (red) and positions of all photoconverted cells at all timepoints (grey). The data are aligned and aggregated over six zebrafish and the wound was located in each case at approximately 1250 µm on the X-position axis. (c) Position of photoconverted neutrophils at increasing timepoints relative to experiment start (red) and positions of all photoconverted cells at all timepoints (grey).
Inspection of cell positions over all time suggested that while the cells moved freely over space close to the wound, away from the wound they tended to move in preferred spatial channels (figure 2). These channels do not appear to correspond to vascular or lymphatic structures, but always occupy the same areas of the fish. The implication is that neutrophils at the wound site may experience different levels of difficulty in leaving the wound. Neutrophils near the channels might find that leaving is easier than it is for neutrophils at the extremes. A complete model of cell behaviour requires a spatial restriction parameter to complement the drift and diffusion processes.
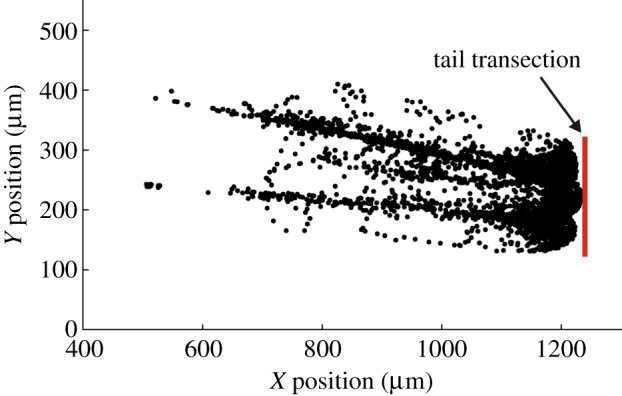
Migration of neutrophils away from the wound indicates preferred channels of movement. The positions of photoconverted neutrophils are shown at all timepoints for an individual zebrafish specimen (black dots). Many of the cell positions appear to be gathered along specific pathways within the extracellular matrix, suggesting that the neutrophils migrate along preferred channels. All six specimens of zebrafish showed a similar pattern. (Online version in colour.)
2.2. Validation of ABC–SMC identification framework on simulated data
We used the approximate Bayesian computation–sequential Monte Carlo (ABC–SMC) algorithm to both estimate model parameters and also select the optimal model for describing neutrophil migration (algorithm 1). ABC–SMC estimates model parameters by randomly sampling parameter values over some defined range, and rejecting values that lead to simulations where observed experimental data are not accurately predicted. Moreover, the ABC–SMC algorithm extends this approach to model selection by allocating each competing model an index value and treating selection of this index as part of the estimation problem (see §4 for full details). The focus in this investigation was on whether pure-diffusion or drift–diffusion models best described neutrophil migration. In order to validate the ability of the ABC–SMC algorithm to discriminate between pure-diffusion and drift–diffusion processes, we simulated and then identified cell migration governed by each of these mathematical descriptions.
Algorithm 1. Parameter estimation using ABC–SMC. |
---|
Require: data, ![]() ![]() ![]() ![]() |
Ensure: a set of parameter vectors ![]() ![]() ![]() |
![]() |
![]() ![]() ![]() ![]() ![]() |
![]() |
![]() ![]() |
![]() |
![]() ![]() ![]() |
![]() ![]() |
![]() ![]() ![]() ![]() ![]() ![]() |
![]() ![]() ![]() ![]() ![]() ![]() |
![]() ![]() |
![]() ![]() ![]() |
![]() ![]() ![]() ![]() |
![]() |
We simulated a pure-diffusion process from the initial cell positions of the experimental data. The diffusivity coefficient of the simulated process was set to 50 µm2 min−1. We collected the simulated cell positions so that they emulated observations of cell positions in the zebrafish. The results of the simulation and the results of applying the ABC–SMC model selection algorithm (algorithm 2) are displayed in figure 3a,b. The pure-diffusion model was correctly chosen with a high degree of confidence (77% of samples). The drift–diffusion model was represented by 23 per cent of samples. The maximum a posteriori (MAP1) estimate of diffusivity was 43 µm2 min−1, and the 90% CI was 30–55 µm2 min−1 with the true value lying within this interval. It is should be noted that diffusivity is related to displacement (and hence speed) indirectly, by the relationship in equation (4.1).
Algorithm 2. Model selection and parameter estimation using ABC–SMC. |
---|
Require: data, ![]() ![]() ![]() ![]() ![]() |
Ensure: a set of parameter vectors ![]() ![]() ![]() ![]() |
![]() |
![]() ![]() ![]() ![]() ![]() ![]() |
![]() |
![]() ![]() |
![]() |
![]() ![]() ![]() |
![]() ![]() |
![]() ![]() ![]() ![]() |
![]() ![]() ![]() ![]() ![]() |
![]() ![]() ![]() ![]() |
![]() ![]() ![]() ![]() ![]() ![]() |
![]() ![]() ![]() ![]() ![]() ![]() |
![]() ![]() |
![]() ![]() ![]() |
![]() ![]() ![]() ![]() ![]() |
![]() |
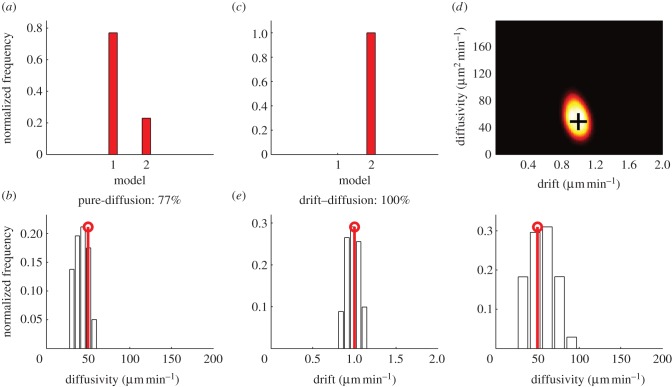
Identification of simulated drift–diffusion processes using ABC–SMC. (a,b) Model selection (a) and parameter estimation (b) of a simulated pure-diffusion process using the ABC–SMC algorithm, where model 1 is pure-diffusion and model 2 is drift–diffusion. The true diffusion parameter is represented by the stem plot. (c–e) Model selection (c) and parameter estimation (d, joint distribution; e, separated distributions) of a simulated drift–diffusion process using the ABC–SMC algorithm, where model 1 is pure-diffusion and model 2 is drift–diffusion. The true parameters are represented by the black cross and the stem plots, respectively. (Online version in colour.)
We simulated a drift–diffusion process, similarly to the pure-diffusion process discussed earlier, with the initial cell positions of the experimental data. The drift coefficient was set to 1 µm min−1, and the diffusivity coefficient was set to 50 µm2 min−1. The results of the simulation and the results of applying the ABC–SMC model selection algorithm (algorithm 2) are displayed in figure 3c–e. The drift–diffusion model was correctly chosen with 100 per cent confidence. The MAP parameters from the joint distribution was a drift of 0.97 µm min−1, and a diffusion of 62 µm2 min−1. The 90% CIs for the marginal distributions were a drift of 0.86–1.10 µm min−1, and a diffusion of 32–81 µm2 min−1, with the true values lying within these intervals. The ABC–SMC algorithm, therefore, robustly distinguishes between pure-diffusion and drift–diffusion processes in these simulated cases.
2.3. Identification of neutrophil dynamics during the resolution phase of inflammation
In order to characterize the migration dynamics of neutrophils in vivo, we applied the ABC–SMC model selection algorithm to experimental neutrophil data from six zebrafish larvae. The data were sampled at 5 min intervals for 980 min. We applied the ABC–SMC model selection algorithm (algorithm 2) with two candidate models: model 1, pure-diffusion and model 2, drift–diffusion. The marginal distribution over the models was 84 per cent for the pure-diffusion model, and 14 per cent for the drift–diffusion model. In the identified pure-diffusion model, the MAP estimate of diffusivity was 25 µm2 min−1, with 90% CI between 16 and 33 µm2 min−1. In the identified drift–diffusion model, the MAP drift coefficient was zero with 90% CI of 0–0.06 µm min−1, supporting the identification of the pure-diffusion model as the preferred model. Simulation of the identified pure-diffusion model demonstrated that the migration process was accurately described over the first half of the experiment (0–490 min; figure 4d). In the second half of the experiment (490–980 min), the distribution of the cells was not predicted as accurately by the model—the cell count at the wound was more than predicted and at approximately 250 µm from the wound, it was less than predicted (figure 4d). We took into account possible spatial restriction of cell movement by generating two further models for inclusion in the selection process: model 3 (diffusion–restriction) and model 4 (drift–diffusion–restriction). The results of model selection are displayed in figure 5a. The marginal distribution over the model was 25 per cent, 3 per cent, 52 per cent, 20 per cent, respectively for models 1–4. Hence, model 3, the pure-diffusion with spatial restriction parameter, was selected as the most likely model. For maximal accuracy in parameter identification, we applied the ABC–SMC parameter estimation algorithm (algorithm 1) only to model 3. The MAP parameter estimate of diffusivity was 45 µm2 min−1 and spatial restriction β = 0.65 (the joint distribution is shown in figure 5b). The inclusion of the spatial restriction parameter in the pure-diffusion model improved the prediction accuracy (figure 5c), so that 90 per cent of non-zero data points lay within the 90% CI (constructed by simulating the identified model 1000 times), as opposed to only 73 per cent with the pure-diffusion model. In order to validate the findings of this study across multiple independent datasets, an additional six examples were studied, and the data analysed, as mentioned earlier. As expected, the diffusion-restriction model was once more chosen with high confidence (see the electronic supplementary material, figure S1), underpinning the broader applicability of this model.
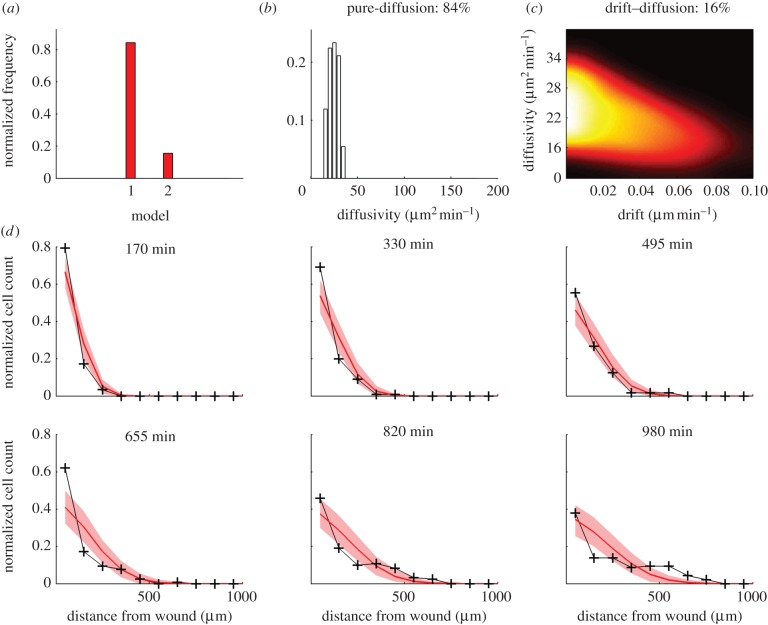
Identification of resolution phase neutrophil migration dynamics with model selection between pure-diffusion and drift–diffusion models. (a) Model selection: model 1 is pure-diffusion and model 2 is drift–diffusion. (b) Estimate of diffusivity for the pure-diffusion model. (c) Joint estimates of drift and diffusivity for the drift–diffusion model (zoomed in with respect to support of full uniform prior). (d) Simulation of the pure-diffusion model, model 1 (red), and comparison with experimental observations (black). The shaded region (red) shows the 90% confidence range obtained from 1000 simulations using the MAP estimate of the diffusion parameter.
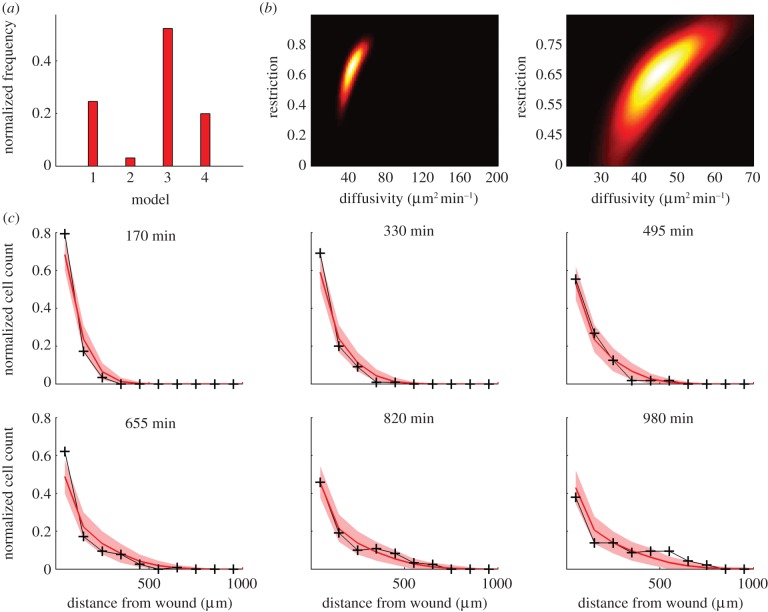
Identification of resolution phase neutrophil migration dynamics with restriction to preferred movement channels. (a) Model selection: model 1 is pure-diffusion; model 2 is drift–diffusion; model 3 pure-diffusion with restriction and model 4 is drift–diffusion with restriction. (b) Estimated joint distribution of parameters for model 3 (left panel over full support of the uniform prior and right panel zoomed in). (c) Simulation of the pure-diffusion with restriction model, model 3 (red), and comparison with experimental observations (black). The shaded region (red) shows the 90% confidence range obtained from 1000 simulations using the MAP estimates of the diffusion–restriction parameters.
3. Discussion
The mechanisms driving inflammation resolution are therapeutically important, and there is a fundamental mechanistic difference between the classes of molecular event that drive neutrophils away from inflammatory sites, and those that allow neutrophils to be blind to, or to ignore, chemotactic gradients that might retain neutrophils at inflammatory sites. The question of whether neutrophil behaviours are modelled best by fugetaxis or by stochastic redistribution is of fundamental importance in our understanding of inflammation resolution. We therefore investigated whether the reverse migration of neutrophils in vivo was best described by a pure-diffusion or a drift–diffusion process. These alternative mathematical descriptions correspond to stochastic redistribution of neutrophils following inherent behavioural patterns and to a directed fugetactic process, respectively. We used ABC–SMC method with model selection to identify these models, using datasets in which the positions of neutrophils leaving a site of tissue injury could be specifically observed.
We first applied the ABC–SMC algorithm in silico in order to verify that we could discriminate between different types of drift–diffusion process. This is an important step when applying ABC–SMC in a new context [32], such as here for the case of cell migration in the inflammation resolution phase. When we applied the ABC–SMC identification algorithm to a pure-diffusion process, the correct model was identified with strong confidence. The alternative model had a low representation in the marginal posterior distribution and, in addition, the MAP drift coefficient was zero. Moreover, when the ABC–SMC algorithm was applied to a simulated drift–diffusion process, the correct model was identified with certainty. Hence, our validation procedure demonstrated that for the drift–diffusion case, the ABC–SMC algorithm can achieve accurate results.
To investigate the reverse migration behaviour of the neutrophils, we applied the estimation algorithm to experimental data from zebrafish larvae during the resolution phase of an inflammation episode. The pure-diffusion model was identified with a high degree of confidence (84%) and the MAP drift coefficient of the alternative drift–diffusion model was estimated to be zero. These results strongly suggest that the process by which neutrophils migrate away from the wound in the zebrafish is best characterized as a form of stochastic redistribution without directional bias. Even in the event that the model was incorrectly identified and a small non-zero drift coefficient was present at the limit of the identified 90% CI (0.06 µm min−1), this would only make a very small contribution to migration (an approx. 60 µm mean shift over the 980 min span of the experiment). This, on its own, would still leave the cells in the vicinity of the wound. Therefore, it is the identified diffusivity arising from the inherent migratory patterns of the neutrophils that is contributing the major part of the motility, and this is why cells are often seen to change direction.
It is apparent from inspecting and comparing the pure-diffusion model simulations and in vivo observations (figure 4d) that the pure-diffusion model does not fully explain the response. Our modelling fits the actual data well at earlier timepoints, but at later times (655–980 min) the predicted distribution of cells did not precisely describe the observed cell behaviour: the cell count at the wound was greater than predicted and was less than predicted at approximately 250 µm from the wound. This suggests that our model is incompletely capturing the nature of neutrophil movements; specifically, it suggests that neutrophils do not move away from the wound as easily as a pure-diffusion model would suggest. This is confirmed by inspection of the distribution of neutrophil positions (figure 2). To address this issue, we included an additional component into the migration model describing preferred paths of cell movement through the tissues of the zebrafish, characterized by a spatial restriction parameter. This addition was motivated by the observation that cell positions appeared to be gathered in specific spatial channels. In addition, we were concerned that omission of a necessary restriction parameter might mask the presence of drift. By including this model term, we enhanced our ability to detect drift, and hence any directed migration of the neutrophils. The data-driven nature of the Bayesian estimation framework meant that the value of this parameter was arrived at independently of any preconceptions or attempts to model any particular feature. It could have been rejected by the model selection algorithm or had a zero value, indicating that it was not necessary, but it was found to have a positive value of 0.65. Such channels might arise owing to physical characteristics of the local environment: it might be physically easier to displace tissue matrix around the notochord than in the tailfin. However, they do not correspond to vascular or lymphatic structures, which would suggest that they are a feature of extravascular tissues. In addition, the site of the channels is similar in different fish, and not a reflection of random paths chosen by individual cells defining paths of subsequent neutrophil migrations. We used the ABC–SMC algorithm to objectively select between models that incorporated combinations of diffusion, drift and spatial restriction parameters and found that the preferred model was pure-diffusion with spatial restriction. An advantage of the ABC–SMC estimation framework used here, in comparison with alternatives based on, for example, regression analysis [28,33,34] is that further identified complexities such as spatial restriction can easily be incorporated into the model as they are discovered.
In a preliminary investigation into the drift–diffusion behaviour of neutrophils using regression analysis, we obtained simulation results that gave an early indication that reverse neutrophil migration was governed by a stochastic redistribution process rather than by a fugetactic process [28]. In those simulations, the distribution mode of the cell count predicted by an identified drift–diffusion model (using regression analysis with model hypothesis testing) shifted away from the wound over time, which was in obvious disagreement with observation [28]. This conflict between simulation analysis and model hypothesis testing was not resolved, motivating us to develop new approaches to definitively answer this important question. In the current study, model selection results using the ABC–SMC framework supported a diffusion-only process with spatial restriction. Moreover, the simulation results of the diffusion–restriction model accurately predicted experimental observations, corroborating this finding. In this investigation, all results are consistent and provide compelling evidence that the reverse migration of neutrophils in the resolution phase of inflammation is a form of stochastic redistribution, not fugetaxis.
Mathias et al. [16] and Brown et al. [17] have described reverse migration of neutrophils in zebrafish using the term retrograde chemotaxis. This seems to have been based on observing a high directionality index in neutrophils migrating away from a wound, comparable to that for incoming cells. However, the directionality index could be misleading, particularly when cells have short-term directional persistence, which has been reported in neutrophil movement [26,35] and is built into many cell migration models (see Dickinson & Tranquillo [34]). Even in the absence of external cues, a high directional index will be observed over any timescale shorter than the characteristic persistence time and also in selected tracks over longer timescales. In our investigation, we have avoided the confounding issue of persistence by observing and analysing cell migration over a longer time-scale and by avoiding any selectivity in the use of cell data.
In summary, we have used a new transgenic zebrafish model, where neutrophils express the photoconvertible protein Kaede, to investigate neutrophil migration during inflammation resolution. Dynamic modelling of neutrophil behaviour using approximate Bayesian computation has revealed that, on a population level, there is no evidence for retrograde chemotaxis/fugetaxis. Instead, we find that the reverse migration of neutrophils from sites of wounding is best described as stochastic redistribution, and reflects inherent behaviours of the neutrophil. This would seem to refute the hypothesis that neutrophils in the zebrafish are repelled from the wound during the resolution phase of inflammation.
4. Methods
4.1. Ethics statement
All animal work was performed according to guidelines and legislation set out in UK law in the Animals (Scientific Procedures) Act 1986. Ethical approval was given by the University of Sheffield Local Ethical Review Panel.
4.2. Transgenic lines
The Tg(lyz:Gal4)i252 [18] and Tg(UAS:Kaede)s1999t [36] zebrafish lines are described elsewhere. Briefly, the yeast transcription factor Gal4, fused to VP16 viral transcriptional activator sequence, recognizes and drives transcription from the upstream activator sequence (UAS). The Gal4 sequence is inserted into a DNA vector 3 of a PCR-generated promoter for lysozyme C, which has almost complete overlap with the neutrophil-specific mpx promoter at early developmental stages. This construct is injected into fertilized eggs, allowing random incorporation into the genome and driving expression of Gal4 in neutrophils in subsequent generations. In parallel, a second transgenic line is generated in the same way, expressing the photoconvertible protein, Kaede, under a UAS sequence. Thus, in the double transgenics, generated by crosses of the two single transgenics, Kaede is expressed in neutrophils. Transgenic lines were maintained according to standard protocols [37].
4.3. Image acquisition
The Tg(lyz:Gal4)i252 [18] and Tg(UAS:Kaede)s1999t [36] zebrafish lines were maintained according to standard protocols [37]. Image acquisition is described elsewhere [18]. Briefly, a Perkin Elmer UltraVIEW VoX ERS 6FR spinning disc confocal (Perkin Elmer, Inc., USA) mounted on an inverted Olympus IX81 microscope, in conjunction with the UltraVIEW PhotoKinesis device was used for photconversion with 40 per cent laser energy for 120 cycles of the 405 nm laser line. Subsequent images were taken with a Nikon Eclipse TE2000-U Inverted Compound Fluorescence Microscope (Nikon UK Ltd.), and initial image processing was performed using Volocity v. 5.3.2 (Perkin Elmer). Individual cell positions at each timepoint were exported for further analysis. Tracking of individual cells was not performed.
4.4. Representation of cell migration: the drift–diffusion equation
It has been reported that neutrophils move according to a correlated random walk (or biased correlated random walk if a directional cue if present) [26,35]. However, over the timescales, we considered in the application to neutrophils, which were greater than the typical directional persistence times [26], this type of motion approaches the limit of a pure-diffusion (or drift–diffusion) process [24]. We modelled this motion in one dimension with a boundary at x = 0, according to the following discrete time model.

where is the position of the ith neutrophil at time t, for
; bout is a bias velocity away from the boundary; D is the underlying diffusivity constant or magnitude of random movement of the neutrophils;
are a family of independent white noise processes; Δt is the time lapse between observations. The observations of cells is described by

where , and so we defined a complete observation set as
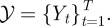
Equation (4.1) implies that cells stop on reaching the boundary until the next time increment.
Equation (4.2) represents there being no correspondence between cell positions at different times (i.e. it was not possible to observe cell tracks) and the possibility that not all cells were observed at every observation time. It was straightforward to simulate this system by sampling the from a normally distributed random number generator.
Equations (4.1) and (4.2) represented both the models we considered: with bout = 0, it is the pure-diffusion model; with bout taking any non-negative value, it is the drift–diffusion model.
4.5. Physical restrictions included in the model
Away from the vicinity of the tailfin area, the neutrophils tended to move preferentially through defined channels (figure 2). This meant they were more likely to leave the wound area if they were near to one of these channels. Conversely, if they were not near the entrance of a channel, their exit was likely to be restricted. In order to account for this in a simplified way, two additional models were proposed.
Positions update: for ,

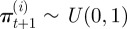

where is now the proposed position of the ith neutrophil at time t + 1; πt(i) is a test random variable for the ith particle for simplified modelling wound exit restriction
is a constant denoting the strength of the wound exit restriction (β = 0 means no restriction); xR is the distance from the wound at which exit restriction is deemed to be experienced. It was chosen as 100 µm, the minimum distance which contains all the cell positions at the time of photoconversion.
Equations (4.4)–(4.6) with is the diffusion–restriction model and with bout taking any non-negative value, it is the drift–diffusion–restriction model.
4.6. Parameter estimation and model selection using approximate Bayesian computation
In general, given a prior distribution over parameters θ, we want to evaluate the posterior distribution over the parameters
where
represents some observations of the system being investigated. According to Bayes' theorem,
where
is the likelihood of θ with respect to the observations.
For systems where the likelihood is intractable, a typical ABC procedure approximates the posterior distribution by

where ρ is a distance function, is a set of simulated observations and S is a function that returns a summary statistic derived from the observations. If S yields a sufficient summary statistic of the data and
is sufficiently small, then the desired approximation will be close to the true posterior distribution. A summary statistic S is necessary where the observations are too complex or high dimensional to compare directly in an efficient way.
It is clear that the joint distribution on right-hand side of equation (4.7) and hence the approximate posterior distribution can be sampled as follows:
—
simulate a parameter vector θ from the prior;
—
simulate a dataset from the process
;
—
calculate the summary statistic
and measure its distance, ρ, from that of the true observation; and
—
accept θ as a sample from the posterior distribution if
.
This is known in the ABC literature as the ABC rejection sampler.
In its simplicity, this algorithm can be inefficient, particularly if the prior distribution is wide relative to the target posterior distribution. This becomes more problematic when the process of simulating a dataset is computationally intensive. Various methods have been developed to improve ABC efficiency. We found that the ABC–SMC approach of [31], which we implemented in Matlab, gave a good balance of efficiency and ease of implementation. This algorithm iteratively improves on the prior over θ with a sequence of converging approximate posterior distributions. It achieves this by using an error tolerance schedule that is relaxed to begin with but narrows progressively to the final error tolerance. The approximate posterior distribution identified at iteration t becomes the updated prior distribution, which is sampled from at iteration t + 1. ABC–SMC also allowed a natural extension to model selection, which is a fundamental issue for the problem we were addressing. In this case, the model index is included as an extra parameter in the system.
Our implementation of the ABC–SMC algorithm and ABC–SMC with model selection algorithm are set out in algorithms algorithms11 and and22.
4.7. The Bhattacharyya distance for comparing simulated and observed cell distributions
In order to apply an ABC scheme for parameter estimation, it was necessary to define a metric for comparing complete observations sets. This, in turn, was dependent on choosing suitable summary statistics of the complete observation set. The Bhattacharyya distance is widely used in statistical signal processing for measuring the distance between distributions [38]. We used this to construct a summary statistic. First, we processed the observations into a normalized distributional format, as follows:
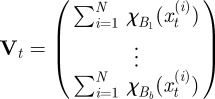
and
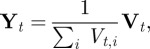
where is a set of spatial intervals forming a partition of the range of the
;
is the indicator function of the interval
; and
is thus the normalized form of
.
For two discrete distributions and
over the same domain X, the Bhattacharyya distance
between them is

So, because we had a discrete distribution at each of the t timepoints, we defined the distance between two complete observation sets as follows:

where is the ith component of the vector
.
4.8. Implementation details
Parameters for the algorithm were chosen as follows, N = 10 and P = 4000. Uniform priors were used over all parameters with the following ranges: diffusivity: 0–200 µm2 min−1, drift: 0–2 µm2 min−1, restriction: 0–1. In practice, the error tolerances were chosen automatically as follows: an initialization run was performed, choosing model parameter sets from the joint prior to form a set of P, parameter sets with associated errors, .
was chosen as 0.75 max
and
was chosen as the first percentile of the
. The intermediate tolerances were chosen such that
. For efficiency, parameter sets from the initialization step were recycled into the first iteration if their associated error was less than
. The parameter perturbation kernel was chosen to be zero mean Gaussian with variance computed as in the algorithm. In applying the model perturbation kernel, we kept the original model with probability 0.6 and chose one of the r remaining models with probability 0.4/r.
In Toni et al. [31], simulations were repeated for every chosen parameter set and the weight for that parameter adjusted according to how many simulations produce an error within the current tolerance. We found it beneficial instead to average the results of the repeated simulations before applying the distance metric. This took into account all the simulation results rather than ignoring those outside the tolerance. We found that this could also be achieved efficiently by simulating multiple copies of the system at once and then applying equations (4.9) to the aggregated results.
Acknowledgments
The authors gratefully acknowledge that this work was supported by the Engineering and Physical Sciences Research Council (EPSRC), UK; a European Research Council Advanced Investigator Award (S.A.B.); an MRC Senior Clinical Fellowship (S.A.R.) (reference number: G0701932) and an MRC Centre grant (G0700091). Microscopy studies were supported by a Wellcome Trust grant to the MBB/BMS Light Microscopy Facility (GR077544AIA).
Footnotes
1If the value of a quantity x is specified by a posterior distribution, then the MAP estimate of x is the x-value corresponding to the maximum value of the distribution. In other words, it is the most likely value of x.
References
Articles from Journal of the Royal Society Interface are provided here courtesy of The Royal Society
Full text links
Read article at publisher's site: https://doi.org/10.1098/rsif.2012.0542
Read article for free, from open access legal sources, via Unpaywall:
https://royalsocietypublishing.org/doi/pdf/10.1098/rsif.2012.0542
Citations & impact
Impact metrics
Article citations
Fusobacterium nucleatum dissemination by neutrophils.
J Oral Microbiol, 15(1):2217067, 04 Jun 2023
Cited by: 4 articles | PMID: 37283724 | PMCID: PMC10240972
GZMKhigh CD8+ T effector memory cells are associated with CD15high neutrophil abundance in non-metastatic colorectal tumors and predict poor clinical outcome.
Nat Commun, 13(1):6752, 08 Nov 2022
Cited by: 21 articles | PMID: 36347862 | PMCID: PMC9643357
Neutrophil reverse migration.
J Inflamm (Lond), 19(1):22, 24 Nov 2022
Cited by: 5 articles | PMID: 36424665 | PMCID: PMC9686117
Review Free full text in Europe PMC
Wound Healing Insights from Flies and Fish.
Cold Spring Harb Perspect Biol, 14(11):a041217, 01 Nov 2022
Cited by: 2 articles | PMID: 35817511 | PMCID: PMC9620851
Review Free full text in Europe PMC
Live Imaging of Heart Injury in Larval Zebrafish Reveals a Multi-Stage Model of Neutrophil and Macrophage Migration.
Front Cell Dev Biol, 8:579943, 19 Oct 2020
Cited by: 11 articles | PMID: 33195220 | PMCID: PMC7604347
Go to all (23) article citations
Similar Articles
To arrive at the top five similar articles we use a word-weighted algorithm to compare words from the Title and Abstract of each citation.
Drift-Diffusion Analysis of Neutrophil Migration during Inflammation Resolution in a Zebrafish Model.
Adv Hematol, 2012:792163, 29 Jul 2012
Cited by: 20 articles | PMID: 22899935 | PMCID: PMC3413999
A Bayesian framework for identifying cell migration dynamics.
Annu Int Conf IEEE Eng Med Biol Soc, 2013:3455-3458, 01 Jan 2013
Cited by: 0 articles | PMID: 24110472
Defining the phenotype of neutrophils following reverse migration in zebrafish.
J Leukoc Biol, 98(6):975-981, 12 Jun 2015
Cited by: 42 articles | PMID: 26071567 | PMCID: PMC4661044
Real-time imaging of inflammation and its resolution: It's apparent because it's transparent.
Immunol Rev, 306(1):258-270, 12 Jan 2022
Cited by: 10 articles | PMID: 35023170 | PMCID: PMC8855992
Review Free full text in Europe PMC
Funding
Funders who supported this work.
Engineering and Physical Sciences Research Council (2)
Grant ID: EP/H00453X/1
Grant ID: EP/G042209/1
Medical Research Council (3)
Grant ID: G0700091B
Centre for Developmental and Biomedical Genetics
Professor Philip Ingham, University of Sheffield
Grant ID: G0700091
Zebrafish genetic approaches to understanding the regulation of inflammatory cell apoptosis.
Professor Stephen Renshaw, University of Sheffield
Grant ID: G0701932
Wellcome Trust (1)
Grant ID: GR077544AIA