Abstract
Free full text

Translational Implications of Tumor Heterogeneity
Abstract
Advances in next-generation sequencing and bioinformatics have led to an unprecedented view of the cancer genome and its evolution. Genomic studies have demonstrated the complex and heterogeneous clonal landscape of tumors of different origins, and the potential impact of intratumor heterogeneity on treatment response and resistance, cancer progression and the risk of disease relapse. However, the significance of subclonal mutations, in particular mutations in driver genes, and their evolution through time and their dynamics in response to cancer therapies, is yet to be determined. The necessary tools are now available to prospectively determine whether clonal heterogeneity can be used as a biomarker of clinical outcome, and to what extent subclonal somatic alterations might influence clinical outcome. Studies that employ longitudinal tissue sampling, integrating both genomic and clinical data, have the potential to reveal the subclonal composition and track the evolution of tumors in order to address these questions, and to begin to define the breadth of genetic diversity in different tumor types, and its relevance to patient outcome. Such studies may provide further evidence for novel drug resistance mechanisms informing novel combinatorial, adaptive and tumour immune-therapies placed within the context of tumor evolution.
Introduction
Over the last few years, genomic studies have demonstrated the complex and heterogeneous landscape in cancer (1,2), and its potential implications for treatment response and prognosis (2-4). Using whole-genome or -exome sequencing these studies have demonstrated that tumors consist of somatic events, defined by mutations and copy number alterations (SCNAs), occurring early in tumor evolution, and somatic events present in some cells, but not all, occurring later in tumor evolution (5). In addition, these studies have shown spatial heterogeneity, branched evolution and mutational patterns that can vary over time (6,7), and in response to cancer therapies (8-10). Given the existence of such heterogeneity in tumors in advanced metastatic disease, the efficacy of therapies targeting somatic driver aberrations, even in combination, may be limited in terms of achieving disease cure, and contribute in some health economies to a prohibitive health economic burden (11). The relevance of subclonal mutations to cancer outcome and therapeutic response requires further investigation (12). Although this review focuses on genetic intratumor heterogeneity, both phenotypic and epigenetic factors are known to play a role in shaping the tumor landscape and are reviewed in-depth elsewhere (13).
Types of Tumor Heterogeneity
There are several types of genetic heterogeneity in cancer biology. The most well known is interpatient tumor heterogeneity (Fig. 1A), whereby no two patients with the same subtype of tumor behave the same clinically, with or without treatment. This may be related to host factors, such as tumor microenvironment, germ line variants influencing treatment response, together with the unique somatic mutations that can occur within the tumor of each individual patient (14). Intratumor heterogeneity (Fig. 1B), describes the existence of distinct cellular populations with specific phenotypic features within tumors and has long been recognized (15,16). It has been described in several tumor types including lung (7,17), breast (6,18,19), ovarian (20), pancreatic (21,22), kidney (23,24), colorectal (25), brain (26,27) and prostate cancers (28,29), as well as hematological malignancies, such as chronic lymphoblastic leukemia (CLL) (30), and acute lymphoblastic leukemia (31). Metastatic lesions at different secondary sites can arise from different cellular populations within a primary tumor, resulting in heterogeneity among metastases, known as intermetastatic heterogeneity (14) (Fig. 1C). In addition, since metastatic lesions can acquire new mutations and evolve independently with each cell division, heterogeneity within a metastasis can also exist, known as intrametastatic heterogeneity (14) (Fig. 1D).
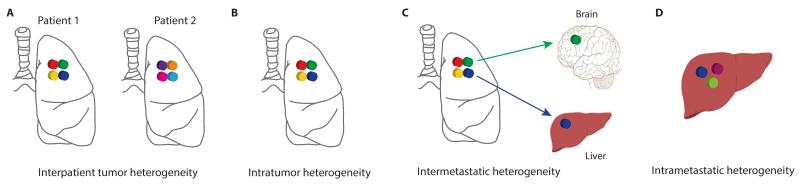
Different types of tumor heterogeneity. (A) Intratumor heterogeneity: the presence of multiple subclones within a primary tumor resulting in heterogeneity among tumor cells. (B) Interpatient heterogeneity: the presence of unique subclones in the tumor of each patient. (C) Intermetastatic heterogeneity: the presence of different subclones in different metastatic lesions of the same patient; some subclones may have been derived from the primary tumor and some may have emerged as a result of acquired alterations within each metastatic lesion. (D) Intrametastatic heterogeneity: the presence of multiple subclones within a single metastatic lesion.
Clonal Evolution and Phylogenetic Analyses
The clonality of somatic mutations within a tumor can be estimated with bioinformatics methods using variables such as tumor purity, copy number variation, and mutation variant allele frequency (32). The analysis of multiple biopsies from the same tumor can reveal the spatial composition and the evolutionary trajectory of subclones. The clonal and subclonal composition of each tumor can be used to construct distance-based phylogenetic trees, where clonal mutations present in all tumor regions occur early in tumorigenesis representing the most recent common ancestor (truncal events on the evolutionary tree), and subclonal mutations present in only a subset of regions, or cells within a single biopsy, occur later in tumorigenesis (branched events on the evolutionary tree) (Fig. 2). The accuracy of such clonality analyses is limited to the number of regions sampled and therefore is likely only based on a subset of tumor cells from the entire tumor. An alternative to this approach may be single-cell sequencing, which has the potential to determine the exact number of tumor cells with distinct mutations and copy number variations, and therefore reveal the true subclonal composition of a tumor.
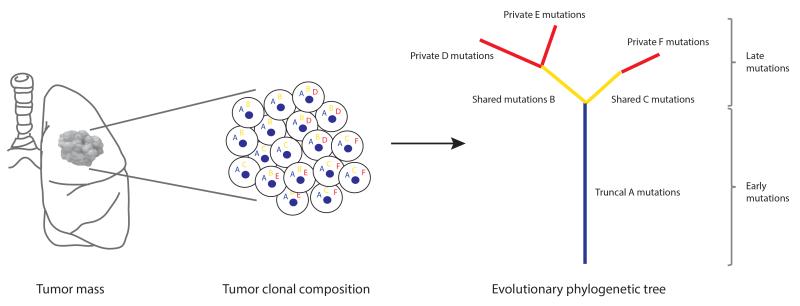
Phylogenetic tree analyses. Primary tumors consist of different subclones with shared and private somatic alterations. Alterations shared by all tumor cells (‘A’) occur early in tumorigenesis represented by the blue trunk of the phylogenetic tree, alterations shared by tumor cells present in some regions of the tumor but not all (‘B’ and ‘C’) occur later in tumorigenesis represented by the yellow branches of the tree, and private alterations (‘D’, ‘E’ and ‘F’) present in only one region of the tumor also occur later in tumorigenesis represented by the red branches of the tree.
Tumor Heterogeneity and Cancer Progression
In a recent study of CLL, Landau and colleagues used whole-exome sequencing and copy number analysis to identify clonal and subclonal driver mutations corresponding to CLL evolution (30). They found that the presence of a subclonal driver was an independent risk factor for disease progression, and those patients treated with cytotoxic chemotherapy were more likely to undergo clonal evolution, such that the extent of heterogeneity evolved during treatment. Studies like this indicate the likely relevance of tumor heterogeneity in predicting adverse outcomes, and the evolution of tumor subclonal composition during treatment. It may not always be the case that the dominant clone dictates tumor growth and malignant potential. Using a mouse xenograft model, Marusyk and colleagues demonstrated that subclones, without a known fitness advantage, could drive tumor growth in a non-cell-autonomous manner by inducing tumor-promoting changes in the microenvironment (33). Furthermore, non-cell-autonomous subclones could be outcompeted by other subclones with greater proliferative potential resulting in tumor collapse. Observations where minority subclones influence progression of the tumor mass suggest challenges for predictive and prognostic biomarker discovery efforts, that have traditionally focused on identifying genomic alterations in the dominant clone as well as the need to fully understand subclonal interactions within a complex ecological framework. The mechanisms, by which subclonal alterations have an impact on tumor biology and phenotype, are yet to be determined and will require further functional genomic studies. Such studies will need to investigate the interactions, both synergistic and antagonistic, between subclones during tumor evolution, and specifically how these relationships may promote or impede cancer progression, and contribute to therapeutic failure and drug resistance (12). Heterogeneity in the tumor microenvironment may also influence the evolution and progression of tumors. Interactions between tumor and stromal cells, changes in the level of hypoxia or acidity, increased or decreased inflammatory cell infiltrate, and remodeling of the extracellular matrix may act as selection pressures and lead to increased phenotypic heterogeneity, potentially influencing treatment response and therefore tumor evolution (for a review please refer to (13)).
Therapeutic Failure and Resistance
Heterogeneous tumors are composed of multiple subclones and under selection pressures, such as chemotherapy, subclones with either intrinsic or acquired resistance, can be selected for, allowing these subclones to dominate a tumor mass and potentially drive disease progression (34,35) (Fig. 3). The selection of resistant subclonal populations as a result of therapy leading to treatment resistance has been shown in several tumor types including lung (36,37), colorectal (38), gastrointestinal (39) and brain (40) tumors as well as chronic myeloid leukemia (41).
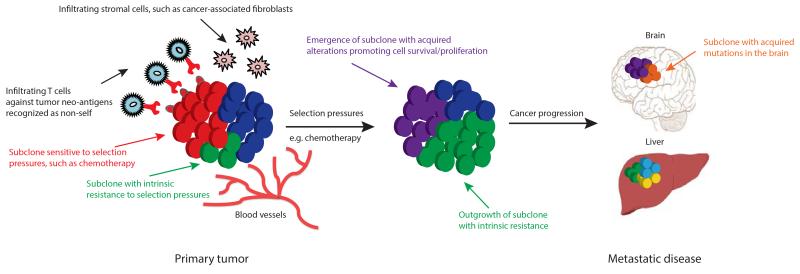
Intratumor heterogeneity and clonal evolution. Primary tumors consisting of different subclones may be subjected to various selection pressures including chemotherapy and microenvironmental factors such as hypoxia, infiltrating stromal and immune cells. Under the influence of such selection pressures, subclones with intrinsic resistance (green subclone) can outgrow a tumor mass potentially leading to disease progression, and/or subclones can acquire somatic alterations (purple subclone) promoting cell survival, proliferation and metastatic tumor formation. The outgrowth of some subclones (red subclone) may be constrained by selection pressures that they are sensitive to, for example, targeted therapy against a tumor subclone with a somatic alteration sensitive to therapy. Tumour infiltrating lymphocytes may recognize neo-antigens presented on the surface of tumor cells as non-self, promoting enhanced T-cell activation and immune cell tumor infiltration.
The mechanisms by which a tumor develops resistance may involve multiple somatic events affecting distinct signal transduction pathways, resulting in multiple distinct drug resistance events within the same tumour (42), which poses considerable challenges for the design and selection of effective drug combinations. However, there is evidence for phenotypic convergence within and across tumor types, suggesting that genetic events driving resistance and disease progression may focus on either one or several signaling pathways that may be therapeutically targetable (5,23,24,40,43-45).
Alternative therapeutic strategies may have the potential to overcome the challenges posed by clonal heterogeneity. Gatenby and colleagues have proposed the concept of adaptive therapy, whereby treatment-sensitive cells are initially targeted, resulting in the likely expansion of treatment-resistant cells that are subsequently targeted having predicted beforehand their likely mechanism of resistance (46). Knowledge of the existence of resistant subclones at diagnosis may allow for the use of combined therapeutic regimens targeting mechanisms of resistance. Evidence for the parallel evolution of subclones during tumour evolution suggests that there exist constraints to tumour growth that might be exploitable. If evolutionary “rule books” of tumor progression could be deciphered, by predicting the likely next-step in the evolution of the tumor, one might be able to devise preventative measures and potentially delay the onset of cancer progression (5). Such tactics will require a good evidence base from studies in different tumor types involving longitudinal tissue sampling, and therapeutic trial designs will need to take into account alternative approaches, such as adaptive therapy.
Host Immunity and Tumor Neo-antigens
The interplay between a tumor and the host immune system can help determine the immunogenicity of a tumor (47). The process of immunosurveillance can select for subclones lacking immunogenic antigens (48) or subclones with reduced sensitivity to immune attack (49) allowing for clonal evolution and tumor progression; a process termed immunoediting (50). Tumors can also create a microenvironment that actively counteracts the host anti-tumor immune response by employing suppressive mechanisms such as the expression of programmed cell death ligand 1 (PD-L1), the expression of indolamine 2,3-dioxygenase, secretion of anti-inflammatory mediators (i.e. transforming growth factor beta, TGF-β) or the induction of T cell anergy (51). Alternatively, tumor cells can attract cell types that suppress invading immune cells and support tumor growth and survival, such as regulatory T cells, tumor-associated macrophages (TAMs), cancer-associated fibroblasts (CAFs), and myeloid-derived suppressor cells (MDSCs). Immunotherapeutic drugs, such as monoclonal antibodies and cancer vaccines, have become a relevant player in the landscape of cancer therapies (52), and compared to conventional therapies, immunotherapy benefits from its ability to induce epitope spreading and immunological memory, which may significantly contribute in preventing disease relapse. In particular, the modulation of immune-regulatory checkpoints has recently been illustrated in clinical trials in which antibody-mediated blockade of the immune inhibitory receptors programmed cell death protein-1 (PD-1), programmed cell death ligand-1 (PD-L1) or cytotoxic T-lymphocyte-associated protein 4 (CTLA-4) produced significant clinical benefits in a variety of cancers, including metastatic melanoma (53-55).
Although evidence suggests that intratumor heterogeneity may limit the efficacy of conventional and targeted therapies, on account of factors such as sampling bias and clonal evolution (45), increased mutational diversity may lead to increased tumor neo-antigen production, and therefore potential new targets for immunotherapeutic drugs, such as immune checkpoint inhibitors (45,56-58). Somatic tumor mutations resulting in mutant short peptide fragments (neo-antigens) are presented on the tumour cell surface by the major histocompatibility complex (MHC), promoting activation and expansion of tumour infiltrating lymphocytes (TILs) (Fig. 3). TILs, in particular CD8+ T-cells, have been shown to be associated with improved treatment response and prognosis in several tumor types (59), including breast (60), ovarian (61-63), melanoma (64) and lung (65). The immunogenicity of a mutant peptide depends on its affinity for binding MHC class I ligands so that it can be presented to, and recognized by, CD8+ T cells (66). Conceivably, the greater the extent of intratumor heterogeneity and mutational burden, the greater the repertoire of potentially exploitable neo-antigens within a tumor (67). Using RNA-sequencing data for several tumor types from The Cancer Genome Atlas, Brown and colleagues identified immunogenic mutations predicted to result in mutational epitopes with high affinity for MHC class I ligands, and found that patients with predicted immunogenic mutations compared to those without had significantly improved overall survival (68). In a study of patients with melanoma treated with CTLA-4 blockade (ipilimumab or tremelimumab), Snyder and colleagues identified candidate tumor neo-antigens ifor each patient, and validated a neo-antigen signature associated with a strong treatment response (69). Such studies have the potential to use the neo-antigen landscape of heterogeneous tumors to predict immunotherapy response and therefore aid treatment stratification.
Insight into Tumor Heterogeneity Using “Liquid Biopsies”
Serial tumor sampling poses practical challenges and is currently not standard practice. An alternative approach may be the use of ‘liquid biopsies’, whereby circulating cell-free tumor DNA (cfDNA) or circulating tumor cells (CTCs) are analyzed in the peripheral blood of cancer patients. Such sampling may offer a relatively easy and non-invasive method for the analysis of primary and metastatic tumors (70). Several studies have demonstrated that genetic alterations in cfDNA are also present in matched tumors, and can be used to track mutational burden over time (10,28,71-74). Structural genomic rearrangements, such as translocations (75), as well as gene amplifications (76), have also been identified in cfDNA. In a study by Murtaza and colleagues, serial sampling and exome-sequencing of plasma in six patients with advanced-stage breast, ovarian and lung cancer was performed during several lines of therapy (71). By quantifying the allele frequencies of mutations in the plasma, they were able to correlate an increased representation of mutant alleles with emerging therapy resistance, including a T790M mutation following gefitinib therapy, a truncating mutation in mediator complex subunit 1 (MED1) following treatment with tamoxifen and trastuzumab, and a truncating mutation in RB1 (retinoblastoma 1) following treatment with cisplatin chemotherapy. Bettegowda and colleagues detected cfDNA in a broad spectrum of both early- and late-stage cancers in a total of 640 patients (10,77). Forty-seven percent of patients with stage I cancers of any type had detectable levels of cfDNA, indicating the use of cfDNA in early detection of cancer. In a subset of patients with colorectal cancer receiving anti-EGFR antibody therapy who subsequently relapsed, they were able to detect the emergence of several mutations in genes involved in the MAPK pathway, and therefore potential mechanisms of resistance, by genetically profiling cfDNA using digital polymerase chain reaction-based technologies. In a study by Diehl and colleagues, cfDNA was used to follow the course of therapy in patients with colorectal cancer (72). Fluctuations in cfDNA after surgery corresponded with the extent of surgical resection, and patients with a detectable cfDNA after surgery tended to relapse within 1 year. Compared to the standard biomarker CEA (carcinoembryonic antigen), cfDNA was found to be more reliable. Similarly, in a study by Dawson and colleagues, cfDNA was found to be a more reliable indicator of tumor burden compared to the standard biomarker CA 15-3 (carcinoma antigen 15-3) (74). Rothé and colleagues used next-generation sequencing of cancer gene panels to analyze matched primary and metastatic tumors as well as cfDNA samples in breast cancer patients (78). In 76% (13/17) of cases, mutations found in the cfDNA were concordant with the tumor, indicating that in some cases the cfDNA could be used as an alternative to metastatic tumor biopsies.
Similar to the studies in cfDNA, CTCs have also been used to study mutations and SCNAs (79-83), and have been shown to correlate with clinical outcome. In a large prospective study by Rack and colleagues, CTC enumeration in breast cancer patients was found to be an independent prognostic marker for disease-free survival (DFS) and overall survival (OS) (84). The worst prognosis was seen in patients with at least 5 CTCs per 30ml blood sample, and the presence of CTCs after cytotoxic chemotherapy was associated with a poorer DFS and OS. One of the advantages of studying CTCs is that next-generation sequencing can allow for the detection of intratumor heterogeneity in terms of driver mutations at single-cell resolution, for example heterogeneity in EGFR expression between CTCs in the same patient (85). Another advantage is the ability to study CTCs in the context of patient-derived mouse models. In a study by Hodgkinson and colleagues, CTCs were found to be tumorigenic in immune-compromised mice (82). CTCs derived from patients with small-cell lung cancer were implanted into mice resulting in CTC-derived explants (CDXs) (82). The genomic profiles of CTCs derived from patients and CDXs showed considerable similarity. Interestingly, CDXs reflected the donor patient’s response to platinum and etoposide chemotherapy, suggesting that such mouse models could be used for therapy testing and increase our understanding of potential resistance mechanisms.
However, although CDXs, as well as patient-derived tumor xenografts (PDTXs), may be effective tools in studying intratumor heterogeneity, there are caveats to consider (for a review please refer to (86)). In a study by Klco and colleagues, subclones derived from peripheral blood in AML patients were injected into immune-compromised mice (87). In order to assess the xenograft subclonal composition, human leukemic cells were purified from bone marrow xenografts using flow cytometry for subsequent whole-genome sequencing. All xenografts had a skewed subclonal composition where discreet subclones, despite a low frequency in the injected sample, defined the engrafted cell population resulting in unequal engraftment and/or growth potential, such that none of the xenografts had a subclonal composition that was identical to the input sample. The engrafted cell population may also be dependent on the mouse strain used, since immunophenotypic variability, based on flow cytometry using human antibodies to CD45, CD33 and/or CD34, was observed between different strains of mice having injected identical AML samples into them. It therefore follows that the interpretation of the clonal composition in PDXs requires some caution, since these models may not represent the genetic heterogeneity of the human-derived cell population and may not mirror the patient’s tumor clonal evolution in parallel longitudinal studies. In addition, one must take into account the potential for acquired mutations during the passage of human cells into immune-compromised mice (88).
Liquid biopsies have the potential to inform early detection of cancer, detect minimal residual disease, and track evolution of resistant disease and therefore detect early relapse (for a review please refer to (89)). Further evidence supporting the use of liquid biopsies to detect early stage cancer may inform cancer screening strategies, and allow the detection of tumors in early evolution. Such strategies could aid early therapeutic intervention prior to the onset of increased intratumor heterogeneity. In addition, they may be of use in circumstances where the biopsy of certain relapse sites, such as brain or bone, is not easily accessible. However, since cfDNA and CTCs may represent cancer genomes derived from multiple metastatic sites within the body, the interpretation of such biopsies may be confounded by intratumor heterogeneity (70). Whether whole-genome or -exome sequencing compared to targeted deep sequencing (90) is the preferred method, or exactly how representative liquid biopsies are of the underlying tumor landscape, and how sensitive they are in identifying clonal and subclonal alterations, is yet to be determined (91). Although not discussed in this review, it is worth noting that tumor DNA may also be detected and analyzed in samples such as urine, stool and circulating cerebrospinal fluid.
Implications for Precision Medicine and Clinical Trial Development
Given the evidence for spatial intratumor heterogeneity, and the evolution of tumor subclones with time and in response to cancer therapies, the potential sampling bias from a single tumor biopsy representing a snap shot in time, may limit the ability to identify and qualify biomarkers for clinical use. Ideally predictive biomarkers should be identified based on the genetic and phenotypic profile of each tumor, alongside the development of new therapies. Several recent studies are modeling such an approach, including the BATTLE-2 (NIH study trial registration number NCT01248247; clintrials.gov) and Lung-MAP (NIH study trial registration number NCT02154490; clintrials.gov) studies. In order to determine the true impact of intratumor heterogeneity on clinical outcome, future therapeutic studies aimed at delivering precision medicine may need to identify biomarkers in the context of tumor spatial heterogeneity. Since potential drivers of disease may vary during tumor evolution and treatment, and the presence of subclonal drivers may impact the efficacy of targeted therapies, potential drug combinations may need to address efficacy in the context of tumor clonal composition (92). In addition, bioinformatics analyses will need to capture low frequency driver events, which may predict for disease relapse and progression.
Taking multiple tumor biopsies to truly determine the clonal composition of tumors is not a simple or practical solution outside the context of clinical studies, but it is an effective approach in order to understand how tumors evolve during the disease course and in response to treatment. Longitudinal studies involving both tissue and liquid biopsies may allow us to develop cancer evolutionary “rule books” in an attempt to predict the disease course and likely beneficial therapeutic interventions. Such studies can also give us the opportunity to characterize exceptional or poor responders to treatment, which may be of benefit to patients with similar genetic or phenotypic patterns.
Whilst precision medicine has the potential to improve clinical outcome, reduce toxicity and increase cost effectiveness, it is not without its challenges including access to sequencing equipment and its associated cost implications, the development of clinical trials involving adequate tumor profiling to identify actionable alterations, the availability of targeted drugs and the validation of predictive and prognostic biomarkers. The appropriate infrastructure for such studies needs to be in place providing adequate computing resources and approved sequencing technologies. Clinical practice will need to move toward the implementation of genomics and detailed molecular characterization of a patient’s tumor in order to enable tailored therapies and attempt to improve patient outcome. Furthermore, clinicians will need to adapt to the challenges in analyzing such data, and the ethics of communicating increased risk or false-positive and false-negative results (93). Ultimately our aim should be to develop economically feasible predictive and prognostic biomarkers to aid clinical decision-making and improve risk stratification, and to work towards significant, as opposed to marginal, improvements in outcome using patient-centered measures such as quality-adjusted life-years. Possible approaches in achieving such goals have been reviewed in depth elsewhere (11).
Conclusions
Increasing evidence for intratumor heterogeneity and its potential impact on clinical outcome requires further exploration of the molecular mechanisms driving genomic instability and clonal evolution in cancer (94). Prospective genomic studies have the potential to allow us to gain a deeper insight into the true extent of tumor heterogeneity and how this evolves during the disease course and in response to therapeutic intervention. Furthermore, understanding the phylogenetic relationship between primary and metastatic tumors, and the significance of subclonal driver mutations, may allow clinicians to make informed therapeutic choices treating patients with drugs tailored to their tumor profile, taking into account tumor clonal composition. An example of such a study is TRACERx (TRAcking non-small cell lung Cancer Evolution through therapy (Rx), NIH study trial registration number NCT01888601; clintrials.gov), in which multi-region and longitudinal tumor sampling and sequencing is performed from diagnosis to relapse, in order to define the genomic landscape of tumors, and to understand the impact of tumor clonal heterogeneity on therapeutic and survival outcome (95). Tumors within TRACERx are genetically profiled to identify the most immunogenic and targetable mutational epitopes, and to determine the relationship between the extent of intratumor heterogeneity and TILs, and therefore clinical outcome. In determining whether circulating biomarkers can truly represent the tumor clonal composition, this study also involves the serial sampling of cfDNA and CTCs at different disease time-points, and in doing so explores the use of circulating biomarkers in tracking clonal evolution, detecting minimal residual disease and early relapse. In light of emerging evidence that driver mutations may be subclonal in some tumors, there is a need to define the relationship between driver clonality and benefit from targeted therapy exposure in prospective clinical studies, such as the DARWIN (Deciphering Anti-tumour Response With INtratumour Heterogeneity, NIH study trial registration number NCT02183883; clintrials.gov) clinical trials programme, which may help determine the impact of subclonal drivers on therapeutic response, disease relapse and progression (32,95).
Collaborations between industry, scientists and clinicians are required to meet the challenges posed by future clinical trials designed to address intratumor heterogeneity. Such studies are likely to require repeated tissue and blood sampling and infrastructural support addressing financial, technological and regulatory aspects to trial design in light of complex subclonal architectures. With the integration of large cohort clinical and genomic data, and the development of bioinformatics analyses of such large datasets, the delivery of precision medicine has the potential to become a reality with the ultimate aim of improving patient outcomes.
Acknowledgments
M. Jamal-Hanjani is a Cancer Research UK Clinical Research Fellow. S.A. Quezada is a Cancer Research UK Career Development Fellow. J. Larkin is supported by the Royal Marsden Hospital/Institute of Cancer Research and the National Institute for Health Research Biomedical Research Centre for Cancer. C. Swanton is a senior Cancer Research UK Clinical Research Fellow and is supported by Cancer Research UK, the National Institute for Health Research University College London Hospitals Biomedical Research Centre for Cancer, the Rosetrees Trust, EU FP7 (projects PREDICT and RESPONSIFY, ID: 259303), the Prostate Cancer Foundation, the European Research Council, and the Breast Cancer Research Foundation.
Footnotes
Disclosure of Potential Conflicts of Interest
No potential conflicts of interest were disclosed.
References
Full text links
Read article at publisher's site: https://doi.org/10.1158/1078-0432.ccr-14-1429
Read article for free, from open access legal sources, via Unpaywall:
https://clincancerres.aacrjournals.org/content/clincanres/21/6/1258.full.pdf
Citations & impact
Impact metrics
Citations of article over time
Alternative metrics
Smart citations by scite.ai
Explore citation contexts and check if this article has been
supported or disputed.
https://scite.ai/reports/10.1158/1078-0432.ccr-14-1429
Article citations
Metabolic Reprogramming of Cancer-Associated Fibroblast in the Tumor Microenvironment: From Basics to Clinic.
Clin Med Insights Oncol, 18:11795549241287058, 21 Oct 2024
Cited by: 0 articles | PMID: 39450056 | PMCID: PMC11500237
Review Free full text in Europe PMC
Multiregional transcriptomic profiling provides improved prognostic insight in localized non-small cell lung cancer.
NPJ Precis Oncol, 8(1):225, 05 Oct 2024
Cited by: 0 articles | PMID: 39369068 | PMCID: PMC11455871
Finding potential targets in cell-based immunotherapy for handling the challenges of acute myeloid leukemia.
Front Immunol, 15:1460437, 30 Sep 2024
Cited by: 0 articles | PMID: 39411712 | PMCID: PMC11474923
Review Free full text in Europe PMC
The role of DNA methylation and DNA methyltransferases (DNMTs) as potential biomarker and therapeutic target in non-small cell lung cancer (NSCLC).
Heliyon, 10(19):e38663, 27 Sep 2024
Cited by: 0 articles | PMID: 39403460 | PMCID: PMC11472108
Review Free full text in Europe PMC
Assessing muscle invasion in bladder cancer via virtual biopsy: a study on quantitative parameters and classical radiomics features from dual-energy CT imaging.
BMC Med Imaging, 24(1):245, 16 Sep 2024
Cited by: 0 articles | PMID: 39285354 | PMCID: PMC11403826
Go to all (287) article citations
Data
Data behind the article
This data has been text mined from the article, or deposited into data resources.
BioStudies: supplemental material and supporting data
Clinical Trials (4)
- (1 citation) ClinicalTrials.gov - NCT02154490
- (1 citation) ClinicalTrials.gov - NCT01248247
- (1 citation) ClinicalTrials.gov - NCT01888601
- (1 citation) ClinicalTrials.gov - NCT02183883
Similar Articles
To arrive at the top five similar articles we use a word-weighted algorithm to compare words from the Title and Abstract of each citation.
Characterizing genetic intra-tumor heterogeneity across 2,658 human cancer genomes.
Cell, 184(8):2239-2254.e39, 07 Apr 2021
Cited by: 216 articles | PMID: 33831375 | PMCID: PMC8054914
Intratumor heterogeneity: evolution through space and time.
Cancer Res, 72(19):4875-4882, 20 Sep 2012
Cited by: 557 articles | PMID: 23002210 | PMCID: PMC3712191
Review Free full text in Europe PMC
Deciphering intratumor heterogeneity using cancer genome analysis.
Hum Genet, 135(6):635-642, 28 Apr 2016
Cited by: 17 articles | PMID: 27126234
Review
Quantitative Clinical Imaging Methods for Monitoring Intratumoral Evolution.
Methods Mol Biol, 1513:61-81, 01 Jan 2017
Cited by: 15 articles | PMID: 27807831
Review
Funding
Funders who supported this work.
Cancer Research UK (6)
Translational Cancer Therapeutics
Professor Charles Swanton, Cancer Research UK London Research Institute
Grant ID: 19310
Translational Cancer Therapeutics
Professor Charles Swanton, Cancer Research UK London Research Institute
Grant ID: A11590
Defining the mechanisms underpinning the function of regulatory and effector tumour-reactive CD4+ T cells during tumorigenesis: applications to cancer immunotherapy
Professor Sergio Quezada, University College London
Grant ID: 12100
CRUK/14/020: Tracking Cancer Evolution through Therapy (Tracer X)
Professor Charles Swanton, University College London
Grant ID: 17786
CRUK/14/020: Tracking Cancer Evolution through Therapy (Tracer X)
Professor Charles Swanton, University College London
Grant ID: A17786
Translational Cancer Therapeutics
Professor Charles Swanton, Cancer Research UK London Research Institute
Grant ID: A19310
National Institute for Health Research (NIHR)
Rosetrees Trust (1)
Grant ID: M179
The Francis Crick Institute (1)
Grant ID: 10169