Abstract
Free full text

Target identification and mechanism of action in chemical biology and drug discovery
Abstract
Target-identification and mechanism-of-action studies have important roles in small-molecule probe and drug discovery. Biological and technological advances have resulted in the increasing use of cell-based assays to discover new biologically active small molecules. Such studies allow small-molecule action to be tested in a more disease-relevant setting at the outset, but they require follow-up studies to determine the precise protein target or targets responsible for the observed phenotype. Target identification can be approached by direct biochemical methods, genetic interactions or computational inference. In many cases, however, combinations of approaches may be required to fully characterize on-target and off-target effects and to understand mechanisms of small-molecule action.
Modern chemical biology and drug discovery each seek to identify new small molecules that potently and selectively modulate the functions of target proteins. Historically, nature has been an important source for such molecules, with knowledge of toxic or medicinal properties often long predating knowledge of precise target or mechanism. Natural selection provides a slow and steady stream of bioactive small molecules, but each of these molecules must perforce confer reproductive advantage in order for nature to ‘invest’ in its synthesis. In recent decades, investments in finding new small-molecule probes and drugs have expanded to a paradigm of screening large numbers (typically 103–106) of compounds for those that elicit a desired biological response1,2. In some cases, these studies interrogate natural products3,4, but more often they involve collections of synthetic small molecules prepared by organic chemistry strategies5,6 that rapidly yield large collections of relatively pure compounds. Thus, the overall discovery paradigm involves investments both in organic synthesis and in biological testing of large compound collections.
Since the revolution in molecular biology, the biological testing component of screening-based discovery has overwhelmingly involved testing compounds for effects on purified proteins. However, with advances in assay technology, many research programs are increasingly turning (or returning) to cell- or organism- based phenotypic assays that benefit from preserving the cellular context of protein function7. The cost paid for this benefit is that the precise protein targets or mechanisms of action responsible for the observed phenotypes remain to be determined. Even after a relevant target is established, additional functional studies may help to identify unwanted off-target effects or establish new roles for the target protein in biological networks. In this review, we address these important steps in the discovery process, termed target identification or deconvolution8, illustrating methods available to approach the problem, highlighting recent advances and discussing how findings from multiple approaches are integrated.
Background
Historically, genetics has provided powerful biological insights, allowing characterization of protein function by manipulation of genetic sequence. A forward genetics (or classical genetics) approach is characterized by identifying, often under experimental selection pressure, a phenotype of interest, followed by identification of the gene (or genes) responsible for the phenotype (see refs. 9, 10). Modern molecular biological methods, particularly genetic engineering approaches, have given rise to reverse genetics (sometimes equated with molecular genetics), in which a specific gene of interest is targeted for mutation, deletion or functional ablation (for example, with RNAi11), followed by a broad search for the resulting phenotype (see refs. 12, 13).
By analogy to genetics, there are two fundamental approaches to understanding the action of small molecules on biological systems14,15. Biochemical screening approaches are analogous to reverse genetics (Fig. 1a). In advance of conducting a high-throughput screen, the protein target is selected and (typically) purified before exposure to small molecules16,17. This target validation or credentialing is a time-consuming process that involves demonstrating the relevance of the protein for a particular biological pathway, process or disease of interest18,19. Once a target has been validated, it is presumed that binders or inhibitors of this protein will affect the desired process. Often, however, such an impact needs to be characterized more completely in cells or animals by observing compound-induced phenotypes; hence, this approach has been termed reverse chemical genetics.
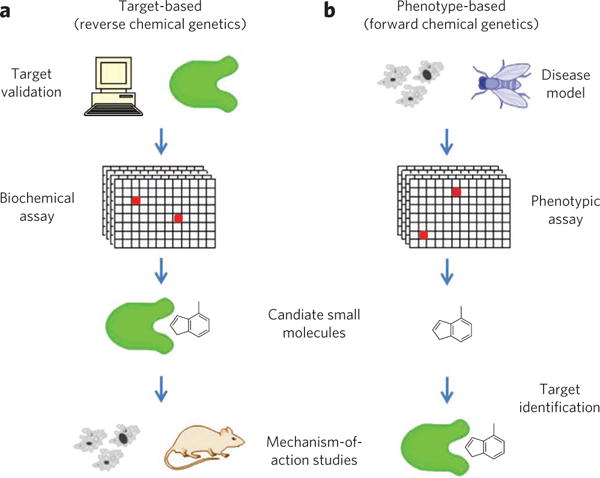
(a) Target-based approaches (reverse chemical genetics) begin with target validation, in which a role is established for a protein in a pathway or disease, followed by a biochemical assay to find candidate small molecules; mechanism-of-action studies are still required to validate cellular activities of candidates and evaluate possible side effects. (b) Phenotype-based approaches (forward chemical genetics) begin with a phenotype in a model system and an assay for small molecules that can perturb this phenotype; candidate small molecules must then undergo target-identification and mechanism-of-action studies to determine the protein responsible for phenotypic change.
In contrast, forward chemical genetics refers to the process of testing small molecules directly for their impact on biological processes, often in cells or even in animals (Fig. 1b)20,21. Phenotypic screens expose candidate compounds to proteins in more biologically relevant contexts than screens involving purified proteins7,22. Because these screens measure cellular function without imposing preconceived notions of the relevant targets and signaling pathways, they offer the possibility of discovering new therapeutic targets. Indeed, several important drug programs have been inspired by phenotypic screening results, including the effects of cyclosporine A and FK506 on T-cell receptor signaling7,23, leading to the discoveries of FKBP12 (ref. 24), calcineurin25 and mTOR26, and the performance of trapoxin A in differentiation and proliferation assays27, leading to the discovery of histone deacetylases28,29. Importantly, such assays ‘prevalidate’ the small molecule and its (initially unknown) protein target as an effective means of perturbing the biological process or disease model under study.
However, phenotypic assays require a subsequent effort to discover the molecular targets of bioactive small molecules, which can be a complex endeavor30. We often assume that direct interaction with a single target is responsible for phenotypic observations, but this need not be the case. Many drugs show side effects owing to interactions with ‘off-target’ proteins31,32, and even small molecule–induced phenotypes observed in cell culture may represent the superposition of effects on multiple targets33,34. For drug development, target identification is important to follow-up studies, aiding medicinal chemistry efforts. Furthermore, identifying both the therapeutic target and other targets that might cause unwanted side effects enables optimization of small-molecule selectivity35. Conversely, polypharmacology can be considered a new tool, leveraging multiple small-molecule effects to gain maximal effect, once again underscoring the benefit of an unbiased approach to screening and the need for target deconvolution36.
Approaches to target identification
In this review, we will cover three distinct and complementary approaches for discovering the protein target of a small molecule: direct biochemical methods, genetic interaction methods and computational inference methods. Direct methods involve labeling the protein or small molecule of interest, incubation of the two populations and direct detection of binding, usually following some type of wash procedure (reviewed in ref. 37). Genetic manipulation can also be used to identify protein targets by modulating presumed targets in cells, thereby changing small-molecule sensitivity (reviewed in ref. 38). Target hypotheses, in contrast, can be generated by computational inference, using pattern recognition to compare small-molecule effects to those of known reference molecules or genetic perturbations39–41. Mechanistic hypotheses, rather than targets per se, for new compounds emerge from such tests. The target pathway or protein of a new small molecule is inferred but remains to be confirmed42. Similarly, hypotheses regarding the mechanism of action of a compound can be generated by gene expression profiling in the presence or absence of compound treatment. Many target-identification projects actually proceed through a combination of these methods, where researchers use both direct measurements and inferences to test increasingly specific target hypotheses. Indeed, we suggest that the problem of target identification will not generally be solved by a single method but rather by analytical integration of multiple, complementary approaches.
Direct biochemical methods
Biochemical affinity purification provides the most direct approach to finding target proteins that bind small molecules of interest37. Because they are based on physical interactions involving mammalian or human proteins, biochemical methods can lead to information about molecular mechanisms of efficacy or toxicity highly relevant to human disease. Similarly, small-molecule optimization efforts can be complemented by biochemical methods when three-dimensional structure information about the target is known. Finally, unbiased protein identification, especially from lysates containing intact protein complexes, potentially allows evaluation of polypharmacology.
Pioneering work in affinity purification involved monitoring chromatographic fractions for enzyme activity after exposure of extracts to compound immobilized on a column, followed by elution43. In general, such an approach requires large amounts of extract, possibly prefractionated, and stringent wash conditions. Such approaches have been used with success to identify certain protein targets, including those of both natural29 and synthetic44 small molecules, and one such approach has been used to elucidate the mechanism of the teratogenic side effect of thalidomide35. However, these methods seem best suited for situations where a high-affinity ligand binds a relatively abundant target protein. High-stringency washes can bias proteins identified to those with the highest-affinity interactions, decreasing the likelihood of finding additional targets that might be important in cellular contexts, as suggested by proteomic profiling studies (for example, those described in refs. 45, 46). Furthermore, stringent washing will also reduce the ability to identify protein complexes in which the direct target participates and whose members’ identities might help illuminate the connection between the direct target and the cellular activity of the small molecule.
Affinity purification experiments also involve the challenge of preparing immobilized affinity reagents that retain cellular activity, so that target proteins will still interact with the small molecule while it is bound to a solid support. A related issue is the identification of appropriate controls and tethers. Different approaches are possible; control beads loaded with an inactive analog47,48 or capped without compound have been used49. These control experiments have limitations, most notably the availability of related inactive compounds. The inactive analog must be sufficiently different from the compound of interest to fail to bind the target, raising the possibility that it will have different physicochemical properties and therefore different nonspecific interactions with proteins. In the case of capped beads, the results are confounded by the background of high-abundance, low-affinity proteins with slight differential binding to the control bead. Elution from the bead-bound small molecule24 or preincubation of lysate with compound is a viable alternative control35,49,50 but is limited by compound solubility. The choice of tether, influencing the type of background proteins identified, also becomes a critical parameter51–55. These challenges can frustrate individual attempts at affinity purification, particularly if a biochemical approach is used in isolation.
Recent affinity-based methods have attempted to overcome one or more of these challenges. Approaches based on chemical or ultraviolet light–induced cross-linking56,57 use covalent modification of the protein target to increase the likelihood of capturing low-abundance proteins or those with low affinity for the small molecule. This method requires prior knowledge of the enzyme activity being targeted, making it a slightly biased approach. If there is no bias, cross-linking of the small molecule to proteins is burdened with the possibility of high, nonspecific background owing to the cross-linking event itself. A variation on the use of photoaffinity reagents couples covalent modification to two-dimensional gel electrophoresis in an attempt to deconvolve nonspecific interactions58. A nonselective universal coupling method that enables attachment to a solid support by a photoaffinity reaction resulted in the identification of an inhibitor of glyoxalase I59. This method assumes that a compound could bind the solid support in multiple ways while some functional relevant sites remain available to interact with the protein target. This approach, however, runs the risk of false-negative results when the functional group is masked in the coupling reaction. Another method for immobilizing small molecules, that is, coupling them to peptides that allow them to recover the probe-protein complex by immunoaffinity purification60, was devised to address this issue.
Some small-molecule libraries are prepared with synthetic handles primed for making affinity matrices after an activity is identified61,62. Because these methods rely on advance modification of the compound structure, they require additional chemistry expertise and may not be possible for all compound classes. Similarly, if a small molecule can be fluorescently labeled, it can be used to probe proteins separated by microarray (reviewed in ref. 63). However, such methods are limited to proteins that can be readily manipulated, usually in heterologous expression systems. The use of purified proteins does not necessarily ensure physiological expression levels, giving incorrect information about relative binding to alternative targets in cells and masking effects due to the formation of protein complexes.
Two interesting new target-identification approaches that circumvent the need to immobilize compounds have emerged in the last few years. One of them uses changes in protein susceptibility to proteolytic degradation upon small-molecule binding (reviewed in ref. 64). The other is based on a characteristic shift in the chromatographic retention-time profile after a compound binds a protein target65. Although the generality of these approaches remains to be determined, their combination with quantitative proteomics is quite promising.
Affinity chromatography has been coupled to powerful new techniques in MS, which can possibly provide the most sensitive and unbiased methods of finding target proteins. Quantitative proteomics66 (reviewed in ref. 67) has been effective in identifying specific protein-protein interactions by affinity methods68,69 and has been increasingly applied to protein–small molecule interactions (reviewed in ref. 70). In the context of target identification, two different quantitative techniques have been used; these can be broadly divided into metabolic and chemical labeling.
Metabolic labeling, more specifically stable-isotope labeling by amino acids in cell culture (SILAC)71, has been effectively used, in experiments with gentle washing and free soluble competitor pre-incubation of lysates, to provide unbiased assessment of multiple direct and indirect targets (Fig. 2)49. It has also been used in combination with serial drug-affinity chromatography to characterize the quantitative changes of the kinome in different phases of the cell cycle72. Metabolic labeling has the advantage of allowing sample pooling early in the process, eliminating quantification errors due to sample handling. A disadvantage is that it limits the workflow to immortalized cell lines73.
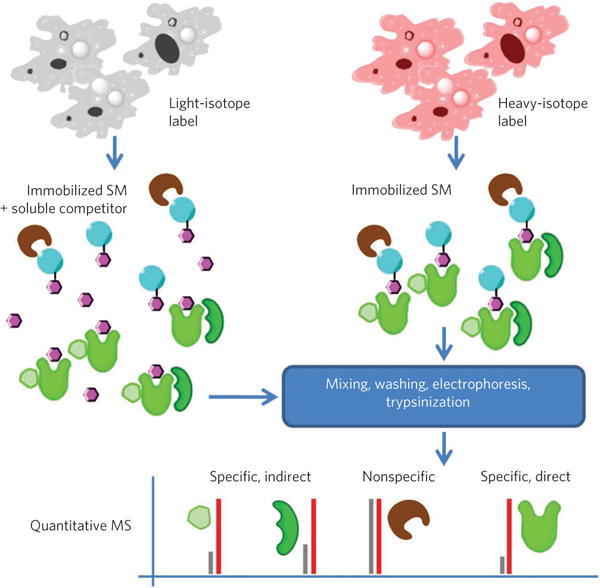
Cells are labeled with either heavy- or light-isotope labels. One sample is exposed to bead-immobilized small molecules (SM) in the presence of soluble competitor compound and the other in the absence of competitor. Following mixing, washing and electrophoresis, samples are digested using trypsin and peptide fragments analyzed by quantitative MS49. Ratios of heavy- and light-labeled peptides are used to determine specificity of interactions for the small molecule, potentially including both direct and indirect targets (for example, members of complexes including the direct target), but not to differentiate them.
Chemical labeling has also been successfully used in the past. Isotope-coded affinity tag (ICAT) technology74, coupled with beads loaded with active and inactive compound as controls, has been used to identify malate dehydrogenase as a specific target of the anticancer compound E7070 (ref. 47). Isobaric tags for relative and absolute quantification (iTRAQ)75, coupled with free soluble competitor for elution as a control, has been used to profile kinases enriched by affinity purification with nonselective kinase-binding small molecules76. There are variations on chemical-labeling strategies, such as mass differential tags for relative and absolute quantification (mTRAQ)75, tandem mass tags (TMT)77 and stable isotope dimethyl labeling78,79. These chemical labeling strategies provide more versatility regarding the type of samples that can be labeled, but a weakness lies in the fact that they generally rely on labeling at the peptide level, later in the proteomic workflow, and thus are prone to more variation and less accuracy80.
These unbiased approaches show great promise but will require new software81–83 and analytical techniques84 to quantify the relative expression of members of candidate target lists to aid in experimental prioritization. In contrast, biased approaches to target deconvolution based on profiling small-molecule activity against a panel of enzymes are now commercially available and widely used. These methods rely on prior knowledge of the enzyme family (kinases, ubiquitinases, demethylases and so on). For example, assay-performance profiling and compounds with a known mode of action were used to predict kinase inhibitory activity for new compounds emerging from a phenotypic screen42. A commercial kinase profiling panel85 then confirmed activity against a subset of kinases. Having information from genetic studies or computational methods to decide which set of enzymes to investigate provides a valuable tool, again underscoring the importance of integrating all methods at the researcher’s disposal.
Genetic interaction and genomic methods
Target identification based on genetic or genomic methods leverages the relative ease of working with DNA and RNA to perform large-scale modifications and measurements. These methods often use the principle of genetic interaction, relying on the idea of genetic modifiers (enhancers or suppressors) to generate target hypotheses. Gene knockout organisms, RNAi (reviewed in ref. 86) and small molecules with well-defined mechanisms can each be used to alter the functions of putative targets, uncovering dependencies on activity. For example, if a gene knockdown phenocopies a compound’s effects, the evidence that the protein could be the target relevant to that phenotype would be strengthened. In this case, hypersensitivity of individual mutants to sublethal concentrations of compound demonstrates a chemical-genetic interaction (Fig. 3a). Similarly, mating of laboratory and wild yeast strains can reveal patterns of small-molecule sensitivity with specific genetic loci (Fig. 3b)87.
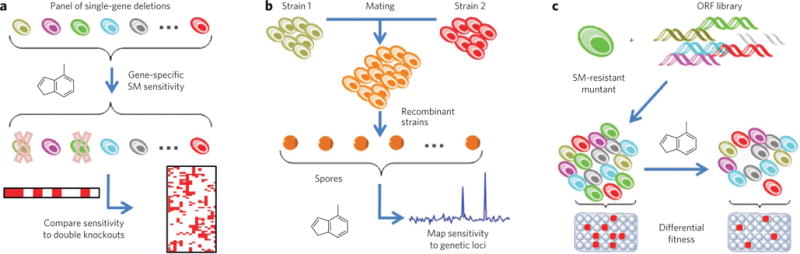
(a) A panel of viable single-gene deletions is tested for small-molecule (SM) sensitivity, indicating synthetic-lethal interactions between potential targets and the original deletion; mechanisms are interpreted by comparing interactions to double-knockout strains146. (b) Different strains of diploid yeast are mated to form F1 recombinants, and meiotic progeny are subjected to small molecules; segregation frequencies allow mapping of small-molecule sensitivity to genetic loci147. (c) A recessive small molecule–resistant mutant is transformed with a wild-type open reading frame library; transformants obtaining a wild-type copy of the mutant gene are selectively sensitive to small molecules, resulting in their depletion among pooled transformants, as quantified by microarray89.
These concepts have been expanded to include molecularly barcoded libraries of open reading frames88 and detection of small molecule–resistant clones by microarray (Fig. 3c). Importantly, these studies provide a conceptual framework for understanding complex diseases. Although direct translation to human biology may not be forthcoming, owing to the lack of conservation of some pathways between yeast and human, this framework enables us to envision technical advances that facilitate this type of analysis in mammalian systems. An accompanying perspective in this series89 addresses these approaches in more detail.
A promising genetics-based technique for target identification involves combining results from small-molecule and RNAi perturbations. This approach enables parallel testing of small-molecule and RNAi libraries for induction of the same cellular phenotype90,91. RNAi experiments can be performed on a genome-wide scale to find phenotypes similar to those induced by small molecules (Fig. 4a)92. Alternatively, when some mechanistic clues already exist, a more focused set of RNAi reagents can be used to find pathway members that alter the effects of a small molecule (Fig. 4b)93. The main strength of combining small-molecule and RNAi perturbations is the ability to measure phenotypic effects in more physiologically relevant cellular contexts, using mammalian, or even human, cells. Clearly, genetic perturbations cannot always recapitulate or phenocopy the effect of a small molecule94, for example because of the risk of genetic compensation. Using RNAi libraries that contain entire families of genes may help alleviate this problem. Another way to dissect these effects is to use a suboptimal concentration of small molecule in combination with genetic knockdown. An elegant study provided proof of concept for RNAi-sensitized small-molecule screens95, which could serve as a follow-up method for the other techniques described in this review.
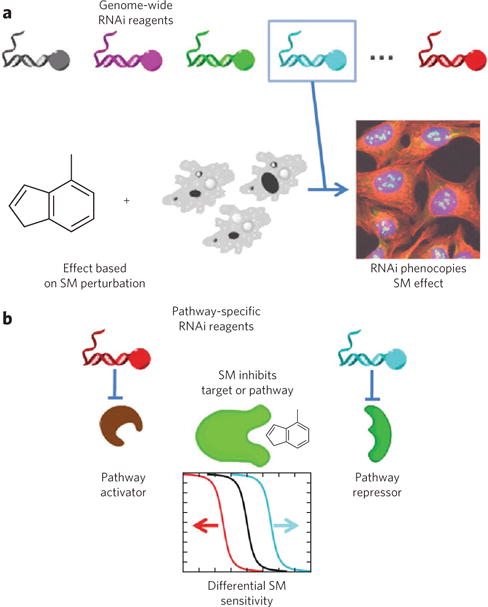
(a) In one implementation, phenotypes from genome-wide RNAi are compared to those induced by a small molecule (SM) of interest; full or partial phenocopy of the small-molecule effect by RNAi provides evidence that the gene product is a small-molecule target92. (b) When prior evidence suggests a particular target pathway, focused sets of RNA reagents can help to generate mechanistic hypotheses. In general, RNAi can enhance or suppress small-molecule effects, as in genetic epistasis analysis; in practice, more complex relationships among proteins than those illustrated may also exist93.
Genetic target-identification efforts are increasingly focused on mammalian cells. For example, examination of compound-resistant clones of cells, using transcriptome sequencing (RNA-seq), identified intracellular targets of normally cytotoxic compounds96. Advantages of this approach include the ability to perform cell type–specific analyses and not having to chemically modify the compound to perform the analysis. Clones of HCT116 colon cancer cells resistant to the polo-like kinase 1 (PLK1) inhibitor BI 2356 were sequenced and compared to the parental line. Although PLK1 was not mutated in every clone, it was the only gene mutated in more than one group; moreover, mutations were present in the known binding site of BI 2356. This proof-of-principle study may pave the way for more rapid target identification in mammalian cells, although this approach is currently limited to cell viability as a phenotype.
Finally, recent efforts to use gene expression signatures to determine compounds’ mechanisms of action illustrate the close relationship between genetic techniques and computational methods. Using transcription profiling data from the Connectivity Map97, a recent study described a weighting scheme to rank order lists of genes across multiple cell lines, resulting in what was termed a prototype ranked list98. The authors then used gene-set enrichment analysis99 to compute pairwise distances between the ranked lists for each compound and constructed a network in which each compound was a node. Clustering revealed communities of nodes connected to each other, two-thirds of which were enriched for similar mechanisms of action, as determined by anatomical therapeutic chemical codes. A limitation of this approach is the reliance on accurate annotation of small-molecule activity, but even so, this type of sophisticated approach will most likely uncover similarities between known bioactive compounds and new screening hits. The line separating genetic and computational approaches increasingly blurs as the technical hurdles to generating massive data sets are surmounted.
Computational inference methods
On their own, computational methods are used to infer protein targets of small molecules, in addition to providing analytical support for proteomic and genetic techniques. These methods can also be used to find new targets for existing drugs, with the goal of drug repositioning or explaining off-target effects. Profiling methods rely on pattern recognition to integrate results of parallel or multiplexed experiments, typically from small-molecule phenotypic profiling100,101. Ligand-based methods also incorporate chemical structures to predict targets. Structure-based methods rely on three-dimensional protein structures to predict protein–small molecule interactions102,103. Owing to their limitation to proteins with known structures, structure-based methods will not be discussed in detail.
In general, bioactivity profiling methods are based on the principle that compounds with the same mechanism of action will have similar behavior across different biological assays. Public databases of, for example, gene expression97,104 or small-molecule screening105,106 data sets house measurements that record phenotypic consequences of small-molecule perturbations. Target or mechanism inference frequently relies on the fact that molecules with known mechanisms of action (sometimes called landmark compounds100) are also profiled, allowing new compounds to be assessed for similar patterns of performance107,108. Small-molecule profiling data can be mined most effectively when there exists a critical mass of data generated using a common set of compounds and cell states (multidimensional screening109). A prototypical example of this type of study is the US National Cancer Institute’s ‘NCI-60’ set of 60 cancer cell lines that was exposed to a large collection of small molecules110. In a seminal study using the NCI-60 data set, protein targets were connected (via their degree of expression in each cell line) to small-molecule sensitivity patterns in the same cell lines39 (reviewed in ref. 101). Similar studies, including those using the COMPARE algorithm110, have allowed researchers to hypothesize new activities for small molecules111. Such approaches represented important conceptual advances in this field because they considered multidimensional profiling as a method to identify small-molecule mechanisms of action.
Gene expression technology has also had an important role in profile-based inference of small-molecule targets and mechanisms. One early study used gene expression profiles of yeast treated with the immunosuppressants FK506 and cyclosporine A. This study not only recapitulated the importance of calcineurin in immunosuppression but also identified a number of other genes that were suggested to be secondary targets112. Later, a compendium of yeast gene expression profiles was derived from both genetic mutations and small-molecule perturbations to annotate uncharacterized genes and pathways40. Commercial databases of rat tissue–based expression profiles following drug treatment were developed to identify toxicities of new chemical entities113. Subsequently, the Connectivity Map was developed as a public database of gene expression profiles derived from small-molecule perturbations of human cell lines (Fig. 5a), which enables the functions of new small molecules to be identified by pattern matching97.
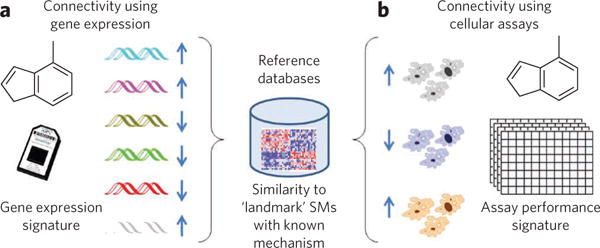
In general, data sets that provide multidimensional readouts of small molecule (SM)-induced phenotypes can be used to provide connections between new small-molecule signatures and reference databases by similarity to ‘landmark’ compounds with known mechanisms of action.
Several groups have developed profiling methods based on other measurement technologies114,115, including affinity profiling of biochemical assays to predict ligand binding to proteins116, and on similarities among side-effect profiles117. Affinity profiling was also used to design biologically diverse screening libraries118. Several promising studies have involved profiling by high-throughput microscopy (sometimes called high-content screening), allowing phenotypes to be clustered, in a manner analogous to transcriptional profiles, to discover potential small-molecule targets119,120. More generally, public databases such as ChemBank105 or PubChem106 provide extensible environments for accumulating rich phenotypic profiles for many compounds exposed to a diverse set of assays and have been exploited to understand both bioinformatic121 and cheminformatic101,122 relationships (Fig. 5b).
More focused profiling methods investigate only particular aspects of biological systems. For example, high-throughput screening (HTS) activity profiling and a guilt-by-association approach were used to determine mechanisms of action for hits in an antimalarial screen123. Another study124 examined small molecule–induced cell death and systematically characterized lethal compounds on the basis of modulatory profiles, created experimentally by measuring cell viability. Comparison of modulatory profile clusters with gene expression– and structure-based profiles demonstrated that that modulatory profiles revealed additional relationships. Bioactivity profile similarity search (BASS) is another method that uses profiles of dose-response cell-based assay results to associate targets with small molecules125. It is increasingly likely that single measurements will be inadequate to the task of determining mechanism of action.
Recent profiling methods have taken full advantage of high-throughput data available in public and proprietary screening databases, for example by developing HTS fingerprints (HTS-FP) with a goal of facilitating virtual screening and scaffold hopping126. Enrichment of gene ontology terms among known protein targets was observed within HTS-FP clusters. Further, HTS-FP can be used to select biologically diverse compounds for screening when testing the full library is not possible.
Using ligand-based predictive modeling to classify compounds is a well-established practice in computational chemistry. Independently, two groups have extended predictive-modeling approaches to explore global relationships among biological targets. In one case127, protein-ligand interactions were explored with a goal of predicting targets for new compounds. Using Laplacian-modified naive Bayesian modeling, trained on 964 target classes in the WOMBAT database, the researchers were able to predict the top three most likely protein targets and validated their approach by examining therapeutic activities of compounds in the MDL Drug Data Report database. Such modeling can also be used to explain off-target effects or to design target- or family-focused libraries. In a separate but similar approach128, a large collection of structure-activity relationship data was assembled from various public and proprietary sources, resulting in a set of 836 human genes that are targets of small molecules with binding affinities less than 10 μM. These connections were used to generate a polypharmacology interaction network of proteins in chemical space. This network enables a deeper understanding of compound and target cross-reactivity (promiscuity) and provides rational approaches to lead hopping and target hopping.
Chemical similarity is often used as a metric of success for pattern-recognition approaches. The similarity ensemble approach (SEA)129 works by quantitatively grouping related proteins on the basis of the chemical similarity of their ligands. For each pair of targets, chemical similarity was computed and properly normalized according to a null model of random similarity. Even though only ligand chemical information was used, biologically related clusters of proteins emerged. Importantly, in the SEA results, there were cases of ligand-based clusters differing from protein sequence–based clusters; for example, ion channels and G protein–coupled receptors are ligand related, but they have no structure or sequence similarity. In contrast, many neurological receptors with related sequences lack pharmacological similarity. The authors documented the utility of SEA by predicting and confirming off-target activities of methadone, loperamide and emetine. Large-scale prediction of off-target activities using SEA was subsequently described130.
SEA is well suited to provide insights into the mechanisms of action of small molecules. With that purpose in mind, SEA was used on the MDL Drug Data Report database to predict new ligand-target interactions131. The predictions were either confirmed by literature or database search or investigated experimentally. Out of 30 experimentally tested predictions, 23 were confirmed. This work was extended to ‘de-orphan’ seven US Food and Drug Administration–approved drugs with unknown targets132. SEA has also been used to predict targets of compounds that were active in a zebrafish behavioral assay133. Out of 20 predictions that were tested experimentally, the authors confirmed 11, with activities ranging from 1 nM to 10 μM. These results suggest that chemical information alone can be sufficient to make predictions using this approach. Additional ligand-based target-identification methods have been reported (reviewed in ref. 103).
Network-based approaches extend systems biology to drug-target and ligand-target networks and are now known as ‘systems chemical biology’134, ‘network pharmacology’135 or ‘systems pharmacology’136. Such approaches are necessary, as many phenotypes are caused by effects of compounds on multiple targets. An exploration of the relationship between drug chemical structures, target protein sequences and drug-target network topology resulted in the creation of a unified ‘pharmacological space’ that could predict ligand-target interactions for new compounds and proteins137. Several additional studies report recent advances in network-based approaches to target prediction138,139.
Inference-based methods arguably have the least bias of any target-identification method as they often rely on experiments done by others. The analyst is distant from the original experimental design and is therefore poised to reveal unanticipated relationships. In contrast, such studies rely on data sets that require substantial time and investment, however distributed, to realize. Fortunately, computational techniques have increasingly emerged to take advantage of the explosion of public data sources. Structured publicly accessible databases are the best hope for the success of such methods; they not only promote the availability of data from multiple sources but also encourage rigor among experimentalists by exposing their data to critical evaluation by the scientific community at large.
Summary and outlook
Each of the described approaches to target-identification and mechanism-of-action studies has strengths and limitations, and, importantly, different laboratories will have different technical strengths. Laboratories with expertise in chemistry or biochemistry might gravitate toward direct biochemical approaches, whereas genetic or cell biology laboratories might favor genetic interaction approaches, and groups with a high degree of computational experience might first pursue methods that investigate databases for clues. Of course, there is no ‘right answer’ about which method is best. Rather, we suggest that a combination of approaches93,140 is most likely to bear fruit (Fig. 6).
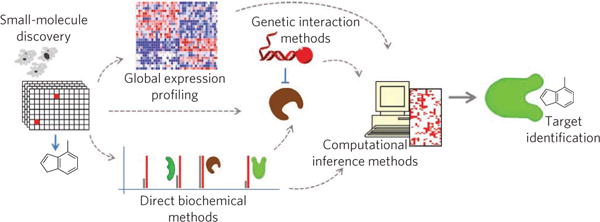
Small-molecule discovery often starts with phenotypic screening, and, depending on the expertise available to the researchers, target identification could proceed using any combination of direct biochemical methods, genetic or genomic methods or using computational inference methods. A key component for success is the integration of data from all available methods to produce the most reliable target and mechanistic hypotheses.
There have been a few examples of successful integration of different methods to help determine the mechanism of action of a small molecule. An integrated approach was used to characterize the ability of a well-known natural product, K252a, to potentiate Nrg1-induced neurite outgrowth141. Integrating quantitative proteomic results with a lentivirus-mediated loss-of-function screen to validate candidate target proteins, the authors found that knockdown of AAK-1 reproducibly potentiated Nrg-1–driven neurite outgrowth. Similarly, the mechanism by which another natural product, piperlongumine, selectively kills cancer cells was determined by a combination of direct proteomic affinity-enrichment and short hairpin RNA methods142.
To determine whether bortezomib neurotoxicity could be attributed to off-target effects, secondary targets of the proteasome inhibitors bortezomib and carfilzomib were explored143. The authors used a multifaceted approach, involving screening a panel of purified enzymes, activity-based probe profiling in cells and cell lysates and mining a database of known and predicted proteases, to show that bortezomib inhibits several serine proteases and to hypothesize that its neurotoxicity may be caused by off-target effects.
Computational data integration and network analysis were instrumental in determining Aurora kinase A as a relevant target of dimethylfasudil in acute megakaryoblastic leukemia144. Dimethylfasudil is a broad-spectrum kinase inhibitor; integrating quantitative proteomics data, kinase inhibition profiling and RNA-silencing data allowed the researchers to generate a testable hypothesis which not only identified the physiologically relevant target of dimethylfasudil but also a potential therapeutic target for acute megakaryoblastic leukemia. Another elegant application of integrating transcriptional data with proteomic data was used to dissect the synergy between two multikinase inhibitors in chronic myelogenous leukemia cell lines145. A combination of proteomic methods to measure drug binding in cell lysates, global phosphoproteomics and genome-wide transcriptomics demonstrated synergy between danusertib and bosutinib in chronic myelogenous leukemia cells harboring the BCR-ABLT315I gatekeeper mutation.
These very recent examples show how powerful the ability to effectively and systematically integrate large sets of disparate data will be in understanding the molecular mechanisms of a small molecule in biological systems. When done in a disciplined and thoughtful manner, such data integration represents a modern instantiation of the scientific method, relying on high-throughput technology, data integration and multidisciplinary approaches to provide clues and avenues to new targets and mechanisms of small-molecule action.
Acknowledgments
This work was supported by US National Institutes of Health Genomics Based Drug Discovery–Target ID Project grant RL1HG004671, which is administratively linked to the US National Institutes of Health grants RL1CA133834, RL1GM084437 and UL1RR024924.
References
Full text links
Read article at publisher's site: https://doi.org/10.1038/nchembio.1199
Read article for free, from open access legal sources, via Unpaywall:
https://www.ncbi.nlm.nih.gov/pmc/articles/PMC5543995
Citations & impact
Impact metrics
Citations of article over time
Alternative metrics
Smart citations by scite.ai
Explore citation contexts and check if this article has been
supported or disputed.
https://scite.ai/reports/10.1038/nchembio.1199
Article citations
A spatial hierarchical network learning framework for drug repositioning allowing interpretation from macro to micro scale.
Commun Biol, 7(1):1413, 30 Oct 2024
Cited by: 1 article | PMID: 39478146 | PMCID: PMC11525566
Total glucosides of Picrorhizae Rhizome alleviate non-alcoholic steatohepatitis (NASH) by specifically targeting acyl-CoA oxidase 1.
Heliyon, 10(21):e39874, 26 Oct 2024
Cited by: 0 articles | PMID: 39524810 | PMCID: PMC11550611
Annonaceous acetogenins mimic AA005 targets mitochondrial trifunctional enzyme alpha subunit to treat obesity in male mice.
Nat Commun, 15(1):9100, 22 Oct 2024
Cited by: 0 articles | PMID: 39438446 | PMCID: PMC11496682
CACTI: an in silico chemical analysis tool through the integration of chemogenomic data and clustering analysis.
J Cheminform, 16(1):84, 24 Jul 2024
Cited by: 0 articles | PMID: 39049122 | PMCID: PMC11270953
Machine learning in preclinical drug discovery.
Nat Chem Biol, 20(8):960-973, 19 Jul 2024
Cited by: 4 articles | PMID: 39030362
Review
Go to all (424) article citations
Other citations
Data
Similar Articles
To arrive at the top five similar articles we use a word-weighted algorithm to compare words from the Title and Abstract of each citation.
The why and how of phenotypic small-molecule screens.
Nat Chem Biol, 9(4):206-209, 01 Apr 2013
Cited by: 56 articles | PMID: 23508174
Target validation using chemical probes.
Nat Chem Biol, 9(4):195-199, 01 Apr 2013
Cited by: 196 articles | PMID: 23508172
Targeting RNA structures in diseases with small molecules.
Essays Biochem, 64(6):955-966, 01 Dec 2020
Cited by: 14 articles | PMID: 33078198 | PMCID: PMC7724634
Review Free full text in Europe PMC
Identification and validation of bioactive small molecule target through phenotypic screening.
Bioorg Med Chem, 20(6):1922-1928, 20 Nov 2011
Cited by: 12 articles | PMID: 22153994
Funding
Funders who supported this work.
NCI NIH HHS (2)
Grant ID: RL1CA133834
Grant ID: RL1 CA133834
NCRR NIH HHS (2)
Grant ID: UL1RR024924
Grant ID: UL1 RR024924
NHGRI NIH HHS (2)
Grant ID: RL1HG004671
Grant ID: RL1 HG004671
NIGMS NIH HHS (2)
Grant ID: RL1 GM084437
Grant ID: RL1GM084437