Abstract
Free full text

Copy number alterations in pancreatic cancer identify recurrent PAK4 amplification
Abstract
Pancreatic cancer is one of the most lethal of all cancers. The median survival is six months and less than 5% of those diagnosed survive five years. Recurrent genetic deletions and amplifications in 72 pancreatic adenocarcinomas, the largest sample set analyzed to date for pancreatic cancer, were defined using comparative genomic hybridization The recurrent genetic alterations identified target a number of previously well-characterized genes, as well as regions that contain possible new oncogenes and tumor suppressor genes. We have focused on chromosome 19q13, a region frequently found amplified in pancreatic cancer and demonstrate how boundaries of common regions of mutation can be mapped and how a gene, in this case PAK4 amplified on chromosome19q13, can be functionally validated. We show that although the PAK4 gene is not activated by mutation in cell lines with gene amplification, an oncogenic form of the KRAS2 gene is present in these cells and oncogenic KRAS2 can activate PAK4. In fact in the three samples we identified with PAK4 gene amplification, the KRAS2 gene was activated and genomically amplified. The kinase activity of the PAK4 protein is significantly higher in cells with genomic amplification as compared to cells without amplification. Our study demonstrates the utility of analyzing copy number data in a large set of neoplasms to identify genes involved in cancer. We have generated a useful dataset which will be particularly useful for the pancreatic cancer community as efforts are undertaken to sequence the pancreatic cancer genome.
Introduction
It has been estimated that this year 37,680 Americans will be diagnosed with pancreatic cancer and that 34,290 Americans will die from this disease (see www.cancer.org/downloads/STT/CAFF2008PWSecured.pdf).1 The five year survival rate for pancreatic cancer is one of the lowest of all the solid malignancies, with a less than 5% five year survival rate. It should not be surprising that pancreatic cancer is the fourth leading cause of cancer death in the United States. Most existing therapies are of marginal efficacy. They do little to extend survival or alleviate pain and that do not cure the patient. At the same time, novel approaches have been developed to target genetic differences between cancer and normal cells, including the synthetic lethal screening methodology described by L. Hartwell.2 For example, the methylthioadenosine phosphorylase (MTAP) gene on chromosome 9p is frequently included in homozygous deletions targeting the p16/CDKN2A gene in pancreatic cancer.3 The deletion of the MTAP gene in pancreatic cancers deletes a critical gene in the synthesis of AMP, making these cancer cells selectively sensitive to inhibitors of the salvage pathway for AMP biosynthesis.
Deletions and amplifications can be accurately identified by array based comparative genomic hybridization (CGH). There are several different array formats for CGH available. These arrays differ in the material utilized for probes, cDNA fragments,4 BAC DNA or representations of BAC DNAs,5 or oligonucleotides, but most rely on total genome or whole genome amplifications of total genome for hybridization. We have developed representational methods for array CGH6,7 which have been adopted by others.8 Our method representational oligonucleotide microarray analysis (ROMA)6,7 utilizes representation of the genomic material,9,10 reducing the complexity of the genome, increasing hybridization efficiency and increasing the signal to noise generated.
We have used ROMA to analyze the copy number fluctuation in a set of 72 well-characterized adenocarcinomas of the pancreas to map the regions frequently amplified and deleted. The carcinomas analysed included 22 cell lines, 26 surgically resected primary carcinomas and 24 xenografts of surgically resected pancreatic adenocarcinomas (independent of the primary tumors analyzed). The primary carcinomas were flow sorted to enrich for neoplastic cells, overcoming one of the major hurdles in the genetic analyses of pancreatic cancer-low neoplastic cellularity.
To increase the number of samples analyzed and to increase the probability of finding genomic regions targeted in pancreatic cancer, we appended data from 16 primary pancreatic carcinomas and four cell lines previously reported by Aguirre and colleagues.11 These carcinomas did not overlap with our series of cancers and the data from the two series are therefore complimentary. The combined dataset therefore included a total of 92 unique pancreatic cancer samples, the largest dataset publicly available for pancreatic cancer. In this dataset we identified the most common regions amplified and deleted that are smaller than 4 MB. As expected, we identified many genes known to be targeted in pancreatic cancer and other cancers as well including p16/CDKN2A (Ink/Arf), TP53, c-MYC, KRAS2, MADH4, TERT, EGFR, ERBB2 and znf217 and a number of regions that have not been previously reported. We also report the genomic regions that are concurrently altered in the same cancer. We report the regions identified and make the data freely accessible to the public as supplemental material (www.lucitolab.cshl.edu/rl_publications.html).
We identified a focal amplification on chromosome 19q13 in 3 of 27 cell lines and in 5 of 42 primary carcinomas. Candidate oncogenes within this region were mapped and the copy number data were used to map the boundaries of the common regions of mutations or the “epicenter” of the amplification. Cell lines and one primary carcinoma, which have the amplicon, were then used to determine expression of the 26 candidate genes within the amplification. From this analysis three candidate genes remained and immunohistochemical labeling was used to eliminate one of the three, leaving the PAK4 and IXL genes.
The IXL gene has been previously shown to increase cell survival.12 The PAK4 gene is a member of the group B p21 activated kinases (PAKs), which also include PAK5 and 6. The PAKs are effectors for Rho GTPases that mediate the effects of Rac and Cdc42 on the cytoskeleton and gene expression.13–17 Of the PAKs, PAK4 has been shown to promote anchorage independent growth when activated.18 In light of this evidence and the fact that other mapped amplicons in the cancer genome have identified multiple targets we further studied the PAK4 gene as being a second target in the 19q13 amplicon in pancreatic cancer. We demonstrate that PAK4 protein is overexpressed in surgically resected primary pancreatic cancer and in pancreatic cancer cell lines that have genomic amplification and that the kinase activity in these cells is much higher than it is in cells without amplification. Furthermore we show that the PAK4 gene is not in a mutated oncogenic form but that activation of the PAK4 gene is likely to be promoted by KRAS2 gene mutation, a very frequent event in pancreatic cancer. The KRAS2 gene was genomically amplified and overexpressed in several of the pancreatic cancers with PAK4 amplification, likely further increasing the ras activity in these cells. It is likely that the PAK4 gene cooperates in the growth of pancreatic cancer cells alone or in cooperation with other genes from the region such as IXL.
Results
We have previously demonstrated that ROMA can be used to accurately resolve copy number variations in cancer as well as in normal individuals.6,25 The oligonucleotide arrays utilized are synthesized by NimbleGen Systems Inc., to our specification and have been described in detail in an earlier publication.6
We obtained 22 cell lines from ATCC, 24 xenografts of pancreatic adenocarcinomas surgically resected at The Johns Hopkins Hospital and 26 primary pancreatic adenocarcinomas, surgically resected at the Johns Hopkins Hospital, the Arizona Cancer Center, or from the Cooperative Human Tissue Network. When matched normal was not available (Suppl. Table 1) samples were compared to a normal male fibroblast reference DNA. To remove system noise inherent to the data statistical methods were used, commonly referred to as Circular Binary Segmentation (CBS).21
Regions found frequently altered are more likely to be meaningful and the probability of finding any one region altered in multiple samples increases with a larger sample set. To increase the sample size of (primary tumors especially) we added data from a published sample set,11 which included an additional 16 primary pancreatic carcinoma samples and six cell lines not included in our initial analyses. The data set was configured to our format and cell lines analyzed that were present in both datasets were used to cross validate the configuration of the data set.
The copy number data for the entire set is shown in Figure 1 as a heat map. With the data in this format is it is relatively easy to identify common regions of alteration. For example, it is clear that the p16/CDKN2A (INK/ARF) locus on chromosome 9p, the TP53 locus on 17p and the MADH4 locus on 18q are all recurrently deleted. If the heat map is used to zoom in it is relatively easy to scan the data to identify the genomic coordinates that can be used as breakpoints to map candidate genes within the regions altered.
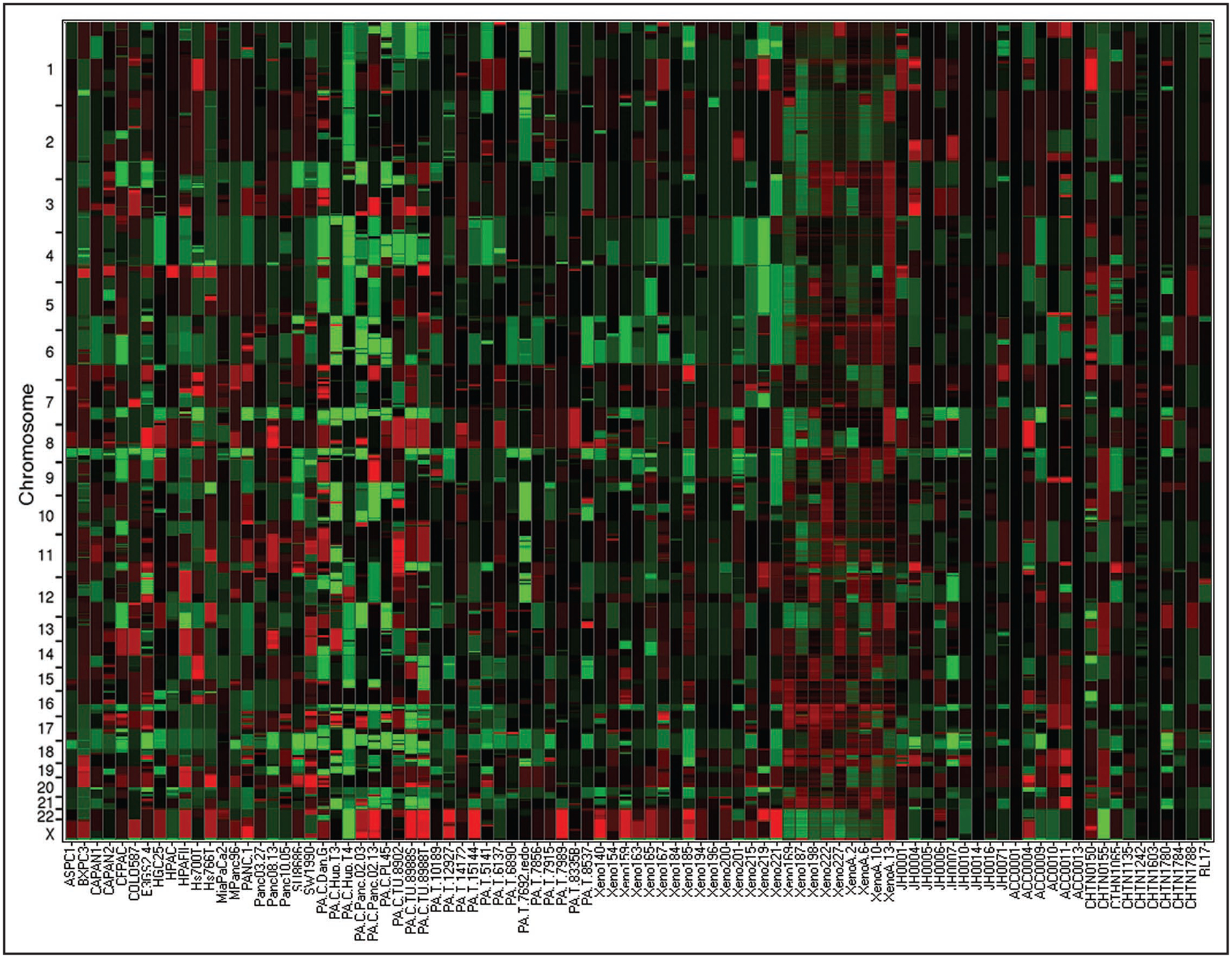
Copy number of the entire dataset translated as a heat map. In this format each column of the heat map represents a sample. The samples are grouped such that tumor cell lines are first, then xenografts followed by primary tumors. Each probe has been reduced to a block and the color of that block is representative of that probes copy number, amplifications being a shade of red and deletions being a shade of green. On the X-axis are the samples names and the Y-axis places probes in genomic position concatenating all chromosomes to allow visualization of the entire genome.
Another common method to visualize alterations identified within a large dataset is a frequency plot, or the frequency with which specific regions are deleted or amplified. Frequency was calculated for all probes by identifying those which varied by 1 SD or more. We compared the frequency plots for each group of samples, i.e., primary carcinomas, xenografts and cell lines, separately and found that the primary carcinomas and the xenografts were very similar and this group of samples differed from the cell lines. Therefore the samples were divided into two groups during further analysis, one group included the primary carcinomas and the xenografts and the second group the cell lines. The changes observed in the cell lines may be a manifestation of increased genomic instability caused by long term culture. The differences in the overall genomic patterns between the primary carcinoma/xenograft data and the cell line data are clearly apparent (Fig. 2), with the cell lines, as may be expected, having more lesions.
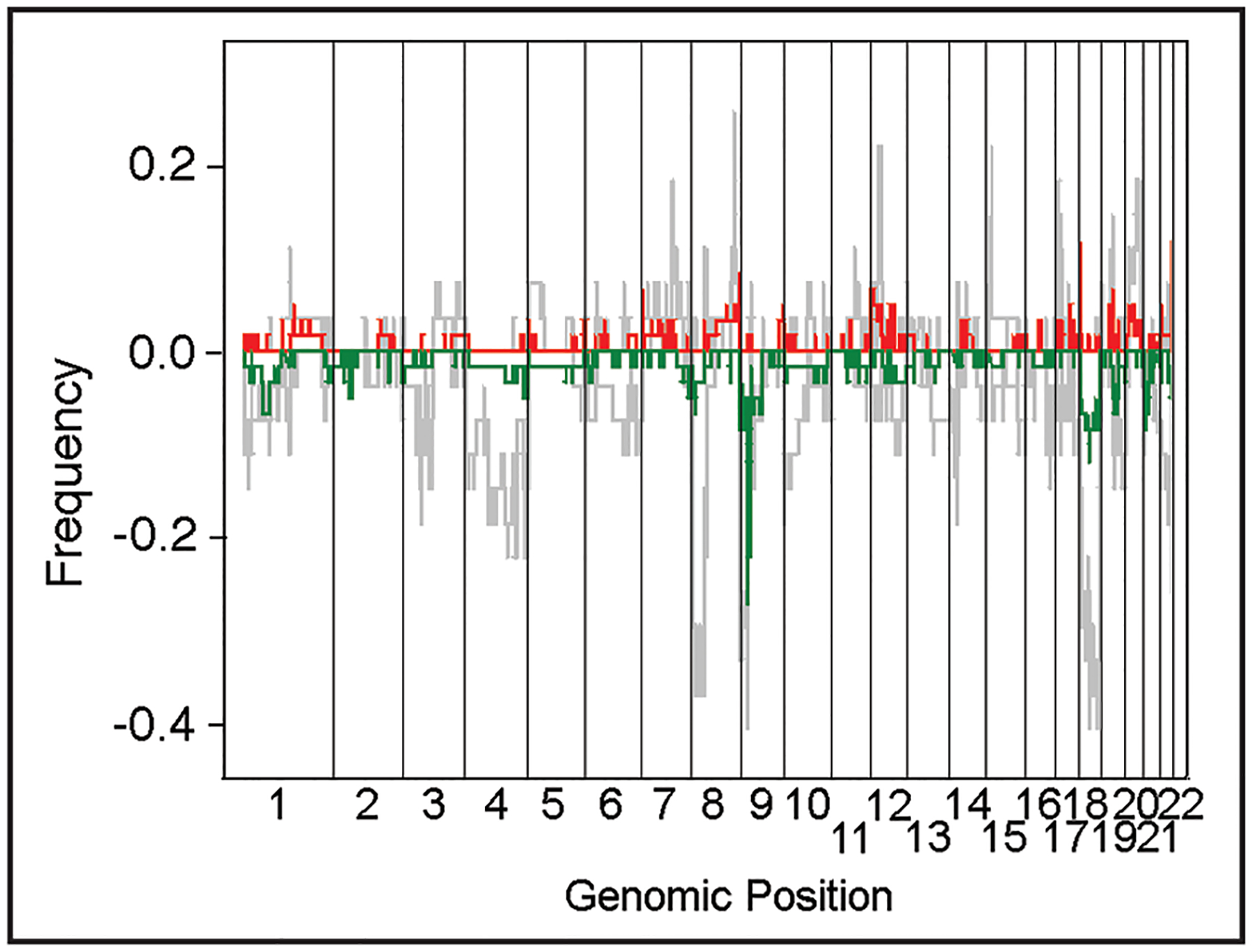
A frequency plot, calculated from the copy number of the entire dataset. The cumulative number of lesions were calculated probe by probe over all experiments and then normalized based on the sample size for the set utilized to calculate the frequency. The two sets utilized for this analysis were cell lines as one set and primary tumors and xenografts as a second set. Amplifications and deletions were calculated separately, for primary tumors and xenografts the amplifications are red and deletions are green, whereas both amplifications and deletions for the cell line set are light grey. The X-axis is the genomic position, concatenating the chromosomes to allow visualization of the entire genome and the Y-axis is the frequency that the copy number abberations are observed in the specific dataset.
A heuristic algorithm was developed to map regions of interest for the identification of candidate genes. We found that although the cell lines had many more lesions than primary carcinomas/xenografts, the cell line data are of great value in delimiting the epicenter of the genetic alterations. The size of the regions identified ranged from 100 kb to many megabases. The regions identified that were smaller than 4 MB or that contained fewer than 20 candidate genes are listed in Table 1. Once regions have been mapped, candidate genes can easily be identified using the outermost array probes as breakpoints. The genomic coordinates of these probes, once entered into a site such as the UCSC genome browser can be used to identify all genes included within the coordinates. Many of the regions we identified have been reported in previous studies using lower resolution technologies such as CGH and microsatellite markers26,27 and some have been reported using technologies with higher resolution.11,28–31 These included regions known to be altered in pancreatic cancer and many of these regions contain genes that are well characterized such as the p16/CDKN2A (INK/ARF) locus on chromosome 9p, the TP53 locus on 17p and the MADH4 locus on 18q.
Table 1
Regions identified from the copy number dataset that were altered in more than one sample
DELETIONS | # PT | # CL | GENES | Possible Targets | AMPLIFICATIONS | # PT | # CL | GENES | Possible Targets | |
---|---|---|---|---|---|---|---|---|---|---|
chr1:24,792,426-26,792,737 | 1 | 2 | RUNX3 | chr1:120,166,947-142,176,070 | 3 | 1 | NOTCH2? | |||
chr2:79344682-84774287 | 1 | 1 | CTNNA2 | chr5:70263-1328598 | 1 | 4 | TERT | |||
chr2:227737707-231987712 | 0 | chr7:1-2,657,200 | 4 | 4 | MAD1L1/GPR30 | |||||
chr3:60087077-60847539 | 1 | 3 | FHIT | chr7:38430305-39908121 | 1 | 3 | RALA | |||
chr4:53516692-56615709 | 1 | 0 | chr7:50407256-54964099 | 1 | 3 | EGFR | ||||
chr4:148950010-154173930 | 1 | 3 | FBXW7 | chr7:93522091-98500777 | 1 | 4 | ||||
chr4:177956667-181580416 | 3 | * | chr8:40349907-41720315 | 2 | 1 | |||||
chr4:185715799-187547587 | 1 | 3 | TLR3/CASP3 | chr8:127526999-129034990 | 2 | >5 | MYC | |||
chr6:148903035-152837151 | 1 | 4 | chr8:143736139-146199402 | 2 | 2 | |||||
chr7:17071918-18953883 | 1 | 1 | chr11:45597347-46350793 | 1 | 1 | MAPK8IP1 | ||||
chr7:125473409-130222701 | 1 | 1 | * | chr11:68,572,941-71,610,635 | 2 | 0 | CCND1 | |||
chr8:16046364-21259961 | >5* | >5* | chr12:16001-2822554 | 1 | 1 | WNT5B | ||||
chr8:32862000-35008370 | 4 | >5 | chr12:24741983-26376173 | 2 | 5 | KRAS | ||||
chr9:1-2150635 | 3 | 2 | SMARCA2? | * | chr12:37293590-38572187 | 1 | 1 | |||
chr9:21896508-22247166 | 9 | 9 | INK/ARF | chr12:67053334-68797675 | 1 | 1 | ||||
chr9:114354535-115458858 | 2 | 1 | DEC1 | TNFSF15 | chr14:30564685-34308246 | 2 | 1 | EGLN3 | ||
chr10:18210059-18829125 | 2 | 1 | chr16:23637946-25161714 | 1 | 0 | PRKCB1 | ||||
chr10:72894101-73682797 | 1 | 1 | chr17:34082394-35383839 | 1 | 3 | ERBB2/GRB7 | ||||
chr10:100502340-105243810 | 2 | 2 | chr17:45180767-46468258 | 2 | 2 | MYST2 | ||||
1 | 1 | chr18:17996634-19733334 | 5 | 0 | MIC1/CTAGE1 | |||||
1 | 3 | chr19:34873620-36259697 | 2 | 0 | CCNE1 | |||||
chr15:54956934-55490856 | 2 | 2 | chr19:43998134-44797178 | 4 | 3 | PAK4 | IXL | |||
chr16:49473273-53566530 | 1 | 3 | RBL2 | chr20:44844257-47304215 | 1 | 5 | ||||
chr17:7524290-7864277 | >10* | >10* | p53 | chr20:50134725-52103020 | 2 | 4 | ZNF217 | |||
chr17:18063537-19492939 | 1 | 2 | ||||||||
chr17:63967955-68794146 | 1 | 3 | MAP2K6 | |||||||
chr18:46807210-47081741 | >5 | >10 | MADH4 | |||||||
chr21:41,720,024-42,590,421 | 1 | 2 |
The table is divided in two, separating amplifications and deletions. For each the first column is the May 04 genomic coordinates (Build 35), the second column is the number of primary tumors and third column is the number of cell lines that the aberration is found in. The fourth column is known genes to be involved in cancer and the fifth column is a possible candidate based on function. The last column has a star marking regions that have few if any genes. The raw and segmented copy number data for the entire dataset is available as supplemental data (http://lucitolab.cshl.edu/rl_publications.html).
Significantly, we also identified regions that are less well characterized and that were not identified earlier as being commonly altered in pancreatic or in other cancers. These regions are particularly interesting since they can be used to localize and identify genes that, although altered less frequently, may still be important for the development and or progression of pancreatic cancer. In addition, the pathways altered when these genes are mutated also provide additional candidate genes that may be targeted in pancreatic cancer.
We also analyzed the data to identify concurrent mutations that is to identify genetic changes that tend to occur together in the same carcinoma. The constraints for size of the region altered in this analysis were removed since we were not concerned with epicenter mapping but more so with the genes that were altered. The results are shown in Table 2 which represents the number of samples where two regions (the intersection on the table) are found altered together in the same cancer (a larger version of Table 2 which includes other genes altered not listed is available as Table S2). Not surprisingly, the alterations that occur together the most frequently are the most frequent genetic events-deletion of the p16/CDKN2A (INK/ARF) locus and the amplification of the KRAS2 gene, the c-myc gene, or deletion of MADH4 gene. Intriguingly, a rather frequent event was the deletion of MADH4 and the amplification of PAK4, which occurs in six samples (five of which are primary tumors).
Table 2
Genomic regions altered within the same sample
![]() |
Along the left side are the names of known genes identified in the dataset as having alterations. Along the top are the same genes forming a matrix. The color corresponds to the copy number, deletion (green) or amplified (red). The second column is the number of samples that have either amplification or deletion of the region in question. The constraints of size imposed for epicenter mapping were removed for this analysis. Other genes that were found altered not listed in Table 1 identified in this analysis due to the removal of size constraints are available as Table S2 in supplemental data.
Regions of copy number variation (CNV) in the normal population are also uncovered with high resolution CGH techniques.6,22,23,25,32 Thus, some regions of copy number fluctuation identified that are small may be CNVs. We therefore filtered out common CNVs from the data utilizing copy number data for 325 normal samples.22,23 The remaining regions of loss or gain in the carcinomas that contain no known genes may yet contain genes proposed by gene finding algorithms. However these regions may also contain unknown noncoding RNAs, such as miRNAs that could be important for the regulation of other gene products.
Almost all regions have multiple gene candidates and analyses to remove candidates to determine which candidate is the target of the copy number alteration must be performed. To illustrate, we focused on a region mapped from the dataset on chromosome 19q13 that we and others have found amplified in pancreatic cancer.11,28,30 In our dataset, with removal of genomic length constraints, this region is amplified in 7% of the carcinomas, including four primary carcinomas of which one has the highest amplification. Of the samples that have this region amplified (Fig. 3A), one of the primary carcinomas (JH0007) had the most informative amplicon (Fig. 3B green line) defining the epicenter to below 1 Mb (NCBI Build 35 coordinates chr19: 43,998,060–44,797,349). This region contains 26 gene candidates.
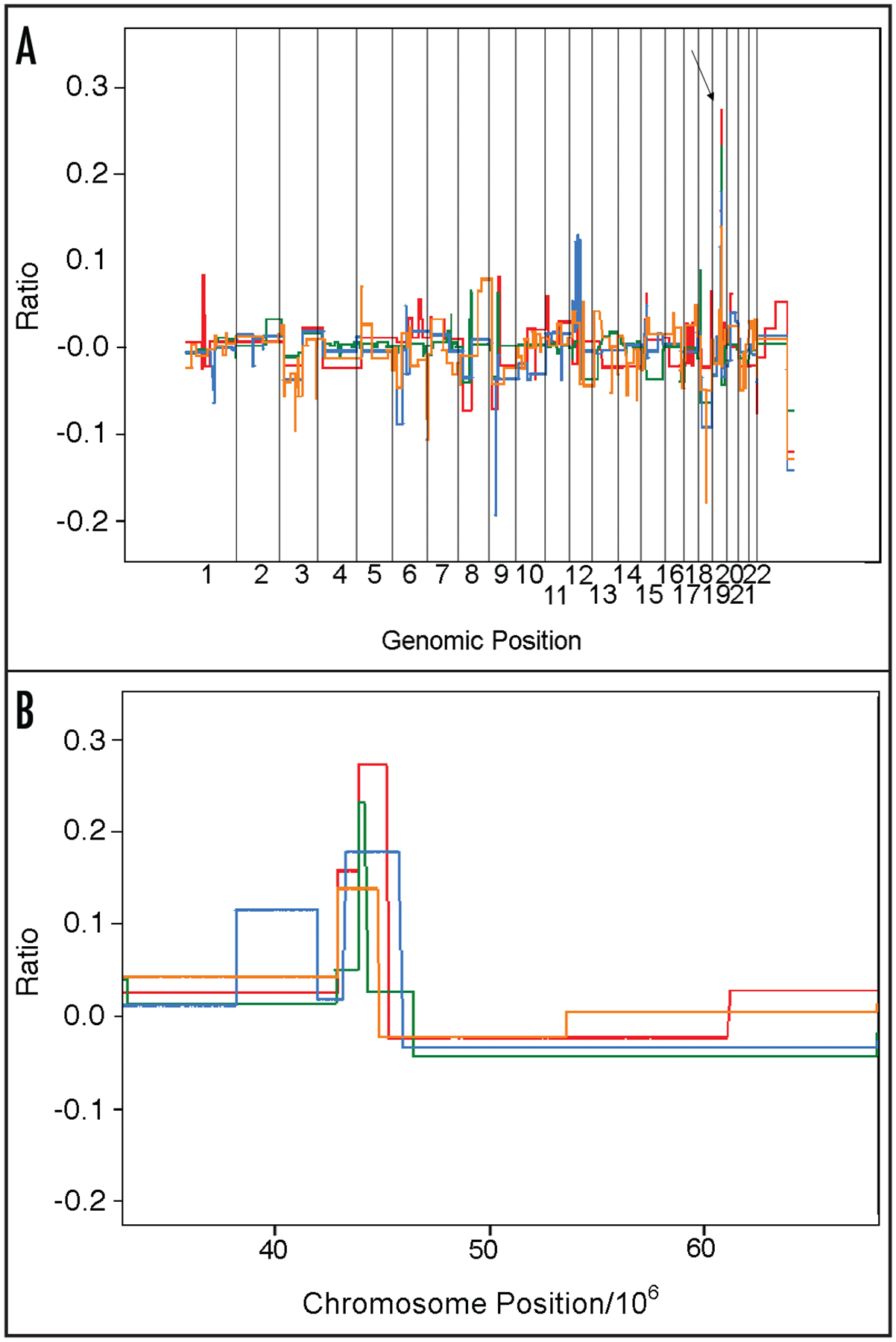
Segmentation values for four samples with genomic amplification of the 19q13 region. (A) shows the genomic profile for four samples with amplification of 19q13 region with an arrow pointing to chromosome 19. (B) shows the region of interest on the q-arm of four tumors. The X-axis is the chromosome position (divided by 106) and the Y-axis is the log ratio of the segmentation value.
Since samples with gene amplification, if the gene is biologically important in the cancer, are likely to have overexpression of the target gene, expression analysis was performed on cell lines and one primary tumor (JH0007) that had amplification of this region. Although non-target genes may be overexpressed merely as a consequence of being gene amplified, it is likely that they will not be overexpressed in all samples with gene amplification, whereas the gene targeted by the amplification should be overexpressed in all samples. After completing this analysis (Table 3), genes that were not consistently overexpressed were removed, leaving only three genes, PAF1 (PD2), IXL and PAK4. In all samples that have genomic amplification, specifically a primary carcinoma which as demonstrated below has much higher levels of the PAK4 protein as compared to normal, the PAF1, IXL and PAK4 genes are overexpressed. All are viable candidates as oncogenes in the region, since both PAK4 and PAF1 have been shown to increase anchorage independent growth under certain conditions18,33 and IXL has been shown to increase cell survival.
Table 3
Affymetrix expression data for those genes within the chromosome 19q13 epicenter of amplification
![]() |
Expression analysis was performed on the U133 Plus2 arrays. The reference sample was the cell line HPDE which is normal human pancreatic ductal epithelium. The first five columns of the table are as follows; start of the gene, end of the gene, accession ID for the gene. The remaining columns are expression data (log scale) for particular cell lines or tumor as follows; Colo320, Colo587, E3GS2.4, PANC1, SU.86.86, JH0007 as compared to the cell line HPDE. The cell lines in blue do not have genomic amplification of the region. The Accession Ids in red mark the genes with the most consistent expression pattern.
To determine their relevance to cancer we analyzed the protein level of PAK4 and PAF1 (unfortunately to date there is no high quality antibody available for the IXL protein) by immunolabeling of primary tumor JH0007, which had gene amplification. Immunolabeling with antibodies to PAK4 protein revealed strong diffuse labeling of the neoplastic cells (Fig. 4A and andB),B), with almost all of the neoplastic cells intensely labeling. By contrast, non-neoplastic pancreatic tissue did not show any labeling. Immunolabeling with antibodies to the PD2 (PAF1) protein revealed diffuse labeling of the neoplastic as well as labeling of the normal cells (Fig. 4C and andD).D). The levels of PD2 protein (PAF1) being equivalent in the normal allowed its removal as a candidate oncogene in this particular environment, which left PAK4 and IXL as the target genes of amplification. It is possible that there are two oncogenes within the region which may cooperate in pancreatic carcinogenesis as this phenomenon has been observed in other genomic regions in other cancer types.34,35 Therefore we further investigated the activation of PAK4.
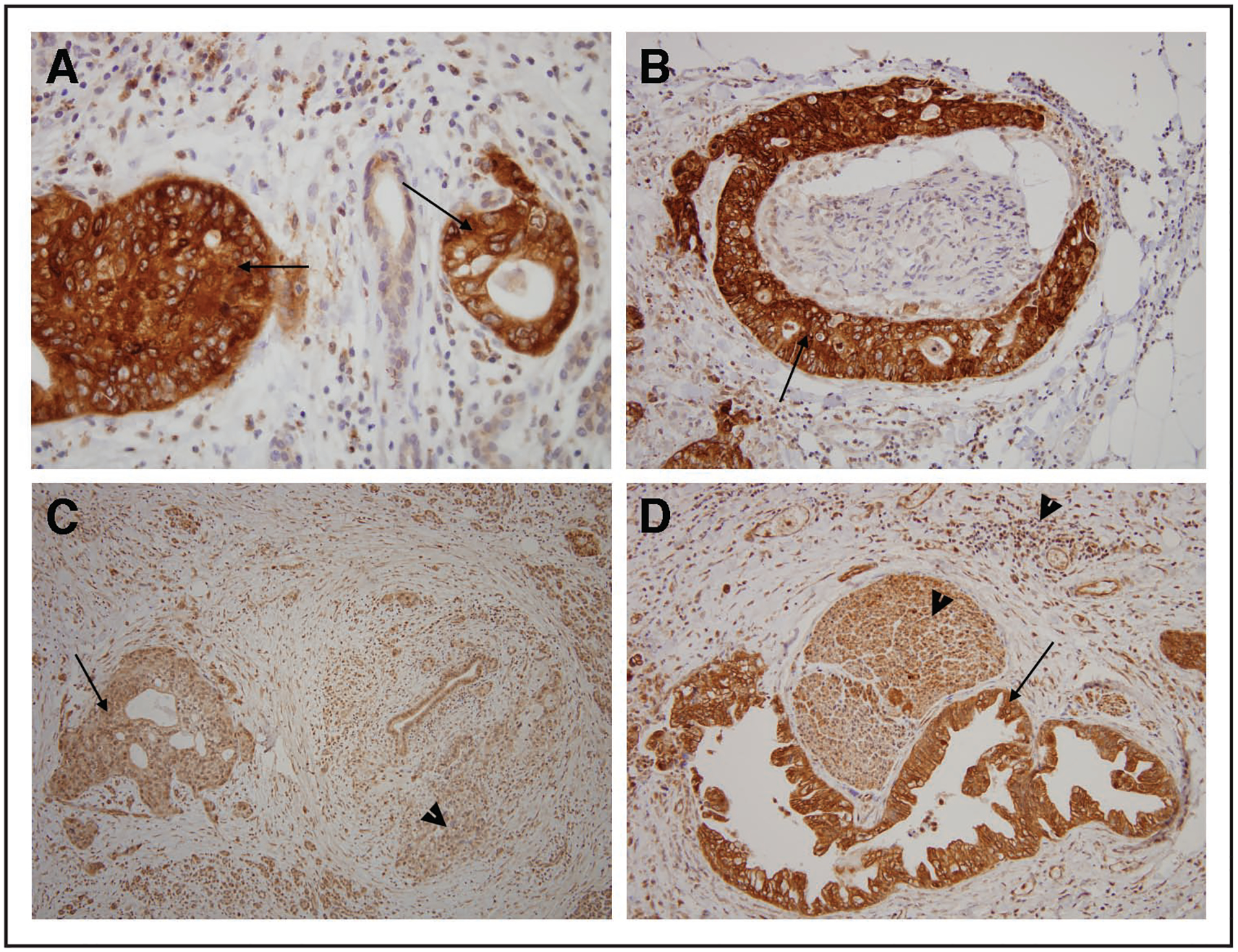
Immunohistochemistry for two genes PAF1 and PAK4 in a tumor with the chromosome 19 amplicon. (A and B) show labeling for PAK4 and (C and D) show labeling for PAF1. (B and D) are sections with perineural invasion. Arrows point to tumor and arrow heads point to normal when appropriate to demonstrate high level of protein in normal.
The PAK4 protein, one of the group B PAKs, can promote anchorage independent growth of neoplastic cells when activated.18 Activation of PAK4 can occur through amplification, point mutations, or as a result of activation of normal cellular pathways including the Ras pathway. To determine whether the PAK4 protein is activated in these cancers we performed an in-vitro kinase assay on extracts from cell lines with the amplicon Figure 5. In comparison to a pancreatic cell line that did not have amplification or overexpression of this gene, the cell lines with an amplification had 10–15 times more protein and 8–10 fold more kinase activity; demonstrating that the protein is active in samples with gene amplification and increased protein level. The activation of PAK4 by gene mutation has been shown to increase anchorage independence; however this form of PAK4 has rarely been identified in neoplasms.36 None the less, we sequenced the PAK4 gene to search for activation mutations in the samples shown to have increased PAK4 kinase activity, but we found no such mutations (Table 4).
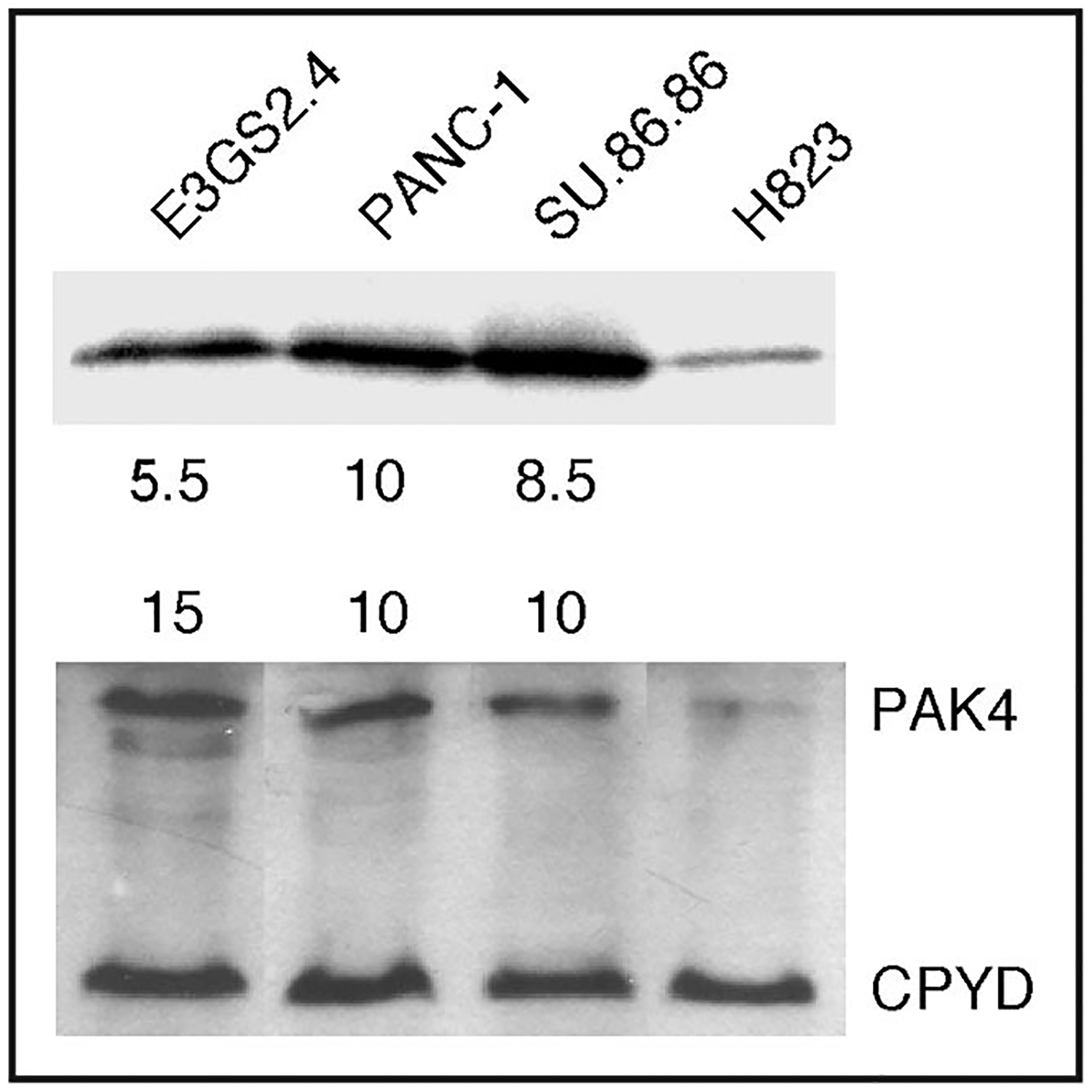
Immuno-blotting and kinase assay of PAK4 for the cell lines with the 19q13 amplicon. The cell lines EGS2.4, SU.86.86, PANC-1 and H82 were utilized to determine protein level and kinase activity. The lower panel is a Western blot of PAK4 and CPDY as a control for protein concentration to determine if the protein level of PAK4 is elevated in cell lines with gene amplification and overexpression. The upper panel shows the results of an in-vitro kinase assay performed with the same protein extracts demonstrating increased kinase activity of PAK4 in cells with gene amplification.
Table 4
Sequence analysis of k-ras and pak4 genes
RAS | PAK4 | |||||
---|---|---|---|---|---|---|
Codon 12 | 13 | 16 | 60 | 61 | 445 | 474 |
Lys (K) | Gly (G) | Lys (K) | Gly (G) | Gln (Q) | Ser (S) | Ser (S) |
Asp (D) | Gly (G) | Lys (K) | Gly (G) | Gln (Q) | Ser (S) | Ser (S) |
Asp (D) | Gly (G) | Lys (K) | Gly (G) | Gln (Q) | Ser (S) | Ser (S) |
Gly (G) | Gly (G) | Lys (K) | N/D | N/D | N/D | N/D |
Val (V) | Gly (G) | Lys (K) | N/D | N/D | N/D | N/D |
Gly (G) | Gly (G) | Lys (K) | Gly (G) | Gln (Q) | Ser (S) | Ser (S) |
Arq (R) | Gly (G) | Lys (K) | N/D | N/D | N/D | N/D |
Gly (G) | Gly (G) | Lys (K) | Gly (G) | Gln (Q) | Ser (S) | Ser (S) |
Sequencing and the coding of amino acids of the k-ras and pak4 genes is shown for codons that are the site for common activating mutations as determined by sequencing. The wild type amino acid is identified at the bottom of the table.
Since PAK4 activity appears to be required for Ras transformation,37 and KRAS2 gene activation by mutation is such a frequent event in pancreatic cancer, the KRAS2 gene was sequenced in samples with amplification of the PAK4 gene (Table 4). Codon 12 (the most frequently mutated codon) of the KRAS2 gene was mutated in 4 of the 5 samples; no other codons were found mutated. In addition, of the four samples that have KRAS2 gene mutations, three samples also were found to have genomic amplification and overexpression of the KRAS2 gene. In all of these samples with oncogenic forms of KRAS2, activation of the Ras pathway could be responsible for the activation of PAK4. Interestingly, the fifth sample, a primary carcinoma (JH0007) that had increased expression (Table 3) and increased protein (Fig. 4), no mutations in the KRAS2 or PAK4 genes (Table 4) were identified. In this sample it is possible that PAK4 is activated by a redundant or perhaps some type of RAS independent pathway.
Thus PAK4 is a target of amplification on 19q13 in pancreatic cancer and thus is likely relevant to the development and/or the progression of pancreatic cancer. The close association of KRAS2 amplification/activation and PAK4 gene amplification/activation suggests that PAK4 protein activation involves Ras activation.
Discussion
Pancreatic cancer is fundamentally a genetic disease. Regions of the pancreatic cancer genome that are either deleted or amplified can help localize tumor suppressor genes and oncogenes involved in the development and progression of this disease. We have analyzed 73 well-characterized pancreatic cancers samples and appended publicly available data for 16 more samples. We report regions recurrently amplified or deleted within the dataset as well as regions altered concurrently within the same sample. One limitation of ROMA is that it only provides information on copy number.38 Beroukim and others have shown that copy-neutral (i.e., having two copies) and copy-gain (i.e., having more than two copies) forms of allelic loss accounted for a significant proportion of losses of heterozygosity in cancer.38,39 Such alterations would not be detected in our analyses.
We identified a recurrent amplicon on chromosome 19q13 and we show that, in addition to the IXL gene, this amplicon contains a second target gene. This gene, PAK4, is a p21 activated kinase, one of the lesser studied members of the PAK family, especially in the involvement of the ras pathway.
Our data, at present is the largest CGH pancreatic tumor dataset and we have made this resource available to the cancer community (http://lucitolab.cshl.edu/rl_publications.html) to allow others to benefit from the interpretation of this valuable dataset and to identify other genes involved in the progression of pancreatic cancer. We anticipate that this dataset will be particularly useful as groups plan and undertake large-scale sequencing of the pancreatic cancer genome.40,41 The genes included within amplicons and deletions can be prioritized for sequencing, or, conversely, genes found to be mutated in pancreatic cancer can be prioritized for follow-up studies if they lie within a recurrent amplification or deletion.
Many more regions of the genome were found altered than are reported in Table 1. Because of space limitations we have only reported regions that have an epicenter of less that 4Mb or fewer than 20 genes. The constraints of size were removed to determine how frequently specific regions were altered in the same samples. The data were presented as pairs, or how many samples any two genes would be found altered.
There are also small regions (listed in Table 1) likely to be legitimate targets of amplification or deletion in the cancer since they have been found altered in more than one sample. These small regions are of particular interest since most contain few if any genes.
Given the number of normal samples (325, see methods) used to remove normal genetic variation (i.e., CNVs) it is unlikely that any remaining regions were common CNVs. An interesting possibility is that these regions could be CNVs that associate with hereditary pancreatic cancer and may contain a tumor suppressor.32 As a consequence these regions would be expected to be enriched in the tumor population. Further analyses of possible frequency differences for these regions and the normal population are ongoing.
Although we observed significant agreement in the copy number profile frequency plots of cell lines and primary carcinomas, there were significant disparities (see Fig. 2), which could be caused by the cultured cells non-specifically accumulating mutations or accumulating mutations which help the cells adapt to tissue culture conditions. Our algorithm to identify regions took this into account. We found that the data from the cell lines were very informative, often having smaller lesions than primary carcinomas and xenografts. These smaller regions can be used to delimit more finely the epicenter of mutation, but should not be used to identify a region of importance. There are of course primary carcinoma samples which have relatively small lesions, an example being the region on chromosome 19 harboring several gene candidates.
Most recently the IXL, SUPT5H and ZFP36 genes were identified as targets of this region and all three were shown to have an effect on cell growth.12 Although we did not, Kuuselo and colleagues determined that PAK4 is amplified to a lesser extent and have excluded PAK4 from their analysis. Our expression analysis removes SUPT5H and ZFP36 as critical candidates since they are not consistently overexpressed, leaving IXL, PAK4 and PAF1. PAF1 was removed as a viable candidate gene because we found that the protein levels did not correlate with mRNA levels, a finding we have observed with other amplified genes (unpublished observation). Thus, we believe that PAK4 and IXL are the most viable targets for the chromosome 19q13 amplification.
We have shown that in addition to there being overexpression, that there is increased protein and that the kinase activity of PAK4 is elevated. The inhibition of kinase activity of group II Pak’s, of which PAK4 is a member, is not as well understood as is the auto inhibitory domain of the group I Pak’s. However recent evidence suggests that the inhibition of PAK4 kinase may be similar to that of the group I Paks where a segment of the protein folds over to inhibit kinase activity. This inhibition42 possibly requires associating with another factor such as Cdc42 for activation. It is known that the PAK4 gene is mutated in some cancers36 and when mutated/activated, PAK4 can promote anchorage independent growth.18 We have functionally validated the PAK4 gene as the target of amplification in pancreatic cancer based on its increased functional activity. We have demonstrated that the PAK4 protein can be active in pancreatic cancer cells without the necessity of mutation and that the activation of PAK4 can be a consequence of KRAS2 gene mutation, often in conjunction with KRAS2 gene amplification and overexpression, as well as a possible Ras independent mechanism. The PAK4 protein through its interation with Cdc42 is involved in altering the cytoskeleton by promoting the formation of filopodia.13 We propose that activation of PAK4 is critical to neoplastic pancreatic cells as they transition to invasiveness. We are currently investigating this potential.
Pancreatic cancer is not the only type of cancer that has frequent amplification of this region. This region is amplified in other tumor types including ovarian and breast cancer. In addition, the kinase activity of PAK 4 is increased in some breast cancer samples with genomic amplification (unpublished observation Lucito). The PAK4 gene is frequently overexpressed without the presence of gene activating mutation in many tumor types other than pancreatic cancer37 suggesting that this gene is an important target during carcinogenesis. We are currently continuing our studies of PAK4 involvement in pancreatic cancer including the possible cooperation with IXL.
In summary we have amassed the largest CGH dataset for pancreatic cancer and present the regions commonly altered. This dataset was used to identify candidate target genes within an amplicon on chromosome19q13 and other methods were utilized to identify the target of amplification, demonstrating the value of the dataset. The dataset we present will be particularly useful for the pancreatic cancer community as efforts are undertaken to sequence the pancreatic cancer genome.
Materials and Methods
Materials.
Enzymes; BglII, T4 DNA ligase, were supplied by new England Biolabs. Primers were supplied by Sigma Genosys. Cot1 DNA and tRNA were supplied by invitrogen. The Megaprime labeling kit, Cy3-conjugated dCTP and Cy5-conjugated dCTP were supplied by Amersham-Bioscience. Taq polymerase (Eppendorf mastermix (2.5X)) was supplied by Eppendorf. Centricon YM-30 filters were supplied by Amicon and formamide was supplied by Amresco. Phenol: chloroform was supplied by Sigma. NimbleGen photoprint arrays were synthesized by NimbleGen Systems Inc., Design of the ROMA array was described previously.6
ROMA.
Arrays were described previously.6 In brief the arrays are based on representational techniques and thus all oligonucleotides map to BglII fragments that are within the representations size range of 200–1000 bp. The array was designed to June 2002 (NCBI Build 30) and the coordinates have been updated to May 2004 (NCBI Build 35). There are over 80,000 features on the array and these are scattered across the genome resulting in an average resolution of 30 kb. All samples without a matched reference were compared to CHP-SKN-1 a normal male fibroblast cell line we use routinely for all unmatched sample analysis. Sample preparation, BglII representations, has been described previously.19 Minor changes in the protocol are as follows: as few as four 250-μL tubes were used for each sample for amplification of the representation each with a 100 ul volume reaction. An equal number of tubes were used for reference for each sample that was co-amplified. The cycle conditions were 95°C for 1 min, 72°C for 3 min, for 20 cycles, followed by a 10-min extension at 72°C. The contents of the tubes were pooled when completed. Representations were cleaned by phenol: chloroform extraction, precipitated, resuspended and the concentration determined. DNA was labeled as described with minor changes.19 Briefly, 2 ug of DNA template was placed (dissolved in TE at pH 8) in a 0.2-mL PCR tube. 5 μL of random nonamers from Sigma Geneosys were added, the volume brought up to 50 μL with dH2O and mixed. The tubes were placed in Tetrad at 100°C for 5 min, then on ice for five minutes. To this 10 μL of buffer two from NEB and 6 ul of dNTP mix (Adenine, guanine and thiamine are 1.2 mM and cytosine is 0.6 Mm), 5 μL of label (Cy3-dCTP or Cy5-dCTP) and 1 μL of NEB Klenow fragment were added. Procedures were followed as reported previously.20 Arrays were scanned with an Axon GenePix 4000B scanner set at a pixel size of 5 μm. GenePix Pro 4.0 software was used to quantify the intensity for the arrays. Array data were imported into S-PLUS for further analysis. Measured intensities without background subtraction were used to calculate ratios. Data were normalized using an intensity-based lowest curve fitting algorithm.
Segmentation.
To decrease or remove probe to probe variation various algorithms can be used. We utilize an algorithm developed by Adam Olshen and colleagues21 called Circular Binary Briefly, the algorithm recursively splits chromosomes into subsegments of at least three probes in size by testing the change-points until none can be found as significant within the existing subsegments, as determined by p-value (α = 0.05) obtained through a hybrid approach. A split point will be removed if the difference between the means of adjacent segments is less than 1.0 SD of the 2.5%-trimmed data. The code for this algorithm is freely available (http://bioconductor.org/packages/2.1/bioc/html/DNAcopy.html).
Copy number data from the Aguirre et al. data set were segmented with methods developed by Dr. Adam Olshen.21 Frequency plots of the data were generated by identifying probes that were 1 SD above and calculating the frequency this occurs.
CNV subtraction.
When utilizing high resolution arrays, germline CNVs will be identified when comparing cancer to normal from a different person. In the present study, common CNVs found in the normal population were filtered out utilizing a set of 325 normal patients.22,23 The dataset if based on all probes would require a large data frame to represent all samples and would drastically slow down computing time. Much of the data from the genome reports non-events or vast regions without CNVs. Therefore, the probe based data is reduced to event based data. In other words only the CNVs, location and ratio, are represented in a file, thereby drastically reducing size without decreasing the information content. This transformation was performed for both the pancreatic cancer and the normal filter sample datasets and filtration processed as follows. The data can be thought of being reduced to binary, either altered or not altered. The test set is compared to the normal set, if a region altered in the test set is found altered in the normal set, this region is removed from the test set.
Expression analysis.
Total RNA was isolated from the cell lines and from the primary tumor using the Qiagen RNeasy kit and the integrity was checked on an Agilent bioanalyzer. Five ug of total RNA was used to produce ds cDNA using the Superscript Choice Labeling Kit (Invitrogen). The product was used as template to produce biotynilated cRNA with the Bioarray high yield RNA transcript labeling kit (Enzo). Twenty ug of biotynilated cRNA was fragmented with reagents from the Superscript Choice Labeling kit. Control oligos (Affymetrix) were spiked into the fragmented product and the material was hybridized to U133 Plus arrays, washed and scanned according to Affymetrix protocols (https://www.affymetrix.com/support/downloads/manuals/expression_s2_manual.pdf). Probe level analysis was done using the “affy” package from the Bioconductor www.bioconductor. org. Briefly the data intensity values for the probe set were extracted by applying the RMA algorithm.24 Ratios were calculated on log base two scale.
Immunoblotting.
A 10 cm plate of cells were lysed in RIPA buffer and collected after scraping. Equal amounts of protein (15 ug) were separated on 10% SDS-polyacrylamide gels and transferred to nitrocellulose membranes. The blots were probed with primary antibodies against human PAK4 (Cell Signaling Tech. cat #3242) the control Ab being Lamin A/C (Santa Cruze Biotech.), both at 1:1000. Blots were then probed with HRP-conjugated secondary antibodies and visualized by chemiluminescence (Santa Cruz Biotech).
Immunolabeling.
All immunolabeling was performed at room temperature and carried out on the Dako Autostainer.(DakoCytomation-Carpinteria, CA). Reagents were used as supplied in the Mach 4* Universal HRP-Polymer Kit, (BioCare Medical, Walnut Creek, CA). BioCare Diva, ph 6.6 was used. Optimum primary antibody (Cell Signalling Tech cat #3242) dilutions were predetermined using known positive control tissues. A known positive control section was included in each run to assure proper labeling. Paraffin sections were cut at 4 μm on a rotary microtome, mounted on positively charged glass slides (Superfrost, Fisher) and baked one hour. Sections were then deparaffinized in xylene and ethanol. Sections were then placed in 200 ml Diva, pH 6.6. The buffer was brought to a temperature of 95 degrees and timed for 40 min. Sections were then removed and allowed to cool in buffer for 5 min, following which they were rinsed thoroughly in deionized water. The sections were then loaded onto the Dako Autostainer. Sections were first quenched with 3% H2O2 for 5 min and the rinsed in buffer. Sections were then incubated with primary antibody. Following removal of primary antibody the sections were incubated for 15 min in BioCare Mach 4* Mouse Probe rinsed in buffer and then incubated for 15 min in MR HRP-Polymer. After rinsing in buffer, the sections were then incubated in a freshly prepared mixture of diaminobenzidine (DAB) and buffer substrate solution for 5 min. Sections were then counterstained with hematoxylin and blued in Richard Allen Bluing Reagent TM, dehydrated in a graded series of ethanols and xylene and coverslipped. Slides were reviewed by light microscopy.
Immunoprecipitation and kinase assay.
Methods were similar to those used in Bagrodia et al. with minor changes.14 Briefly, cells were lysed in 40 mM Hepes (pH 7.4), 1% Nonidet P-40, 100 mM NaCl, 1 m MEDTA, 10 ug/ml leupeptin and 10 ug/ml PMSF and centrifuged at 12,000 xg for 15 min at 4°C. Lysates were precleared with IgG and protein A/G PLUS agarose (santa cruz biotech cat #sc2003). 10 ul of PAK4 Ab (Cell Signalling Tech. cat #3242) was added to the precleared lysates and incubated on ice for 60 min followed by protein A/G PLUS agarose (Santa Cruz Biotech cat #sc2003) for 30 min. the immunoprecipitate was spun and washed with lysate buffer four times and then washed with 2X phosphorylation buffer (10 mM MgCl2, 40 mM Hepes (pH 4.7)). To the tube the following was added; 5 ug myelin basic protein (Sigma cat# M1891) 10 uCi of gamma 32P ATP (3000 Ci/mmol) and 20 uM ATP in 30 ul total volume for 20 minutes at RT. Protein gel loading buffer was added and the samples was run on SDS PAGE, transferred to a membrane and autoradiography performed.
Acknowledgements
We thank Linda Van Aelst for critical comments on the manuscript. We also thank Sohail Khan and Christopher Johns for data analysis. This work was supported by grants awards to R.L. from the National Institutes of Health and NCI (K01CA93634-01), the department of Defense (W81XWH-05-1-0068) and the Lustgarten Foundation, as well as by grants awarded to R.H.: The Sol Goldman Pancreatic Cancer Research Center and the NIH SPORE (Specialized Programs of Research Excellence) in Gastrointestinal Cancer Grant CA62924 and grants awarded to D.V.H. and H.H.:(CA109552-0141). Some tumor samples were supplied by the Cooperative Human Tissue Network, which is funded by the National Cancer Institute. Other investigators may have received samples from these same tissues.
Footnotes
Supplementary materials can be found at: www.landesbioscience.com/supplement/ChenCBT7-11-Sup.pdf
References
Full text links
Read article at publisher's site: https://doi.org/10.4161/cbt.7.11.6840
Read article for free, from open access legal sources, via Unpaywall:
https://www.tandfonline.com/doi/pdf/10.4161/cbt.7.11.6840?needAccess=true
Citations & impact
Impact metrics
Citations of article over time
Article citations
PAK4 inhibition augments anti-tumour effect by immunomodulation in oral squamous cell carcinoma.
Sci Rep, 14(1):14092, 18 Jun 2024
Cited by: 1 article | PMID: 38890401 | PMCID: PMC11189426
Copy Number Variations in Pancreatic Cancer: From Biological Significance to Clinical Utility.
Int J Mol Sci, 25(1):391, 27 Dec 2023
Cited by: 1 article | PMID: 38203561 | PMCID: PMC10779192
Review Free full text in Europe PMC
Anticancer Efficacy of KRASG12C Inhibitors Is Potentiated by PAK4 Inhibitor KPT9274 in Preclinical Models of KRASG12C-Mutant Pancreatic and Lung Cancers.
Mol Cancer Ther, 22(12):1422-1433, 01 Dec 2023
Cited by: 1 article | PMID: 37703579 | PMCID: PMC10690049
PAK4 inhibition significantly potentiates Gemcitabine activity in PDAC cells via inhibition of Wnt/β-catenin, p-ERK/MAPK and p-AKT/PI3K pathways.
Biochem Biophys Rep, 35:101544, 09 Sep 2023
Cited by: 1 article | PMID: 37720313 | PMCID: PMC10500449
Comprehensive analysis of the prognostic implications and functional exploration of PAK gene family in human cancer.
Cancer Cell Int, 22(1):275, 05 Sep 2022
Cited by: 5 articles | PMID: 36064705 | PMCID: PMC9442929
Go to all (80) article citations
Data
Similar Articles
To arrive at the top five similar articles we use a word-weighted algorithm to compare words from the Title and Abstract of each citation.
Detailed genomic mapping and expression analyses of 12p amplifications in pancreatic carcinomas reveal a 3.5-Mb target region for amplification.
Genes Chromosomes Cancer, 34(2):211-223, 01 Jun 2002
Cited by: 25 articles | PMID: 11979555
Identification of PAK4 as a putative target gene for amplification within 19q13.12-q13.2 in oral squamous-cell carcinoma.
Cancer Sci, 100(10):1908-1916, 23 Jun 2009
Cited by: 36 articles | PMID: 19594544
High-resolution genomic and expression profiling reveals 105 putative amplification target genes in pancreatic cancer.
Neoplasia, 6(5):432-439, 01 Sep 2004
Cited by: 72 articles | PMID: 15548351 | PMCID: PMC1550328
Regulation of KRAS-PAK4 axis by microRNAs in cancer.
Curr Pharm Des, 20(33):5275-5278, 01 Jan 2014
Cited by: 2 articles | PMID: 24479809
Review
Funding
Funders who supported this work.
NCI NIH HHS (6)
Grant ID: CA62924
Grant ID: K01 CA093634
Grant ID: CA109552-0141
Grant ID: K01CA93634-01
Grant ID: P01 CA109552
Grant ID: P50 CA062924