Abstract
Free full text

PNAS Plus
MAP kinase and autophagy pathways cooperate to maintain RAS mutant cancer cell survival
Associated Data
Significance
Currently, there is no effective targeted therapy for oncogenic KRAS-driven cancer. We set out to identify RAS effector and stress response genes that critically support KRAS addiction, and therefore could serve as potential targets for KRAS mutant cancer. Using a combinatorial siRNA platform, we systematically interrogated the patterns of oncoeffector dependency in KRAS mutant and WT colorectal and pancreatic cancer cell lines and in normal cell lines. We found that RAF kinases are the major KRAS oncoeffectors and that cotargeting BRAF and CRAF kinases together with the autophagy E1 ligase ATG7 could efficiently eliminate KRAS mutant cells while minimizing toxicity in normal cells. Our work thus establishes a framework for the rational selection of target combinations for cancer treatment.
Abstract
Oncogenic mutations in the small GTPase KRAS are frequently found in human cancers, and, currently, there are no effective targeted therapies for these tumors. Using a combinatorial siRNA approach, we analyzed a panel of KRAS mutant colorectal and pancreatic cancer cell lines for their dependency on 28 gene nodes that represent canonical RAS effector pathways and selected stress response pathways. We found that RAF node knockdown best differentiated KRAS mutant and KRAS WT cancer cells, suggesting RAF kinases are key oncoeffectors for KRAS addiction. By analyzing all 376 pairwise combination of these gene nodes, we found that cotargeting the RAF, RAC, and autophagy pathways can improve the capture of KRAS dependency better than targeting RAF alone. In particular, codepletion of the oncoeffector kinases BRAF and CRAF, together with the autophagy E1 ligase ATG7, gives the best therapeutic window between KRAS mutant cells and normal, untransformed cells. Distinct patterns of RAS effector dependency were observed across KRAS mutant cell lines, indicative of heterogeneous utilization of effector and stress response pathways in supporting KRAS addiction. Our findings revealed previously unappreciated complexity in the signaling network downstream of the KRAS oncogene and suggest rational target combinations for more effective therapeutic intervention.
In response to extracellular stimuli, the RAS family of small GTPases serves as a signaling nexus to transmit mitogenic signal from growth factor receptors to their intracellular effector pathways, which, in turn, regulate a variety of cellular processes, including cell proliferation, survival, motility, and gene expression (1). Oncogenic mutations in RAS genes are frequently detected in human cancers. Among the three RAS family members NRAS, HRAS, and KRAS, KRAS accounts for the majority of RAS mutations in solid tumors (~90% pancreatic, ~50% colorectal, and ~30% lung adenocarcinomas). Direct inhibition of the KRAS oncoproteins has proved challenging, with only the KRASG12C mutant being tractable thus far (2). As an alternative strategy, inhibitors targeting RAS effectors, many of which are druggable kinases, have been a major focus in blocking oncogenic RAS signaling (3). Inhibitors for RAS effector kinases, including RAF, MEK, PI3K, and AKT, have demonstrated impressive antitumor activities in preclinical studies (4, 5). However, they have not delivered significant efficacy against KRAS mutant cancers either as monotherapies or in combination settings in clinical trials (6, 7). This may be attributable to at least two reasons. First, since RAS signals through multiple pathways, oncogene addiction to mutant KRAS could be functionally distributed across multiple effectors. Thus, KRAS mutant cells could use multiple effector pathways to maintain their proliferation and survival advantage. Consequently, inhibiting a single RAS effector may be insufficient to kill KRAS mutant cells (8). Second, some RAS effector pathways, including the MAP kinase (MAPK) and PI3K pathways, also play an important role for the proliferation and survival of normal stem and progenitor cells in the body (9, 10). Shutting off these pathways using potent inhibitors often introduces significant toxicity in normal tissues, which could limit the therapeutic window (11–16).
To identify more effective strategies for targeting RAS effectors, it is important to distinguish oncogenic signaling by mutant KRAS from that of normal, physiological signaling by wild-type (WT) KRAS protein (1, 8). We hypothesize that a subset of RAS effectors, which we term “oncoeffectors,” could play a more critical role in mediating KRAS oncogene addiction than physiological RAS signaling. We reason that pinpointing these oncoeffectors and selectively targeting them could reduce toxicity in normal cells. In addition to oncogene addiction, cancer cells driven by KRAS and other oncogenes experience extensive oncogenic stress, a phenomenon we previously conceptualized as nononcogene addiction (17). We hypothesize that inhibiting cellular stress response pathways that are critical for the survival of KRAS mutant cells could also serve as an effective therapeutic strategy. Furthermore, it stands to reason that cotargeting RAS effector pathways and stress response pathways may lead to greater loss of survival signaling, and thus enhance the killing of KRAS mutant cells (18, 19).
Previously, we and others have carried out extensive genome-wide shRNA and CRISPR library screens to identify functional vulnerabilities in KRAS mutant cells (20–26). Collectively, these works revealed two somewhat unexpected findings. The first was that no universal synthetic lethal partners of KRAS have been identified. This indicates that the pattern of nononcogene addiction in KRAS mutant cells is highly dependent on context, and it is likely that no single stress response pathway is responsible for alleviating oncogenic stress in all KRAS mutant cells (3, 24). The second observation was that few canonical RAS effectors were recovered as consistent dependencies in KRAS mutant cells (24, 25). This is attributable to several reasons. First, as we previously noted, the utilization of different RAS effector pathways to support KRAS addiction could be heterogeneous across different KRAS mutant cell lines (27), resulting in no consistent oncoeffector dependency. Second, and as mentioned above, KRAS addiction might be functionally distributed across multiple pathways. Thus, no single effector gene could dominate the KRAS dependency phenotype. The third reason could be due to gene paralog redundancy. In the mammalian genome, many gene nodes in the RAS pathway consist of multiple gene paralogs that often have some degree of functional redundancy. For example, we and others have shown that the three RAF family genes that constitute the RAF kinase node, ARAF, BRAF, and RAF1 (CRAF), could partially compensate for each other’s loss to sustain MAPK signaling in human cells (28–30). Because current RNAi and CRISPR library screens are primarily single-gene–dependency assays, they would fail to uncover the function of gene families with redundant paralogs.
Our goal is to understand how KRAS addiction is functionally distributed across its various downstream effector pathways and across various stress response pathways. To do so, we need to cotarget multiple genes simultaneously in the same cell to gain a systems-level view of how gene paralog redundancy and pathway cooperativity contribute to KRAS addiction. Because conventional RNAi and CRISPR screens are mostly limited to interrogating single-gene activity, we overcame this limitation by developing a combinatorial siRNA platform to simultaneously cotarget up to seven different genes in the same cell (29). Using this approach, we recently showed that, at the single effector node level, different KRAS mutant cell lines exhibit different degrees of effector node dependency, and the subset of KRAS mutant lines that are less dependent on KRAS exhibit enhanced dependency on the p90RSK kinase node (27).
In this current study, we extended our combinatorial siRNA analysis to interrogate higher order gene combinations that cotarget gene node pairs and gene combinations from two or more pathways. Studies of this type have not been previously attempted in KRAS mutant cells. Since combination therapy involving drugs with orthogonal mechanisms of action has become increasingly necessary to achieve meaningful benefit in patients, our study should directly inform the rational design of target combination. We discovered that the RAF kinase node, particularly the BRAF and CRAF paralogs, are key oncoeffectors of KRAS addiction. We further demonstrated that whereas the RAC and autophagy pathways each contribute little to KRAS dependency alone, cotargeting these pathways together with the RAF node could significantly enhance the killing of KRAS mutant cells. Extensive gene paralog deconvolution identified BRAF, CRAF, and ATG7 as a target combination that provides the best discrimination between KRAS mutant cells and normal, untransformed cells. Our study therefore provides a systems biology approach to rationally evaluate target combination in KRAS mutant cells.
Results
Curation of a Sensor siRNA Library for RAS Effector and Stress Response Genes.
We wished to examine how KRAS addiction is partitioned through canonical RAS effectors, including the MAPK, PI3K, RHO, RAC, RAL, and PLCe pathways. Similar to our recent report (27), we selected 19 gene nodes in these six pathways that consist of 47 genes and paralogs. To understand how nononcogene addiction contributes to KRAS addiction, we included in our analysis 10 gene nodes consisting of 26 genes and paralogs that correspond to indirect RAS effectors and stress response pathways that could contribute to nononcogene addiction in KRAS mutant cells (SI Appendix, Fig. S1A). Thus, our analysis included a total of 73 genes representing 29 discrete gene nodes. To identify KRAS oncoeffectors and stress response genes that are critical in mediating KRAS addiction, we sought to access the dependency profiles of KRAS mutant cells for various combinations of these genes using siRNA-mediated multigene knockdown. Previously, we established a sensor siRNAs technology platform to curate highly potent siRNAs that can work reliably in combination to achieve efficient knockdown of up to seven gene targets simultaneously with minimal cross-interference or off-target effects (29). Because many gene nodes consist of two to four paralogs, our platform thus enables us to interrogate the cell’s dependency on multiple gene nodes. To construct a library of sensor siRNAs targeting these 73 genes, we screened a large number of candidate sensor shRNAs for each gene and identified highly potent sensor siRNA sequences that could maintain >70% target mRNA knockdown at a low concentration in a pool with three to six other highly potent siRNAs (27, 29, 31). This ensures that these siRNAs could reproducibly knock down their targets in a combinatorial setting. To reduce potential siRNA off-target effects, we curated two independent sensor siRNAs with similar knockdown efficiency for all but six genes in our library (SI Appendix, Fig. S1B). Using these two sets of siRNAs, we constructed two parallel libraries containing the same gene combinations (set 1 and set 2 libraries, respectively; SI Appendix, Fig. S1E). Overall, >80% of the siRNAs gave >80% target mRNA knockdown (SI Appendix, Fig. S1C), and there is comparable knockdown efficiency between set 1 and set 2 siRNAs (SI Appendix, Fig. S1D). Thus, phenotypic concordance between corresponding siRNA pools from the two libraries would suggest an siRNA on-target effect, whereas phenotypic discordance would suggest an siRNA off-target effect.
Taking advantage of the higher order siRNA combination platform we have developed, we constructed a combinatorial siRNA library to systematically interrogate these RAS effector and stress response genes for their cooperative role in supporting KRAS addiction. The siRNA pools in the library were designed to interrogate gene interactions at two levels of complexity. To overcome gene paralog redundancy, we constructed 29 siNode pools targeting each of the 29 gene nodes included in this study. Each siNode pool consists of siRNAs targeting all gene paralogs for the nodes. To examine gene node and pathway interactions, we constructed 406 siNodePair pools that represent all pairwise combinations of the 29 gene nodes. Each siNodePair pool consists of siRNAs targeting all gene paralogs in the two gene nodes being targeted (SI Appendix, Fig. S1E and Dataset S1).
Single-Node Dependency Analysis Identifies RAF Kinases as a Key Oncoeffector Node.
We first investigated how each siNode pool impacted cell viability in a panel of colorectal cancer (CRC) cell lines consisting of five KRAS mutant (DLD-1, HCT116, SW620, LoVo, and SW403) and two KRAS WT (SW48 and Caco-2) lines. As we previously reported (27, 29), the KRAS mutant lines were dependent on KRAS and sensitive to KRAS knockdown by two independent, validated KRAS siRNAs (SI Appendix, Fig. S2A). In contrast and as expected, the KRAS WT lines were not sensitive to KRAS knockdown. The set 1 and set 2 siNode libraries were independently tested in these cell lines (SI Appendix, Fig. S2A). For a given siNode pool, the two libraries gave concordant results (SI Appendix, Fig. S2B), although we noticed that set 1 siRNAs tended to have a slightly stronger effect in general. Outlier analysis comparing the phenotypic distance between the two libraries identified the siNode pool for hexokinases to likely contain siRNA off-target effects (SI Appendix, Fig. S2C). We therefore excluded the hexokinase siRNAs from all subsequent analyses.
To identify KRAS oncoeffector nodes whose knockdown most closely phenocopy siKRAS, we performed unsupervised hierarchical clustering using averaged cell viability data from set 1 and set 2 siRNAs (Fig. 1A). Several patterns in the data became salient. First, the siBCL2 pool, which targets multiple prosurvival BCL-2 family members, clustered with the siDeath-positive control. This indicates that shutting down BCL-2 family function could be generally toxic irrespective of the cell’s genotype. Second and somewhat surprisingly, many siNode pools showed relatively little toxicity in these cell lines. This could be due either to a lack of dependency on these nodes or to the fact that these siRNAs, despite our best effort, were unable to knock down target genes at a sufficiently deep level to reveal their dependency. Third, only the siRAF node closely clustered with KRAS siRNAs and showed a similar sensitivity profile among KRAS mutant cells. This indicates RAF is the most critical oncoeffector node that mediates oncogenic KRAS signaling.
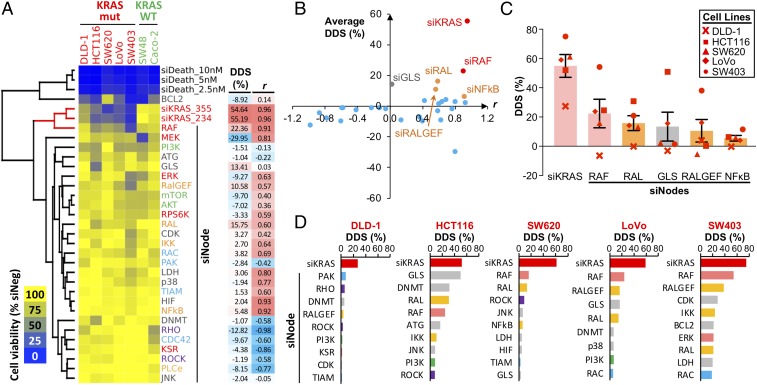
Single-node dependency in KRAS mutant cells. (A) Cell viability data of single-node knockdown in CRC cell lines. Cell viability was measured 5 d post-siRNA transfection and was normalized to the siNeg control for the respective cell line. Average viability data for set 1 and set 2 siNode libraries were clustered based on Euclidean distance. Dendrogram branches involving siKRAS are highlighted in red. For each siNode, the averaged DDS and r metrics with siKRAS are shown next to the viability heat map. (B) Scatter plot of averaged DDS vs. r metrics to visualize the distance between various siNodes and the siKRAS-positive control. (C) Top five public single-node dependencies based on the average DDS. Bars represent average DDS metrics across five KRAS mutant cell lines (error bars represent SEM in all figures unless otherwise stated). (D) Top private single-node dependency for each cell line. Single-node dependency was ranked based on the cell line-specific DDS. siKRAS was included as a positive control.
To quantitatively measure how much of KRAS dependency (as indicated by the effect of siKRAS) is captured by each siNode and to reduce data complexity, we established two simple metrics from aggregated data analysis. The differential dependency score (DDS; range: −100 to 100%) for a KRAS mutant line represents the viability difference between the mean viability of all KRAS WT cell lines and that of the KRAS mutant line. A positive DDS indicates that an siRNA pool has greater toxicity in the KRAS mutant line compared with KRAS WT cells. Conversely, a negative DDS indicates greater toxicity in KRAS WT cells. A DDS of 0% means the siRNA pool has no differential toxicity between KRAS WT and mutant cells. The correlation r (range: −1 to 1) is the Pearson correlation between all KRAS mutant cell lines’ sensitivity to an siNode and that to siKRAS. Thus, an oncoeffector is expected to score high for both DDS and r. Plotting DDS vs. r for the 28 siNode pools revealed that the majority of them were relatively distant from siKRAS, which resides in the upper right quadrant (Fig. 1B). This analysis suggested RAF, RAL, RALGEF, and NF-κB nodes as oncoeffector nodes with a positive DDS and r (Fig. 1 B and C and SI Appendix, Fig. S3 A and B). Among these four, the RAF node had substantially stronger metrics for both the DDS (Fig. 1C) and r (SI Appendix, Fig. S3A). However, the RAF node was only able to capture less than 50% of KRAS dependency in mutant cells (Fig. 1C). The glutaminase node (GLS) also scored a positive DDS. A closer examination of its r score, however, showed poor correlation with siKRAS because the phenotype was primarily driven by strong toxicity in one cell line, HCT116 (SI Appendix, Fig. S3C). Thus, our analysis indicates that the RAF node is a major oncoeffector downstream of mutant KRAS, although no single node is able to fully capture KRAS dependency.
Somewhat unexpectedly and unlike the RAF node, the MEK and ERK nodes did not score highly in our initial analysis. Both DDS and r metrics for the MEK and ERK nodes were lower than those of the RAF node (SI Appendix, Fig. S3 A, D, and E). One possibility would be that the MEK and ERK siNode pools were less potent than the RAF siNode pool. We compared the knockdown efficiency of these siNodes for their cognate targets and for their ability to reduce the level of phospho-ERK in cells. As expected, all siNode pools effectively and consistently knocked down their target proteins in multiple KRAS mutant and WT cell lines (SI Appendix, Fig. S4A). Furthermore, all RAF, MEK, and ERK siNode pools had a comparable inhibitory effect on phospho-ERK levels (SI Appendix, Fig. S4B). These results suggest that the effect of RAF, MEK, and ERK siNode pools cannot be attributed to differences in their ability to down-regulate MAPK pathway activity. To exclude the possibility that the toxicity of siRNAs in the pools was due to off-target effects, we performed rescue experiments using sequence-specific C911 control siRNAs that corresponded to set 1 siRNAs in these pools (32). As shown in SI Appendix, Fig. S4C, replacing all on-target siRNAs with their C911 counterparts rescued cell viability in sensitive cell lines. Thus, the higher toxicity of RAF siRNAs in KRAS mutant cells is likely a result of their on-target effects. We noticed that one of the KRAS WT cell lines, SW48, is sensitive to MEK knockdown (Fig. 1A). SW48 cells harbor a MEK1Q56P-activating mutation (33–35), and this could confound our analysis. We thus repeated the clustering analysis and the calculation of DDS and r metrics by leaving out the SW48 dataset (SI Appendix, Fig. S4D). This improved the scoring for the MEK node but not for the ERK node. However, the MEK node still had a substantially lower DDS than the RAF node in the ranking of top oncoeffectors (SI Appendix, Fig. S4E). Thus, our analysis indicates that KRAS mutant cells are more dependent on the RAF node than the MEK and ERK nodes, and that RAF could present a better target than MEK and ERK within the MAPK pathway.
Similar to our previous report (27), we observed a wide range in sensitivity to KRAS knockdown among the KRAS mutant CRC cell lines (Fig. 1D). When DDS metrics were calculated and ranked for individual KRAS mutant cell lines, we saw a heterogeneous pattern of single-node dependency (Fig. 1D). For example, SW403 cells are highly sensitive to the knockdown of the RAF node, whereas HCT116 cells are uniquely dependent on glutaminase. In DLD-1, SW620, and LoVo cells, no dominant node dependencies were observed. This finding suggests that different KRAS mutant cancer cell lines could utilize different pathways to support KRAS addiction.
Paired-Node Dependency Analysis Reveals RAF Node Combinations Best Capture KRAS Dependency.
Next, we assessed the sensitivity of KRAS mutant and WT CRC cell lines to all 378 siNodePair pools (excluding the hexokinase node) to investigate the impact of knocking down two nodes together. Cell viability responses to set 1 and set 2 siNodePair libraries were mostly concordant (SI Appendix, Fig. S5 A and B), suggesting that higher order siRNA combinations did not introduce substantially more off-target effects. Outlier analysis identified two node-pair combinations, RHO + ROCK and RHO + TIAM, to show significant disagreement between set 1 and set 2 siRNAs (SI Appendix, Fig. S5C). They were excluded from subsequent analysis.
We applied the aforementioned analysis pipeline to identify node pairs that best phenocopied the effect of siKRAS. Unsupervised hierarchical clustering of viability data identified several distinct clusters (Fig. 2A). Notably, the subset of siNodePair combinations that clustered closest to siKRAS consisted exclusively of RAF node combinations (Fig. 2B). DDS and r metrics confirmed the top-ranked node combinations were all RAF node combinations (Fig. 2C). Several RAF node combinations had a higher DDS than the RAF node alone; these include RAF in combination with the RAC, RAL, ROCK, and ATG nodes (Fig. 2D). Node-pair combinations involving RAC, RAL, ROCK and ATG, but not RAF, did not score. This finding supports the notion that the RAF node is the most critical oncoeffector in KRAS mutant cells and suggests that cotargeting RAF with a second effector node could generate better therapeutic efficacy.
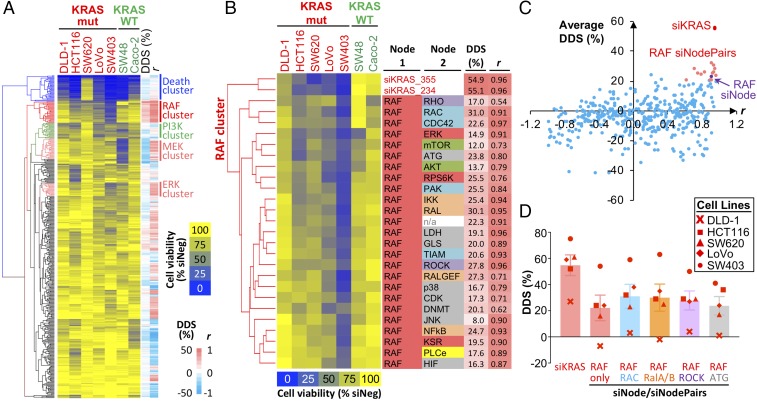
Paired-node dependency in KRAS mutant cells. (A) Cell viability data of node-pair knockdown in CRC cell lines. Cell viability was measured 5 d post-siRNA transfection and was normalized to the siNeg control for the respective cell line. Average viability data for set 1 and set 2 siNodePair libraries, as well as the viability data from the single-node analysis, were combined and clustered based on Euclidean distance. For each siNodePair, the averaged DDS and r metrics are shown next to the viability heat map. Notable clusters of node pairs consisting of the RAF, PI3K, MEK, and ERK nodes, respectively, are highlighted. (B) More detailed view of the RAF cluster in A showing the inclusion of siKRAS and siRAF in this cluster, together with various node pairs involving RAF. (C) Scatter plot of the averaged DDS vs. r to visualize the distance between various siNodePairs and the siKRAS-positive control. The RAF siNode is highlighted in purple, and siNodePairs involving RAF are highlighted in red. (D) Top five public single-node dependencies based on average DDS metrics. Bars represent average DDS metrics across five KRAS mutant cell lines.
In addition to the RAF node cluster, the analysis revealed other clusters in which the node combinations share similar dependency profiles. Clustering closest to the siDeath-positive control were the BCL-2 node combinations (SI Appendix, Fig. S6A). These combinations are likely to be generally toxic regardless of the cell’s KRAS genotype. Two RAF node combinations, RAF + PI3K and RAF + MEK, appeared in this Death cluster but not in the main RAF node cluster. This suggests that cotargeting two essential pathways (RAF + PI3K) or the deep inhibition of one essential pathway (RAF + MEK) could reduce the combination’s selectivity. This is in agreement with the increased toxicity of MAPK and PI3K inhibitor combinations seen in clinical studies (12–16). We noted a discrete PI3K node cluster that showed no correlation with KRAS status (SI Appendix, Fig. S6B). Interestingly, the cell lines sensitive to PI3K node combinations correlated with their PI3K mutation status: The five sensitive cell lines harbor mutations in the PIK3CA or PIK3CB genes (DLD-1, PIK3CAE545K; HCT116, PIK3CAH1047R; LoVo, PIK3CBE1051K; SW403, PIK3CAQ546K; and SW48, PIK3CAG914R), whereas the two insensitive cell lines, SW620 and Caco-2, are WT cell lines for these genes. Thus, distinct patterns of dependencies can be determined by co-occurring driver mutations.
Our analysis showed that MEK and ERK node combinations did not cluster close to siKRAS (Fig. 2A and SI Appendix, Fig. S6 C and D). To rule out the confounding effect of the MEK1 mutant SW48 cell line, we removed the SW48 dataset and repeated the DDS and r calculations (SI Appendix, Fig. S7A). The results confirmed that RAF node combinations were superior at capturing KRAS dependency compared with MEK and ERK node combinations. In this latter analysis, the top-ranked RAF node combinations captured about 70–80% of KRAS dependency, whereas the top MEK and ERK node combinations only captured 40–50% of KRAS dependency (SI Appendix, Fig. S7B).
When we calculated and ranked the siNodePair DDS for each individual KRAS mutant cell line, we made two notable observations. First, similar to single-node dependency, node-pair dependency exhibited significant heterogeneity across different cell lines. Within a given cell line, the top-scoring node-pair combinations were predominantly driven by the top-scoring single node (SI Appendix, Fig. S8). Second, we found that in some cell lines, including DLD-1 and HCT116, their respective top-scoring node-pair combinations were able to capture nearly all KRAS dependency, albeit such combinations were cell line-specific (SI Appendix, Fig. S8). This analysis further highlighted the heterogeneous nature of effector pathway dependency across different KRAS mutant cell lines. Thus, analogous to the “public” and “private” sensitivity of cancer cell lines to single-agent small molecule inhibitors (36), the public target combinations that work for the majority of KRAS mutant cell lines may not be the best private combination for any given cell line.
Identification of BRAF, CRAF, and ATG7 as the Minimal Oncoeffector Combination That Best Discriminates KRAS Mutant Cancer Cells and Normal Cells.
We selected four of the top-scoring RAF node combinations for further validation using set 1 siRNAs. These include RAF in combination with the RAC, RAL, ROCK, and ATG nodes (Fig. 2D). Whereas the first three nodes represent interpathway combinations among RAS effectors, the last one represents combination with a stress response pathway that is known to genetically interact with the KRAS oncogene (37, 38). We expanded our analysis to include several pancreatic ductal adenocarcinoma (PDAC) cell lines consisting of five KRAS mutant lines (MIA PaCa-2, Hup-T4, SUIT-2, AsPC-1, and PA-TU-8902) and one KRAS WT line (BxPC-3). In these 13 CRC and PDAC cell lines, combined knockdown of RAF with the RAC, RAL, and ATG nodes all led to greater toxicity in KRAS mutant cells compared with RAF node knockdown alone (SI Appendix, Fig. S9A). DDS and r metrics for these combinations showed that the RAF + ATG node combination had a higher DDS compared with the RAF node alone (Fig. 3A), while all three node combinations retained good r scores compared with the RAF node alone (SI Appendix, Fig. S9 B and C). To further validate the on-target effect of these siRNA combinations, we performed rescue experiments using sequence-specific C911 control siRNAs for each siRNA in the pool. As expected, replacing all on-target siRNAs with their C911 counterparts abolished the toxicity of the siRNA pools (SI Appendix, Fig. S9D). This confirmed that the effects of the siRNA combinations were likely on-target effects. Taken together, these results indicate that KRAS addiction is mediated through multiple effector and stress response pathways, and that the gene node combinations we identified could reflect common pathway dependencies among KRAS mutant cells.
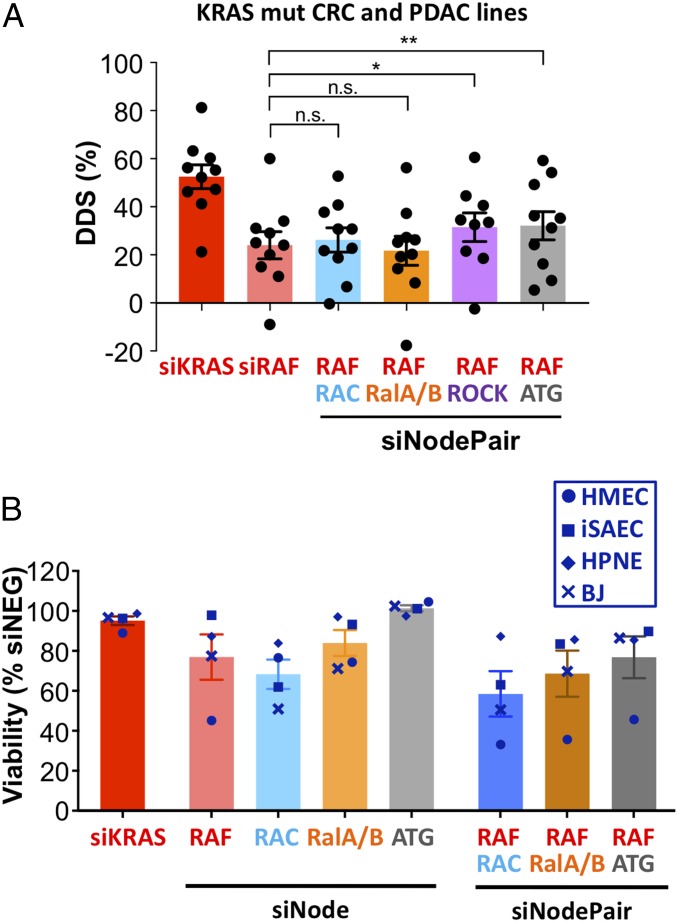
Validation of top node-pair dependencies. (A) KRAS mutant and WT CRC and PDAC cell lines were transfected with siKRAS, an siRAF node, and siNodePair combinations as indicated. Cell viability was determined 5 d posttransfection and normalized to siNeg control, and the DDS for each KRAS mutant cancer cell line was calculated. Bars represent the average DDS metrics across 10 KRAS mutant cell lines, and dots represent data points for individual cell lines. *P < 0.05; **P < 0.01. n.s., not significant. (B) Immortalized HMECs, iSAECs, human pancreas duct normal epithelial cells (HPNE), and human BJ fibroblasts (BJ) were transfected with siKRAS or siRNAs against single RAS effector nodes (siNode) or node pairs (siNodePair) as indicated. Cell viability was determined 4 d posttransfection.
Physiological RAS signaling is critical for the proliferation of normal tissues. In mice, the double knockout of Mek1 and Mek2 or that of Erk1 and Erk2 leads to lethality (9, 39). Although Araf/Braf/Craf triple-knockout mice have not been characterized, it is likely that the complete absence of RAF signaling in normal tissue could not be tolerated. However, double knockout of Braf and Craf in mice is well tolerated (39), suggesting normal tissue can solely rely on ARAF to satisfy physiological RAF signaling. Similarly, double knockout of RalA and RalB leads to lethality in mice, whereas RalB knockout is tolerated well (40). To examine the potential toxicity of RAF, RAC, RAL, and ATG node knockdown in normal cells, we tested the impact of their siNode and siNodePair pools on the viability of several immortalized but untransformed normal cell lines. These include human mammary epithelial cells (HMECs), immortalized human small airway epithelial cells (iSAECs) (41), human pancreatic duct normal epithelial cells, and the human fibroblast cell line BJ. RAF node knockdown introduced substantial toxicity in HMECs, whereas iSAECs and BJ cells are sensitive to RAC node knockdown. RAL node knockdown was moderately toxic in HMECs and BJ cells. In contrast, knocking down the ATG node was well tolerated in all of the normal cell lines. Knocking down node-pair combinations led to additive toxicity in these normal cell lines (Fig. 3B). These results, together with previous mouse genetic studies, suggest that cotargeting all gene paralogs within a RAS effector node would not be well tolerated in normal cells.
To minimize toxicity in normal cells, we hypothesized that KRAS oncoeffectors can be further distinguished at the gene paralog level. We reasoned that by targeting one or two critical oncoeffector paralogs within each RAS effector node while sparing other paralogs, we may be able to effectively attenuate oncogenic KRAS signal while preserving a minimal level of physiological RAS signaling that is essential for normal cell viability. In support of this notion, it has been shown that Craf is critical for KRAS-driven tumors but dispensable in normal mouse tissues (39, 42, 43). We therefore sought to identify the minimal gene paralog combinations that would give the least toxicity in normal cell lines while retaining toxicity in KRAS mutant cells. We first tested siRNAs targeting individual gene paralogs within the RAF, RAC, RAL, and ATG nodes in the normal cell lines. Indeed, toxicity associated with single-gene knockdown was significantly reduced compared with whole-node knockdown, likely due to functional redundancy among paralogs (SI Appendix, Fig. S10A). For RAF kinases, single RAF gene knockdown had little impact on the sensitive HMECs. However, single-gene BRAF and CRAF knockdown also had minor impact on KRAS mutant cells (SI Appendix, Fig. S10B). Codepletion of BRAF and CRAF, while sparing ARAF, led to an intermediate situation compared with whole-RAF node knockdown: It retained a significant fraction of the DDS in KRAS mutant cells (SI Appendix, Fig. S10B), yet the toxicity in HMECs was reduced (SI Appendix, Fig. S10A). These results reinforce the notion that oncogenic KRAS signaling hijacks part of physiological RAS signaling, and that maximizing the therapeutic window might require the preservation of some pathway activity in normal cells.
To identify a minimal gene combination that would give the best selectivity toward KRAS mutant cells, we deconvolved the node-pair combinations down to their constituent gene paralog combinations. Because previous studies have demonstrated a critical role for RAC1 (44) and RALB (22, 45) in KRAS oncogenesis, we chose RAC1 and RALB as the candidate paralogs from their respective nodes. We chose ATG7 from the autophagy node as it is the only known E1 enzyme in this pathway and is required for mutant RAS-driven tumor growth (37, 38). With BRAF, CRAF, RAC1, RALB, and ATG7 as the five key candidates, we codepleted them in various combinations of one to five genes in the 13 CRC and PDAC cancer cell lines and in the four normal cell lines. To quantify the relative impact of these siRNA pools in KRAS mutant cells vs. normal cells, we calculated a differential dependency score vs. normal cells (DDSn), which uses the mean viability of the normal cell lines (instead of that of KRAS WT cancer cells) as the baseline for evaluating genotype selectivity. Clustering analysis showed that BRAF and CRAF remain the main determinants for KRAS dependency (Fig. 4A), and these combinations also had the best DDS, DDSn, and r metrics (SI Appendix, Fig. S11 A and B). The scatter plot of DDS vs. DDSn metrics showed a strong correlation between these two metrics for all combinations (SI Appendix, Fig. S11C), suggesting that normal cell lines and KRAS WT cell lines share a reduced dependency on these genes for survival. Closer inspection of the DDS and DDSn metrics, however, revealed notable differences. When KRAS WT cancer cells were used as the baseline to measure selectivity (DDS metrics), knocking down the entire RAF node provided better capture of KRAS dependency than BRAF and/or CRAF knockdown, and none of the other RAF paralog combinations performed better than RAF node knockdown (Fig. 4B and SI Appendix, Fig. S11D). However, when normal cell lines were used as the baseline to measure selectivity (DDSn metrics), knocking down the entire RAF node gave no better capture of KRAS dependency than BRAF + CRAF knockdown due to increased toxicity of RAF node knockdown in normal cells (Fig. 4B and SI Appendix, Fig. S11E). Top-scoring gene combinations also differed based on the two metrics. The five-gene combination of BRAF + CRAF + RAC1 + RALB + ATG7 scored highest with the DDS metrics, but only ninth in the DDSn metrics (SI Appendix, Fig. S11 D and E), indicating higher toxicity of this combination in normal cells. The top two combinations with the best DDSn metrics involve the three-gene combination of BRAF + CRAF + ATG7 and the four-gene combination of BRAF + CRAF + ATG7 + RAC1. These were also among the top five scoring combinations based on the DDS (Fig. 4B). Importantly, these two combinations were better at capturing KRAS dependency than targeting BRAF + CRAF only (Fig. 4B).
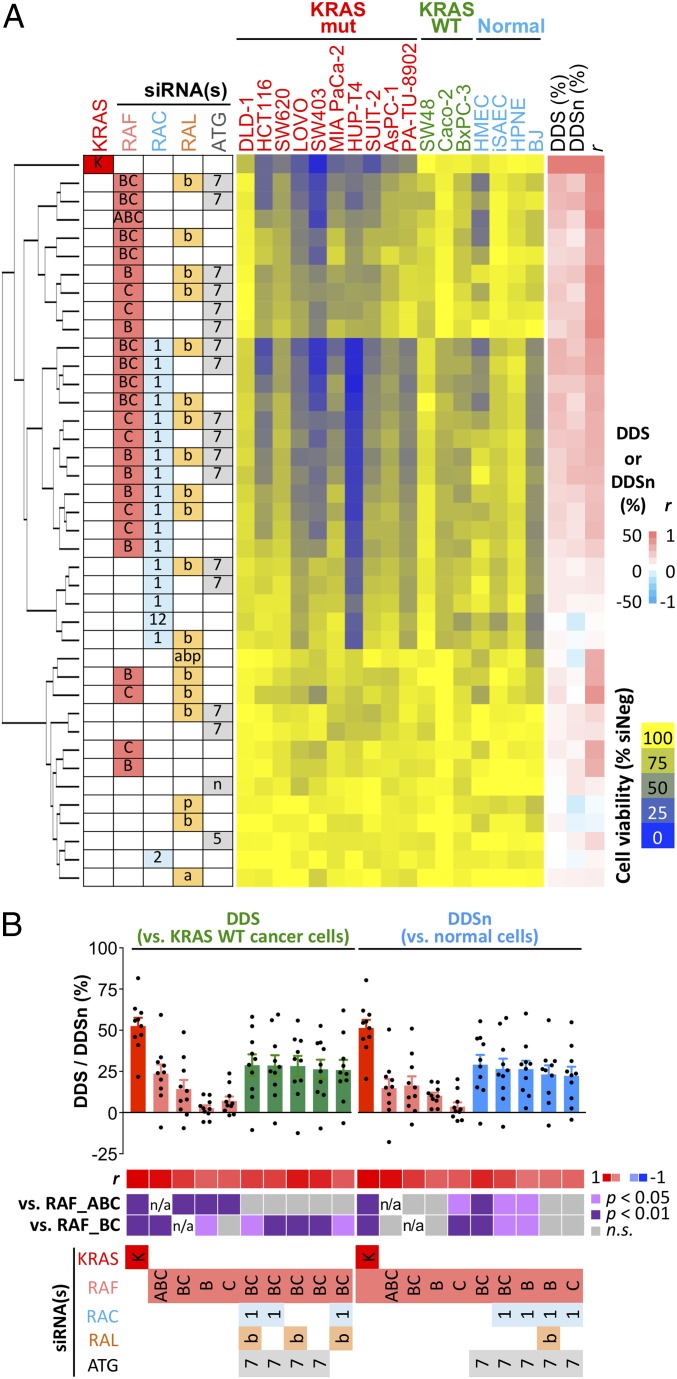
Deconvolution of top gene node-pair dependencies. (A) KRAS mutant and WT CRC and PDAC cell lines and immortalized normal human cell lines were transfected with siRNAs targeting various gene paralog combinations of the RAF, RAC, RAL, and ATG nodes. Cell viability was measured 4–5 d post-siRNA transfection and was normalized to the siNeg control for the respective cell line. Cell viability was clustered based on Euclidean distance. The average DDS, average DDSn, and r metrics of each combination are shown on the right as heat maps. (B) Top-scoring siRNA combinations were ranked separately based on their DDS and DDSn metrics. The siRNAs targeting KRAS and RAF paralogs are included for comparison. Bars represent average DDS and DDSn metrics across 10 KRAS mutant CRC and PDAC cell lines, and dots represent data points for individual cell lines. n/a, not applicable; n.s., not significant. A heat map for corresponding r and P values (compared with RAF knockdown) is shown below the bar chart. Gene symbol abbreviations: A, ARAF; a, RALA; B, BRAF; b, RALB; C, CRAF; K, KRAS; n, BECN1; p, RALBP1; 1, RAC1; 2, RAC2; 5, ATG5; 7, ATG7.
Cotargeting RAF and Autophagy Enhances Cell Cycle Arrest and Cell Death in KRAS Mutant Cells.
To validate the on-target effect of these siRNA combinations, we again performed rescue experiments using sequence-specific C911 control siRNAs. Replacing all on-target siRNAs with their C911 counterparts abolished the toxicity of these siRNA pools (SI Appendix, Fig. S12). We next confirmed the impact of BRAF, CRAF, RAC1, and ATG7 knockdown using Western blot analysis. As expected, all siRNAs performed effectively at depleting their target proteins regardless of the combinatorial setting (SI Appendix, Fig. S13). KRAS knockdown and combined BRAF + CRAF knockdown both down-regulated MAPK pathway activity. We observed reciprocal regulation of two ERK substrates, the transcription factor FRA1 and the proapoptotic protein BIM, such that loss of ERK signaling led to the destabilization of FRA1 and the stabilization of BIM (SI Appendix, Fig. S13). ATG7 knockdown significantly inhibited the activity of the autophagy pathway. This was reflected by the loss of ATG12-conjugated ATG5 and the accumulation of unconjugated ATG5, the decrease in LC3 lipidation, and the destabilization of p62 (SI Appendix, Fig. S13). In agreement with the cell viability data, corresponding C911 siRNAs for each of these genes did not reduce target protein expression or alter pathway activity (SI Appendix, Fig. S13). Together, these results indicate that the activity of these siRNA combinations were on-target effects.
Our finding suggests that cotargeting BRAF, CRAF, RAC1, and ATG7 could show efficacy in KRAS mutant cells while reducing general toxicity in normal cells. Although we do not currently have paralog-specific inhibitors against BRAF and CRAF, we attempted to support the translational potential of our findings by combining RAC1 and ATG7 siRNAs with two selective inhibitors of the MAPK pathway: the US Food and Drug Administration (FDA)-approved MEK inhibitor trametinib and a recently disclosed RAF inhibitor, RAF709, that has little paradoxical effect in RAS mutant cells (46, 47). Depleting ATG7 and/or RAC1 sensitized the KRAS mutant cell lines HCT116 and MIA PaCa-2 to both RAF709 and trametinib, with a decrease in IC50 values between threefold and sevenfold (Fig. 5 and SI Appendix, Fig. S14). In contrast, such an effect was not observed in the KRAS WT BxPC-3 cells (Fig. 5 and SI Appendix, Fig. S14). In agreement with the siRNA combination knockdown data (Fig. 4B), ATG7 knockdown had a greater sensitizing effect than RAC1 knockdown in these pharmacological experiments.
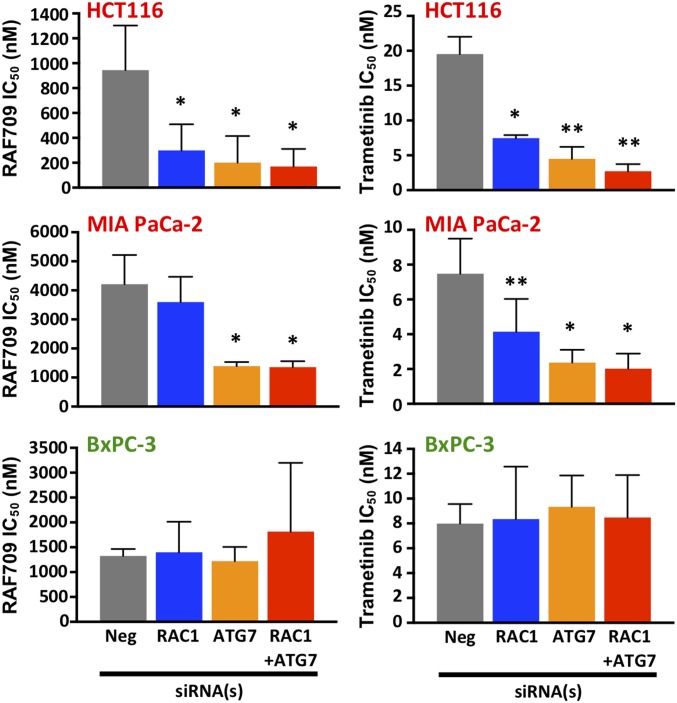
RAC1 and ATG7 depletion sensitizes KRAS mutant cells toward MAPK pathway inhibitors. The KRAS mutant cancer cell lines HCT116 and MIA PaCa-2 and KRAS WT cancer cell line BxPC-3 were transfected with siRNAs against RAC1 and/or ATG7. One day posttransfection, cells were treated with various concentrations of the RAF inhibitor RAF709 or the MEK inhibitor trametinib. Cell viability was determined 4 d later to obtain dose–response curves, and RAF709 and trametinib IC50 values were determined and are shown as bar charts (error bars represent SD). *P < 0.05 vs. negative (Neg); **P < 0.01 vs. Neg.
MAPK pathway inhibition often leads to G1 cell cycle arrest (48, 49). We hypothesized that ATG7 and RAC1 knockdown could synergize with RAF inhibition either by causing stronger cell cycle arrest or by inducing apoptosis in KRAS mutant cells. Cell cycle analysis showed that KRAS knockdown caused G1 arrest in the KRAS mutant cell lines HCT116 and MIA PaCa-2. In agreement with their effect on cell viability, codepletion of BRAF and CRAF did not lead to a significant cytostatic effect. However, codepleting BRAF and CRAF together with ATG7 and/or RAC1 resulted in G1 arrest that was comparable to KRAS knockdown (Fig. 6A). In the KRAS mutant cell lines HCT116 and SW403, KRAS knockdown also increased apoptosis, as judged by an increased sub-G1 population and elevated caspase activity in these cells. Whereas codepleting BRAF and CRAF did not strongly induce cell death, the addition of ATG7 and RAC1 to the combination led to a strong apoptosis response in these cells that was comparable to KRAS knockdown (Fig. 6 B and C). Knocking down ATG7 alone had little impact on cell cycle and apoptosis, whereas RAC1 knockdown had a modest but genotype-independent effect on G1 arrest in both KRAS mutant and WT cells (SI Appendix, Fig. S15). The enhanced G1 arrest and apoptosis effect following BRAF + CRAF + ATG7 codepletion was not observed in KRAS WT cell lines, or with their corresponding C911 control siRNAs (Fig. 6). Taken together, our finding suggests that cotargeting the MAPK and autophagy pathways using the target combination of BRAF, CRAF, and ATG7 could be a viable strategy that offers a good therapeutic window in KRAS mutant cells.
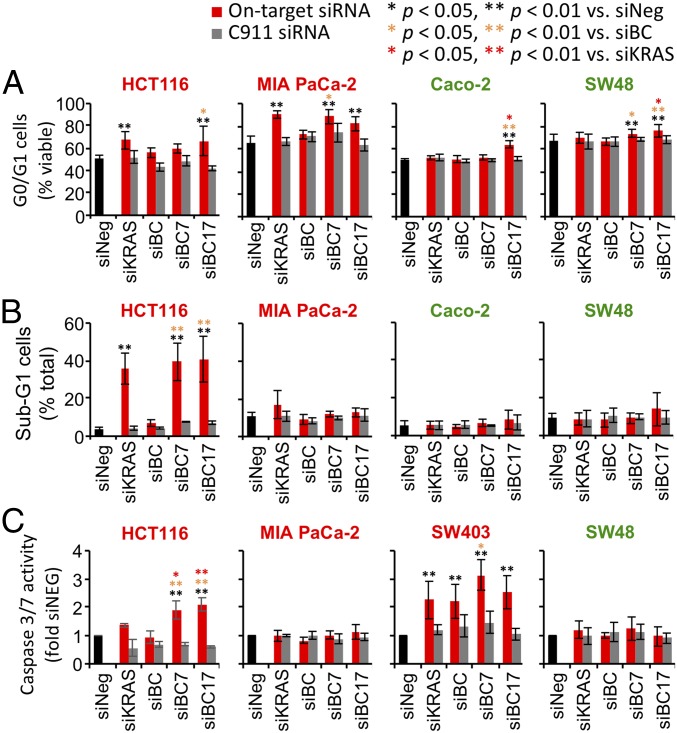
Impact of siRNA combinations on cell cycle and apoptosis in KRAS mutant cells. KRAS mutant (HCT116, MIA PaCa-2, and SW403) and KRAS WT (Caco-2 and SW48) cancer cell lines were transfected with various siRNA combinations targeting BRAF (B), CRAF (C), RAC1 (1), and ATG7 (7). Corresponding C911 siRNA pools were included as rescue controls. Cell cycle and apoptosis status were analyzed 3 d posttransfection. (A) Changes in G0/G1 viable cell populations by flow cytometry. (B) Changes in sub-G1 dead cells by flow cytometry. (C) Changes in caspase activity in cells.
Discussion
Functional genomics screens using genome-wide RNAi and CRISPR/Cas9 libraries have been extensively used for target identification. However, large-scale loss-of-function screens have been mostly limited to the analysis of single-gene phenotypes. In mammalian cells, false-negative results due to pervasive gene paralog redundancy is a significant issue for these studies. Indeed, many canonical RAS effectors, including RAF and PI3K paralogs, were rarely scored in KRAS synthetic lethal screens using both shRNA and CRISPR libraries (20–26). To overcome this limitation, we have developed a combinatorial siRNA platform that uses experimentally validated and highly potent sensor siRNAs to achieve efficient multigene knockdown in the same cell. This platform can reliably achieve the simultaneous knockdown of up to seven genes in the cell (29). Combinatorial gene targeting at this level of complexity is not easily achievable with current CRISPR/Cas9 technologies. Our approach offers several advantages compared with traditional single-gene library screens. First, it enables a comprehensive dissection of gene paralog redundancy within a gene node to accurately assess its function. Second, it enables the analysis of gene and pathway interactions at the systems level to understand how different genes within the network, such as those in the RAS network, could cooperate with or antagonize each other to modulate the network’s output. This was not previously possible with single-gene analysis. Third, our platform offers a powerful and highly scalable approach to rationally evaluate target combination. We demonstrated that siRNAs can be combined and transfected into cells at high efficiency, the simplicity of which rivals that of drug combinations. Thus, virtually any target combination can be investigated in an unbiased fashion regardless of whether small molecule inhibitors are readily available. Our approach could thus accelerate the development of effective combination therapy for precision medicine.
Our systematic interrogation of RAS effector and stress response pathways for their role in mediating KRAS addiction led to several important mechanistic insights with translational implications. Our analysis of nearly ~500 single-gene nodes, gene node pairs, and gene paralog combinations revealed that the RAF node is the most critical oncoeffector downstream of mutant KRAS and should constitute the backbone of combination therapies. This is in agreement with previous studies showing that the MAPK pathway is essential for RAS-driven cell proliferation (34). Unexpectedly, we noted that knocking down the RAF, MEK, and ERK nodes within the MAPK pathway led to unequal degrees of differential dependency in KRAS mutant cells. This trend was also observed in our previous single-node study across 92 KRAS mutant and WT cancer cell lines (27). Our observation is in agreement with a previous study showing that Kras-driven colorectal tumors in mice are more sensitive to RAF inhibition than MEK inhibition (50). The mechanism by which KRAS dependency is better captured by RAF knockdown compared with MEK and ERK knockdown is currently unclear. We found that knocking down the RAF, MEK, and ERK nodes all had a similar impact on the phospho-ERK level in cells; thus, this difference cannot be simply attributed to MAPK pathway flux. It is possible that RAF knockdown might lead to a more sustained loss of ERK activity in the nucleus (51). Alternatively, RAF could potentially mediate KRAS oncogene addiction through both MAPK pathway-dependent and -independent means (43, 50, 52–55). Thus, further studies are needed to clarify the mechanism of RAF dependency in KRAS mutant cells.
By carefully dissecting gene paralog dosage effect, we demonstrated that codepleting BRAF and CRAF, while sparing ARAF, is sufficient to disrupt much of the oncoeffector function of the RAF node, while minimizing toxicity in normal cells. Because RAF signaling is likely to be essential in normal tissues, a pan-RAF inhibitor could have excessive toxicity. Our finding highlights the importance of careful target selection to discriminate the oncogenic activity of the MAPK pathway from its physiological activity. Previously, it has been shown that Craf, but not Braf, is critical for Kras mutant tumor development in mice (39, 42, 43). Our results suggest that in human KRAS mutant cells, BRAF and CRAF need to be cotargeted to effectively disrupt oncogenic KRAS signaling. We propose that the ideal RAF inhibitor would be ARAF-sparing and without paradoxical activity. One promising approach to develop compounds with these properties would be to identify small molecules that selectively disrupt BRAF/CRAF dimerization (56).
Our work provides support for the notion that oncogenic KRAS signaling is mediated by multiple pathways. We showed that cotargeting the autophagy E1 ligase ATG7 or the small GTPase RAC1, together with BRAF and CRAF, could further improve the capture of KRAS dependency. The identification of the autophagy pathway as a RAF cotarget is a valuable insight, as autophagy is an intimate component of tumor development (57). Previous studies using mouse models of Kras mutant lung and pancreatic cancer have demonstrated a critical role of Atg7 and Atg5 for tumor progression and for the maintenance of energy and nucleotide supply in tumor cells, suggesting the autophagy pathway as a target in KRAS-driven cancer (38, 58–60). In agreement with previous in vitro studies showing that the proliferation of some KRAS-mutant cancer cell lines under nutrient-replete conditions is not strongly impacted by pharmacological inhibition of autophagy, by RNAi-mediated acute ATG5/ATG7 knockdown, or by CRISPR-mediated ATG7 knockout (61), we found that ATG7 knockdown alone had relatively little effect on the viability of the cancer cell lines tested in this study. However, we found that ATG7 knockdown enhances the toxicity of BRAF and CRAF siRNAs, consistent with a role of RAS/RAF signaling in the metabolic rewiring of cancer cells (57, 62). Together, these prior studies and our current study support a model where KRAS-driven metabolic alterations in cancer cells render them particularly dependent on the autophagy pathway as a survival mechanism upon the acute inhibition of the RAF/MAPK pathway (SI Appendix, Fig. S16). Due to the nonessentiality of autophagy in normal cells under nutrient-replete conditions and the fact that adult mice with acute, systemic deletion of Atg7 are able to survive for several months (38), we expect the combination of autophagy with RAF inhibition to be well tolerated in vivo. The autophagy pathway is a nononcogene addiction mechanism in KRAS mutant cells. Thus, the RAF + ATG7 target combination exploits both oncogene and nononcogene addiction in KRAS mutant cells, and this could lead to a better and more durable response than targeting oncogene addiction alone. This notion is supported by a previous study showing ATG7 deficiency enhances the antitumor activity of BRAF inhibitor in BRAF mutant melanomas (63). Although no ATG7 inhibitors are currently available, selective inhibitors have been developed for neddylation and SUMOylation E1 ligases (64, 65), and these experiences could provide guidance for an ATG7 inhibitor discovery program. Furthermore, hydroxychloroquine, an FDA-approved antimalaria drug that also inhibits autophagosome fusion with the lysosome, is currently undergoing clinical trials as an anticancer agent (3). Our findings would support its rational combination with either MEK inhibitors or paradox-breaker RAF inhibitors currently in clinical trial for KRAS mutant tumors. Combined targeting of RAF kinases and the autophagy pathway could potentially offer less toxicity and better efficacy compared with drug combinations currently being tested.
Our study also suggests that RAC1 is a potential cotarget with RAF. Rac1 is critical for KRAS-driven lung and pancreatic cancer initiation in mice (44, 66). However, RAC1 might have an essential role in normal tissue function (67), and RAC1 knockdown is toxic in some KRAS WT cells. Thus, further work is necessary to evaluate whether cotargeting RAC1 and RAF could provide a sufficiently large therapeutic window. Our combinatorial analysis revealed several principles for the rational selection of target combination. First, combinations targeting two essential pathways, such as the RAF + PI3K combination, may paradoxically offer less selectivity due to higher toxicity in KRAS WT cells. In this setting, pan-paralog inhibitors are not desirable and paralog-selective inhibitors that can restrict oncogenic signaling while sparing physiological signaling are necessary to preserve a good therapeutic window. Second, top-scoring single targets, such as those involving RAF and RAL paralogs, may not necessarily result in the best combinations. Third, nonessential single targets, such as ATG7, could, in fact, be a valuable partner in a combination setting. These functional interplays would be difficult to predict based on single-gene and single-node analysis. Thus, direct analysis of gene node and pathway interactions at the systems level is critical for unmasking complex behaviors in the RAS signal network.
Our study has uncovered a significant degree of heterogeneity among KRAS mutant cell lines with regard to the specific RAS effector and stress response pathways used to support KRAS addiction. Previously, we showed that strong KRAS dependency is associated with MAPK pathway dependency, whereas KRAS mutant cells that are less dependent on KRAS exhibit dependency on p90RSK kinases (27). In the current study, we showed that each KRAS mutant cell line has a distinct dependency signature for top-scoring gene node combinations. The public node-pair dependencies across multiple cell lines are, in general, less effective at capturing KRAS dependency than the best private node-pair dependencies with respect to an individual cell line. This is analogous to previous work examining public and private drug sensitivities in lung cancer cells (36). How such heterogeneity in effector and stress response pathway dependency arises among KRAS mutant tumor cells is poorly understood. Possible contributing factors include the etiology of tumor evolution and the presence of co-occurring mutations in the same tumor cell. A translational implication of this heterogeneity is that KRAS mutation alone may not be sufficient as a single biomarker to direct the choice of targeted therapies, as drug combinations designed to work for the majority of KRAS mutant tumors are not expected to work particularly well for any given KRAS mutant tumor. Multiple combination therapies, with each optimized for a subset of KRAS mutant tumors sharing a similar effector and stress pathway dependency profile, might be necessary to adequately address tumor heterogeneity in this setting. Thus, additional biomarkers are needed to subdivide KRAS mutant tumors based on their functional profiles in effector and stress pathway dependency. Our current study is only powered to detect common dependencies across KRAS mutant cell lines. Expanding this analysis to a much larger panel of KRAS mutant cell lines (27) will enable us to further subcategorize KRAS mutant cells based on their patterns of effector and stress response pathway dependency and to discover the biomarkers that are associated with each category to better direct the choice of drug combination.
Our analysis revealed a significant phenotypic gap between targeting the KRAS oncoprotein itself vs. targeting its downstream effector network. We noted that none of the siRNA combinations could fully phenocopy KRAS knockdown: The best effector combination we identified only captures a little over 50% of KRAS dependency. Although several explanations could be offered (discussed in SI Appendix, SI Notes), it is possible that the functional overlap between oncogenic and physiological RAS signaling imposes a selectivity ceiling. Thus, targeting KRAS oncoeffectors may never achieve the same therapeutic window as targeting KRAS oncoprotein itself. Recent efforts in developing novel KRASG12C inhibitors have gained significant traction (68–70). A useful future direction would be to identify KRAS oncoeffectors that strongly synergize with KRASG12C inhibitors to enhance the genotype-dependent killing of KRAS mutant cancer cells.
Materials and Methods
RAS Effector and Stress Pathway Gene siRNA Library Curation.
Sensor siRNAs against the list of RAS effector genes interrogated in this study were generated as previously described (29, 31) and as detailed in SI Appendix. For a selected subset of on-target siRNAs in our library, we generated their sequence-specific C911 rescue siRNAs as previously described (32). All siRNA sequences are listed in Dataset S1.
Cell Lines and Reagents.
All cell lines used in this study were cultured as described in SI Appendix. Trametinib and RAF709 (MedChem Express) were dissolved in DMSO at stock concentrations of 1 mM and 10 mM, respectively.
Transfection of siRNA Combinations and Inhibitor Treatment.
To knock down multiple gene targets simultaneously and to cotreat cells with siRNAs and inhibitors, reverse siRNA transfection was performed as previously described by Garimella et al. (71), with slight modifications as described in SI Appendix.
Cell Viability, Caspase 3/7 Activity, and Cell Cycle Assays.
Cell viability and caspase 3/7 assays were performed by using the CellTiter-Glo Luminescent Cell Viability Assay (Promega) and ApoLive-Glo Multiplex Assay (Promega), respectively, and as described in SI Appendix. Cell cycle analysis was performed as previously described by Weng et al. (72) and in SI Appendix.
Immunoblotting.
To examine the effect of siRNA-mediated knockdown on protein expression and pathway activity, at 5 d post-siRNA transfection, whole-cell extract was harvested as described in SI Appendix.
Data Analysis and Statistics.
To assess the differential impact of siRNAs on KRAS mutant and WT cancer cell lines as well as immortalized, nontransformed normal cell lines, we normalized cell viability and quantified the effect of each siRNA pool as described in SI Appendix. Unsupervised hierarchical clustering was performed using Partek Genomic Suite software (Partek, Incorporated). Outlier analysis, paired t tests, and ANOVA and postanalysis were performed using GraphPad Prism (GraphPad Software).
Supplementary Material
Supplementary File
Supplementary File
Acknowledgments
We thank Drs. Eric Batchelor, Jayne Stommel, and Rosandra Kaplan for their constructive suggestions, and the Flow Cytometry Core Facility of the Center for Cancer Research at the National Cancer Institute (NCI) for the technical support. This work was supported by NCI Intramural Grant ZIA BC 011437 (to J.L.) and by an NCI Director’s Innovation Award (to C.-S.L.).
Footnotes
Conflict of interest statement: F.M. is a consultant for the following companies: Aduro Biotech; Amgen; Daiichi Ltd.; PellePharm; Pfizer, Inc.; PMV Pharma; and Portola Pharmaceuticals. F.M. is Scientific Director of the National Cancer Institute RAS Initiative at Frederick National Laboratory for Cancer Research/Leidos Biomedical Research, Inc. F.M. is a consultant for and cofounder of the following companies: Avidity, BridgeBio, KGen, and Quartz. L.C.L. is a current employee of Loxo Oncology. T.L.Y. is a current employee of Novartis.
This article is a PNAS Direct Submission.
See Commentary on page 3965.
This article contains supporting information online at www.pnas.org/lookup/suppl/10.1073/pnas.1817494116/-/DCSupplemental.
References
Articles from Proceedings of the National Academy of Sciences of the United States of America are provided here courtesy of National Academy of Sciences
Full text links
Read article at publisher's site: https://doi.org/10.1073/pnas.1817494116
Read article for free, from open access legal sources, via Unpaywall:
https://www.pnas.org/content/pnas/116/10/4508.full.pdf
Citations & impact
Impact metrics
Citations of article over time
Alternative metrics
Smart citations by scite.ai
Explore citation contexts and check if this article has been
supported or disputed.
https://scite.ai/reports/10.1073/pnas.1817494116
Article citations
Is Autophagy Targeting a Valid Adjuvant Strategy in Conjunction with Tyrosine Kinase Inhibitors?
Cancers (Basel), 16(17):2989, 28 Aug 2024
Cited by: 0 articles | PMID: 39272847 | PMCID: PMC11394573
Review Free full text in Europe PMC
The safety and efficacy of binimetinib for lung cancer: a systematic review.
BMC Pulm Med, 24(1):379, 01 Aug 2024
Cited by: 1 article | PMID: 39090580 | PMCID: PMC11295668
Review Free full text in Europe PMC
Response to trametinib, hydroxychloroquine, and bevacizumab in a young woman with NRAS-mutated metastatic intrahepatic cholangiocarcinoma: a case report.
Explor Target Antitumor Ther, 5(3):780-788, 28 Jun 2024
Cited by: 0 articles | PMID: 38966164 | PMCID: PMC11220291
Off-target autophagy inhibition by SHP2 allosteric inhibitors contributes to their antitumor activity in RAS-driven cancers.
J Clin Invest, 134(15):e177142, 06 Jun 2024
Cited by: 0 articles | PMID: 38842946 | PMCID: PMC11291269
Protein phosphatase 6 activates NF-κB to confer sensitivity to MAPK pathway inhibitors in <i>KRAS</i>- and <i>BRAF</i>-mutant cancer cells.
Sci Signal, 17(836):eadd5073, 14 May 2024
Cited by: 0 articles | PMID: 38743809 | PMCID: PMC11238902
Go to all (75) article citations
Data
Data behind the article
This data has been text mined from the article, or deposited into data resources.
BioStudies: supplemental material and supporting data
Similar Articles
To arrive at the top five similar articles we use a word-weighted algorithm to compare words from the Title and Abstract of each citation.
Relationships among KRAS mutation status, expression of RAS pathway signaling molecules, and clinicopathological features and prognosis of patients with colorectal cancer.
World J Gastroenterol, 25(7):808-823, 01 Feb 2019
Cited by: 17 articles | PMID: 30809081 | PMCID: PMC6385012
BRAF associated autophagy exploitation: BRAF and autophagy inhibitors synergise to efficiently overcome resistance of BRAF mutant colorectal cancer cells.
Oncotarget, 7(8):9188-9221, 01 Feb 2016
Cited by: 40 articles | PMID: 26802026 | PMCID: PMC4891035
LY3009120, a panRAF inhibitor, has significant anti-tumor activity in BRAF and KRAS mutant preclinical models of colorectal cancer.
Oncotarget, 8(6):9251-9266, 01 Feb 2017
Cited by: 37 articles | PMID: 27999210 | PMCID: PMC5354729
Inhibition of the RAF/MEK/ERK Signaling Cascade in Pancreatic Cancer: Recent Advances and Future Perspectives.
Int J Mol Sci, 25(3):1631, 28 Jan 2024
Cited by: 3 articles | PMID: 38338909 | PMCID: PMC10855714
Review Free full text in Europe PMC
Funding
Funders who supported this work.
Intramural NIH HHS (1)
Grant ID: ZIA BC011437
NCI NIH HHS (2)
Grant ID: P30 CA008748
Grant ID: ZIA BC011437