Abstract
Background
Patients hospitalized with coronavirus disease 2019 (COVID-19) frequently require mechanical ventilation and have high mortality rates. However, the impact of viral burden on these outcomes is unknown.Methods
We conducted a retrospective cohort study of patients hospitalized with COVID-19 from 30 March 2020 to 30 April 2020 at 2 hospitals in New York City. Severe acute respiratory syndrome coronavirus 2 (SARS-CoV-2) viral load was assessed using cycle threshold (Ct) values from a reverse transcription-polymerase chain reaction assay applied to nasopharyngeal swab samples. We compared characteristics and outcomes of patients with high, medium, and low admission viral loads and assessed whether viral load was independently associated with intubation and in-hospital mortality.Results
We evaluated 678 patients with COVID-19. Higher viral load was associated with increased age, comorbidities, smoking status, and recent chemotherapy. In-hospital mortality was 35.0% (Ct <25; n = 220), 17.6% (Ct 25-30; n = 216), and 6.2% (Ct >30; n = 242) with high, medium, and low viral loads, respectively (P < .001). The risk of intubation was also higher in patients with a high viral load (29.1%) compared with those with a medium (20.8%) or low viral load (14.9%; P < .001). High viral load was independently associated with mortality (adjusted odds ratio [aOR], 6.05; 95% confidence interval [CI], 2.92-12.52) and intubation (aOR, 2.73; 95% CI, 1.68-4.44).Conclusions
Admission SARS-CoV-2 viral load among hospitalized patients with COVID-19 independently correlates with the risk of intubation and in-hospital mortality. Providing this information to clinicians could potentially be used to guide patient care.Free full text

Impact of Severe Acute Respiratory Syndrome Coronavirus 2 Viral Load on Risk of Intubation and Mortality Among Hospitalized Patients With Coronavirus Disease 2019
Abstract
Background
Patients hospitalized with coronavirus disease 2019 (COVID-19) frequently require mechanical ventilation and have high mortality rates. However, the impact of viral burden on these outcomes is unknown.
Methods
We conducted a retrospective cohort study of patients hospitalized with COVID-19 from 30 March 2020 to 30 April 2020 at 2 hospitals in New York City. Severe acute respiratory syndrome coronavirus 2 (SARS-CoV-2) viral load was assessed using cycle threshold (Ct) values from a reverse transcription-polymerase chain reaction assay applied to nasopharyngeal swab samples. We compared characteristics and outcomes of patients with high, medium, and low admission viral loads and assessed whether viral load was independently associated with intubation and in-hospital mortality.
Results
We evaluated 678 patients with COVID-19. Higher viral load was associated with increased age, comorbidities, smoking status, and recent chemotherapy. In-hospital mortality was 35.0% (Ct <25; n = 220), 17.6% (Ct 25–30; n = 216), and 6.2% (Ct >30; n = 242) with high, medium, and low viral loads, respectively (P < .001). The risk of intubation was also higher in patients with a high viral load (29.1%) compared with those with a medium (20.8%) or low viral load (14.9%; P < .001). High viral load was independently associated with mortality (adjusted odds ratio [aOR], 6.05; 95% confidence interval [CI], 2.92–12.52) and intubation (aOR, 2.73; 95% CI, 1.68–4.44).
Conclusions
Admission SARS-CoV-2 viral load among hospitalized patients with COVID-19 independently correlates with the risk of intubation and in-hospital mortality. Providing this information to clinicians could potentially be used to guide patient care.
(See the Editorial Commentary by Mathers on pages e4206–7.)
Severe acute respiratory syndrome coronavirus 2 (SARS-CoV-2) is a novel pathogen that has rapidly caused a devastating pandemic of coronavirus disease 2019 (COVID-19). As of 10 June 2020, SARS-CoV-2 had infected more than 7 million people and killed more than 400 000 people throughout the world [1]. Although the majority of patients who develop COVID-19 have mild presentations [2], 18%–33% of patients who are hospitalized require mechanical ventilation and up to 20% of hospitalized patients die [3–7]. Investigations of risk factors for intubation and mortality with COVID-19 in hospitalized patients have largely focused on patient characteristics, such as older age, obesity, and comorbidities, as well as presenting symptoms and laboratory parameters [5, 7–9]. In contrast, the impact of SARS-CoV-2 viral load on clinical outcomes in hospitalized patients has not been thoroughly investigated. In 2 studies of hospitalized patients in China, those with severe presentations of COVID-19 had higher viral loads than those with mild presentations. However, the impact of SARS-CoV-2 viral load on the risk of intubation or death was not evaluated [10, 11].
The current standard-of-care test to diagnose COVID-19 is to collect a nasopharyngeal (NP) swab and use a reverse transcription-polymerase chain reaction (RT-PCR) assay to detect SARS-CoV-2 RNA [12]. These RT-PCR assays provide clinicians only with information on whether SARS-CoV-2 is detected or not detected. However, these assays also contain quantitative information on cycle threshold (Ct) values that are inversely correlated with viral load and are not reported clinically. We hypothesized that assessing SARS-CoV-2 viral load by analyzing Ct values from an initial NP swab sample could be a clinically valuable tool for identifying patients at highest risk of intubation and death and provide insights into the pathogenesis of COVID-19. We therefore conducted this retrospective analysis of SARS-CoV-2 viral loads on admission, clinical presentations, and outcomes at 2 affiliated New York City hospitals using a high-throughput RT-PCR assay.
METHODS
Study Population and Setting
This retrospective, observational study consisted of all patients who were hospitalized at NewYork–Presbyterian Hospital/Weill Cornell Medical Center and affiliated Lower Manhattan Hospital and had an NP swab sample collected and analyzed for SARS-CoV-2 using the cobas 6800 RT-PCR System (Roche Molecular Systems, Inc., Branchburg, NJ) between 30 March 2020 and 30 April 2020. The predominant NP swab collection and transport kits used were the BD Universal Viral Transport System (Becton, Dickinson and Company, Franklin Lakes, NJ) and the Universal Transport Medium (Hardy Diagnostics, Santa Maria, CA). Patients who did not have an NP swab sample collected and analyzed within 1 day of hospital admission or whose sample was analyzed on a different diagnostic platform or at a different institution were excluded. The policy during the study period was to only perform SARS-CoV-2 tests in patients who were thought to require hospital admission. However, some patients who were tested were subsequently discharged from the emergency department (ED) without hospital admission.
Viral Load Assessment
The US Food and Drug Administration provided emergency use authorization approval for the cobas SARS-CoV-2 RT-PCR test, and the test was performed according to the manufacturer’s instructions [13]. This assay amplifies 2 targets within the SARS-CoV-2 genome: ORF1ab, a SARS-CoV-2–specific target, and the E gene, a pan-Sarbecovirus target that is present in SARS-CoV-2 and SARS-CoV but not in seasonal coronaviruses or Middle East respiratory syndrome CoV. For routine clinical care, results are classified as detected if either the ORF1ab or E gene is detected or as not detected if neither target is detected. However, the instrument also generates a Ct value for each target that correlates inversely with quantitative viral load and is not released to clinicians. The Ct value represents the number of replication cycles required for sufficient gene amplification to produce a fluorescent signal that crosses a predefined threshold.
For this study, we reviewed Ct values for both gene targets for all initial SARS-CoV-2 tests that were performed on NP swab samples that were collected from study participants for routine clinical care within 1 day of hospital admission. We separated the Ct values for the SARS-CoV-2–specific target (ORF1ab) into terciles based on the quantitative values. We then designated high viral load samples as the lowest Ct tercile, medium viral load samples as the middle tercile, and low viral load samples as the highest tercile. Specimens that were designated positive for SARS-CoV-2 but for which only the E gene was detected were designated low viral load samples.
Data Collection
Data were retrospectively abstracted manually from the electronic medical record using a quality-controlled protocol and entered into a REDCap database [14]. All data collectors were trained, and a random resampling of data previously showed high interrater reliability (mean Cohen’s kappa of 0.92) [5]. Data included demographics, comorbidities, social characteristics, selected outpatient medications on admission, presenting symptoms on arrival to the hospital, oxygen supplementation required within 3 hours of presentation, laboratory parameters, chest radiograph findings, concurrent bloodstream infections, in-hospital complications, and in-hospital mortality. Clinical data after hospital discharge were not consistently available, thus only outcomes that occurred during the hospital admission were analyzed. The Weill Cornell Medicine Institutional Review Board approved the study with a waiver of informed consent.
Statistical Analyses
We compared baseline characteristics and outcomes of hospitalized patients with COVID-19 who had high, medium, and low initial viral loads using the nonparametric nptrend command in Stata (StataCorp, College Station, TX) that tests for trend across ordered groups. Continuous variables were represented with medians and interquartile ranges and categorical variables were represented as proportions. A 2-sided P value of ≤ .05 was used to designate statistical significance. The risk of in-hospital intubation and death was also compared across 8 numerical Ct value ranges. We also constructed Cox proportional hazards models to compare the cumulative risks of intubation and death during the inpatient admission among patients with high, medium, and low viral loads. We then identified baseline factors that were associated with in-hospital mortality and intubation using univariate logistic regression models. All variables that were statistically significantly associated with each outcome were then entered into separate multivariate logistic regression models. Adjusted odds ratios of mortality and intubation were calculated for each of these variables with 95% confidence intervals (CIs). Analyses were conducted using Stata, version 15.0.
RESULTS
Cycle Threshold Values and Establishment of Viral Load Categories
A total of 678 NP swab samples were available for analysis from unique hospitalized patients who met the study inclusion criteria (Figure 1). Ct values for the ORF1ab locus ranged from 14.3 to 36.4 (Supplementary Figure), and 10 samples were considered positive for SARS-CoV-2 based on detection of the E gene even though ORF1ab was not detected. The median Ct value was 27.9. The lowest third of Ct values were <25.2, the middle third were between 25.2 and 30.3, and the highest third were >30.3. For simplicity, we designated high viral load samples to have Ct values <25 (n = 220), medium viral load samples to have Ct values between 25 and 30 (n = 216), and low viral load samples to have Ct values >30 or for which only the E gene was detected (n = 242). Of the 49 patients who had a positive SARS-CoV-2 test but were discharged from the ED and not admitted, the median Ct value was 29.1.
Patient Characteristics and Presentations Stratified by Viral Load
The median age of patients with high, medium, and low viral loads was 72, 69, and 63 years, respectively (P < .001; Table 1). In addition to older age, patients with higher viral loads were more likely to have coronary artery disease, congestive heart failure, cerebrovascular disease, hypertension, chronic obstructive pulmonary disease (COPD), chronic kidney disease, and active cancer. They were also more likely to be a former or current smoker or have received recent chemotherapy. Patients with high viral loads had a median of 7 days from symptom onset until hospital admission compared with 8 and 10 days for patients with medium and low viral loads, respectively (P < .001). Patients with higher viral loads were also more likely to require oxygen by a nonrebreather, high-flow nasal cannula or mechanical ventilation within 3 hours of presentation to the ED but were less likely to present with fever, nausea, or vomiting. Lymphopenia, anemia, and thrombocytopenia were more common among patients with higher viral loads, whereas alanine aminotransferase elevations were less common. There were no differences in chest X-ray findings among patients with high, medium, or low viral loads. There were also no differences in viral loads among different racial or ethnic categories or between patients who did and did not use angiotensin-converting enzyme inhibitors (ACEIs), angiotensin II receptor blockers (ARBs), or hydroxychloroquine.
Table 1.
Baseline Characteristics of Hospitalized Patients With Coronavirus 2019 Stratified by Admission Viral Load
Variable | High Viral Load (Ct <25), n = 220 | Medium Viral Load (Ct 25–30), n = 216 | Low Viral Load (Ct >30), n = 242 | P Valuea |
---|---|---|---|---|
Age, years | 72 (60–81) | 69 (58–79) | 63 (50–73) | <.001 |
Female gender | 81 (36.8) | 84 (38.9) | 99 (40.9) | .37 |
Raceb (n = 585) | ||||
![]() | 89 (45.1) | 79 (43.4) | 91 (44.2) | .54 |
![]() | 25 (12.7) | 27 (14.8) | 30 (14.6) | .74 |
![]() | 42 (21.3) | 32 (17.6) | 36 (17.5) | .23 |
Hispanic ethnicityb (n = 628) | 48 (23.9) | 54 (27.1) | 60 (26.3) | .58 |
Comorbidities | ||||
![]() | 60 (27.6) | 70 (33.5) | 79 (33.3) | .20 |
![]() | 44 (20.0) | 45 (21.8) | 31 (12.8) | .039 |
![]() | 28 (12.7) | 15 (6.9) | 13 (5.4) | .004 |
![]() | 27 (12.3) | 15 (6.9) | 13 (5.4) | .007 |
![]() | 82 (37.3) | 71 (32.9) | 77 (31.8) | .22 |
![]() | 136 (61.8) | 144 (66.7) | 121 (50.0) | .008 |
![]() | 49 (22.3) | 40 (18.5) | 38 (15.7) | .07 |
![]() ![]() | 21 (9.6) | 11 (5.1) | 9 (3.7) | .009 |
![]() ![]() | 20 (9.1) | 22 (10.1) | 20 (8.3) | .75 |
![]() | 32 (14.6) | 22 (10.2) | 20 (8.3) | .032 |
![]() ![]() | 22 (10.0) | 13 (6.0) | 12 (5.0) | .035 |
![]() | 4 (1.8) | 3 (1.4) | 5 (2.1) | .83 |
![]() | 22 (10.0) | 14 (6.5) | 6 (2.5) | .001 |
![]() ![]() | 13 (5.9) | 8 (3.7) | 5 (2.1) | .032 |
![]() ![]() | 9 (4.1) | 6 (2.8) | 2 (0.8) | .025 |
![]() | 15 (6.8) | 8 (3.7) | 9 (3.7) | .12 |
![]() | 12 (5.5) | 13 (6.0) | 12 (5.0) | .81 |
Social characteristics | ||||
![]() | 78 (35.5) | 54 (25.0) | 62 (25.6) | .022 |
![]() | 41 (18.6) | 41 (19.0) | 34 (14.1) | .18 |
![]() | 7 (3.2) | 10 (4.6) | 9 (3.7) | .80 |
Home medications | ||||
![]() | 68 (30.9) | 74 (34.3) | 68 (28.1) | .49 |
![]() | 5 (2.3) | 1 (0.5) | 9 (3.7) | .27 |
![]() | 27 (12.3) | 21 (9.7) | 19 (7.9) | .11 |
![]() ![]() | 13 (5.9) | 11 (5.1) | 9 (3.7) | .19 |
![]() ![]() | 11 (5.0) | 7 (3.2) | 9 (3.7) | .49 |
![]() ![]() | 12 (5.5) | 7 (3.2) | 9 (3.7) | .36 |
Chemotherapy within the previous 6 months | 9 (4.1) | 3 (1.4) | 1 (0.4) | .004 |
Clinical presentation | ||||
![]() | 7 (3–9) | 8 (5–13) | 10 (6–14) | <.001 |
![]() | ||||
![]() ![]() | 138 (62.7) | 152 (70.4) | 173 (71.5) | .046 |
![]() ![]() | 160 (72.7) | 148 (68.5) | 166 (68.6) | .34 |
![]() ![]() | 156 (70.9) | 136 (63.0) | 169 (69.8) | .85 |
![]() ![]() | 18 (8.2) | 17 (7.9) | 11 (4.6) | .12 |
![]() ![]() | 16 (7.3) | 15 (6.9) | 25 (10.3) | .23 |
![]() ![]() | 38 (17.3) | 41 (19.0) | 54 (22.3) | .17 |
![]() ![]() | 30 (13.6) | 31 (14.3) | 51 (21.1) | .03 |
![]() ![]() | 49 (22.3) | 50 (23.2) | 68 (28.1) | .14 |
![]() ![]() | 38 (17.3) | 41 (19.0) | 29 (12.0) | .11 |
![]() ![]() | 7 (3.2) | 12 (5.6) | 14 (5.8) | .20 |
![]() ![]() | 12 (5.5) | 20 (9.3) | 20 (8.3) | .27 |
Highest oxygen requirement within 3 hours of arrival to the emergency department | ||||
![]() | 78 (35.5) | 84 (38.9) | 92 (38.0) | .58 |
![]() | 81 (36.8) | 86 (39.8) | 113 (46.7) | .03 |
![]() | 50 (22.7) | 38 (17.6) | 33 (13.6) | .011 |
![]() | 11 (5.0) | 8 (3.7) | 4 (1.7) | .046 |
Laboratory values | ||||
![]() | 41 (22.2) | 39 (19.8) | 40 (18.6) | .46 |
![]() | 135 (71.8) | 140 (72.5) | 127 (59.6) | .008 |
![]() | 52 (27.4) | 45 (22.8) | 41 (19.1) | .048 |
![]() | 46 (24.2) | 45 (22.8) | 26 (12.1) | .002 |
![]() | 118 (64.5) | 144 (75.4) | 142 (68.9) | .38 |
![]() | 46 (24.7) | 44 (22.8) | 79 (37.6) | .004 |
![]() | 17 (14.5) | 9 (8.4) | 5 (6.8) | .07 |
Inflammatory makers | ||||
![]() | 0.26 (0.12–0.64) | 0.22 (0.1–0.56) | 0.2 (0.11–0.45) | .12 |
![]() | 12.9 (7.8–20.4) | 11.1 (6.3–19.0) | 11.4 (6.7–20.2) | .57 |
![]() | 849 (409–1417) | 842 (409–1542) | 821 (340–1361) | .42 |
![]() | 535 (309–990) | 472 (300–980) | 547 (354–1716) | .17 |
Chest X-ray findings | ||||
![]() | 22 (10.0) | 13 (6.0) | 17 (7.0) | .24 |
![]() | 27 (12.3) | 23 (10.7) | 25 (10.3) | .51 |
![]() | 166 (75.5) | 169 (78.2) | 194 (80.2) | .22 |
![]() | 8 (3.6) | 5 (2.3) | 3 (1.2) | .09 |
Outcomes | ||||
![]() | 64 (29.1) | 45 (20.8) | 36 (14.9) | <.001 |
![]() ![]() | 2 (0–3) | 2 (0–4) | 2 (0–5) | .66 |
![]() | 77 (35.0) | 36 (17.6) | 14 (6.2) | <.001 |
![]() ![]() | 7 (4–14) | 8 (3–15) | 10 (3–32) | .32 |
Other complications | ||||
![]() | 16 (7.3) | 10 (4.6) | 5 (2.1) | .007 |
![]() | 14 (6.4) | 6 (2.8) | 6 (2.5) | .032 |
![]() | 29 (13.2) | 18 (8.3) | 20 (8.3) | .08 |
![]() | 33 (15.0) | 18 (8.3) | 7 (2.9) | <.001 |
Variables are expressed as number (%) or median (interquartile range). Bolded values indicated variables with statistically significant associations.
Abbreviation: Ct, cycle threshold.
a P values were calculated using the nonparametric nptrend command in Stata, version 15.0, that tests for trend across ordered groups.
bThis variable was not assessed in all participants. The denominator is listed next to the variable.
cAspartate aminotransferase elevation indicates a value >34 units/L.
dAlanine aminotransferase elevation indicates a value >55 units/L.
Outcomes Stratified by Viral Load
The last day of study follow-up was 8 June 2020. By that day, 19.2% of patients had died during their admission, 75.8% had been discharged alive, 1.6% had been transferred to another hospital, and 3.4% were still hospitalized. The risk of intubation and death increased with higher viral loads. In-hospital mortality was 35.0% in patients with a high viral load compared with 17.6% in patients with a medium viral load and 6.2% in patients with a low viral load (P < .001; Table 1). The risk of intubation was 29.1% in patients with a high viral load compared with 20.8% and 14.9% in patients with a medium or low viral load, respectively (P < .001; Table 1). These associations were also observed in time-based analyses (Figure 2) in which, compared with a low viral load, a high viral load was associated with a hazard ratio (HR) of 5.06 (95% CI, 2.86–8.96; P < .001) for in-hospital mortality and an HR of 2.15 (95% CI, 1.31–3.53; P = .003) for intubation, whereas a medium viral load was associated with mortality (HR, 2.52; 95% CI, 1.36–4.67; P = .003) but not with risk of intubation (HR, 1.53; 95% CI, .91–2.60; P = .11). When viral load was assessed by continuous Ct values instead of being grouped into high, medium, and low viral load categories, the risk of death increased with decreasing Ct values and the risk of intubation was greater with Ct values <27 compared with >27 (Figure 3).
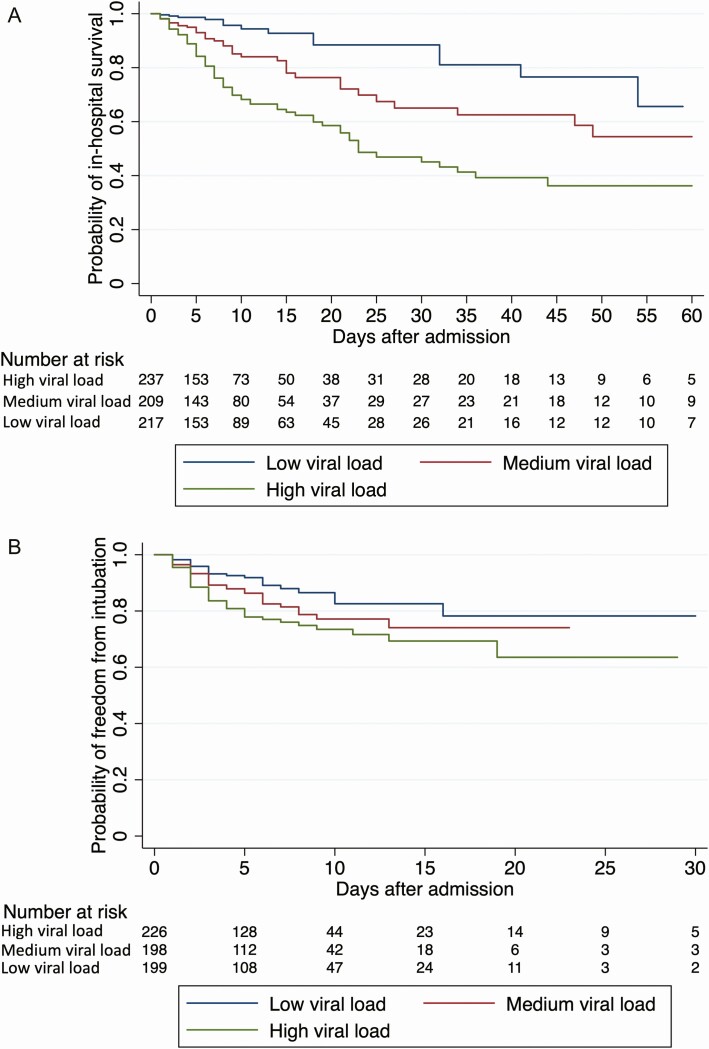
Probability of in-hospital survival (A) and freedom from intubation (B) during COVID-19 hospitalization among patients with high, medium, and low viral loads. A medium viral load was associated with a hazard ratio (HR) of in-hospital mortality of 2.52 (95% confidence interval [CI], 1.36–4.67; P = .003) and a HR of intubation of 1.53 (95% CI, .91–2.60; P = .11) compared with a low viral load. A high viral load was associated with a HR of in-hospital mortality of 5.06 (95% CI, 2.86–8.96; P < .001) and a HR of intubation of 2.15 (95% CI, 1.31–3.53; P = .003) compared with a low viral load.
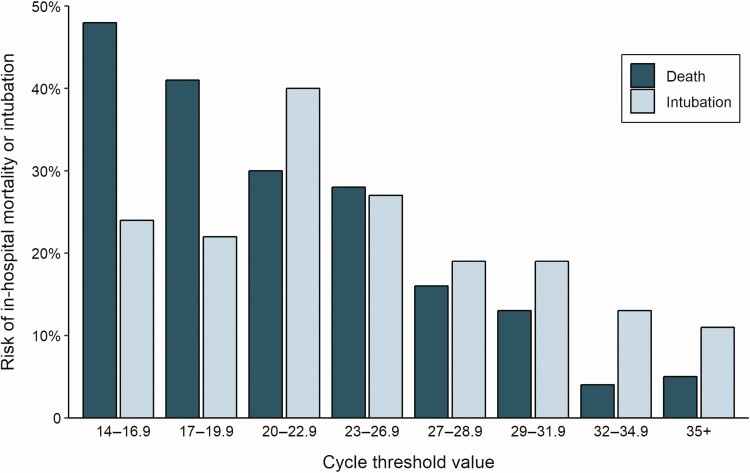
Cycle threshold values from nasopharyngeal swab samples on admission and risk of intubation and death during the hospitalization.
In a multivariate model that adjusted for age, race, coronary artery disease, congestive heart failure, cerebrovascular disease, hypertension, COPD, days of symptoms prior to admission, symptoms upon presentation, initial chest X-ray findings, and level of oxygen support within 3 hours of arrival to the ED (Table 2), having a high viral load was independently associated with increased risk of in-hospital mortality (adjusted odds ratio [aOR], 6.05; 95% CI, 2.92–12.52; P < .001) compared with having a low viral load. The risk of in-hospital mortality was also higher in patients with a medium viral load compared with a low viral load, but this association was not statistically significant (aOR, 2.06; 95% CI, .98–4.34; P = .058). Compared with those with a low viral load, having a high viral load was also independently associated with increased risk of intubation (aOR, 2.73; 95% CI, 1.68–4.44; P < .001), whereas the risk of intubation associated with a medium viral load did not reach statistical significance (aOR, 1.59; 95% CI, .96–2.63; P = .07). Patients with higher viral loads were also more likely to develop myocardial infarction, congestive heart failure, and acute kidney injury requiring hemodialysis (Table 1).
Table 2.
Multivariate Logistic Regression Models of Factors Associated With Intubation and In-hospital Mortality
Intubation | Mortality | |||
---|---|---|---|---|
Variable | Adjusted OR (95% CI) | P Value | Adjusted OR (95% CI) | P Value |
Age, per year increase | 1.10 (1.07–1.13) | <.001 | ||
White race | 1.00 (.58–1.72) | .99 | ||
Obesity | 1.41 (.93–2.12) | .10 | ||
Coronary artery disease | 1.48 (.79–2.78) | .22 | ||
Congestive heart failure | 2.10 (.89–4.93) | .09 | ||
Cerebrovascular disease | 1.24 (.54–2.86) | .61 | ||
Hypertension | 0.74 (.41–1.33) | .31 | ||
Chronic obstructive pulmonary disease | 0.65 (.23–1.82) | .42 | ||
Use of oral steroids as an outpatient | 1.86 (.84–4.12) | .13 | ||
Days of symptoms prior to admission, per day increase | 0.97 (.92–1.02) | .23 | ||
Symptoms on admission | ||||
![]() | 1.37 (.87–2.17) | .18 | 1.38 (.77–2.46) | .28 |
![]() | 0.64 (.36–1.13) | .12 | ||
![]() | 1.99 (1.20–3.30) | .008 | ||
![]() | 0.56 (.16–1.97) | .37 | ||
![]() | 1.24 (.60–2.58) | .56 | ||
![]() | 0.50 (.21–1.19) | .12 | ||
![]() | 1.29 (.65–2.55) | .46 | ||
![]() | 0.88 (.26–3.00) | .84 | ||
Highest level of supplemental oxygen within 3 hours of arrival to the emergency department a | ||||
![]() | Reference | |||
![]() | 3.79 (1.86–7.73) | <.001 | ||
![]() | 5.58 (2.50–12.46) | <.001 | ||
![]() | 23.34 (6.29–86.51) | <.001 | ||
Chest X-ray findings | ||||
![]() | Reference | Reference | ||
![]() | 1.72 (.30–9.84) | .54 | 4.36 (1.09–17.45) | .037 |
![]() | 9.94 (2.37–41.74) | .002 | 4.98 (1.47–16.94) | .01 |
Viral load by nasopharyngeal swab | ||||
![]() | Reference | Reference | ||
![]() | 1.59 (.96–2.63) | .07 | 2.06 (.98–4.34) | .058 |
![]() | 2.73 (1.68–4.44) | <.001 | 6.05 (2.92–12.52) | <.001 |
Only variables that had a significant association with intubation or mortality in a univariate logistic regression model were included in the corresponding multivariate model. Empty cells indicate that the variable was not associated with the corresponding outcome in the univariate logistic regression model. Variables with statistically significant associations are in bold.
Abbreviations: CI, confidence interval; OR, odds ratio.
aThis variable was analyzed as a risk factor for mortality but was not analyzed as a factor associated with intubation because 1 of the oxygen supplementation categories was mechanical ventilation.
DISCUSSION
In this study, we demonstrate that patients who were admitted to the hospital with high SARS-CoV-2 viral loads, as assessed by Ct values of NP swab samples, were more likely to be intubated or die during their hospitalization. This association persisted even when we adjusted for age, comorbidities, presenting symptoms, chest radiography findings, and degree of presenting hypoxia.
Prior studies have indicated that viral load correlates with severity of COVID-19 presentation [10, 11], and our study of a larger cohort of hospitalized patients adds to this knowledge base by identifying that admission viral load has important prognostic implications. Reporting SARS-CoV-2 viral load based on Ct values from admission NP swab samples could therefore help identify patients who are at highest risk of adverse outcomes and who therefore may benefit from more intensive monitoring. Identifying patients with high viral loads could also be helpful for allocating scarce therapeutic interventions such as antiviral agents (eg, remdesivir) [15]. Our findings also suggest that stratification or adjustment for baseline viral load would benefit the design of clinical trials of antiviral agents for COVID-19. It is also possible that viral load could be used along with other factors, such as age, comorbidities, and severity of symptoms and hypoxia, to decide on the need for hospital admission. However, additional studies that evaluate viral loads and clinical outcomes among all patients who present to the ED are warranted prior to pursuing this strategy clinically.
Older age and the presence of comorbidities such as hypertension, coronary artery disease, congestive heart failure, COPD, and cancer are known to be associated with worse outcomes in COVID-19 [2, 7, 16, 17]. These patients may have decreased cardiopulmonary reserve and thus are less likely to tolerate the physiologic insults caused by COVID-19. Our findings suggest that these patients also have higher SARS-CoV-2 viral loads when they present to the hospital, which may contribute to the worse outcomes observed in these patients. Reasons for higher viral loads specifically in these populations are not well understood and warrant further investigation.
Given that SARS-CoV-2 uses the angiotensin-converting enzyme 2 receptor (ACE2) for entry into host cells [18], there have been concerns that use of ACEIs and ARBs may upregulate ACE2 expression and lead to increased viral proliferation into host cells [19]. Although patients with hypertension and congestive heart failure were more likely to have higher viral loads, use of ACEIs and ARBs was not associated with higher viral load. Our findings are consistent with those from observational studies that have not demonstrated worse outcomes in patients who use ACEIs or ARBs [20–22] and support the recommendations of professional societies of not discontinuing these medications in the setting of COVID-19 [23].
Another notable finding from this study is that there were no differences in admission SARS-CoV-2 viral loads or outcomes among different racial or ethnic groups. In the United States, Hispanic and black communities have been disproportionately affected by COVID-19, with a greater proportion of deaths among these patients than what would be expected based on their population proportions [24–26]. Our finding that admission viral loads were not different among race and ethnicity groups suggests that these disparities are not related to viral load but instead may be related to comorbid illnesses and nonbiological factors such as social determinants of health. This further underscores the importance of studies that examine the impact of social determinants of health on outcomes during the COVID-19 pandemic.
We also found that patients with higher viral loads were more likely to develop myocardial infarction, congestive heart failure, and acute kidney injury. It is unclear whether these associations were from chance, were related to increased hypoxia in heart and kidney tissue, or were related to increased viral infection of these organs. A recent autopsy study demonstrated that SARS-CoV-2 frequently directly infects both the heart and kidney [27] and that kidney injury and myocardial injury are commonly reported complications of severe COVID-19 [28, 29]. Additional studies are warranted to assess the relationships between viral loads in NP swab samples, disease burden in the heart and kidney, and clinical outcomes.
Our study has limitations. We evaluated only the viral load of a single NP swab sample per patient at the time of hospital admission and thus could not assess viral load dynamics over time or the infectious burden at the time of infection onset. However, we found that this single sample on admission had important prognostic implications among hospitalized patients. In order to maintain consistency, we only analyzed Ct values from a single diagnostic platform, and thus our findings may not apply to all COVID-19 diagnostic assays. However, other studies have demonstrated that the Panther Fusion SARS-CoV-2 test (Hologic, Inc) and the Xpert Xpress SARS-CoV-2 test (Cepheid, Inc), 2 commonly used RT-PCR assays, yield nearly identical Ct values as the cobas 6800 assay used in this study [30, 31]. Thus, we suspect that our findings may also be applicable to other diagnostic platforms. We encourage others to evaluate the relationship between clinical outcomes and Ct values using other diagnostic platforms and other patient populations. Another potential role for reporting SARS-CoV-2 viral loads through Ct values is to guide the use of isolation precautions, given that viral load correlates with infectivity [32–34]. Our study did not assess this potential use of Ct values, but we believe this is an important area for future investigation. Another limitation is that our study was retrospective and relied on data that were documented in the electronic medical record and thus could have misclassified patient characteristics or outcomes. However, our data abstraction process used a standardized protocol and our queries identified high interrater reliability for data collection. Last, we focused on in-hospital mortality and did not capture deaths that occurred after discharge from the hospital.
In conclusion, we found that admission SARS-CoV-2 viral loads, as determined by Ct values that are generated with standard-of-care diagnostic assays, are independently associated with intubation and death among hospitalized patients with COVID-19. These findings highlight the critical role of viral load in SARS-CoV-2 pathogenesis and suggest that Ct values should be reported to assist clinicians in identifying patients at high risk for adverse COVID-19–related outcomes.
Supplementary Data
Supplementary materials are available at Clinical Infectious Diseases online. Consisting of data provided by the authors to benefit the reader, the posted materials are not copyedited and are the sole responsibility of the authors, so questions or comments should be addressed to the corresponding author.
ciaa851_suppl_Supplementary_Appendix
ciaa851_suppl_Supplementary_Figure
Notes
Acknowledgments. The authors acknowledge the work of our data abstraction team at Weill Cornell Medicine who are highlighted by name in our supplementary document. We also acknowledge Melissa Cushing, MD, Massimo Loda, MD, and the clinical laboratory scientists who performed the severe acute respiratory syndrome coronavirus 2 laboratory testing.
Financial support. This work was partially supported by the National Center for Advancing Translational Science (UL1 TR002384 to Julianne Imperato-McGinley) at the National Institutes of Health.
Potential conflicts of interest. L. F. W. reports receipt of consulting fees from Roche Molecular Systems, Inc and receipt of grants from BioFire Diagnostics, LLC outside the submitted work. M. S. S. reports receipt of consulting fees from Roche Diagnostics and receipt of grants from Amgen outside the submitted work. M. J. S. reports receipt of grants from BioFire Diagnostics outside the submitted work. All other authors report no potential conflicts. All authors have submitted the ICMJE Form for Disclosure of Potential Conflicts of Interest. Conflicts that the editors consider relevant to the content of the manuscript have been disclosed.
References
Articles from Clinical Infectious Diseases: An Official Publication of the Infectious Diseases Society of America are provided here courtesy of Oxford University Press
Full text links
Read article at publisher's site: https://doi.org/10.1093/cid/ciaa851
Read article for free, from open access legal sources, via Unpaywall:
https://europepmc.org/articles/pmc7337625?pdf=render
Citations & impact
Impact metrics
Article citations
SARS-CoV-2 viral load is linked to remdesivir efficacy in severe Covid-19 admitted to intensive care.
Sci Rep, 14(1):20825, 06 Sep 2024
Cited by: 0 articles | PMID: 39242658 | PMCID: PMC11379941
Uncovering the Contrasts and Connections in PASC: Viral Load and Cytokine Signatures in Acute COVID-19 versus Post-Acute Sequelae of SARS-CoV-2 (PASC).
Biomedicines, 12(9):1941, 23 Aug 2024
Cited by: 0 articles | PMID: 39335455 | PMCID: PMC11428903
Review Free full text in Europe PMC
Telemedicine for Patients with COVID-19: A Telehealth Experience in the Elderly at a Center in Southern Brazil.
Telemed Rep, 5(1):205-211, 18 Jul 2024
Cited by: 0 articles | PMID: 39081456
Microfluidic Immunoarray for Point-of-Care Detection of Cytokines in COVID-19 Patients.
ACS Omega, 9(27):29320-29330, 24 Jun 2024
Cited by: 0 articles | PMID: 39005811
The Impact of Viral Load on the Severity and Outcome Among Patients With COVID-19: A Cross-Sectional Study.
Cureus, 16(7):e64137, 09 Jul 2024
Cited by: 0 articles | PMID: 39119409 | PMCID: PMC11307488
Go to all (247) article citations
Data
Data behind the article
This data has been text mined from the article, or deposited into data resources.
BioStudies: supplemental material and supporting data
Similar Articles
To arrive at the top five similar articles we use a word-weighted algorithm to compare words from the Title and Abstract of each citation.
The Impact of SARS-CoV-2 Viral Load on the Mortality of Hospitalized Patients: A Retrospective Analysis.
Cureus, 13(7):e16540, 21 Jul 2021
Cited by: 0 articles | PMID: 34430148 | PMCID: PMC8378280
SARS-CoV-2 Viral Load Predicts Mortality in Patients with and without Cancer Who Are Hospitalized with COVID-19.
Cancer Cell, 38(5):661-671.e2, 15 Sep 2020
Cited by: 144 articles | PMID: 32997958 | PMCID: PMC7492074
SARS-CoV-2 infection: Initial viral load (iVL) predicts severity of illness/outcome, and declining trend of iVL in hospitalized patients corresponds with slowing of the pandemic.
PLoS One, 16(9):e0255981, 16 Sep 2021
Cited by: 28 articles | PMID: 34529675 | PMCID: PMC8445469
Clinical Characteristics and Morbidity Associated With Coronavirus Disease 2019 in a Series of Patients in Metropolitan Detroit.
JAMA Netw Open, 3(6):e2012270, 01 Jun 2020
Cited by: 390 articles | PMID: 32543702 | PMCID: PMC7298606
Review Free full text in Europe PMC
Funding
Funders who supported this work.
NCATS NIH HHS (1)
Grant ID: UL1 TR002384
NIH HHS
National Center for Advancing Translational Sciences (1)
Grant ID: UL1 TR002384