Abstract
Free full text

Threat-related learning relies on distinct dorsal prefrontal cortex network connectivity
Abstract
Conditioned changes in the emotional response to threat (e.g. aversive unconditioned stimulus; UCS) are mediated in part by the prefrontal cortex (PFC). Unpredictable threats elicit large emotional responses, while the response is diminished when the threat is predictable. A better understanding of how PFC connectivity to other brain regions varies with threat predictability would provide important insights into the neural processes that mediate conditioned diminution of the emotional response to threat. The present study examined brain connectivity during predictable and unpredictable threat exposure using a fear conditioning paradigm (previously published in Wood et al., 2012) in which unconditioned functional magnetic resonance imaging data was reanalyzed to assess effective connectivity. Granger causality analysis was performed using the time series data from 15 activated regions of interest after hemodynamic deconvolution, to determine regional effective connectivity. In addition, connectivity path weights were correlated with trait anxiety measures to assess the relationship between negative affect and brain connectivity. Results indicate the dorsomedial PFC (dmPFC) serves as a neural hub that influences activity in other brain regions when threats are unpredictable. In contrast, the dorsolateral PFC (dlPFC) serves as a neural hub that influences the activity of other brain regions when threats are predictable. These findings are consistent with the view that the dmPFC coordinates brain activity to take action, perhaps in a reactive manner, when an unpredicted threat is encountered, while the dlPFC coordinates brain regions to take action, in what may be a more proactive manner, to respond to predictable threats. Further, dlPFC connectivity to other brain regions (e.g. ventromedial PFC, amygdala, and insula) varied with negative affect (i.e. trait anxiety) when the UCS was predictable, suggesting that stronger connectivity may be required for emotion regulation in individuals with higher levels of negative affect.
1.0 Introduction
Pavlovian conditioning research has traditionally focused on learning-related changes in behavior that develop in anticipation of a threat (i.e. the unconditioned stimulus; UCS). However, the advantage to being able to predict a threat is that it allows one to respond to that threat more effectively. Thus, from a functional perspective, the response to the threat itself is equally, if not more important than the anticipatory response (Domjan, 2005). Although prior research suggests conditioned changes in the emotional response to threat are mediated by neural circuitry that includes the prefrontal cortex (PFC) and amygdala (Dunsmoor et al., 2008, Wood et al., 2012), the effective connectivity of these brain regions during threat exposure has received limited attention. A better understanding of the connectivity of this network would provide valuable insight into the neural processes that mediate conditioned changes in the emotional response to threat.
Prior conditioning research has demonstrated learning-related changes in the emotional response to a threat. Specifically, the emotional response is diminished when a threat is predictable (Knight et al., 2010, Knight et al., 2011). These conditioned changes in the threat-elicited response appear to be mediated by brain regions that include the amygdala, insula, PFC, and inferior parietal lobule (IPL) (Dunsmoor et al., 2008, Knight et al., 2010, Wood et al., 2012, Wood et al., 2013). Many of these brain regions have been implicated in emotion regulation (Ochsner and Gross, 2005, Hartley and Phelps, 2010, Ochsner et al., 2012), and functional interactions between these brain regions likely influence the emotional response produced.
Neural circuitry that includes the PFC and amygdala is functionally connected and appears to mediate the conditioned emotional response. Prior work has demonstrated increased functional connectivity between the dorsomedial PFC (dmPFC) and amygdala following fear conditioning (Schultz et al., 2012). Further, the ventromedial PFC (vmPFC) shows learning-related connectivity to dmPFC and amygdala during the conditioned response (Delgado et al., 2008). Other research indicates that dorsolateral PFC (dlPFC) connectivity to the amygdala is dependent on the formation of predictive associations (Eippert et al., 2012). These findings suggest that the connectivity of the dlPFC, dmPFC, vmPFC, and amygdala is important for anticipatory fear learning (Delgado et al., 2008, Eippert et al., 2012, Schultz et al., 2012). However, there has been limited research on the functional connectivity of these brain regions in response to the threat itself (UCS). Although prior work has shown weaker parahippocampal gyrus connectivity to vmPFC during threat exposure in individuals with PTSD compared to individuals without PTSD (Linnman et al., 2011), there has been no prior research investigating differences in brain connectivity to predictable compared to unpredictable threat.
Functional and effective connectivity techniques are often used to investigate the neural network interactions underlying cognitive function. While functional connectivity describes non-directional instantaneous correlations in activity between brain regions, effective connectivity describes the directional influence of one neural system on another (Friston, 2011). Specifically, time lagged effective connectivity techniques (e.g. Granger causality), can infer the directional causal influence of one brain region on another (Granger, 1969). Further, network analysis of connectivity data allows the investigation of hierarchical and modular patterns of brain connectivity (Rubinov and Sporns, 2010, Park and Friston, 2013). These techniques may provide new insight into the network interactions that mediate threat-related learning.
Personality traits, such as negative affect, also appear to vary with the function and connectivity of brain regions that support emotion processes. Prior work has shown that negative affect is associated with the threat-elicited response within the dmPFC, dlPFC, and IPL (Wood et al., 2012). Similarly, individuals with high trait anxiety show low dmPFC activity when attending to threatening faces (Klumpp et al., 2011). Furthermore, individuals with high trait anxiety show high amygdala activity and low vmPFC activity during cues that predict threat (Indovina et al., 2011). Additionally, healthy individuals with higher anxiety demonstrate greater functional connectivity between dmPFC and the amygdala during rest (Kim et al., 2011, Robinson et al., 2012). Thus, effective connectivity of brain regions that support emotion processes may vary with negative affect. However, little is known about how these psychological traits relate to brain connectivity during threat exposure.
The present study investigated fMRI effective connectivity during predictable and unpredictable threat exposure to better understand the processes that influence learning-related changes in the emotional response to threat by reanalyzing data from a previously published Pavlovian conditioning study (Wood et al., 2012). Given the importance of the amygdala, dlPFC, dmPFC, and vmPFC in the diminution of the emotional response to threat (Dunsmoor et al., 2008, Knight et al., 2010, Wood et al., 2012, Wood et al., 2013), we hypothesized that these brain regions would show differential connectivity to predictable and unpredictable threats. Further, we expected connectivity within this neural network to vary with individual differences in trait anxiety.
2.0 Materials and Methods
2.1 Participants
Data from twenty-one healthy right-handed volunteers (12 males, 9 females; age = 20.81 ± 0.71 years; range = 19–33 years) that participated in a previously published neuroimaging study of fear conditioning (Wood et al., 2012) were reanalyzed for the present investigation. All subjects provided written informed consent as approved by the University of Alabama at Birmingham Institutional Review Board.
2.2 Task design
Presentation software (Neurobehavioral Systems, Inc.; Albany, CA) was used to present visual and auditory stimuli during fMRI using an IFIS-SA LCD video screen (Invivo Corp.; Gainesville, FL) with MR-compatible pneumatic headphones. During the task, participants were exposed to a fear conditioning procedure during which two distinct tones (700 and 1300 Hz) served as conditioned stimuli (CSs). Each of the first four scans were 590 seconds long and contained 8 trials in which one tone (CS+) co-terminated with a white noise UCS (100dB; 500 milliseconds duration) and 8 trials in which the second tone (CS−) was presented without the UCS. Each of the four scans contained three test trials that consisted of one trial in which the CS+ co-terminated with the UCS (CS+UCS), one trial in which the CS− co-terminated with the UCS (CS−UCS), as well as one presentation of the UCS alone for a total of 19 trials in each scan (see Wood et al., 2012 for additional detail). The final scan was 920 seconds long and contained 30 test trials (10 CS+UCS trials, 10 CS−UCS trials, and 10 UCS alone trials) (see Supplemental Figure S1). The current analysis assessed connectivity during the 30 test trials of the last scanning block.
2.3 Behavioral data
Participants rated the unpleasantness of the UCS on a scale from 0 (not unpleasant) to 10 (most unpleasant). Participants generally rated the UCS as moderately unpleasant (M = 3.44, SEM = 0.54). UCS expectancy, skin conductance response (SCR), and State-Trait Anxiety Inventory (STAI form Y; Spielberger, 1983) data were also collected. The methods and results of the behavioral data collection and analysis have been described previously (Knight and Wood, 2011, Wood et al., 2012). Briefly, participants were asked to rate their expectancy of the UCS using a continuous rating scale from 0 to 100 (Supplemental Figure S2). UCS expectancy did not differ between early CS−UCS test trials and UCS alone presentations. However, on late test trials, participants had significantly higher UCS expectancy ratings on CS−UCS trials compared to the UCS alone. UCS expectancy to the CS+UCS remained significantly greater than the UCS alone and CS−UCS throughout the task. Skin conductance was also collected during the task as a physiological index of learning. SCR was significantly diminished on CS+UCS trials compared to CS−UCS trials and UCS alone trials. Unconditioned SCR did not differ on CS−UCS and UCS alone trials. The scores on the trait scale of the STAI were used in the current study as an index of the long term predisposition for negative affectivity (Spielberger, 1983). Detailed statistical analyses of these data have been published previously (Wood et al., 2012).
2.4 Functional MRI acquisition
Functional magnetic resonance imaging (fMRI) data were acquired on a 3 Tesla Siemens Allegra scanner (TR = 2000ms, TE = 30ms, flip angle = 70°, FOV = 24cm, matrix = 64×64, slice thickness = 4mm). A high-resolution anatomical image (MPRAGE) was obtained in the sagittal plane using a T1 weighted series (TR = 2300 ms, TE = 3.9 ms, flip angle = 12°, FOV = 25.6 cm, matrix = 256×256, slice thickness = 1 mm, 0.5 mm gap).
2.5 Analyses
Detailed information on functional image processing and analyses have been previously reported (Wood et al., 2012). Briefly, image processing was executed using the Analysis of Functional NeuroImages (AFNI) software package (Cox, 1996), using standard processing techniques (see Wood et al., 2012 for more details). Functional MRI data were modeled with the gamma variate hemodynamic response function and analyzed at the individual subject level using multiple linear regression. The regressors included reference waveforms for the CS+, CS−, CS+UCS (i.e. UCS that followed the CS+), CS−UCS (i.e. UCS that followed the CS−), and UCS alone, as well as nuisance regressors for joystick movement and head motion parameters. Functional data were normalized to the Talairach and Tournoux stereotaxic coordinate system (Talairach and Tournoux, 1988).
A group level repeated-measures ANOVA was conducted to identify regional differences in unconditioned fMRI signal amplitude during CS+UCS, CS−UCS, and UCS alone test trials. Additional analyses comparing brain behavior relationships were also conducted. A FWE p<0.05 cluster level correction was applied in all analyses to control for multiple comparisons. Based on these analyses (Wood et al., 2012), 15 activation defined regions of interest (ROI) were chosen for the effective connectivity analysis. ROI volume and center of mass are listed in Table 1. The mean time series from the entire volume of each functional ROI were extracted for all participants and used in subsequent effective connectivity analysis.
Table 1
Regions of Interest
Region | Volume (mm3) | Talairach (x,y,z) |
---|---|---|
Medial PFC | ||
dmPFC | 22771 | 1, 20, 39 |
vmPFC | 656 | 12, 49, 14 |
dlPFC | ||
Right(1) | 3668 | 30, 44, 27 |
Right(2) | 14229 | 40, 13, 37 |
Left(1) | 1110 | −33, 50, 19 |
Left(2) | 908 | −21, 41, 40 |
Left(3) | 11710 | −38, 10, 38 |
IPL | ||
Right(1) | 892 | 49, −42, 25 |
Right(2) | 3210 | 42, −53, 43 |
Left | 4672 | −42, −51, 43 |
Insula | ||
Right | 1398 | 38, 15, 0 |
Left | 4731 | −38, 14, −1 |
Amygdala | ||
Right | 460 | 25, −4, −16 |
Left | 511 | −25, −4, −15 |
PCC | 11381 | −2, −34, 33 |
Numbers within parentheses reflect distinct ROI within a particular brain region (e.g. dlPFC).
Abbreviations: L, left; R, right; dlPFC, dorsolateral prefrontal cortex; dmPFC, dorsomedial prefrontal cortex; vmPFC, ventromedial prefrontal cortex; Amyg, amygdala; IPL, inferior parietal lobule; PCC, posterior cingulate cortex.
2.5.1 Effective Connectivity Model
In this study we utilized the concept of Granger Causality (GC) to perform effective connectivity analysis. GC is based on the principle that the causal influence of one region X on another region Y can be obtained if past values of the time series from the region X help predict the present and future values of the time series from the region Y (Granger, 1969). This method is implemented using a multivariate autoregressive (MVAR) model. Given n fMRI time series represented as X(t) = [x1(t) x2(t) … xn(t)], the MVAR model with the n time series as its input can be formulated as shown below
In the above equation (Eq. 1) the parameters α, Δ, and σ represent the model coefficients, the model error and the order of the model, respectively. The order of the model (σ) is determined using the Akaike/Bayesian information criterion (Akaike, 1974, Schwarz, 1978). In this model, the effect of instantaneous correlation on causality is minimized by including both instantaneous influences between the time series α(0) and causal influences between the time series α(g) (here g=1 … σ) (Deshpande et al., 2010b). These model coefficients represent causal relationships between time series over the entire duration of the experiment, and allowing them to dynamically vary across time permits comparative evaluation of effective connectivity evoked by different external stimuli. The MVAR model shown in Eq. 1 can be made dynamic by varying the model coefficients (α) as a function of time (as shown below in Eq. 2)
Using the algorithm proposed by Arnold et al. (Arnold et al., 1998), the coefficients of the dynamic model αpq(g,t) (with p,q = 1 ‥ n) can then be adaptively estimated and used to obtain the Dynamic Granger causality (DGC) as shown below
Several earlier studies have used these MVAR models based on the GC framework to study the predictive relationship between time series from different brain regions (Roebroeck et al., 2005, Abler et al., 2006, Stilla et al., 2007, Deshpande et al., 2008, Stilla et al., 2008, Deshpande et al., 2009, Deshpande et al., 2010a, Deshpande et al., 2011, Hampstead et al., 2011, Krueger et al., 2011, Lacey et al., 2011, Preusse et al., 2011, Sathian et al., 2011, Strenziok et al., 2011, Deshpande and Hu, 2012, Deshpande et al., 2012). However it was shown that using raw fMRI time series in GC analysis could lead to confounds in the estimated causal connectivity metrics (David et al., 2008, Deshpande et al., 2010c), which can be attributed to the spatial variability of the hemodynamic response function (HRF) which may in part be of non-neural origin (Handwerker et al., 2004). Furthermore, recent work has suggested that vascular confounds may pose a concern for causal modeling (Webb et al., 2013). However, a vascular effect must manifest itself in the hemodynamic response. Consequently, blind deconvolution of the hemodynamic response and subsequent GC analysis in the latent neuronal space has been employed previously (David et al., 2008, Ryali et al., 2011, Deshpande et al., 2013, Sathian et al., 2013, Grant et al., 2014). Since the hemodynamic response is itself deconvolved from the fMRI time series, any vascular or other effects of non-neural origin that manifest in the hemodynamic response are also removed from the fMRI signal. Therefore, in the current study, we obtained the condition specific connectivity values by applying the dynamic MVAR model described earlier (Eq. 2) to the latent neuronal variables estimated by blind hemodynamic deconvolution using a cubature Kalman filter as described below (Havlicek et al., 2011).
Let us consider the n fMRI time series x1(t), x2(t) … xn(t). A dynamic state-space model can be formulated as shown below
In the state equation (Eq. 4), variables r, i, and h represent the latent neuronal state variables, the exogenous input and the HRF parameter variables respectively. The subscript T and superscript n represent continuous time and number of time series, respectively, while the function J links the current neuronal state to the previous neuronal state, HRF parameters, and exogenous input. The variables C, V, and B represent the zero mean Gaussian state noise vectors. The measurement equation linking the state variables to the observed variables (fMRI time series) is shown in Eq. 5.
Here M is the measurement function, and the variables t and k represent the discrete time and measurement noise, respectively. The inputs to the model are the exogenous input (i) and the fMRI time series xn(t). As shown earlier, the cubature Kalman filter performs very efficient joint estimation of the latent neuronal variables and the HRF parameters (Havlicek et al., 2011). In addition to this, the latent neuronal variables can be successfully estimated at a finer temporal resolution by using a smaller time step (10 times smaller than the TR) while discretizing the continuous time model during the estimation. In this study, instead of the raw fMRI time series xn(t), the estimated neuronal variables rn(t) were input to the dynamic MVAR model (Eq. 2) to obtain the condition specific GC values.
2.5.2 Effective connectivity analysis
The mean time series from 15 regions of interest were extracted for all participants. These average time series were temporally normalized and then the latent neuronal state variables were obtained by hemodynamic deconvolution of the fMRI time series using the cubature Kalman filter (Havlicek et al., 2011). A boxcar function corresponding to the input stimulus (CS+UCS, CS−UCS, and UCS alone) was used as the exogenous input to the deconvolution model along with normalized fMRI time series from previously identified activated ROI. The hidden neuronal variables obtained after deconvolution were input into a dynamic MVAR model to obtain dynamic effective connectivity between every pair of ROI for all the participants. Samples of task specific connectivities were obtained by populating the causality values from all participants into three different samples based on the CS+UCS, CS−UCS, and UCS alone (test trials) conditions.
An ANOVA was performed on these samples to find the paths which were significantly different (p<0.05) between the three trial types. Only such paths were considered for further statistical analyses. For the paths identified above, differences in effective connectivity were evaluated by first using a one sample t-test to identify the paths that were significant within CS+UCS, CS−UCS, and UCS alone trial types (p<0.05) followed by a two sample t-test to determine paths that were significantly different between the CS+UCS and UCS alone trial types (p<0.01). A schematic illustrating the effective connectivity analysis pipeline and associated statistical analyses is shown in Figure 1.
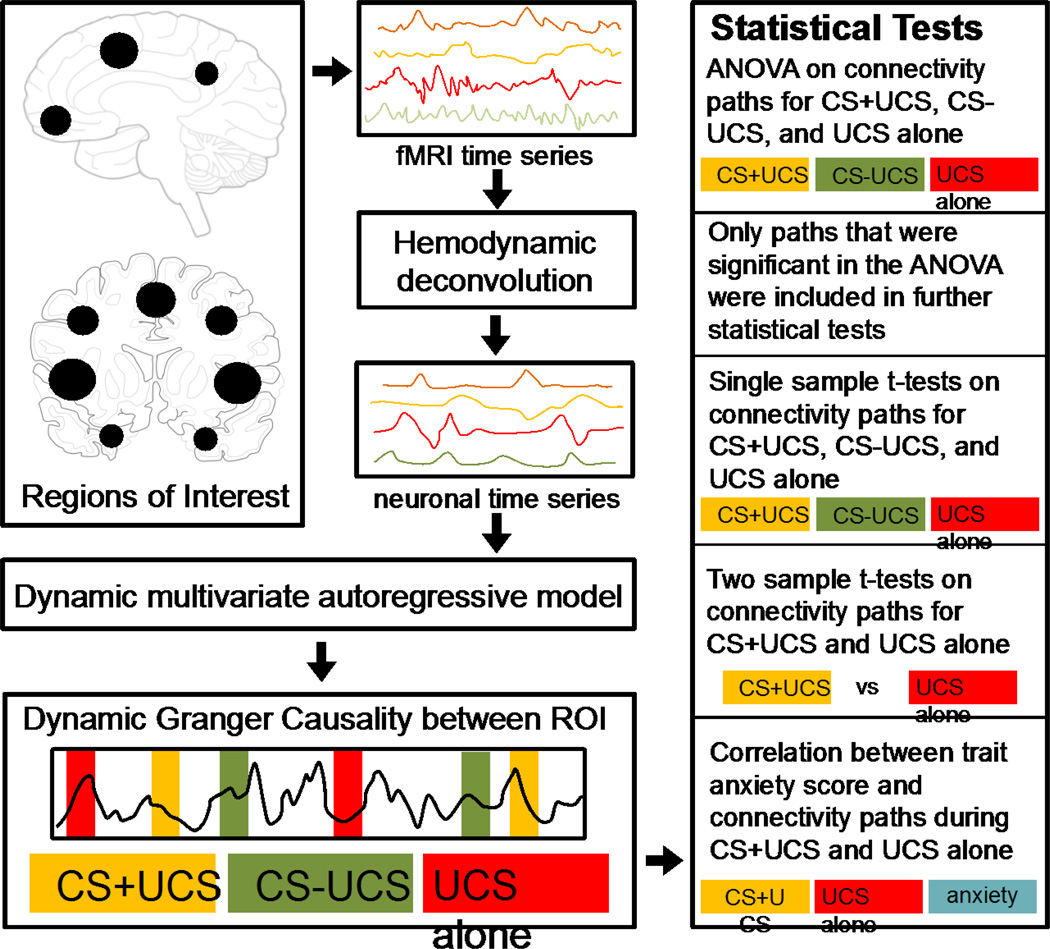
A schematic illustrating the effective connectivity analysis pipeline. Based on prior analyses (Wood et al., 2012), 15 activation defined regions of interest (ROI) were chosen for the effective connectivity analysis. The mean fMRI time series from each ROI were extracted for all participants. The time series was temporally normalized and deconvolved using a cubature Kalman filter to obtain the latent neuronal response. The neuronal response from each ROI was included in the dynamic multivariate autoregressive model to obtain the causal connectivity metrics between every pair of ROI. Samples of task specific connectivities were obtained by populating the causality values into three different samples based on the CS+UCS, CS−UCS, and UCS alone (test trials) conditions. Statistics were performed within trial type to obtain significant connections during CS+UCS, CS−UCS, and UCS alone. Two sample t-tests were used to find connections which were significantly different between CS+UCS compared to UCS alone. Finally, path weights on CS+UCS and UCS alone trials were correlated with negative affect (as measured with trait anxiety scale score).
The results of this analysis were summarized using Gephi (Bastian et al., 2009). Topological properties of effective connectivity networks were investigated using various graph-theoretic metrics (i) closeness was calculated as the shortest path length between two ROI, (ii) Betweenness was calculated as the number of times a ROI acted as a bridge along the shortest path between two other ROI, (iii) In-degree was defined as the total number of paths directed to a ROI, (iv) Out-degree was defined as the number of paths directed from an ROI to other ROI, and finally (v) Neural hubs, which are ROI that were most central to the network, were identified based on out-degree.
We also investigated the relationship between individual differences in brain connectivity and negative affect as indexed by trait anxiety scale scores. Correlation analysis was used to compare ROI connectivity path weights on CS+UCS and UCS alone trials with trait anxiety scores to assess the relationship between general negative affect and effective connectivity. Trait anxiety scores were missing for one subject. Thus, data from 20 subjects were used for the correlation analysis.
3.0 Results
The effective connectivity analysis revealed several common hubs across trial types (Figure 2). Based on out-degree, the left IPL was identified as a neural hub during UCS presentation on CS+UCS, CS−UCS, and UCS alone trials. On CS−UCS trials the left IPL, amygdala, and dlPFC (left dlPFC(2)) had the highest number of outward projections (out-degree =14, 14, 14 respectively; Table S1 and Figure 2b). Neural hubs on UCS alone trials included the left IPL, dmPFC, and dlPFC (left dlPFC(3)) (out-degree = 14, 10, 10 respectively; Table S1 and Figure 2c). On CS+UCS trials, the dlPFC (left dlPFC(2)) had the highest number of outward projections (out-degree = 14; Table S1 and Figure 2a) followed by the left IPL (out-degree = 12) and right Insula (out-degree = 11).
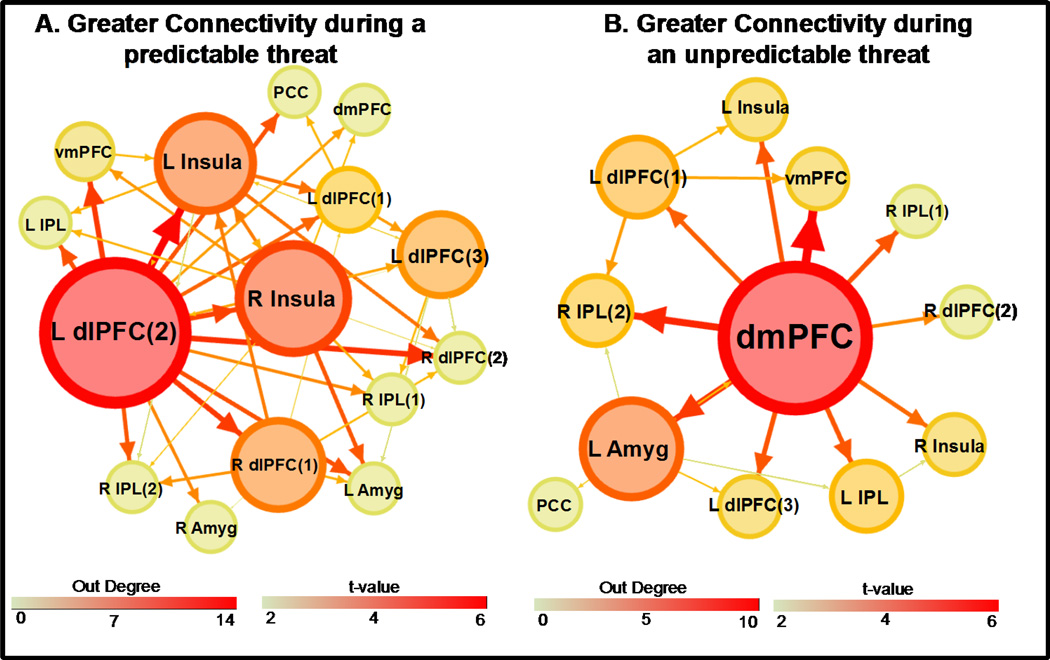
Effective connectivity on CS+UCS (A), CS−UCS (B), and UCS alone (C) trials. Arrows depict connectivity direction. Line size and color reflect the t-value for the one sample t-test using the sample of connectivity values obtained from the corresponding condition. ROI size and color depict ‘out degree’ (i.e. the total number of outward projections from a ROI). Numbers within parentheses reflect distinct ROI within a particular brain region (e.g. dlPFC). Abbreviations: L, left; R, right; dlPFC, dorsolateral prefrontal cortex; dmPFC, dorsomedial prefrontal cortex; vmPFC, ventromedial prefrontal cortex; Amyg, amygdala; IPL, inferior parietal lobule; PCC, posterior cingulate cortex. (1.5 columns)
Our previous paper (Wood et al., 2012) showed that UCS expectancy on the CS−UCS trials increased from early to late test trials, resulting in a combination of both low and high UCS expectancy on CS−UCS trials in the current connectivity analysis. In other words, the UCS was unpredictable on early CS−UCS trials, but predictable on late CS−UCS trials. These changes in the behavioral response to the CS−UCS makes interpreting the connectivity data related to predictability difficult. Due to the complexity of interpretation the CS−UCS connectivity data, all further results focus on differences in effective connectivity on CS+UCS and UCS alone trials.
3.1 Primary Hubs
3.1.1 Dorsolateral PFC
The dlPFC (left dlPFC(2)) showed greater effective connectivity to other ROI during CS+UCS than UCS alone trials (Figure 3a and Table S2). Further, the dlPFC had the highest number of outward projections of any ROI in the network (out-degree = 14; Table S4). Based on out-degree, the dlPFC was identified as the primary hub differentiating effective connectivity on CS+UCS versus UCS alone trials (Figure 3a).
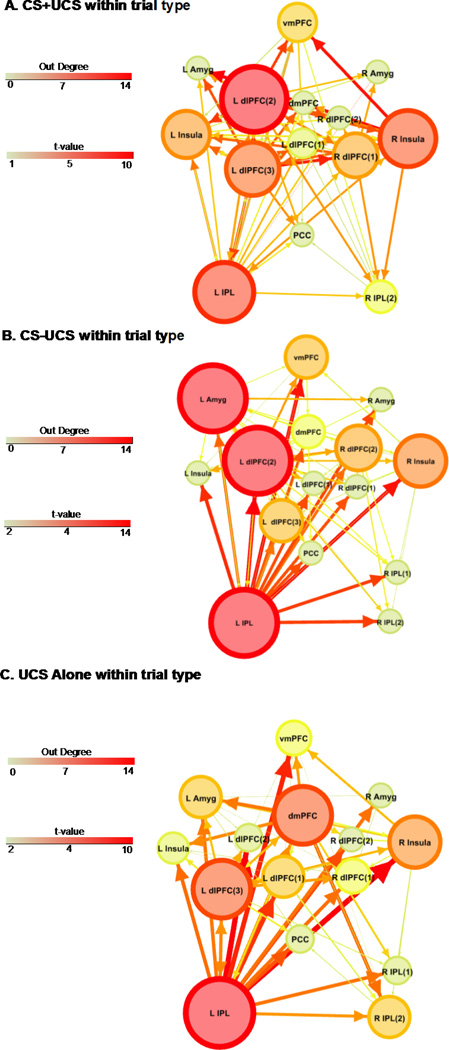
Differential effective connectivity. Greater connectivity during a predictable UCS (CS+UCS) than during an unpredictable UCS (UCS alone) (A) and greater connectivity during an unpredictable UCS than during a predictable UCS (B). Line size and color reflect the t-value for the two sample t-test. ROI size and color depict ‘out degree’ (i.e. the total number of outward projections from a ROI). Numbers within parentheses reflect distinct ROI within a particular brain region (e.g. dlPFC). Abbreviations: L, left; R, right; dlPFC, dorsolateral prefrontal cortex; dmPFC, dorsomedial prefrontal cortex; vmPFC, ventromedial prefrontal cortex; Amyg, amygdala; IPL, inferior parietal lobule; PCC, posterior cingulate cortex. (2 columns)
3.1.2 Dorsomedial PFC
The dmPFC showed greater effective connectivity to other ROI during UCS alone than during CS+UCS trials (Figure 3b and Table S3). Further, the dmPFC also had the highest number of outward projections (out-degree = 10) of any ROI in the network (Table S5). Based on out-degree, the dmPFC was identified as the primary hub differentiating effective connectivity on UCS alone compared to CS+UCS trials (Figure 3b).
3.2 Secondary Hubs
3.2.1 Insula
The right insula showed greater effective connectivity during CS+UCS than during UCS alone trials (Figure 3a and Table S2). Further, the insula had the second highest number of outward projections of any ROI in the network with an out-degree of 9 (Table S4). Based on out-degree, the insula was identified as an important secondary neural hub that differentiates effective connectivity on CS+UCS compared to UCS alone trials (Figure 3a).
3.2.2 Amygdala
The left amygdala was also identified as a secondary neural hub. The amygdala showed greater effective connectivity during UCS alone than during CS+UCS trials (Figure 3b and Table S3). The amygdala had the second highest number of outward projections of any ROI in the network (out-degree = 5; Table S5) in this contrast. Based on out-degree, the amygdala was identified as an important secondary neural hub during UCS alone compared to CS+UCS trials (Figure 3b).
3.3 Effective Connectivity and Negative Affect
Individual differences in brain connectivity and negative affect (trait anxiety scores) were also investigated. CS+UCS and UCS alone connectivity path weights (obtained from each subject) were correlated with trait anxiety scores. Effective connectivity path weights on CS+UCS, but not UCS alone trials, showed significant correlations with trait anxiety scores. Specifically, connections from dlPFC (left dlPFC(2)) to dmPFC, vmPFC, left amygdala, right insula, IPL (left IPL and right IPL(2)), and PCC were positively correlated (p<0.05 uncorrected) with trait anxiety scores (Figure 4, Table 2).
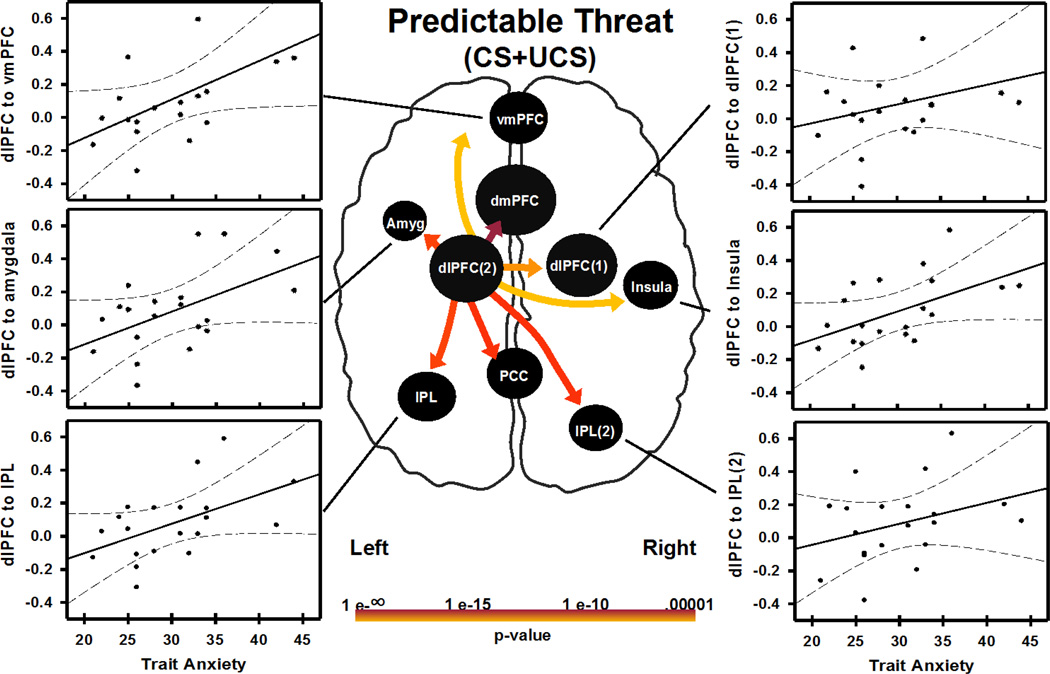
Relationship between negative affect (indexed as trait anxiety score) and connectivity during a predictable threat (i.e. CS+UCS). Trait anxiety correlated with left dlPFC hub connectivity strength to regions depicted in the figure (p<0.05 uncorrected). Trait anxiety score also correlated with dlPFC connectivity strength to PCC and dmPFC (graphs not shown). Abbreviations: Abbreviations: L, left; R, right; dlPFC, dorsolateral prefrontal cortex; dmPFC, dorsomedial prefrontal cortex; vmPFC, ventromedial prefrontal cortex; Amyg, amygdala; IPL, inferior parietal lobule; PCC, posterior cingulate cortex. (2 columns)
Table 2
Correlation of Trait anxiety and CS+UCS connectivity path weights from dlPFC hub
Left dlPFC hub connectivity to | Pearson r | R2 | p value |
---|---|---|---|
dmPFC | 0.461 | 0.212 | 0.041 |
R dlPFC(1) | 0.474 | 0.225 | 0.035 |
PCC | 0.450 | 0.202 | 0.047 |
L IPL | 0.510 | 0.261 | 0.021 |
R Insula | 0.523 | 0.273 | 0.018 |
R IPL(2) | 0.402 | 0.213 | 0.040 |
vmPFC | 0.546 | 0.298 | 0.013 |
L Amygdala | 0.509 | 0.259 | 0.022 |
Abbreviations: L, left; R, right; dlPFC, dorsolateral prefrontal cortex; dmPFC, dorsomedial prefrontal cortex; vmPFC, ventromedial prefrontal cortex; IPL, inferior parietal lobule; PCC, posterior cingulate cortex.
4.0 Discussion
The present study examined differences in effective brain connectivity to predictable and unpredictable presentations of a threat (i.e. UCS) to better understand the neural mechanisms of threat-related learning. Our findings indicate that predictable and unpredictable threats elicit significantly different patterns of effective connectivity between several brain regions that mediate learning-related changes in the emotional response to threat. Specifically, dlPFC (left dlPFC(2)) connectivity to brain regions including the vmPFC, amygdala, bilateral insula, and bilateral IPL was greater during predictable (i.e. CS+UCS trials) than unpredictable (i.e. UCS alone trials) UCS presentations. In contrast, the dmPFC showed greater connectivity to regions that include the vmPFC, dlPFC, amygdala, insula, and IPL when the UCS was unpredictable (i.e. UCS alone trials) compared to predictable (i.e. CS+UCS trials). Furthermore, the effective connectivity between dlPFC (left dlPFC(2)) and several brain regions was correlated with trait anxiety scores when the UCS was predictable (i.e. CS+UCS). These findings suggest that dlPFC and dmPFC connectivity mediates learning-related changes to threat and indicates individual differences in negative affect may be associated with the connectivity of brain regions that respond to threat.
The dlPFC and dmPFC are part of a neural circuit that plays an important role in emotion regulation (Arnsten, 2009, Hartley and Phelps, 2010, Ochsner et al., 2012). In general, the dlPFC and dmPFC appear to regulate activity in vmPFC (Hartley and Phelps, 2010) which, in turn, regulates amygdala activity that controls important aspects of the peripheral expression of emotion. Further, prior work indicates that activity within the dmPFC and dlPFC is highly correlated (Fox et al., 2005), suggesting these brain regions closely interact with one another (Fan et al., 2005, Seeley et al., 2007). In fact, prior research investigating the neural mechanisms of threat-related learning have consistently shown activation of the dmPFC and dlPFC (Dunsmoor et al., 2008, Knight et al., 2010, Wood et al., 2012, Wood et al., 2013), suggesting both of these regions are important for threat-related learning.
Although the dmPFC and dlPFC both appear to support emotion regulation processes, these brain regions may mediate slightly different aspects of cognitive control (Cole and Schneider, 2007, Dosenbach et al., 2008, Braver, 2012). More specifically, different components of the PFC-amygdala circuit may support somewhat distinct aspects of emotional control. One component, centered on the dlPFC, may use a proactive process to maintain attentional resources in preparation for important upcoming events (Braver et al., 2007, Braver, 2012), like a threat. Findings from the present study demonstrate the dlPFC has stronger effective connectivity to other brain regions during predictable (CS+UCS) than unpredictable threat (UCS alone). Further, the dlPFC was identified as the primary neural hub during predictable threat exposure (Figure2a and and3a).3a). These findings are consistent with the view that the dlPFC is a central component of a neural network that manages threat-related information in a proactive manner.
In contrast to the proactive control processes the dlPFC appears to support, the dmPFC (including the dorsal anterior cingulate (dACC)) appears to mediate reactive control processes (MacDonald, 2000, Braver, 2012). Reactive control processes detect and resolve information only as needed (i.e. primarily after a stimulus is presented) (Braver, 2012). Reactive control is necessary when events are unpredictable, surprising, or ambiguous (Keri et al., 2004, Hayden et al., 2011). For example, neuronal firing rate within the dmPFC is greater when a reward is unexpected (Hayden et al., 2011). Further, dmPFC activity varies with the uncertainty of the information being processed (Hayden et al., 2011). The dmPFC also demonstrates functional connectivity to the amygdala and other prefrontal brain regions when processing ambiguous stimuli (Nomura et al., 2003, Zaretsky et al., 2010). In the present study we observed greater effective connectivity during unpredictable threat (UCS alone) within the dmPFC. This finding is consistent with prior work that suggests the dmPFC supports reactive control processes (Braver, 2012) in the face of uncertain (Touroutoglou et al., 2012) or unexpected (Hayden et al., 2011) events. Further, the dmPFC was identified as the primary neural hub during unpredictable threat exposure in the present study (Figure2c and and3b),3b), suggesting the dmPFC coordinates brain activity in a reactive manner to respond to unpredictable threat during fear conditioning.
While prefrontal regions play an important role in controlling the response to predictable and unpredictable threats, other brain structures also appear to contribute to the emotional processing of threat. For example, the insula has been implicated in the integration of disparate brain networks that process information and produce appropriate feelings and actions (Chang et al., 2013). Further, prior work suggests the insula is involved in learning cues that predict negative events (Knight et al., 2005, Cheng et al., 2006). In the present study, the insula showed greater effective connectivity during predictable threat (CS+UCS) than to unpredictable threat (UCS alone). Further, the insula was identified as a secondary hub during the CS+UCS (Figure2a and and3a)3a) reflecting the importance of insula connectivity during the emotional response to threat.
The amygdala was also identified as an important secondary hub in the present study. The amygdala plays an important role in the evaluation of emotional stimuli and production of the peripheral emotional response (Davis and Whalen, 2001, Cheng et al., 2003, Knight et al., 2005, Cheng et al., 2006, Berntson et al., 2007). Further, the amygdala is critical for fear learning and memory (Knight et al., 2005, Cheng et al., 2006). Specifically, the amygdala is important for fear acquisition and fear extinction (Stein et al., 2007). The amygdala has also been shown to respond in a bottom-up manner when a threat is surprising (Ochsner et al., 2009), and appears to play an important role in evaluating uncertainty (Etkin et al., 2009, Prater et al., 2013) and ambiguity (Brown et al., 2014). The amygdala is connected to the dmPFC (Phan et al., 2002, 2004, Ochsner et al., 2012), and dmPFC connectivity with amygdala is altered by stress exposure (van Marle et al., 2010). Amygdala connectivity is also altered in disorders of emotion regulation such as generalized anxiety disorder (Etkin et al., 2009) and post-traumatic stress disorder (Dosenbach et al., 2008, Singh-Curry and Husain, 2009). In the present study, we observed greater effective connectivity from the amygdala to other brain regions during unpredictable threat (UCS alone; Figure2c and and3b).3b). Further, the amygdala was identified as an important neural hub during unpredictable threat trials, consistent with the view that the amygdala plays an important role in bottom-up threat-related processes.
In the present study, we identified several brain regions that showed differential effective connectivity to predictable and unpredictable threat. However other regions appear to support threat-related processes as well, regardless of the threat’s predictability. Specifically, the IPL was identified as a major hub during CS+UCS, CS−UCS, and UCS alone trials (Figure 2). However, the effective connectivity analysis did not differentiate IPL connectivity between these trial types (Figure 3). This finding suggests that the IPL is necessary for processing and producing the threat response independent of the predictability of the threat. This suggests the IPL may play a more general role in emotion processing. Although meta-analyses of emotion research typically focus on the role of brain regions like the amygdala, vmPFC, and dmPFC, the findings from these meta-analyses also clearly show the IPL is typically activated in emotion studies (Phan et al., 2002, 2004, Ochsner et al., 2012). In fact, IPL activation is observed in nearly 65% of all emotion research (Viviani, 2013). This prior work suggests the IPL plays a vital role in emotion processing. Further the IPL effective connectivity to dlPFC observed during threat exposure in the present study is consistent with theories of a frontoparietal attention network (Dosenbach et al., 2008, Singh-Curry and Husain, 2009), and suggests important attentional resources are deployed in response to threat.
Finally, we assessed the relationship between connectivity (i.e. during predictable and unpredictable threats) and trait anxiety scores in the present study. This analysis showed that when a threat was predictable, dlPFC connectivity to other emotion brain regions such as the amygdala and vmPFC were correlated with trait anxiety scores. Trait anxiety scores were not correlated with network connectivity during UCS alone trials. These analyses were performed with significance criteria that were not corrected for multiple comparisons and should be considered preliminary. However, these preliminary findings suggest that individuals with higher negative affect may require greater dlPFC connectivity to coordinate brain activity during predictable threats than individuals with low negative affect. This increased connectivity during predictable threat is consistent with research suggesting there is a threat-related attentional bias in individuals with anxiety disorders (Bar-Haim et al., 2007, Bishop, 2009), and suggests that dlPFC connectivity may play an important role in the etiology of anxiety related-disorders.
The present findings are based on analyses that compared CS+UCS (predictable) and UCS alone (unpredictable) trials. These two trials types differed in their predictability of the UCS, as demonstrated by our UCS expectancy data. However, these trial types also differed in relation to the tone CS that preceded the UCS. Specifically, a tone preceded the UCS on CS+UCS (predictable) trials, but not on UCS alone (unpredictable) trials. Although the tone CS certainly influences the predictability of the UCS, our prior work indicates learning-related changes in unconditioned fMRI signal and behavioral responses are not simply due to the presentation of a tone prior to the UCS(Dunsmoor et al., 2008, Knight et al., 2010, Knight et al., 2011, Wood et al., 2012). For example, greater diminution of unconditioned SCR and fMRI signal responses were demonstrated on CS+UCS than CS−UCS trials in the original analyses of these data (Wood et al., 2012). Both CS+UCS and CSUCS trials were preceded by a tone CS. Thus, the learning-related changes we observed cannot be explained merely by the presentation of a tone prior to the UCS. Instead, the evidence indicates these differential responses are due to associative learning processes that influence UCS predictability (Dunsmoor et al., 2008, Knight et al., 2010, Knight et al., 2011, Wood et al., 2012).
5.0 Conclusions
This study provides evidence of distinct prefrontal network connectivity that appears to mediate learning-related changes in the threat response. Specifically, this study demonstrates dlPFC has greater effective connectivity during predictable threat and dmPFC has greater effective connectivity during unpredictable threat. These findings are consistent with the view that separate brain networks support related, yet distinct aspects of emotional control. This study also highlights the importance of IPL connectivity during the threat response. Finally, our findings suggest that individuals with high negative affectivity preferentially use a dlPFC network during predictable threat.
Supplementary Material
1
Figure S1:Acquisition and Test Trials. Participants were exposed to a two tone discrimination procedure. During the acquisition phase, one tone (CS+) predicted a loud (100dB) white noise UCS, and the second tone (CS−) was presented alone. Test Trials consisted of presentations of the CS+ paired with the UCS, CS− paired with the UCS, and the UCS alone. Granger causality analysis was performed on the final scan which contained 30 test trials (10 CS+UCS trials, 10 CS−UCS trials, and 10 UCS alone trials).
2
Figure S2:UCS expectancy rating bar. Subjects were instructed to rate their UCS expectancy on a continuous scale from 0 to 100 (0 = certain the UCS would not be presented, 50 = uncertain whether the UCS would be presented, 100 = certain that the UCS would be presented), and were directed to continuously update their rating to reflect their current UCS expectancy. The UCS rating bar was presented throughout the duration of the conditioning task.
3
4
5
6
7
Acknowledgments
Role of funding source: This research was supported by the University of Alabama at Birmingham Faculty Development Grant Program and NIH grant MH098348 (D.C.K).
Abbreviations
CS | conditioned stimulus |
UCS | unconditioned stimulus |
CS+ | CS paired with the UCS |
CS+UCS | UCS that follows CS+ on test trials |
CS− | CS presented alone on acquisition blocks |
CS−UCS | UCS that follows CS− on test trials |
PFC | prefrontal cortex |
IPL | inferior parietal lobule |
dmPFC | dorsomedial prefrontal cortex |
dlPFC | dorsolateral prefrontal cortex |
vmPFC | ventromedial prefrontal cortex |
Footnotes
Publisher's Disclaimer: This is a PDF file of an unedited manuscript that has been accepted for publication. As a service to our customers we are providing this early version of the manuscript. The manuscript will undergo copyediting, typesetting, and review of the resulting proof before it is published in its final citable form. Please note that during the production process errors may be discovered which could affect the content, and all legal disclaimers that apply to the journal pertain.
Conflict of interest: The authors report no conflicts of interest.
References
- Abler B, Roebroeck A, Goebel R, Hose A, Schfnfeldt-Lecuona C, Hole G, Walter H. Investigating directed influences between activated brain areas in a motor-response task using fMRI. Magnetic Resonance Imaging. 2006;24:181–185. [Abstract] [Google Scholar]
- Akaike H. A new look at the statistical model identification. Automatic Control, IEEE Transactions on. 1974;19:716–723. [Google Scholar]
- Arnold M, Miltner W, Witte H, Bauer R, Braun C. Adaptive AR Modeling of Nonstationary Time Series by Means of Kalman Filtering. IEEE TRANSACTIONS ON BIOMEDICAL ENGINEERING. 1998;45:553–562. [Abstract] [Google Scholar]
- Arnsten AF. Stress signalling pathways that impair prefrontal cortex structure and function. Nature reviews Neuroscience. 2009;10:410–422. [Europe PMC free article] [Abstract] [Google Scholar]
- Bar-Haim Y, Lamy D, Pergamin L, Bakermans-Kranenburg MJ, van IMH. Threat-related attentional bias in anxious and nonanxious individuals: a meta-analytic study. Psychol Bull. 2007;133:1–24. [Abstract] [Google Scholar]
- Bastian M, Heyman S, Jacomy M. Gephi: an open source software for exploring and manipulating networks. International AAAI Conference on Weblogs and Social Media. 2009 [Google Scholar]
- Berntson GG, Bechara A, Damasio H, Tranel D, Cacioppo JT. Amygdala contribution to selective dimensions of emotion. Social cognitive and affective neuroscience. 2007;2:123–129. [Europe PMC free article] [Abstract] [Google Scholar]
- Bishop SJ. Trait anxiety and impoverished prefrontal control of attention. Nature neuroscience. 2009;12:92–98. [Abstract] [Google Scholar]
- Braver TS. The variable nature of cognitive control: a dual mechanisms framework. Trends in cognitive sciences. 2012;16:106–113. [Europe PMC free article] [Abstract] [Google Scholar]
- Braver TS, Gray JR, Burgess GC. Explaining the many varieties of working memory variation: Dual mechanisms of cognitive control. In: Conway ARA, et al., editors. Variation in Working Memory. New York: Oxford University Press; 2007. pp. 76–106. [Google Scholar]
- Brown VM, LaBar KS, Haswell CC, Gold AL, Mid-Atlantic MW, McCarthy G, Morey RA. Altered resting-state functional connectivity of basolateral and centromedial amygdala complexes in posttraumatic stress disorder. Neuropsychopharmacology : official publication of the American College of Neuropsychopharmacology. 2014;39:351–359. [Europe PMC free article] [Abstract] [Google Scholar]
- Chang LJ, Yarkoni T, Khaw MW, Sanfey AG. Decoding the role of the insula in human cognition: functional parcellation and large-scale reverse inference. Cerebral cortex. 2013;23:739–749. [Europe PMC free article] [Abstract] [Google Scholar]
- Cheng DT, Knight DC, Smith CN, Helmstetter FJ. Human amygdala activity during the expression of fear responses. Behavioral Neuroscience. 2006;120:1187–1195. [Abstract] [Google Scholar]
- Cheng DT, Knight DC, Smith CN, Stein EA, Helmstetter FJ. Functional MRI of human amygdala activity during Pavlovian fear conditioning: Stimulus processing versus response expression. Behavioral Neuroscience. 2003;117:3–10. [Abstract] [Google Scholar]
- Cole MW, Schneider W. The cognitive control network: Integrated cortical regions with dissociable functions. NeuroImage. 2007;37:343–360. [Abstract] [Google Scholar]
- Cox RW. AFNI: software for analysis and visualization of functional magnetic resonance neuroimages. Computers and Biomedical Research. 1996;29:162–173. [Abstract] [Google Scholar]
- David O, Guillemain I, Saillet S, Reyt S, Deransart C, Segebarth C, Depaulis A. Identifying Neural Drivers with Functional MRI: An Electrophysiological Validation. PLoS Biology. 2008;6(12):2683–2697. [Europe PMC free article] [Abstract] [Google Scholar]
- Davis M, Whalen PJ. The amygdala: vigilance and emotion. Molecular psychiatry. 2001;6:13–34. [Abstract] [Google Scholar]
- Delgado MR, Nearing KI, Ledoux JE, Phelps EA. Neural circuitry underlying the regulation of conditioned fear and its relation to extinction. Neuron. 2008;59:829–838. [Europe PMC free article] [Abstract] [Google Scholar]
- Deshpande G, Hu X. Investigating Effective Brain Connectivity from fMRI Data Past Findings and Current Issues with Reference to Granger Causality Analysis. Brain Connectivity. 2012;2:235–245. [Europe PMC free article] [Abstract] [Google Scholar]
- Deshpande G, Hu X, Lacey S, Stilla R, Sathian K. Object familiarity modulates effective connectivity during haptic shape perception. NeuroImage. 2010a;49(43):1991–2000. [Europe PMC free article] [Abstract] [Google Scholar]
- Deshpande G, Hu X, Stilla R, Sathian K. Effective Connectivity during Haptic Perception: A study using Granger causality analysis of functional magnetic resonance imaging data. NeuroImage. 2008;40(4):1807–1814. [Europe PMC free article] [Abstract] [Google Scholar]
- Deshpande G, LaConte S, James G, Peltier S, Hu X. Multivariate Granger causality analysis of brain networks. Human Brain Mapping. 2009;30(4):1361–1373. [Europe PMC free article] [Abstract] [Google Scholar]
- Deshpande G, Libero LE, Sreenivasan KR, Deshpande HD, Kana RK. Identification of neural connectivity signatures of autism using machine learning. Frontiers in Human Neuroscience. 2013;670 [Europe PMC free article] [Abstract] [Google Scholar]
- Deshpande G, Santhanam P, Hu X. Instantaneous and causal connectivity in resting state brain networks derived from functional MRI data. NeuroImage. 2011;54(52):1043–1052. [Europe PMC free article] [Abstract] [Google Scholar]
- Deshpande G, Sathian K, Hu X. Assessing and Compensating for Zero-lag Correlation Effects in Time-lagged Granger Causality Analysis of fMRI. IEEE Transactions on Biomedical Engineering. 2010b;57:1446, 1456. [Europe PMC free article] [Abstract] [Google Scholar]
- Deshpande G, Sathian K, Hu X. Effect of hemodynamic variability on Granger causality analysis of fMRI. NeuroImage. 2010c;52:884–896. [Europe PMC free article] [Abstract] [Google Scholar]
- Deshpande G, Sathian K, Hu X, Buckhalt J. A rigorous approach for testing the constructionist hypotheses of brain function. Behavioral and Brain Sciences. 2012;35(33):148. [Abstract] [Google Scholar]
- Domjan M. Pavlovian conditioning: a functional perspective. Annual review of psychology. 2005;56:179–206. [Abstract] [Google Scholar]
- Dosenbach NU, Fair DA, Cohen AL, Schlaggar BL, Petersen SE. A dual-networks architecture of top-down control. Trends in cognitive sciences. 2008;12:99–105. [Europe PMC free article] [Abstract] [Google Scholar]
- Dunsmoor JE, Bandettini PA, Knight DC. Neural correlates of unconditioned response diminution during Pavlovian conditioning. NeuroImage. 2008;40:811–817. [Europe PMC free article] [Abstract] [Google Scholar]
- Eippert F, Gamer M, Buchel C. Neurobiological mechanisms underlying the blocking effect in aversive learning. The Journal of neuroscience : the official journal of the Society for Neuroscience. 2012;32:13164–13176. [Europe PMC free article] [Abstract] [Google Scholar]
- Etkin A, Prater KE, Schatzberg AF, Menon V, Greicius MD. Disrupted amygdalar subregion functional connectivity and evidence of a compensatory network in Generalized Anxiety Disorder. Archives of General Psychiatry. 2009;66:1361–1372. [Abstract] [Google Scholar]
- Fan J, McCandliss BD, Fossella J, Flombaum JI, Posner MI. The activation of attentional networks. NeuroImage. 2005;26:471–479. [Abstract] [Google Scholar]
- Fox MD, Snyder AZ, Vincent JL, Corbetta M, Van Essen DC, Raichle ME. The human brain is intrinsically organized into dynamic, anticorrelated functional networks. Proceedings of the National Academy of Sciences of the United States of America. 2005;102:9673–9678. [Europe PMC free article] [Abstract] [Google Scholar]
- Friston KJ. Functional and effective connectivity: a review. Brain connectivity. 2011;1:13–36. [Abstract] [Google Scholar]
- Granger C. Investigating causal relations by econometric models and cross-spectral methods. Econometrica. 1969;37(3):424–438. [Google Scholar]
- Grant MM, White D, Hadley J, Hutcheson N, Shelton R, Sreenivasan KR, Deshpande G. Early life trauma and directional brain connectivity within major depression. Human brain mapping. 2014;35:15–26. [Europe PMC free article] [Abstract] [Google Scholar]
- Hampstead B, Stringer A, Stilla R, Deshpande G, Hu X, Moore A, Sathian K. Activation and effective connectivity changes following explicit memory training for face-name pairs in patients with mild cognitive impairment: A pilot study. Neurorehabilitation and Neural Repair. 2011;25(23):210–222. [Europe PMC free article] [Abstract] [Google Scholar]
- Handwerker D, Ollinger J, D’Esposito M. Variation of BOLD hemodynamic responses across subjects and brain regions and their effects on statistical analyses. NeuroImage. 2004;21:1639–1651. [Abstract] [Google Scholar]
- Hartley CA, Phelps EA. Changing fear: the neurocircuitry of emotion regulation. Neuropsychopharmacology : official publication of the American College of Neuropsychopharmacology. 2010;35:136–146. [Europe PMC free article] [Abstract] [Google Scholar]
- Havlicek M, Friston KJ, Jan J, Brazdil M, Calhoun VD. Dynamic modeling of neuronal responses in fMRI using cubature Kalman filtering. NeuroImage. 2011;56:2109–2128. [Europe PMC free article] [Abstract] [Google Scholar]
- Hayden BY, Heilbronner SR, Pearson JM, Platt ML. Surprise signals in anterior cingulate cortex: neuronal encoding of unsigned reward prediction errors driving adjustment in behavior. The Journal of neuroscience : the official journal of the Society for Neuroscience. 2011;31:4178–4187. [Europe PMC free article] [Abstract] [Google Scholar]
- Indovina I, Robbins TW, Nunez-Elizalde AO, Dunn BD, Bishop SJ. Fear-conditioning mechanisms associated with trait vulnerability to anxiety in humans. Neuron. 2011;69:563–571. [Europe PMC free article] [Abstract] [Google Scholar]
- Keri S, Decety J, Roland PE, Gulyas B. Feature uncertainty activates anterior cingulate cortex. Human brain mapping. 2004;21:26–33. [Europe PMC free article] [Abstract] [Google Scholar]
- Kim, Loucks RA, Palmer AL, Brown AC, Solomon KM, Marchante AN, Whalen PJ. The structural and functional connectivity of the amygdala: from normal emotion to pathological anxiety. Behavioural brain research. 2011;223:403–410. [Europe PMC free article] [Abstract] [Google Scholar]
- Klumpp H, Ho SS, Taylor SF, Phan KL, Abelson JL, Liberzon I. Trait anxiety modulates anterior cingulate activation to threat interference. Depression and anxiety. 2011;28:194–201. [Europe PMC free article] [Abstract] [Google Scholar]
- Knight DC, Lewis EP, Wood KH. Conditioned diminution of the unconditioned skin conductance response. Behavioral Neuroscience. 2011;125:626–631. [Abstract] [Google Scholar]
- Knight DC, Nguyen HT, Bandettini PA. The role of the human amygdala in the production of conditioned fear responses. NeuroImage. 2005;26:1193–1200. [Abstract] [Google Scholar]
- Knight DC, Waters NS, King MK, Bandettini PA. Learning-related diminution of unconditioned SCR and fMRI signal responses. NeuroImage. 2010;49:843–848. [Europe PMC free article] [Abstract] [Google Scholar]
- Knight DC, Wood KH. Investigating the neural mechanisms of aware and unaware fear memory with fMRI. Journal of Visualized Experiments. 2011;56 [Europe PMC free article] [Abstract] [Google Scholar]
- Krueger F, Landgraf S, Van der Meer E, Deshpande G, Hu X. Effective connectivity of the multiplication network: A functional MRI and multivariate Granger causality mapping study. Human Brain Mapping. 2011;32(39):1419–1431. [Europe PMC free article] [Abstract] [Google Scholar]
- Lacey S, Hagtvedt H, Patrick V, Anderson A, Stilla R, Deshpande G, Hu X, Sato J, Reddy S, Sathian K. Art for reward’s sake: Visual art recruits the ventral striatum. NeuroImage. 2011;55(51):420–433. [Europe PMC free article] [Abstract] [Google Scholar]
- Linnman C, Zeffiro TA, Pitman RK, Milad MR. An fMRI study of unconditioned responses in post-traumatic stress disorder. Biology of mood & anxiety disorders. 2011;1:8. [Europe PMC free article] [Abstract] [Google Scholar]
- MacDonald AW. Dissociating the Role of the Dorsolateral Prefrontal and Anterior Cingulate Cortex in Cognitive Control. Science. 2000;288:1835–1838. [Abstract] [Google Scholar]
- Nomura M, Iidaka T, Kakehi K, Tsukiura T, Hasegawa T, Maeda Y, Matsue Y. Frontal lobe networks for effective processing of ambiguously expressed emotions in humans. Neuroscience Letters. 2003;348:113–116. [Abstract] [Google Scholar]
- Ochsner KN, Gross JJ. The cognitive control of emotion. Trends in cognitive sciences. 2005;9:242–249. [Abstract] [Google Scholar]
- Ochsner KN, Ray RR, Hughes B, McRae K, Cooper JC, Weber J, Gabrieli JDE, Gross JJ. Bottom-up and top-down processes in emotion generation. Psychological Science. 2009;20:1322–1331. [Europe PMC free article] [Abstract] [Google Scholar]
- Ochsner KN, Silvers JA, Buhle JT. Functional imaging studies of emotion regulation: a synthetic review and evolving model of the cognitive control of emotion. Annals of the New York Academy of Sciences. 2012;1251:E1–E24. [Europe PMC free article] [Abstract] [Google Scholar]
- Park HJ, Friston K. Structural and functional brain networks: from connections to cognition. Science. 2013;342:1238411. [Abstract] [Google Scholar]
- Phan KL, Wager T, Taylor SF, Liberzon I. Functional neuroanatomy of emotion: a meta-analysis of emotion activation studies in PET and fMRI. NeuroImage. 2002;16:331–348. [Abstract] [Google Scholar]
- Phan KL, Wager T, Taylor SF, Liberzon I. Functional neuroimaging studies of human emotions. CNS. 2004;9:258–266. [Abstract] [Google Scholar]
- Prater KE, Hosanagar A, Klumpp H, Angstadt M, Phan KL. Aberrant amygdala-frontal cortex connectivity during perception of fearful faces and at rest in generalized social anxiety disorder. Depression and anxiety. 2013;30:234–241. [Europe PMC free article] [Abstract] [Google Scholar]
- Preusse F, Van der Meer E, Deshpande G, Krueger F, Wartenburger I. Fluid intelligence allows flexible recruitment of the parieto-frontal network in analogical reasoning. Frontiers in Human Neuroscience. 2011;5:22. [Europe PMC free article] [Abstract] [Google Scholar]
- Robinson OJ, Charney DR, Overstreet C, Vytal K, Grillon C. The adaptive threat bias in anxiety: amygdala-dorsomedial prefrontal cortex coupling and aversive amplification. NeuroImage. 2012;60:523–529. [Europe PMC free article] [Abstract] [Google Scholar]
- Roebroeck A, Formisano E, Goebel R. Mapping directed influence over the brain using Granger causality and fMRI. NeuroImage. 2005;25:230–242. [Abstract] [Google Scholar]
- Rubinov M, Sporns O. Complex network measures of brain connectivity: uses and interpretations. NeuroImage. 2010;52:1059–1069. [Abstract] [Google Scholar]
- Ryali S, Supekar K, Chen T, Menon V. Multivariate dynamical systems models for estimating causal interactions in fMRI. Neuroimage. 2011;54:807–823. [Europe PMC free article] [Abstract] [Google Scholar]
- Sathian K, Deshpande G, Stilla R. Neural changes with tactile learning reflect decision-level reweighting of perceptual readout. The Journal of Neuroscience. 2013;33:5387–5398. [Europe PMC free article] [Abstract] [Google Scholar]
- Sathian K, Lacey S, Stilla R, Gibson G, Deshpande G, Hu X, LaConte S, Glielmi C. Dual pathways for haptic and visual perception of spatial and texture information. NeuroImage. 2011;57:462–475. [Europe PMC free article] [Abstract] [Google Scholar]
- Schultz DH, Balderston NL, Helmstetter FJ. Resting-state connectivity of the amygdala is altered following Pavlovian fear conditioning. Frontiers in human neuroscience. 2012;6:242. [Europe PMC free article] [Abstract] [Google Scholar]
- Schwarz G. Estimating the Dimension of a Model. The Annals of Statistics. 1978;6:461–464. [Google Scholar]
- Seeley WW, Menon V, Schatzberg AF, Keller J, Glover GH, Kenna H, Reiss AL, Greicius MD. Dissociable intrinsic connectivity networks for salience processing and executive control. The Journal of neuroscience : the official journal of the Society for Neuroscience. 2007;27:2349–2356. [Europe PMC free article] [Abstract] [Google Scholar]
- Singh-Curry V, Husain M. The functional role of the inferior parietal lobe in the dorsal and ventral stream dichotomy. Neuropsychologia. 2009;47:1434–1448. [Europe PMC free article] [Abstract] [Google Scholar]
- Spielberger CD. State-trait anxiety inventory for adutls. RedwoodCity, CA: Mind Garder; 1983. [Google Scholar]
- Stein JL, Wiedholz LM, Bassett DS, Weinberger DR, Zink CF, Mattay VS, Meyer-Lindenberg A. A validated network of effective amygdala connectivity. NeuroImage. 2007;36:736–745. [Abstract] [Google Scholar]
- Stilla R, Deshpande G, LaConte S, Hu X, Sathian K. Posteromedial parietal cortical activity and inputs predict tactile spatial acuity. The Journal of Neuroscience. 2007;27:11091–11102. [Europe PMC free article] [Abstract] [Google Scholar]
- Stilla R, Hanna R, Hu X, Mariola E, Deshpande G, Sathian K. Neural processing underlying tactile microspatial discrimination in the blind: A functional magnetic resonance imaging study. Journal of Vision. 2008;8:13, 11–19. [Europe PMC free article] [Abstract] [Google Scholar]
- Strenziok M, Krueger F, Deshpande G, Lenroot R, van der Meer E, Grafman J. Fronto-Parietal Regulation of Media Violence Exposure in Adolescents: A Multi-Method Study. Social Cognitive and Affective Neuroscience. 2011;5:22. [Europe PMC free article] [Abstract] [Google Scholar]
- Talairach J, Tournoux P. Co-planar Stereotaxic Atlas of the Human Brain. New York: Thieme; 1988. [Google Scholar]
- Touroutoglou A, Hollenbeck M, Dickerson BC, Feldman Barrett L. Dissociable large-scale networks anchored in the right anterior insula subserve affective experience and attention. NeuroImage. 2012;60:1947–1958. [Europe PMC free article] [Abstract] [Google Scholar]
- van Marle HJ, Hermans EJ, Qin S, Fernandez G. Enhanced resting-state connectivity of amygdala in the immediate aftermath of acute psychological stress. NeuroImage. 2010;53:348–354. [Abstract] [Google Scholar]
- Viviani R. Emotion regulation, attention to emotion, and the ventral attentional network. Frontiers in human neuroscience. 2013;7:746. [Europe PMC free article] [Abstract] [Google Scholar]
- Webb JT, Ferguson MA, Nielsen JA, Anderson JS. BOLD Granger causality reflects vascular anatomy. PloS one. 2013;8:e84279. [Europe PMC free article] [Abstract] [Google Scholar]
- Wood KH, Kuykendall D, Ver Hoef LW, Knight DC. Neural substrates underlying learning-related changes of the unconditioned fear response. The Open Neuroimaging Journal. 2013;7:41–52. [Europe PMC free article] [Abstract] [Google Scholar]
- Wood KH, Ver Hoef LW, Knight DC. Neural mechanisms underlying the conditioned diminution of the unconditioned fear response. NeuroImage. 2012;60:787–799. [Abstract] [Google Scholar]
- Zaretsky M, Mendelsohn A, Mintz M, Hendler T. In the eye of the beholder: Internally driven uncertainty of danger recruits the amygdala and dorsomedial prefrontal cortex. Journal of Cognitive Neuroscience. 2010;22:2263–2275. [Abstract] [Google Scholar]
Full text links
Read article at publisher's site: https://doi.org/10.1016/j.neuroimage.2014.08.005
Read article for free, from open access legal sources, via Unpaywall:
https://europepmc.org/articles/pmc4252829?pdf=render
Citations & impact
Impact metrics
Citations of article over time
Alternative metrics
Smart citations by scite.ai
Explore citation contexts and check if this article has been
supported or disputed.
https://scite.ai/reports/10.1016/j.neuroimage.2014.08.005
Article citations
The subcortical brain regions influence the cortical areas during resting-state: an fMRI study.
Front Hum Neurosci, 18:1363125, 26 Jun 2024
Cited by: 0 articles | PMID: 39055533 | PMCID: PMC11271203
Associations of resting-state perfusion and auditory verbal hallucinations with and without emotional content in schizophrenia.
Neuroimage Clin, 40:103527, 10 Oct 2023
Cited by: 0 articles | PMID: 37871539 | PMCID: PMC10598456
Stress-induced Changes in Autonomic Reactivity Vary with Adolescent Violence Exposure and Resting-state Functional Connectivity.
Neuroscience, 522:81-97, 10 May 2023
Cited by: 0 articles | PMID: 37172687
Noninvasive Characterization of Functional Pathways in Layer-Specific Microcircuits of the Human Brain Using 7T fMRI.
Brain Sci, 12(10):1361, 07 Oct 2022
Cited by: 0 articles | PMID: 36291295 | PMCID: PMC9599333
Sex-related differences in violence exposure, neural reactivity to threat, and mental health.
Neuropsychopharmacology, 47(13):2221-2229, 27 Aug 2022
Cited by: 4 articles | PMID: 36030316 | PMCID: PMC9630543
Go to all (52) article citations
Data
Data behind the article
This data has been text mined from the article, or deposited into data resources.
BioStudies: supplemental material and supporting data
Similar Articles
To arrive at the top five similar articles we use a word-weighted algorithm to compare words from the Title and Abstract of each citation.
Stress-Induced Changes in Effective Connectivity During Regulation of the Emotional Response to Threat.
Brain Connect, 12(7):629-638, 31 Dec 2021
Cited by: 1 article | PMID: 34541896 | PMCID: PMC9634990
Controllability modulates the neural response to predictable but not unpredictable threat in humans.
Neuroimage, 119:371-381, 03 Jul 2015
Cited by: 26 articles | PMID: 26149610 | PMCID: PMC4564348
Neural mechanisms underlying the conditioned diminution of the unconditioned fear response.
Neuroimage, 60(1):787-799, 27 Dec 2011
Cited by: 31 articles | PMID: 22227141
Pavlovian conditioned diminution of the neurobehavioral response to threat.
Neurosci Biobehav Rev, 84:218-224, 02 Dec 2017
Cited by: 20 articles | PMID: 29203422 | PMCID: PMC5744889
Review Free full text in Europe PMC
Funding
Funders who supported this work.
NIMH NIH HHS (2)
Grant ID: MH098348
Grant ID: R01 MH098348