Abstract
Free full text

Cumulative Haploinsufficiency and Triplosensitivity Drive Aneuploidy Patterns to Shape the Cancer Genome
Summary
Aneuploidy has been recognized as a hallmark of cancer for over 100 years, yet no general theory to explain the recurring patterns of aneuploidy in cancer has emerged. Here we develop Tumor Suppressor and Oncogene (TUSON) Explorer, a computational method that analyzes the patterns of mutational signatures in tumors and predicts the likelihood that any individual gene functions as a tumor suppressor (TSG) or oncogene (OG). By analyzing >8200 tumor-normal pairs we provide statistical evidence suggesting many more genes possess cancer driver properties than anticipated, forming a continuum of oncogenic potential. Integrating our driver predictions with information on somatic copy number alterations, we find that the distribution and the potency of TSGs (STOP genes), OGs and essential genes (GO genes) on chromosomes can predict the complex patterns of aneuploidy and copy number variation characteristic of cancer genomes. We propose that the cancer genome is shaped through a process of cumulative haploinsufficiency and triplosensitivity.
Introduction
A key goal of cancer research is to identify genes whose mutation promotes the oncogenic state. Research over the last 40 years has identified numerous potent drivers of the cancer phenotype (Meyerson et al., 2010; Stratton et al., 2009; Vogelstein et al., 2013). Perhaps the most striking characteristics of cancer genomes are their frequent somatic copy number alterations (SCNAs) and extensive aneuploidies. Deletions and amplifications of whole chromosomes, chromosome arms, or focal regions are rampant in cancer, as are other rearrangements such as translocations and chromothripsis. Understanding how these events drive tumorigenesis is a major unmet need in cancer research.
While ostensibly random, these alterations follow a non-random pattern that suggest they are under selection and likely to be cancer drivers rather than passengers. If so, we should be able to explain how they drive tumorigenesis. A recent clue as to how this might work came from the integration of a genome-wide RNAi proliferation screen with focal SCNA information (Solimini et al., 2012). The screen identified STOP and GO genes which are negative and positive regulators of cell proliferation, respectively. Hemizygous recurring focal deletions were enriched for STOP genes and depleted of GO genes suggesting that the deletions maximize their pro-tumorigenic phenotype through cumulative haploinsufficiency of STOP and GO genes. Haploinsufficiency describes a genetic relationship in a diploid organism in which loss of one copy of a gene causes a phenotype. The converse is triplosensitivity, in which an additional copy of a gene produces a phenotype. However, the distributions of STOP and GO genes were not able to predict aneuploidy or chromosome arm SCNA frequencies, perhaps because they represent only one aspect of tumorigenesis (proliferation) or are too diluted by non-haploinsufficient genes. We hypothesized that the drivers of sporadic tumorigenesis might provide a more representative and potent set of STOP and GO genes with which to explore this phenomenon. Furthermore, this gene set may possess a higher frequency of haploinsufficiency.
In this study we developed methods to identify tumor suppressor genes (TGSs) and oncogenes (OGs) from tumor DNA sequences. We implicate many new drivers in cancer causation and find many more cancer drivers than expected that exist in a continuum of decreasing phenotypic potential. Furthermore, we found that the distribution and potency of TSGs, OGs and essential genes on chromosomes can explain copy number alterations of whole chromosomes and chromosome arms during cancer evolution through a process of cumulative haploinsufficiency and triplosensitivity.
Results
Cancer driver genes have been described as mountains and hills (Wood et al., 2007). Mountains are driver genes that are very frequently mutated in cancer while hills represent less frequently mutated driver genes. It has become clear from recent international sequencing efforts that most potent drivers (mountains) have been discovered. A key issue is how to determine the identity of the significant but less frequently mutated drivers, the hills. A recent analysis searching for very high confidence cancer drivers in a database of ~400K mutations estimated that there were 71 TSGs and 54 OGs (Vogelstein et al., 2013). It is likely that there also exists additional functionally significant cancer drivers with weaker phenotypes and lower probabilities that are selected less frequently. A central question is how to identify these genes. In principle, with more samples analyzed, greater statistical significance can be placed on the outliers allowing discovery of lower penetrance drivers. However, it is likely that there is more information present in the current data that may allow these lower frequency events to be detected.
To approach this question, we sought to devise a method to predict TSGs and OGs in cancer based on the properties of gene mutation signatures of these two distinct classes of driver genes. We hypothesized that the proportion of the different types of mutations with different functional impact would be informative in predicting these two types of drivers (Fig. 1A). Each gene has a background mutation rate that is dependent on transcription, replication timing and possibly other unknown parameters that can be estimated by the number of mutations that are unlikely to affect its function (such as silent or functionally benign mutations), whose observed frequency is not dependent on selective pressure during cancer evolution. The proportion of functionally relevant mutations of particular classes compared to this background mutation rate will be dependent on the degree of selection and will predict the likelihood that a gene will act as a cancer driver. TSGs and OGs can be distinguished among the cancer driver genes based on the characteristic pattern of the different types of mutations (i.e. loss of function (LOF), missense, silent) that are typically observed for those two classes of drivers relative to neutral genes as illustrated in Fig. 1B.
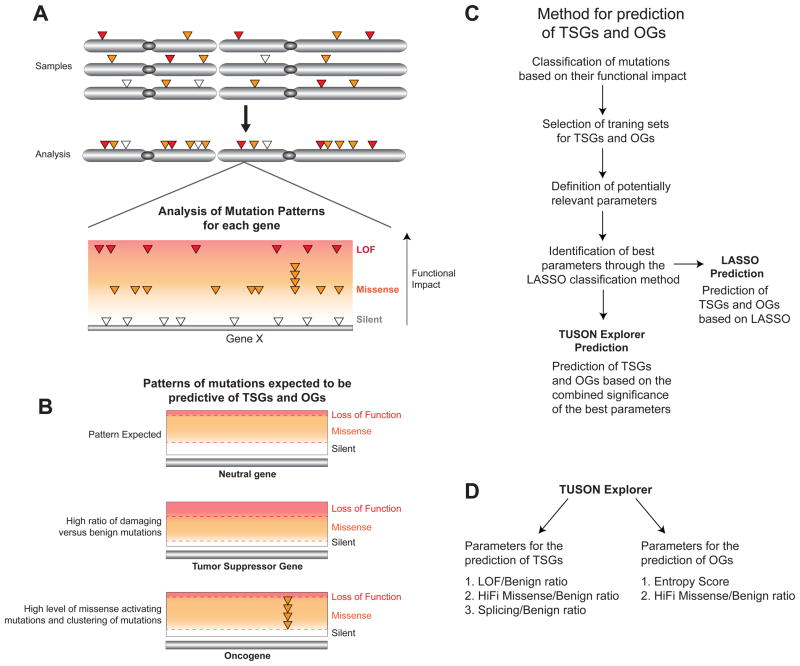
A) Schematic representation of our method for the detection of cancer driver genes based on the assessment of the overall mutational profile of each gene as compared to the average profile found in neutral genes. The somatic mutations in each gene from all tumor samples are combined and classified based on their predicted functional impact. The main classes of mutations (silent, missense and LOF) are depicted.
B) Schematic depicting the most important features of the distributions of mutation types expected for a typical TSG, OG and Neutral gene. Compared to a ‘neutral’ gene, TSGs are expected to display a higher level of inactivating mutations compared to their background mutation rate (benign mutations) and OGs are expected to display a higher level of activating missense mutations and a characteristic pattern of recurrent missense mutations in specific residues.
C) A flowchart delineating the main steps in our method for identifying predictive parameters for TSGs and OGs from the classification of mutations based on their functional impact through Lasso, and the use of these selected parameters for the prediction of TSGs and OGs by TUSON Explorer (or the Lasso method).
D) Schematics related to (C) depicting the main parameters of the mutation profiling method employed by TUSON Explorer for the prediction of TSGs and OGs (HiFI: High Functional Impact). For TSGs, the parameters are the LOF/Benign ratio, the HiFI/Benign ratio and the Splicing/Benign ratio, while for OGs they are the Entropy score and the HiFI/Benign ratio.
Also see Supp. Fig. 1 and Supp. Table 1.
Identification of parameters predicting TSGs and OGs
We set out to determine the most reliable parameters for the prediction of TSG and OG in an unbiased way (Fig. 1C). We used sequence data from over 8200 tumors from the COSMIC (Forbes et al., 2010) and TCGA (http://cancergenome.nih.gov/) databases and a recently published database (Alexandrov et al, 2013) comprising over 1,000,000 mutations (Supp. Fig. 1, Supp. Table 1). We defined a list of 22 parameters primarily based on the different classes of mutations and used the classification method Lasso and three training sets of known TSGs and OGs (from the Cancer Gene Census, Futreal et al., 2004) (Supp. Table 2a), and neutral genes to identify those parameters that best predict the two classes of driver genes (see methods). We employed PolyPhen2 to predict the functional impact of missense mutations in order to classify them into those with potentially high (HiFI) or low (LoFI) functional impact. LoFI mutations are typically conservative amino acid changes or changes in poorly conserved residues (Adzhubei et al., 2010). We defined the combination of silent and LoFI missense as “Benign” mutations to provide a larger, more reliable value for estimating background mutation rates. As a majority of known OGs show an atypical distribution of recurrent mutations in one or a few key residues, we utilized Entropy, a well-defined concept in physics and information theory (Shannon and Weaver, 1949) to measure the degree of reoccurring mutations within a gene (see methods). The Entropy score represents the sum of the probabilities across a gene that a site is mutated. The best parameters found for the prediction of TSGs and OGs are described below and visualized in Fig. 1D and Fig. 2.
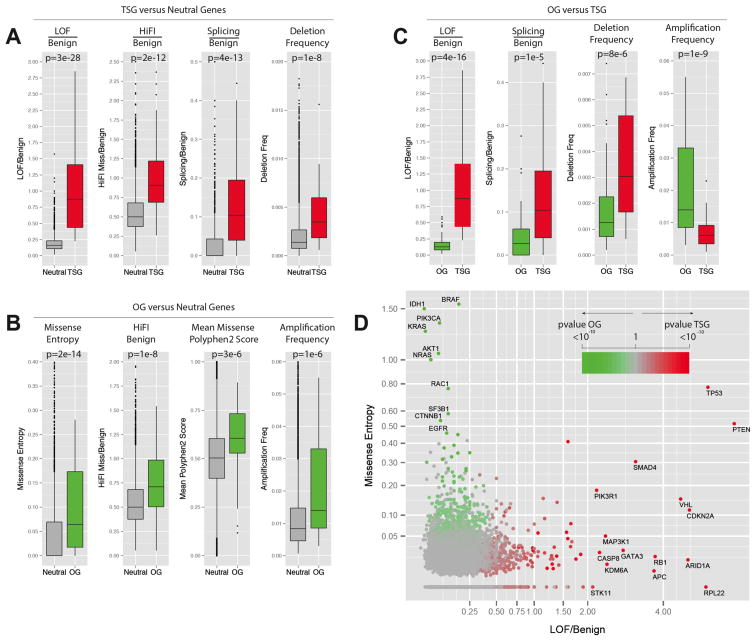
Panels A–C contain a boxplot representation of the distribution of the values for the indicated parameters in the indicated Neutral genes (grey), TSG training set (red) and OG training set (green). The median, first quartile, third quartile and outliers in the distribution are shown. The p-value for the difference in the two indicated distributions is shown as derived by the Wilcoxon test.
A) Boxplots showing the distribution of LOF/Benign, HiFI missense/Benign, Splicing/Benign ratios and the high frequency of focal deletion among the Neutral gene set and the TSG.
B) Boxplots showing the distribution of Missense Entropy, HiFI missense/Benign, mean of PolyPhen2 score and the high frequency of focal amplification among the Neutral gene set and the OGs.
C) Boxplots showing the distribution of LOF/Benign, Splicing/Benign ratios, high-frequency of deletion and high frequency of amplification among the TSG and OG sets.
D) Plot of the LOF/Benign ratio and Missense Entropy for each gene, the best parameters for discriminating between TSGs and OGs. Specific genes with high levels of LOF/Benign or Entropy Missense are shown along with their p-value for being a TSG or an OG (TUSON Explorer).
Also see Supp. Fig. 2 and Supp. Table 2.
Tumor suppressors versus Neutral genes (Fig. 2A and Supp. Table 2b)
The most predictive parameters for TSGs are: 1) the ratio of LOF mutations to Benign (p = 2.51 × 10−28, Wilcoxon, one-tailed test); 2) the ratio of Splicing to Benign mutations (p= 4.0 × 10−14); 3) the ratio of HiFI missense mutations to Benign mutations (p = 1.95 × 10−12); and 4) high-level deletion frequency (p= 1.46 × 10−8). A 20-fold cross-validation shows a high prediction accuracy of 93.2% on these training sets.
Oncogenes versus Neutral genes (Fig. 2B and Supp. Table 2b)
The most predictive parameters for OGs are: 1) the entropy for missense mutations (p-value = 2.2 × 10−14); 2) the ratio of HiFI missense mutations to Benign mutations (p= 1.2 × 10−8); and 3) high-level amplification frequency (p = 1.4 × 10−6). The 20-fold cross-validation accuracy on these training sets is 85.2%.
Tumor suppressor genes versus Oncogenes (Fig. 2C and Supp. Table 2b)
One important aim of our prediction method is the discrimination between TSGs and OGs. The most predictive parameters between these two sets are: 1) LOF/Benign (p=3.8 × 10−16); 2) amplification frequency (p= 1.3 × 10−9); 3) high-level deletion frequency (p= 7.6 × 10−6); and 4) the ratio of Splicing/Benign mutations (p= 1.1 × 10−5). The 20-fold cross-validation accuracy is 91.9%. Overall, Lasso identified parameters that make intuitive sense for these classes of genes and clearly delineated TSGs and OGs from each other and from neutral genes. In sum, we identified independent parameters that strongly predict and distinguish between TSGs and OGs (Fig. 2D).
Identifying OGs and TSGs
Having identified these predictive parameters, we set out to predict the probability of a given gene being a TSG or OG. To do this, we developed a method we call Tumor Suppressor and Oncogene Explorer (TUSON Explorer) that combined the selected parameters to derive an overall significance and ranking for each gene. First, we derived a p-value (and q-value) for each gene for the ratios of LOF/Benign, Splicing/Benign, HiFI/Benign and missense Entropy based on the comparison to the Neutral gene set (see methods). For the LOF/Benign parameter, we applied a correction to normalize for the non-uniform codon usage among genes for the occurrence of nonsense mutations (see methods). Finally, we used an extension of Liptak’s method to provide a combined p-value for the parameters for each gene. TUSON Explorer does not take into account SCNA information and this allows us to perform a rigorous analysis of our cancer driver genes for their abilities to predict the frequency of deletion and amplification (see below).
For TSGs, the combined p and q values were derived from individual values from the LOF/Benign, Splicing/Benign and HiFI/Benign ratios. For OGs, the combined values were derived from the Missense Entropy and the HiFI/Benign ratio. The LOF/Benign parameter for discrimination between TSGs and OGs was subsequently utilized to define a final list of OGs (see methods). As a second strategy to predict the probability of a given gene being a TSG or OG, we employed the Lasso model, which that also takes into account SCNAs (see Supp. Methods and Tables 4 & 5). The ranked lists of predicted TSGs and OGs by both Lasso and TUSON Explorer are contained in Supp. Table 3a and 3b. This list provides a facile look-up table that can be easily sorted for different parameters for all those who are interested in the mutational behavior of a given gene in this dataset.
Both ranking strategies performed similarly and eliminated the errors of inappropriately including giant genes and genes in highly mutable regions as found in MuSIC (Dees et al., 2012) without the need to consider expression level or replication timing as in MutSigCV (Lawrence et al., 2013). Importantly, both of our strategies distinguish between TSGs and OGs, which are predicted to have functionally opposite roles in the control of cell growth and have different implications for potential cancer therapeutics.
Estimates of the numbers of TSG and OG
Every ranking of this nature is a mixture of truly significant genes and false positive genes that are present due to the overlap with the distribution of the null hypothesis values. Thus, we sought to get a minimal estimate of the number of predicted TSGs and OGs from the analysis of the distribution of the combined p-value for the prediction of each class of cancer driver genes. To achieve this, we utilized a histogram-based method (Mosig et al., 2001) to estimate the number of rejected hypotheses from the distributions of the combined p-values calculated for each gene. With our dataset, this method estimated approximately ~320 TSGs and ~250 OGs. Calculations obtained from the p-values of individual or combined parameters give very similar numbers. This long list of TSGs and OGs suggest that there are many more drivers than anticipated and that they exist in a continuum of decreasing probabilities (See Discussion, Fig. 7A). For the analyses described below we considered the top 300 TSGs genes (FDR <0.18) and 250 OGs (FDR <0.22) as our working lists. Given the fact that the deviation of the mutation signatures from the normal pattern is a function of the degree of selection and the frequency of mutation, increasing the number of tumor samples will detect even more cancer drivers of progressively weaker selective pressure. To determine the potential TSG number upon additional sequencing we applied TUSON and estimated the number of TSGs (Mosig’s method) on random subsets of the dataset with increasing numbers of mutations and observed that the number of statistically significant TSGs continue to increase with additional samples. We observe a slight slowing in TSG increase at the highest number of mutations examined (Supp. Fig. 2) possibly indicating a future plateau.
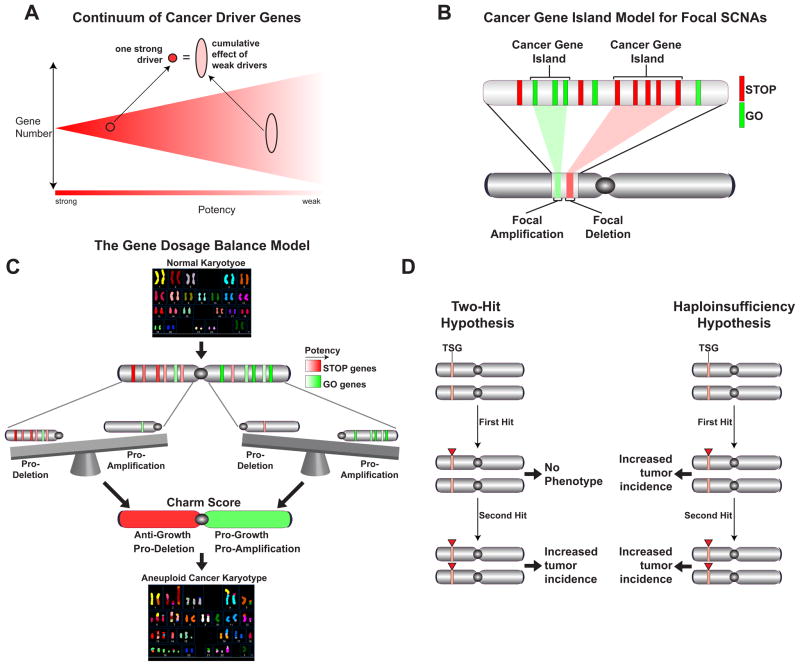
Illustrative schematics of different concepts highlighted in the Discussion. A) The phenotypic continuum of cancer driver. B) The cancer gene island model for focal SCNAs. C) The cumulative gene dosage balance model for predicting the patterns of aneuploidy. D) Comparison of the predictions of Knudson’s Two-hit Hypothesis for TSGs compared the Haploinsufficiency Hypothesis presented in this study.
PAN-Cancer mutational analysis
Gene Ontology (GO) term and pathway analysis of our list of potential TSGs showed enrichment for functions highly relevant to tumorigenesis including cell cycle control, embryonic development, promotion of differentiation, apoptosis and blood vessel development (Supp. Table 3c, Fig. 3). In addition, there was a strong enrichment for transcription regulation (q-value= 6.19 × 10−11) and chromatin modification (q-value= 5.7 × 10−12). Furthermore, we noticed an enrichment for genes involved in the immune system (q-value= 5.8 × 10−3), antigen processing and presentation represented by the MHC class I system. Two major HLA genes (HLA-A and HLA-B) were in the top 90 candidate TSGs (q-value<0.0002) and the β2 microglobulin (B2M) gene, which is an obligatory complex component of both HLA proteins, ranked 43th (q-value= 9.2 × 10−9) on our TSG list underscoring that escaping from immune-surveillance is a significant selective force in tumorigenesis (Hanahan and Weinberg, 2011) (Figs. 3, ,4).4). Furthermore, IL32, which stimulates the immune responses of NK cells and CD8+ T cells that monitor MHC status (Conti et al., 2007), is also in the top 50 TSGs. Unexpectedly, negative regulation of cell adhesion (q-value= 4.32 × 10−4) was enriched, indicating that increase of cell adhesion may confer a selective advantage to tumor cells. Traditionally it has been thought that reducing adhesion promotes tumorigenesis, however, recent findings suggest a potentially different role for cell-to-cell-adhesion. First, it has been shown that circulating tumor cells exist in clusters in the blood (Hou et al., 2011). Secondly, PVRL4, which ranked well in our Lasso OG list, was shown to promote transformation through cell adhesion, as do several other oncogenes like MYC overproduction, activated KRAS and PI3K and loss of PTEN (Pavlova et al., 2013). Thus, promotion of adhesion may be a driving force in tumorigenesis.
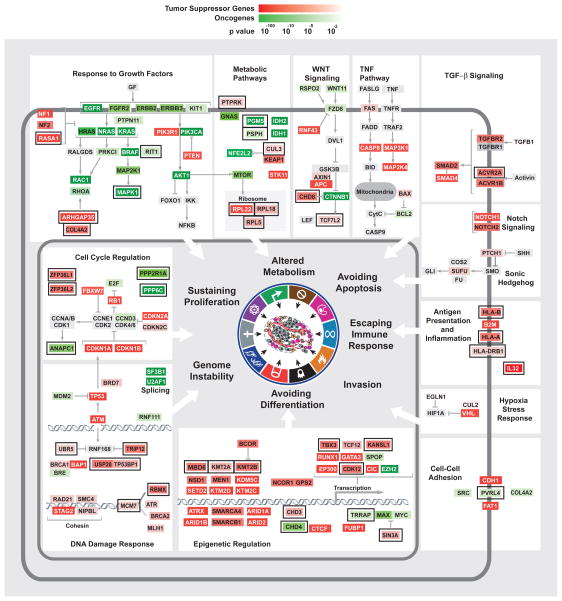
Placement of predicted cancer drivers within specific cellular pathways. TSGs and OGs were predicted by TUSON Explorer and ranked by their associated combined q values. The predicted TSGs and OGs belonging to many known cellular pathways or complexes are shown and how they generally correspond to the hallmarks of cancer. TSGs are shown in red while OGs are shown in green and the color intensity is proportional to the combined q value as indicated. For some pathways, additional genes absent from the predicted TSGs and OGs were added and marked in grey for clarity of the pathway representation. While several genes are known to affect multiple pathways and hallmarks, only one function is presented for the sake of limiting the complexity of the diagram. An external black box outside the colored gene box highlights genes previously less well characterized for their roles in tumorigenesis. Also see Supp. Fig. 3 and Supp. Table 3.
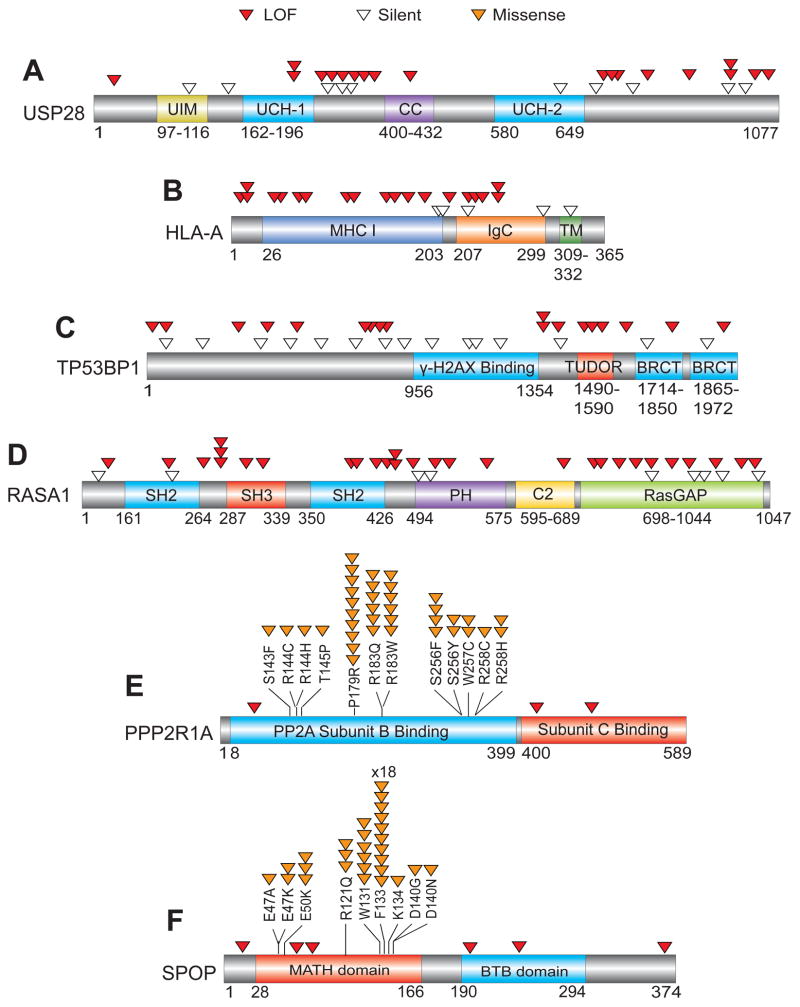
The mutational pattern of selected TSGs and OGs are depicted. For TSGs (A–D), the locations of LOF (red) and Silent (white) mutations within the coding regions are shown. For OGs (E–F), the location of recurrent Missense (orange) and LOF (red) mutations within the coding regions is shown. USP28, TP53BP1 and RASA1 are previously less well-characterized candidate TSGs in the TUSON PAN-Cancer analysis. SPOP and PPP2R1A are previously less-well characterized candidate OGs. Also see Supp. Table 3.
New potential cancer drivers
New components of pathways previously linked to tumorigenesis have also been detected. For example, the DNA damage response pathway is central to the maintenance of genomic stability and both members of a key DDR complex, the TP53BP1/USP28 complex (Zhang et al., 2006), which are substrates of the ATM kinase (Matsuoka et al., 2007), were identified within the top 110 TSGs (q-value <0.15, Figs. 3, ,4).4). Two components that regulate ATM-dependent chromatin remodeling, UBR5 and TRIP12 (Gudjonsson et al., 2012) are also high on the TSG list (q-value <0.25). RBMX, which controls ATR and BRCA2 expression (Adamson et al., 2012), ranked 76th on the list (q-value= 1.1 × 10−4, Fig. 4). There are several candidate OGs with enzymatic functions that could serve as drug targets (Supp. Tables 3b and 7b) including three phosphatases (PPP6C, PTPN11, PTPRF) and regulators such as PPP2R1A, as well as several kinases MAPK1, MAPK8, BRSK1 among others. There are many other new potential TSGs and OGs on these lists that cannot be discussed here due to limitations of space but several of these are presented in Fig. 3.
Consistent with the enrichment of cell cycle and apoptosis GO terms, integration of the PAN-Cancer analysis with functional gene sets revealed that essential genes are significantly depleted for deleterious mutations (see below). An exception to that finding was the presence of RPL22, RPL5 and RPL18 large ribosomal subunit genes in the top 210 TSGs (q-value < 0.07, Fig. 3). Heterozygous mutations in ribosomal genes promote tumorigenesis in zebrafish (Lai et al., 2009). Familial mutations in ribosomal proteins have been associated with Diamond-Blackfan anemia which is associated with an increased risk of leukemia (Willig et al., 2000).
Analysis of individual tumor types
Identification of cancer drivers using the PAN-Cancer analysis favors discovery of genes whose functions contribute to many different types of cancer. Certain cancer drivers may miss the cutoff for significance in the PAN-Cancer analysis because they are primarily involved in controlling tissue-specific differentiation networks or because they are rate-limiting for a particular function in only certain tissues. Thus, we anticipate that new drivers can be discovered through analysis of mutation signatures in individual tumor types despite their lower numbers. We performed the same analysis as above for each of 20 tumor types (Supp. Table 1 and 4a, b). This analysis found many TSGs that are specific for one tissue type such CDH1 and GATA3 in breast adenocarcinoma, VHL and PBMR1 in kidney clear renal cell carcinoma and ID3 and NPM1 in hematological malignances (Supp. Table 4a). Genes whose FDRs for the different subtypes were below 0.25 were all already relatively highly ranked already in the PAN-Cancer analysis. This indicates that the majority of tissue specific drivers were detected in the PAN-Cancer analysis (TUSON).
We wanted to determine how many new TSGs might be expected from the analysis of a new cancer subtype. For this we calculated the number of TSGs in the whole dataset lacking an individual tumor type (Supp. Table 4c) and compared this list to the TSGs in that tumor type which averaged 14 genes. We found that the average new cancer type added about 5 TSGs to the PAN-Cancer list. Thus, on average ~70% of the TSGs detected in a single tumor type were already detected in the PAN-Cancer analysis performed after excluding the mutations in that type of tumor. This suggests that that most cancer genes selected during tumor evolution act in cellular pathways whose role in tumorigenesis is widespread among different tumor types.
Analysis of TSGs and OGs
Behavior of functional gene sets
The PAN-Cancer mutation dataset allows us to interrogate the behavior of functional gene sets derived through experimental approaches. We previously showed that STOP genes are over-represented in regions of deletion (Solimini et al., 2012). Examination of their abundance in the set of candidate TSGs showed that STOP genes are significantly enriched in the TSG set (p= 0.0031, Fisher’s Exact Test) comprising ~10% of the top 300 TSGs (68% more than expected). The STOP gene set showed a 21% higher ratio of LOF/Silent than the average for the neutral gene set, (p= 2.0 × 10−15 Wilcoxon test: Fig. 5A). Furthermore, the STOP genes showed a significant increase in the Splicing/Benign and HiFI/Benign ratios, two of the most potent parameters for the prediction of TSGs (Fig. 5A). This analysis further underscores the fundamental connection between cell proliferation and cancer.
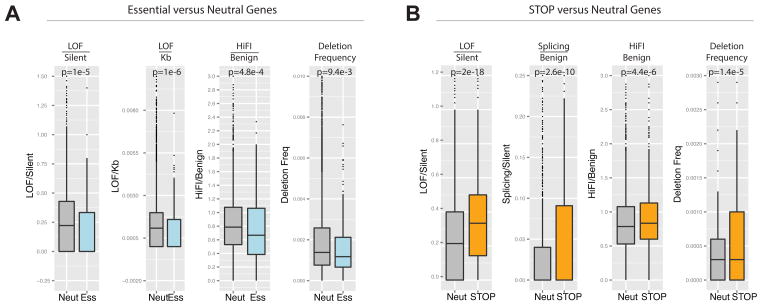
Panels A–B contain a boxplot representation of the distribution of the values for the indicated parameters in the Neutral genes compared with the Essential genes and STOP genes. The median, first quartile third quartile and outliers in the distribution are shown. The p-value for the difference in the two indicated distributions is shown as derived by the Wilcoxon test.
A) Boxplots showing the distribution of LOF/Silent, LOF/Kb, HiFI missense/Benign ratios and the high frequency of focal deletion among the Neutral gene set and the Essential genes.
B) Boxplots showing the distribution of LOF/Silent, Splicing/Benign, HiFI missense/Benign, ratios and the high frequency of focal deletion among the Neutral gene and STOP gene sets.
Also see Supp. Table 5.
We next investigated a high confidence set of 145 genes predicted to be essential at the cellular level based on their housekeeping cellular functions and their high evolutionary conservation (Supp. Table 5a and see methods). This set was depleted from regions of recurring deletions (Beroukhim et al., 2010) by 45% (p= 0.0198, Fisher’s Exact Test) and a larger set of 332 essential genes were depleted by 25% (p= 0.014). Examination of the LOF/Silent ratio showed that for this set of 332, the frequency of LOF/silent was 27% lower than the rate for the neutral gene set (p= 1 × 10−5). Additionally, the LOF/kb and HiFI/Benign ratios were also significantly decreased in the essential gene set. Given that the mutations and deletions in question are heterozygous, the reduced LOF mutation and deletion frequency of the essential genes as a group argues that between 27% and 45% are haploinsufficient. Interestingly, our TSGs were enriched in recurring focal deletions (68%, p= 0.000281) and depleted from recurring amplifications (28%, p= 0.015), while the OGs were enriched in amplifications (25%, p= 0.046) and depleted from focal deletions (23%).
General Properties of Cancer Drivers
High interactivity
To search for unique properties of TSGs and OGs we examined the degree to which these drivers participate in protein complexes using an experimentally validated set of human protein complexes from the CORUM database (Ruepp et al., 2010). We found that both TSGs and OGs were significantly more likely to be in protein complexes than a typical protein. The 13.4% of all proteins found in CORUM are in a complex. However, 36.7% of the predicted TSGs were in complexes (p= 3.1 × 10−24) and 26.4% of the predicted OGs were in complexes (p= 3.5 × 10−8, Supp. Fig. 3A).
High Betweenness
A second property of complexes is the degree to which they are connected to other proteins and complexes. We explored this by assessing a property called “betweenness” which is proportional to the number of times the protein is part of the shortest paths between all pairs of proteins in a network. High betweenness indicates a greater connectivity. The TSG and OG candidate gene lists were mapped onto the most current BioGRID human protein-protein interaction network (Stark et al., 2006). Both the predicted TSGs and OGs show a high degree of betweenness (TSG p= 6.16 × 10−32, OG p = 1.68 × 10−6, Supp. Fig. 3B), indicating they are optimally positioned to impact information flow through networks.
Greater Length
Proteins with greater interactivity often have more domains. Thus, we examined gene length. Cancer drivers are significantly longer than the average gene (1700 nt) with the mean for TSGs at 3234 nt (p= 2 × 10−21) and OGs at 2107 nt (p=9.7 × 10−6). Importantly, this observation is also characteristic of the genes our training sets (TSGs, 4133 nt, p=6.7 × 10−10; OGs, 2260 nt, p= 0.0016) (see Discussion).
An unusually high concentration of TSGs on the X chromosome
While examining the distribution of TSGs across chromosomes, we found that the X is unusually enriched for TSGs (p-value = 0.004181, exact binomial test) relative to autosomes. Examining the top 300 TSGs, we find that while only 3.9% of all genes are on the X, it contains 7.3% of all predicted TSGs, 86% more than expected and was the only chromosome with a significant enrichment of TSGs (Supp. Table 5b). Given the fact that the X is functionally haploid both in males and females, this observation has certain implications for evolutionary selection of cancer drivers during tumorigenesis and haploinsufficiency of TSGs (see discussion).
Interestingly, in the top 400 TSGs we found two potential TSGs on the Y, ZFY and UTY (q-value<0.22). Both have homologs on the X that escape X-inactivation, each of which also display tumor suppressor properties: ZFX (p= 0.019) and UTX/KDM6A (p= 3.3 × 10−46). This could explain the observation that frequent Y nullisomy is observed in prostate, renal cell, head and neck, Barrret’s esophagus adenocarcinoma, bladder, pancreatic adenocarcinoma and other cancers at frequencies of 30–80% (Bianchi, 2009; Mitelman et al, 2007).
Furthermore, we analyzed the silent mutation rates along entire chromosomes and found an enhanced mutation rate on the X chromosome relative to autosomes in males (30% increase, p = 1.1 × 10−9). This is even greater in females (77.5%, p =1.6 × 10−11) (Supp. Table 5c). Possible explanations for this phenomenon are detailed in the discussion.
Distribution and potency of cancer drivers on chromosomes predicts arm and chromosome SCNA frequencies
In addition to focal SCNAs, a less frequent but significant chromosomal alteration is whole arm loss or gain. We hypothesized that the distribution and potency of TSGs and OGs on chromosomes might explain the average frequency of chromosomal whole arm SCNAs seen in cancer. To this end, we generated a chromosome arm score, Charm, which provides an assessment of each arm based on the density of TSGs and OGs and their potency (weights of TSGs and OGs are based on their rank on their respective lists and serve as a metric for their potency). The Charm score represents a measure of the amount of positive or negative growth and survival potential that wild-type OGs or TSGs might normally impart to a given arm and therefore how SCNAs might impact cancer evolution by altering this balance during tumorigenesis. Importantly, for Charm calculations we employed the parameters from TUSON Explorer, which does not include copy number information. To lessen the diluting impact of false positives for this analysis, we applied stringency cutoffs of an FDR of 0.25 for TSGs and 0.35 for OGs, and a minimum of 10 missense mutations for OGs and 8 LOF mutations for TSGs to get a tighter list of 264 TSGs and 219 OGs (see methods). The analysis of the CharmTSG score versus frequency of chromosomal arm deletion revealed a strong positive correlation (r=0.578 p-value = 5.8 × 10−5, Pearson correlation, Fig. 6A, Supp. Table 6). Interestingly, the CharmTSG score also showed a strong negative correlation with arm amplification frequency, thus a high CharmTSG score indicates a significantly reduced tendency to be amplified (r=−0.59, p = 2.8 × 10−5, Fig. 6B). Simple TSG densities without weighting by rank also showed correlations with arm deletions (Supp. Fig. 4A), but these correlations are improved by Charm. In contrast to CharmTSG, the CharmOG score showed a negative correlation with arm deletion frequency (r=0.52, p-value = 3.2 × 10−4, Fig. 6C). Moreover the density of OGs weakly positively correlated with arm amplification frequency (r=0.45, p-value = 1.8 × 10−3, Fig. 6D) but was not improved by the Charm score (not shown).
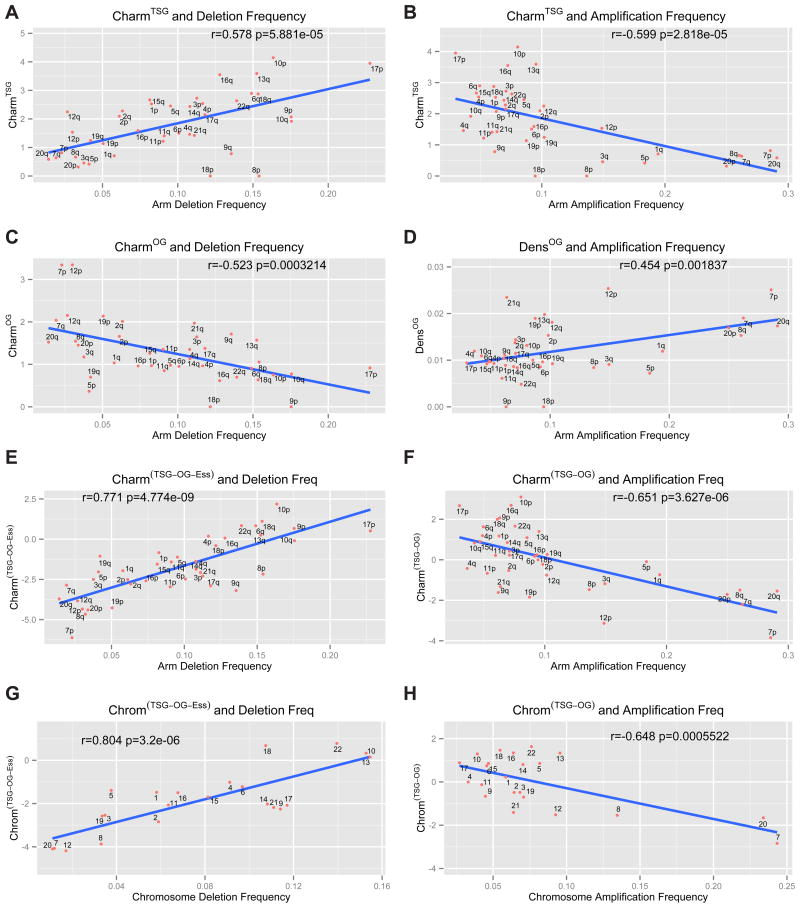
The correlation analysis (Pearson’s correlation) of the Charm scores for TSGs (CharmTSG, A–B), OGs (CharmOG, C), essential genes (CharmEss, D) and combinations of these classes (CharmTSG-OG-Ess and CharmTSG-OG, E–F) and the corresponding Chrom scores (ChromTSG-OG and ChromTSG-OG-Ess, G–H) in relationship to the arm- or chromosome-level deletion or amplification frequency. The Charm scores refer to a weighted density of TSGs, OGs or essential genes present on each chromosome arm, where each gene is weighted based on its rank position within the list of predicted TSGs and OGs ranked by TUSON Explorer. The Chrom score is the equivalent of the Charm score for whole chromosome SCNAs. Also see Supp. Figs. 4&5 and Supp. Table 6.
Like GO genes in focal deletions, we reasoned that the chromosome arms most frequently deleted in cancer would be depleted of genes that promote the fitness of cancer cells. Using our in silico list of essential genes, we estimated their fitness potency by estimating their avoidance of damaging mutations using the (LOF + HiFI)/Benign ratios. By determining a CharmEss score for each arm, we found a negative correlation between CharmEss scores and the frequency of arm-level deletions (r= 0.34, p= 1.6 × 10−2, Supp. Fig. 4D). No correlation was found between CharmEss and amplification frequency, as expected.
Since the CharmTSG, CharmOG and CharmEss scores correlate with arm-level deletion, we combined them by giving a positive weight to the CharmTSG score and a negative weight to the CharmOG and CharmEss scores to derived a cumulative CharmTSG-OG-Ess score. The CharmTSG-OG-Ess score gave an even stronger positive correlation with arm deletion frequency (r = 0.77; p-value = 4.7 × 10−9, Fig. 6E, Supp. Table 6). For amplification, we used the CharmTSG-OG score and found a strong negative correlation with amplification frequency (r = 0.65; p-value = 3.6 × 10−6, Fig. 6F). We also combined amplification and deletion frequencies into a single score for copy number variation on each arm and compared that to the CharmTSG-OG score. This also gave a strong significant correlation (r = 0.74, p = 2.7 × 10−8, Supp. Fig. 5A).
We extended our analysis of cancer driver scores and SCNAs to whole chromosome aneuploidy using its Charm equivalent score that we call Chrom (Fig. 6G–H, Supp. Fig. 4E–H, Supp. Fig. 5B, E–F). ChromTSG significantly correlated with chromosome deletion frequency (r = 0.66, p = 3.7 × 10−4, Supp. Fig. 4E) and anticorrelated with amplification frequency (r = 0.54, p = 4.0 × 10−3, Supp. Fig. 4F). Impressively, when we combined all three classes, TSGs, OGs and essential genes, the ChromTSG-OG-Ess was strongly predictive of the frequency of chromosome loss (r = 0.80, p = 3.2 × 10−6, Fig. 6G), and ChromTSG-OG was predictive of chromosome gains (r = 0.64, p = 5.5 × 10−4, Fig. 6H). Very similar results were obtained using just the TUSON ranking without stringency cutoffs (Supp. Fig. 6C–F).
Together these data strongly argue that a selective force in generating chromosomal arm and whole chromosome SCNAs derives from the integration of the relative densities and potencies of positively and negatively acting cancer drivers on a particular chromosome. Thus, the SCNAs in cancer genomes may be selected during tumor evolution through cumulative haploinsufficiency for deletions, as previously proposed for STOP genes in focal deletions (Solimini et al., 2012), as well as cumulative triplosensitivity for amplifications (see Discussion).
Discussion
In this study we analyzed the mutational data from more than 8,700 sporadic cancers to predict cancer driver genes. We determined the most predictive parameters for identifying TSGs and OGs and used them to develop an algorithm called TUSON Explorer to predict the probability that an individual gene functions as a TSG or an OG in cancer. This unbiased approach demonstrated that the probability of being a cancer driver can be assessed by the significance of the distortion of its mutational pattern from the pattern expected for a “neutral” gene. Combining data from our analyses of drivers and copy number changes, the average tumor in our dataset has a mean number of ~1 OG mutation, ~3 TSG mutations (LOF and damaging missense), ~3 chromosomal arm gains, ~5 chromosomal arm losses, ~2 whole chromosome gain, ~2 whole chromosome loss, ~12 focal deletions and ~11 focal amplifications (Zack et al., 2013). Thus SCNAs comprise a very large proportion of cancer driving events.
A continuum of cancer driver genes
A central conclusion from this study is that there are likely to be many more cancer drivers than anticipated. Our estimate of the number of TSGs based either on the combined significance of the different parameters or on the single best parameter for the prediction of TSGs, i.e. the LOF/Benign ratio, predicted ~320 TSGs with the current database from 8200 tumors. Likewise, we also predict more OGs than anticipated. The view of the cancer landscape emerging from our analysis does not contain a clear cut off for predicting cancer drivers. Instead there exists a continuum of decreasing probability of a given gene being a driver (either TSG or OG). This probability is revealed by the degree of selection the gene experiences during tumor evolution, which should be proportional to the phenotypic effect caused by its loss or gain. This continuum of decreasing potency of potential cancer drivers is likely to correspond to a continuum of increasing numbers of genes with decreasing phenotypic severity as illustrated schematically in Fig. 7A. In addition, we hypothesize that events that simultaneously affect multiple weak drivers can cumulatively have an effect equal to a single potent driver. Our modeling of the progressively higher number of driver genes identified as increasing numbers of tumors are analyzed suggests that this number will continue to climb as more sequence information becomes available but may be beginning to plateau. However, the newly identified drivers are likely to display progressively less potency with lower therapeutic significance. This is analogous to GWAS studies for which increasing sample sizes allow the identification of progressively weaker acting variants.
Our analysis provides a probability of each gene being a cancer driver and, as such, there will be false positives regardless of the threshold of minimum probability we employ. Identifying bona fide drivers from the regions with significant p-values but higher FDR values, i.e. weaker phenotypic signatures, can be aided by considering other information such as their involvement in SCNAs, biochemical connections to known OGs and TSGs, and functional information gleaned from the literature. These heuristic methods can be used to increase confidence and rescue genes onto the likely cancer driver list (Supp. Table 7a,b).
PAN-Cancer and tissue-specific analysis
Analysis of individual tumors types identified distinct sets of drivers in each tumor type, but the majority of these were also identified in the PAN-Cancer analysis as lower confidence candidates (Supp. Table 4a-c). Thus while there is clearly tissue specificity, there is still significant overlap among different tumor types and a PAN-Cancer analysis samples a sufficient number of similar tumors to detect most of the largely tissue-specific or tissue-biased cancer drivers. Our analysis suggests that significantly deeper sequencing of individual tumor types is unlikely to uncover many new potent drivers beyond what we have already identified and further sequencing is likely to suffer from diminishing returns. This view is consistent with a recent review that argues that nearly all potent drivers have been identified (Vogelstein et al., 2013). Sequencing of more rare, relatively unexplored cancer types may identify a few novel potent drivers that are specific to those tumor types, but the vast majority of potent drivers will already have been seen in other cancers. The major effects of continued sequencing will likely be to solidify the continuum by bringing much weaker drivers into the realm of statistical significance.
Properties of new potential cancer driver genes
Analysis of the lists enriched for cancer drivers revealed several general properties that distinguish these genes from non-driver genes. The cancer gene driver list of both TSGs and OGs is strongly enriched both for residence in protein complexes and for a property known as betweenness which is a measure of the degree to which a set of genes is enriched for hubs within an interaction network. Thus, the driver genes are much more highly connected than the average protein in the human gene network. Highly connected nodes are better positioned to control the flow of information, and their removal or hyperactivation will have the highest impact across a network due to their centrality. In addition, we find that both TSGs and OGs are significantly longer than the average gene. This property is likely to aid their ability to associate with multiple proteins in their roles in integrating growth and survival information. The inter-relatedness of TSGs and OGs is further illustrated in Fig. 3 where sets of genes are shown in the context of their known pathways and their effects on the hallmarks of cancer.
Unexpected properties of the X chromosome
Given the potentially deleterious effects of mutating TSGs, we anticipated that TSGs would be depleted from the X chromosome by natural selection since it is haploid in males and is functionally haploid in females due to dosage compensation. However, our analysis revealed just the opposite, namely that the X has 85% more TSGs than expected. Oncogenes on the other hand are not over-represented on the X. The likely explanation is that a deleterious mutation in a TSG on the X is more penetrant because there is not a WT copy to compensate for its loss. This further suggests that natural selection has not completely depleted TSGs from the X, possibly because cancer is largely a post-reproductive disease.
We found a higher mutation rate for the X than for autosomes, and this is further exaggerated in females. In females, the additional increase in X mutability is likely to be due to the presence of the inactive X which has very little transcription and hence less transcription coupled repair and is enriched in late replicating heterochromatin, which tends to be more mutagenic (Stamatoyannopoulos et al., 2009). The mechanism underlying these differences and their biological significance remains to be determined. However, these differences might indicate that the mutation rates of whole chromosomes are set by evolution and that the higher mutability of the X is advantageous over evolutionary time if it also occurs in the germline.
Haploinsufficiency and cancer
The clonal expansion theory of tumorigenesis argues that in order for an individual mutation to be selected, it must cause an expansion of the clone derived from that mutant cell by increasing relative proliferation and survival (Vogelstein and Kinzler, 1993). This is intuitive for OGs as they are dominant, but less so for TSGs. For a hemizygous mutation in a TSG to be selected in cancer, we have to assume that either the mutation is dominant negative or that the TSG is haploinsufficient. Our current analysis of the degree to which essential genes are absent from hemizygous recurring focal deletions coupled with the reduced frequency with which essential genes experience LOF mutations in tumors conservatively suggests ~30% haploinsufficiency overall among human genes (See Supp. Experimental Procedures). A recent analysis of haploinsufficiency by the mouse knockout consortium (White et al., 2013) found that 42% of genes examined produced a phenotype when heterozygous, similar to our estimates. Evidence suggesting our sporadic TSG list is largely haploinsufficient comes from a comparison of the enrichment in focal deletions of STOP genes versus our sporadic TSGs. STOP genes, which are TSG-like, are enriched by 20%. If we assume that only 30% of this gene set is haploinsufficient and all of the selective enrichment comes from haploinsufficiency genes, then a list of purely haploinsufficient STOP genes would be expected to be enriched by 67%. Perhaps coincidentally, our list of TSGs is enriched 68% in recurring focal deletions suggesting that a significant proportion, and possibly all, of sporadic TSGs are haploinsufficient.
We propose that two classes of TSGs might exist, ones that are haploinsufficient and contribute to sporadic cancer, and ones that are haplosufficient and do not significantly contribute to sporadic cancer through mutation. Circumstances under which organisms inherit only one functional copy of those haplosufficient TSGs might result in cancer since loss of the second allele would produce a selectable phenotype. This situation occurs with familial TSGs and the classic two-hit hypothesis of tumorigenesis. This hypothesis is consistent with the fact that out of a list of 73 familial TSGs culled from the literature, only 32% of them had a combined q-value<0.25 in the PAN-Cancer analysis (Supp. Table 3d). Another circumstance with only one functional allele per cell occurs on the X where we see a ~86% higher density of TSGs than on the autosomes (p= 0.015). If the predicted rate of ~30% haploinsufficiency is correct, then one might expect a ~200% increase over autosomes, but negative selective pressure on the X could have reduced that number. Thus, it is possible that there are actually similar densities of TSGs on the X and autosomes (haploinsufficient sporadic TSGs, and haplosufficient potential TSGs), but those on the X realize their tumorigenic potential at a higher rate than those on the autosomes.
The PAN-Cancer mutational analysis predicts aneuploidy in cancer
Aneuploidy is a hallmark of cancer and can have both advantageous and deleterious consequences for cells (Tang et al. 2013, Luo et al. 2009) but there is no general theory explaining how patterns of aneuploidy emerge. Knowing the identity and potential potency of cancer drivers has allowed us to uncover a driving force behind selection of arm- and chromosome-level SCNAs. Our analysis using Charm as an integrated assessment of the density and potency of the different classes of cancer driver genes on chromosomes displayed a robust ability to predict the patterns of whole arm amplifications and deletions and aneuploidy. The fact that the Charm score improves the correlations with SCNAs compared to the simple gene density of the different classes of genes indicates that the ranking of driver genes by TUSON Explorer is likely to represent an accurate estimate of the potency of their phenotypic effect in cancer and further supports the continuum theory.
DensOG and CharmOG do not predict arm amplification as well as CharmTSG. This reduced predictive potential is likely to be because the OGs were selected on the basis of the ability to be activated by mutation and because simply increasing the dosage by 50% might not strongly impact the networks they control. CharmOG, however, does show a strong negative correlation with arm deletion frequency, indicating that normally the WT OGs are acting to promote proliferation and survival and the cumulative reduction of their levels by 50% is deleterious. In this respect the OGs are behaving like the essential genes with respect to focal deletions and the inclusion of a high confidence list of 332 essential genes (Supp. Table 5a) together with OGs further improves the predictive ability for whole arm SCNA (Supp. Fig. 4A, B). As expected, the essential genes have no predictive power for amplifications.
CharmTSG strongly predicts whole arm deletions. Unexpectedly, it also strongly predicts chromosomal amplification, providing a strong negative correlation. This suggests that increasing the gene dosage of a group of TSGs can have deleterious effects on tumorigenesis through the process of cumulative triplosensitivity. If TSGs are truly haploinsufficient, their WT protein levels may be only marginally sufficient to execute their roles. If so, TSGs may well be more sensitive to increased gene dosage to further enhance their pathways than typical genes. In other words, haploinsufficient genes may be more likely to display triplosensitivity. This property of sporadic TSGs being both haploinsufficient and triplosensitive, therefore, may make their cumulative Charm score an even better parameter to explain SCNAs of chromosome arms and aneuploidy in general. Developing a combined CharmTSG-OG-Ess and ChromTSG-OG-Ess score can now predict ~80% of the frequency of arm and chromosome loss and ~65% of the amplifications observed across all cancers.
While the correlation between Charm/Chrom scores and SCNAs is striking, there are several areas for improvement. The first area concerns our lack of knowledge of the full complement of essential genes and which of these are haploinsufficient. Secondly, only a subset of OGs will be dosage sensitive and this knowledge would improve the correlation. In addition, there are two classes of OGs. Class I contains classical oncogenes such as KRAS that are activated by mutation but whose WT copies are not necessarily activated by overexpression and will not be predictive of amplification. Class II OGs contains genes such as cyclin D that can be activated by overexpression but are difficult to activate by missense mutations and thus lack a mutational signature. Class II OGs cannot be identified with confidence through mutational signatures yet are likely to display triplosensitivity and would positively correlate with amplification. Third, some TSGs can be difficult to distinguish from OGs. These are TGSs that have low haploinsufficiency but can produce a selectable phenotype by generation of dominant negative alleles that have low entropy. Such genes will lack a strong LOF signature, but will show a significant number of deleterious missense mutations, which are likely to predominantly occur in one or a few crucial residues, thus conferring a significant entropy score. In addition, early SCNA events might influence subsequent events as is the case when specific aneuploidy co-occurs (Ozery-Flato, et al 2011), which would confound our analysis to some degree. Finally, refining these lists of cancer drivers will only improve their predictive power. The current programs for prediction have their strengths and weaknesses, and are likely to be further improved in the future. More precise knowledge of these essential and cancer driver genes should significantly improve SCNA predictability and our understanding of the cancer genome. Finally, the SCNA frequencies might vary according to tumor type, thus comparison of datasets within one tumor type might provide more predictive power. In addition, we do not know the background frequency of SCNAs upon which selection acts, so the observed SCNA frequency cannot be normalized like mutation rates can and, therefore, the observed SCNA frequency detected might reflect both frequency of the event and its selective power, which could confound the correlation.
Models of Cancer Evolution
Our work suggests a very important role for cumulative haploinsufficiency and triplosensitivity operating during cancer evolution to drive tumorigenesis. In each genomic region there are STOP (TSG) and GO (OG and essential) genes that will exert a negative or positive phenotypic effect on tumorigenesis. Both for focal deletions as illustrated by the Cancer Gene Island Model (Fig. 7B) and for chromosomes and chromosomal arms SCNAs as indicated by the Charm and Chrom analysis (Fig. 7C), the integrated cumulative balance of these positive and negative tumorigenic effects of individual genes affected in each SCNA event provides the selective potency to that event and can predict its frequency across cancers.
For the past 40 years, the tumor suppressor field has been guided by Knudson’s classical Two-Hit Hypothesis of tumorigenesis for familial cancers. While there are certainly bona fide examples of the Two-Hit Model in sporadic cancer, this model conflicts with the theory of clonal evolution of sporadic cancer in the assumption that the first hit is fully recessive and a second hit is required to contribute to tumorigenesis. While it is difficult to measure the frequency with which the Two-Hit Hypothesis operates in cancers because the role and extent of methylation inactivation is not yet known in each tumor, analysis of LOF mutational events suggests that it is likely to be a relatively infrequent event except in the case of a few genes such as TP53 (p53) and CDKN2A (p16), both of which are inducible responders to oncogenic stress which can increase during tumorigenesis. In the cases of sporadic cancer where the two-hit hypothesis does operate, it is still possible, and even probable, that the genes involved are haploinsufficient to begin with. Our results have led us to propose that the vast majority, if not all, of sporadic TSGs are likely to be haploinsufficient and that therefore sporadic TSGs are most likely to operate through the Haploinsufficiency Model shown in Figure 7D. It is important to note that these hypotheses are not mutually exclusive as loss of the second allele of a haploinsufficient TSG, the second hit, will undoubtedly provide a stronger selective pressure than the first hit. However, a tumor has multiple paths through which to evolve and it may not require loss of that second allele as it obtains growth-promoting power through the accumulation of other events.
The analysis built on the theories presented herein has allowed us to demonstrate that the distribution and the potency of TSGs, OGs and essential genes on chromosomes can be used to predict and explain the complex patterns of aneuploidy and copy number variation characteristic of cancer genomes. We propose that these selective forces shape the cancer genome through the processes of cumulative haploinsufficiency and triplosensitivity.
Experimental Procedures
Somatic mutation dataset
The dataset of somatic mutations included data from The Cancer Genome Atlas (TCGA, http://cancergenome.nih.gov/) research network, from the Catalogue of somatic mutations in cancer (COSMIC, http://cancer.sanger.ac.uk/cancergenome/projects/cosmic/) and the dataset published by Alexandrov et al., Nature 2013 (Alexandrov et al., Nature 2013). The dataset contained ~1,200,000 mutations from 8207 tumor samples from more than 20 tumor types (Supp. Table 1) and will be available at http://elledgelab.med.harvard.edu/. All data related to SCNAs were derived from the TCGA Genome Data Analysis Center at the Broad Institute (Zach et al., 2013).
TUSON Explorer predictions
The PolyPhen2 algorithm (Adzhubei et al., 2010) was used to predict the functional impact of each missense mutation and to classify them as high functional impact (HiFI) or low functional impact (LoFI). We defined the four following classes of mutations: 1) Benign mutations: Silent + LoFI Missense; 2) Loss of Function mutations (LOF): Nonsense and Frameshift mutations; 3) Splicing mutations: mutations affecting splicing sites; and 4) HiFI missense mutations. An additional parameter considered was the Entropy score, which measures the degree of randomness of the distribution of missense mutations.
Among 22 potential parameters, we selected the most predictive ones by using Lasso prediction model and three training sets of known TSGs and OGs (from the Cancer Gene Census, Futreal et al., 2004) and putative Neutral genes. LOF/Benign, Splicing/Benign and HiFI/Benign ratios were selected by Lasso for the prediction of TSGs, while HiFI/Benign ratio and the Entropy score were selected for the prediction of OGs. TUSON predictions are based on the calculation of a combined p-value (and q-value) of the selected parameters, by using an extended version of the Liptak method (Supp. Table 3a, b). Based on the combined p-values derived with the TUSON method, we estimated the total number of predicted TSGs and OGs by using a histogram-based method (Mosig et al., 2001).
Charm and Chrom score and correlation with frequency of SCNAs
For each arm and chromosome respectively, the Charm and Chrom scores for a certain gene set (TSGs, OGs or Essential genes) represent the density of the genes contained in that set weighted by their predicted potency. The potency of each gene corresponds to its rank position within its gene set list ranked by the TUSON p-value or by the LOF/Benign ratio for the Essential genes. For the cumulative CharmTSG-OG-Ess and ChromTSG-OG-Ess score the scores of OGs and Essential genes were subtracted from the scores relative to the TSGs. The correlation analysis was performed using two-sided Pearson’s correlation test between the Charm and Chrom score and the frequency of deletion and amplification of each arm and chromosome across all tumors (Supp. Table 6).
Analysis of functional gene sets
The STOP gene list was derived from an analysis performed using RNAi gene enrichment ranking (RIGER) algorithm (Cheung et al., 2011) on a previously described functional shRNA-based proliferation screen (Solimini et al., 2012, Supp. Table 5a). An in silico list of 332 Essential genes was derived by considering the intersection between the lists of genes predicted to be housekeeping genes and highly conserved genes (Marcotte et al., 2012, Supp. Table 5a). We used the Fisher’s exact test to examine the significance of the association between the presence of a gene in recurrent SCNAs and its presence among a certain gene set.
For additional information see the Supplemental Experimental Procedures.
Supplementary Material
01
02
03
04
05
06
07
08
Acknowledgments
We thank Simon Forbes and Michael Stratton (Wellcome Trust Sanger Institute, UK) for the data from the Cosmic dataset (http://cancer.sanger.ac.uk/cancergenome/projects/cosmic/), Eric Wooten for help on data extraction and Chad Creighton and Kim Rathmell for allowing access to their unpublished data on KICH SCNAs. We also thank Andrew Futreal, Semin Lee, David Livingston, David Page, Mary-Claire King and Atanas Kamburov for helpful advice; Bert Vogelstein, Judith Glaven and members of the Elledge lab for helpful comments on the manuscript. This work was funded by a DOD Breast Cancer Innovator Award and NIH grant to S.J.E, U54LM008748 to P.J.P and K08DK081612 to J.C.Y. S.J.E. is an investigator with the Howard Hughes Medical Institute. We apologize to our colleagues whose papers we could not cite due to space limitations.
Footnotes
Publisher's Disclaimer: This is a PDF file of an unedited manuscript that has been accepted for publication. As a service to our customers we are providing this early version of the manuscript. The manuscript will undergo copyediting, typesetting, and review of the resulting proof before it is published in its final citable form. Please note that during the production process errors may be discovered which could affect the content, and all legal disclaimers that apply to the journal pertain.
References
- Adamson B, Smogorzewska A, Sigoillot FD, King RW, Elledge SJ. A genome-wide homologous recombination screen identifies the RNA-binding protein RBMX as a component of the DNA-damage response. Nature cell biology. 2012;14:318–328. [Europe PMC free article] [Abstract] [Google Scholar]
- Adzhubei IA, Schmidt S, Peshkin L, Ramensky VE, Gerasimova A, Bork P, Kondrashov AS, Sunyaev SR. A method and server for predicting damaging missense mutations. Nature methods. 2010;7:248–249. [Europe PMC free article] [Abstract] [Google Scholar]
- Alexandrov LB, Nik-Zainal S, Wedge DC, Aparicio SA, Behjati S, Biankin AV, Bignell GR, Bolli N, Borg A, Borresen-Dale AL, et al. Signatures of mutational processes in human cancer. Nature. 2013;500:415–421. [Europe PMC free article] [Abstract] [Google Scholar]
- Beroukhim R, Mermel CH, Porter D, Wei G, Raychaudhuri S, Donovan J, Barretina J, Boehm JS, Dobson J, Urashima M, et al. The landscape of somatic copy-number alteration across human cancers. Nature. 2010;463:899–905. [Europe PMC free article] [Abstract] [Google Scholar]
- Cancer Genome Atlas Research Network. Comprehensive molecular portraits of human breast tumours. Nature. 2012;490:61–70. [Europe PMC free article] [Abstract] [Google Scholar]
- Cancer Genome Atlas Research Network. Comprehensive genomic characterization defines human glioblastoma genes and core pathways. Nature. 2008;455:1061–1068. [Europe PMC free article] [Abstract] [Google Scholar]
- Cancer Genome Atlas Research Network. Kandoth C, Schultz N, Cherniack AD, Akbani R, Liu Y, Shen H, Robertson AG, Pashtan I, Shen R, et al. Integrated genomic characterization of endometrial carcinoma. Nature. 2013;497:67–73. [Europe PMC free article] [Abstract] [Google Scholar]
- Conti P, Youinou P, Theoharides TC. Modulation of autoimmunity by the latest interleukins (with special emphasis on IL-32) Autoimmunity reviews. 2007;6:131–137. [Abstract] [Google Scholar]
- Dees ND, Zhang Q, Kandoth C, Wendl MC, Schierding W, Koboldt DC, Mooney TB, Callaway MB, Dooling D, Mardis ER, et al. MuSiC: identifying mutational significance in cancer genomes. Genome research. 2012;22:1589–1598. [Europe PMC free article] [Abstract] [Google Scholar]
- Forbes SA, Tang G, Bindal N, Bamford S, Dawson E, Cole C, Kok CY, Jia M, Ewing R, Menzies A, et al. COSMIC (the Catalogue of Somatic Mutations in Cancer): a resource to investigate acquired mutations in human cancer. Nucleic acids research. 2010;38:D652–657. [Europe PMC free article] [Abstract] [Google Scholar]
- Futreal PA, Coin L, Marshall M, Down T, Hubbard T, Wooster R, Rahman N, Stratton MR. A census of human cancer genes. Nature reviews Cancer. 2004;4:177–183. [Europe PMC free article] [Abstract] [Google Scholar]
- Gudjonsson T, Altmeyer M, Savic V, Toledo L, Dinant C, Grofte M, Bartkova J, Poulsen M, Oka Y, Bekker-Jensen S, et al. TRIP12 and UBR5 suppress spreading of chromatin ubiquitylation at damaged chromosomes. Cell. 2012;150:697–709. [Abstract] [Google Scholar]
- Hanahan D, Weinberg RA. Hallmarks of cancer: the next generation. Cell. 2011;144:646–674. [Abstract] [Google Scholar]
- Hou JM, Krebs M, Ward T, Sloane R, Priest L, Hughes A, Clack G, Ranson M, Blackhall F, Dive C. Circulating tumor cells as a window on metastasis biology in lung cancer. The American journal of pathology. 2011;178:989–996. [Europe PMC free article] [Abstract] [Google Scholar]
- Lai K, Amsterdam A, Farrington S, Bronson RT, Hopkins N, Lees JA. Many ribosomal protein mutations are associated with growth impairment and tumor predisposition in zebrafish. Developmental dynamics: an official publication of the American Association of Anatomists. 2009;238:76–85. [Europe PMC free article] [Abstract] [Google Scholar]
- Lawrence MS, Stojanov P, Polak P, Kryukov GV, Cibulskis K, Sivachenko A, Carter SL, Stewart C, Mermel CH, Roberts SA, et al. Mutational heterogeneity in cancer and the search for new cancer-associated genes. Nature. 2013;499:214–218. [Europe PMC free article] [Abstract] [Google Scholar]
- Luo J, Solimini NL, Elledge SJ. Principles of cancer therapy: oncogene and non-oncogene addiction. Cell. 2009 Mar 6;136(5):823–37. [Europe PMC free article] [Abstract] [Google Scholar]
- Matsuoka S, Ballif BA, Smogorzewska A, McDonald ER, 3rd, Hurov KE, Luo J, Bakalarski CE, Zhao Z, Solimini N, Lerenthal Y, et al. ATM and ATR substrate analysis reveals extensive protein networks responsive to DNA damage. Science. 2007;316:1160–1166. [Abstract] [Google Scholar]
- Meyerson M, Gabriel S, Getz G. Advances in understanding cancer genomes through second-generation sequencing. Nature reviews Genetics. 2010;11:685–696. [Abstract] [Google Scholar]
- Mosig MO, Lipkin E, Khutoreskaya G, Tchourzyna E, Soller M, Friedmann A. A whole genome scan for quantitative trait loci affecting milk protein percentage in Israeli-Holstein cattle, by means of selective milk DNA pooling in a daughter design, using an adjusted false discovery rate criterion. Genetics. 2001;157:1683–1698. [Europe PMC free article] [Abstract] [Google Scholar]
- Nik-Zainal S, Van Loo P, Wedge DC, Alexandrov LB, Greenman CD, Lau KW, Raine K, Jones D, Marshall J, Ramakrishna M, et al. The life history of 21 breast cancers. Cell. 2012;149:994–1007. [Europe PMC free article] [Abstract] [Google Scholar]
- Ozery-Flato M, Linhart C, Trakhtenbrot L, Israeli S, Shamir R. Large-scale analysis of chromosomal aberrations in cancer karyotypes reveals two distinct paths to aneuploidy. 2011. [Europe PMC free article] [Abstract] [Google Scholar]
- Pavlova NN, Pallasch C, Elia AE, Braun CJ, Westbrook TF, Hemann M, Elledge SJ. A role for PVRL4-driven cell-cell interactions in tumorigenesis. eLife. 2013;2:e00358. [Europe PMC free article] [Abstract] [Google Scholar]
- Ruepp A, Waegele B, Lechner M, Brauner B, Dunger-Kaltenbach I, Fobo G, Frishman G, Montrone C, Mewes HW. CORUM: the comprehensive resource of mammalian protein complexes--2009. Nucleic acids research. 2010;38:D497–501. [Europe PMC free article] [Abstract] [Google Scholar]
- Scheel C, Weinberg RA. Cancer stem cells and epithelial-mesenchymal transition: concepts and molecular links. Seminars in cancer biology. 2012;22:396–403. [Abstract] [Google Scholar]
- Shannon CE, Weaver W. The Mathematical Theory of Communication. Urbana, IL: University of Illinois Press; 1949. [Google Scholar]
- Solimini NL, Xu Q, Mermel CH, Liang AC, Schlabach MR, Luo J, Burrows AE, Anselmo AN, Bredemeyer AL, Li MZ, et al. Recurrent hemizygous deletions in cancers may optimize proliferative potential. Science. 2012;337:104–109. [Europe PMC free article] [Abstract] [Google Scholar]
- Stamatoyannopoulos JA, Adzhubei I, Thurman RE, Kryukov GV, Mirkin SM, Sunyaev SR. Human mutation rate associated with DNA replication timing. Nature genetics. 2009;41:393–395. [Europe PMC free article] [Abstract] [Google Scholar]
- Stark C, Breitkreutz BJ, Reguly T, Boucher L, Breitkreutz A, Tyers M. BioGRID: a general repository for interaction datasets. Nucleic acids research. 2006;34:D535–539. [Europe PMC free article] [Abstract] [Google Scholar]
- Stratton MR, Campbell PJ, Futreal PA. The cancer genome. Nature. 2009;458:719–724. [Europe PMC free article] [Abstract] [Google Scholar]
- Tang YC, Amon A. Gene copy-number alterations: a cost-benefit analysis. Cell. 2013;152(3):394–405. [Europe PMC free article] [Abstract] [Google Scholar]
- Vogelstein B, Kinzler KW. The multistep nature of cancer. Trends in genetics: TIG. 1993;9:138–141. [Abstract] [Google Scholar]
- Vogelstein B, Papadopoulos N, Velculescu VE, Zhou S, Diaz LA, Jr, Kinzler KW. Cancer genome landscapes. Science. 2013;339:1546–1558. [Europe PMC free article] [Abstract] [Google Scholar]
- White JK, Gerdin AK, Karp NA, Ryder E, Buljan M, Bussell JN, Salisbury J, Clare S, Ingham NJ, Podrini C, et al. Genome-wide Generation and Systematic Phenotyping of Knockout Mice Reveals New Roles for Many Genes. Cell. 2013;154:452–464. [Europe PMC free article] [Abstract] [Google Scholar]
- Willig TN, Gazda H, Sieff CA. Diamond-Blackfan anemia. Current opinion in hematology. 2000;7:85–94. [Abstract] [Google Scholar]
- Wood LD, Parsons DW, Jones S, Lin J, Sjoblom T, Leary RJ, Shen D, Boca SM, Barber T, Ptak J, et al. The genomic landscapes of human breast and colorectal cancers. Science. 2007;318:1108–1113. [Abstract] [Google Scholar]
- Zack TI, Schumacher SE, Carter S, Cherniack A, Saksena G, Tabak Barbara, Lawrence S, Zhang C-Z, et al. Pan-cancer patterns of somatic copy number alteration. Nat Gen. 2013;45:1134–1140. [Europe PMC free article] [Abstract] [Google Scholar]
- Zhang D, Zaugg K, Mak TW, Elledge SJ. A role for the deubiquitinating enzyme USP28 in control of the DNA-damage response. Cell. 2006;126:529–542. [Abstract] [Google Scholar]
Full text links
Read article at publisher's site: https://doi.org/10.1016/j.cell.2013.10.011
Read article for free, from open access legal sources, via Unpaywall:
http://www.cell.com/article/S0092867413012877/pdf
Citations & impact
Impact metrics
Article citations
Aneuploidy as a driver of human cancer.
Nat Genet, 56(10):2014-2026, 02 Oct 2024
Cited by: 0 articles | PMID: 39358600
Review
Regulation of p53 by the mitotic surveillance/stopwatch pathway: implications in neurodevelopment and cancer.
Front Cell Dev Biol, 12:1451274, 27 Sep 2024
Cited by: 0 articles | PMID: 39398482 | PMCID: PMC11466822
Review Free full text in Europe PMC
Proteogenomic characterization of skull-base chordoma.
Nat Commun, 15(1):8338, 27 Sep 2024
Cited by: 0 articles | PMID: 39333076 | PMCID: PMC11436687
SSCI: Self-Supervised Deep Learning Improves Network Structure for Cancer Driver Gene Identification.
Int J Mol Sci, 25(19):10351, 26 Sep 2024
Cited by: 0 articles | PMID: 39408682 | PMCID: PMC11476395
The History of Chromosomal Instability in Genome-Doubled Tumors.
Cancer Discov, 14(10):1810-1822, 01 Oct 2024
Cited by: 0 articles | PMID: 38943574 | PMCID: PMC7616501
Go to all (485) article citations
Data
Data behind the article
This data has been text mined from the article, or deposited into data resources.
BioStudies: supplemental material and supporting data
Similar Articles
To arrive at the top five similar articles we use a word-weighted algorithm to compare words from the Title and Abstract of each citation.
Somatic selection distinguishes oncogenes and tumor suppressor genes.
Bioinformatics, 36(6):1712-1717, 01 Mar 2020
Cited by: 20 articles | PMID: 32176769 | PMCID: PMC7703750
SWAN pathway-network identification of common aneuploidy-based oncogenic drivers.
Nucleic Acids Res, 50(7):3673-3692, 01 Apr 2022
Cited by: 10 articles | PMID: 35380699 | PMCID: PMC9023287
[Oncogenes, tumor suppressor genes, and aneuploidy: the sum of the nuances].
Bull Cancer, 101(4):340, 01 Apr 2014
Cited by: 0 articles | PMID: 24918235
Aneuploidy, the somatic mutation that makes cancer a species of its own.
Cell Motil Cytoskeleton, 47(2):81-107, 01 Oct 2000
Cited by: 121 articles | PMID: 11013390
Review
Funding
Funders who supported this work.
NIDDK NIH HHS (2)
Grant ID: K08DK081612
Grant ID: K08 DK081612
NIGMS NIH HHS (2)
Grant ID: T32 GM007753
Grant ID: R01 GM044664
NLM NIH HHS (2)
Grant ID: U54 LM008748
Grant ID: U54LM008748