Abstract
Free full text

Risk and protective factors of SARS‐CoV‐2 infection
To the Editor,
It has been reported that coexistent chronic diseases are strongly associated with coronavirus disease‐2019 (COVID‐19) severity. 1 Investigations of predictors for severe acute respiratory syndrome coronavirus 2 (SARS‐CoV‐2) infection itself, however, have been seldom performed. Although metaregression has been traditionally utilized to investigate heterogeneity in meta‐analysis, 2 that considering a nation as a study in meta‐analysis may be of use to screen potential risk and protective factors for SARS‐CoV‐2 infection. To screen the factors, metaregression of data from nations worldwide were herein conducted.
I extracted (a) total confirmed COVID‐19 cases in 180 nations worldwide on 31 May 2020 from the World Health Organization (WHO, https://www.who.int/emergencies/diseases/novel-coronavirus-2019/situation-reports/); (b) national total population in 2018, life expectancy at birth in 2016, medical‐doctor and nursing/midwifery‐personnel density in 2010 to 2018, hypertension prevalence in 2015, obesity prevalence in 2016, annual particulate matter 2.5 (PM2.5) concentrations in urban areas in 2016, population using safely managed drinking‐water/sanitation services and hand‐washing facility with soap/water in 2017, and daily ambient ultraviolet radiation in 1997 to 2003 from the WHO (https://www.who.int/gho/publications/world_health_statistics/2020/en/; https://apps.who.int/gho/data/node.main.122?lang=en); (c) population ages 0 to 14 and ≥65 in 2018, Gross Domestic Product and Gross National Income per capita, Purchasing Ppower Parity in 2016 to 2018 (mostly 2018), and diabetes prevalence in 2019 from the World Bank (https://data.worldbank.org/indicator/); (d) inbound tourism in 2014 to 2018 from the United Nations World Tourism Organization (https://www.e-unwto.org/doi/abs/10.5555/unwtotfb0000270020142018202001); and (e) bachelor's or equivalent (International Standard Classification of Education 6) in 2016 to 2018 from the United Nations Educational, Scientific and Cultural Organization (http://uis.unesco.org/en/topic/educational-attainment), all of which are listed in Table S1. Restricted maximum‐likelihood metaregression in the random‐effects model was performed using Comprehensive Meta‐Analysis version 3 (Biostat, Englewood, NJ). To adjust for other covariates, we conducted the hierarchical multivariate models in addition to the univariate model.
Results of the metaregression are summarized in Table 1. A slope (coefficient) of the metaregression line for the COVID‐19 prevalence in the multivariable models was significantly negative for population ages 0 to 14 (–0.0636; P=
.0021; Figure 1A) and positive for obesity prevalence (0.0411; P
=
.0099; Figure 1B) and annual PM2.5 concentrations in urban areas (0.0158; P
=
.0454; Figure 1C), which would indicate that the COVID‐19 prevalence decreases significantly as the proportion of children increases and that the COVID‐19 prevalence increases significantly as the proportion of the obese and PM2.5 increases.
Table 1
Metaregression summary
Model, covariate | Coefficient | Lower limit of 95% CI | Upper limit of 95% CI | P | Figure | |
---|---|---|---|---|---|---|
Univariable model I | ||||||
Age distribution | ||||||
[1] Population ages 0‐14 (%) | –0.1136 | –0.1360 | –0.0913 | <.0001* | ||
[2] Population ages ≥65 (%) | 0.1521 | 0.1114 | 0.1928 | <.0001* | ||
Economy | ||||||
[3] GDP per capita, PPP (current international $) | 0.0001 | 0.0000 | 0.0001 | <.0001* | ||
[4] GNI per capita, PPP (current international $) | 0.0001 | 0.0001 | 0.0001 | <.0001* | ||
Multivariable model I including all the above‐mentioned covariates [1] to [4] | ||||||
[1] Population ages 0‐14 (%) | –0.0636 | –0.1038 | –0.0234 | .0021* | Figure 1A | |
[2] Population ages ≥65 (%) | –0.0163 | –0.0738 | 0.0411 | .5750 | ||
[3] GDP per capita, PPP (current international $) | 0.0000 | –0.0000 | 0.0001 | .8818 | ||
[4] GNI per capita, PPP (current international $) | 0.0000 | –0.0000 | 0.0001 | .1768 | ||
Multivariable model II‐1 adjusting for covariates [1] to [4] | ||||||
Public health | ||||||
[5] Life expectancy at birth (years) | 0.0300 | –0.0385 | 0.0984 | .3885 | ||
[6] Medical‐doctor density (/10![]() | 0.0292 | 0.0056 | 0.0529 | .0156* | ||
[7] Nursing/midwifery‐personnel density (/10![]() | 0.0047 | –0.0035 | 0.0130 | .2604 | ||
Multivariable model II‐2 adjusting for covariates [1] to [4] and [8] to [10] | ||||||
[5] Life expectancy at birth (years) | 0.0133 | –0.0654 | 0.0919 | .7396 | ||
[6] Medical‐doctor density (/10![]() | 0.0158 | –0.0085 | 0.0402 | .2015 | ||
[7] Nursing/midwifery‐personnel density (/10![]() | 0.0022 | –0.0058 | 0.0103 | .5844 | ||
Multivariable model III adjusting for covariates [1] to [7] | ||||||
Disease | ||||||
[8] Hypertension prevalence (%) | –0.0086 | –0.0790 | 0.0619 | .8107 | ||
[9] Obesity prevalence (%) | 0.0411 | 0.0100 | 0.0722 | .0099* | Figure 1B | |
[10] Diabetes prevalence (%) | –0.0261 | –0.0935 | 0.0413 | .4454 | ||
Multivariable model IV adjusting for covariates [1] to [10] | ||||||
Environment | ||||||
[11] Annual PM2.5 concentrations in urban areas, µg/m3 | 0.0158 | 0.0003 | 0.0313 | .0454* | Figure 1C | |
[12] Daily ambient ultraviolet radiation, J/m2 | 0.0001 | –0.0002 | 0.0003 | .6534 | ||
Multivariable model V adjusting for covariates [1] to [12] | ||||||
Health infrastructure | ||||||
[13] Population using safely managed drinking‐water services (%) | 0.0171 | –0.0044 | 0.0386 | .1180 | ||
[14] Population using safely managed sanitation services (%) | –0.0030 | –0.0222 | 0.0161 | .7521 | ||
[15] Population using hand‐washing facility with soap/water (%) | –0.0170 | –0.0406 | 0.0065 | .1525 | ||
Miscellaneous | ||||||
[16] Inbound tourism (millions) | 0.0027 | –0.0056 | 0.0110 | .5243 | ||
[17] Bachelor's or equivalent (ISCED 6) (%) | –0.0029 | –0.0485 | 0.0427 | .9004 |
Abbreviations: CI, confidence interval; GDP, gross domestic product; GNI, gross national income; ISCED, International Standard Classification of Education; PM2.5, particulate matter 2.5; PPP, purchasing power parity.
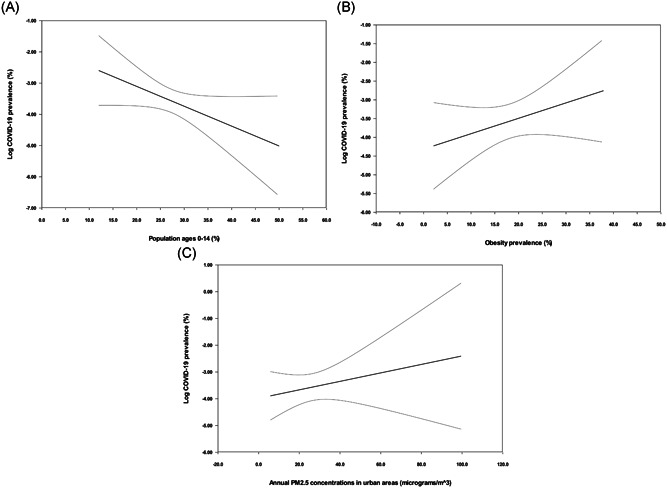
Metaregression lines with their 95% confidence interval curves depicting the coronavirus disease‐2019 prevalence (plotted as the logarithm‐transformed prevalence on the y‐axis) as a function of a given covariate (plotted on the x‐axis)
The present metaregression to screen potential risk and protective factors suggests that children may be negatively and independently, and obesity/PM2.5 may be positively and independently associated with SARS‐CoV‐2 infection. Our findings could be strengthened by low case fatality in children with COVID‐19 (only one death in a total of 1124 cases) 3 and obesity predicting poor prognosis of COVID‐19 4 demonstrated in recent systematic reviews. The present results, however, never denote directly that, for instance, the obese are at high risk for SARS‐CoV‐2 infection, which should be noted. Our findings demonstrate simply that the COVID‐19 prevalence is higher in the nation where the obese are more. To determine whether, for instance, the obese are at high risk for SARS‐CoV‐2 infection, two approaches are considered. First, potential risk and protective factors could be investigated in a cohort including both COVID‐19 patients and non‐COVID‐19 subjects (retrospective cohort study). It is never easy, however, to investigate the factors in the non‐COVID‐19 (healthy nonhospitalized) subjects. Second, the factors could be examined in both COVID‐19 patients and control subjects (case‐control study). In this occasion, however, the control subjects, who should be very comparable to the patients with COVID‐19, must be strictly selected. Metaregression by use of “weighted” data from multiple clinical trials differs from simple regression by means of individual‐patient data from a single study and accordingly can generate an equation of a “best‐fit” regression line to express the relation between an outcome and a covariate. 5 Metaregression applied in the present study may be alternative to the above‐mentioned approaches and could be of use at least to screen the factors.
In conclusion, children (negatively) and obesity/PM2.5 (positively) may be independently associated with SARS‐CoV‐2 infection.
REFERENCES
Full text links
Read article at publisher's site: https://doi.org/10.1002/jmv.26427
Read article for free, from open access legal sources, via Unpaywall:
https://onlinelibrary.wiley.com/doi/pdfdirect/10.1002/jmv.26427
Citations & impact
Impact metrics
Citations of article over time
Smart citations by scite.ai
Explore citation contexts and check if this article has been
supported or disputed.
https://scite.ai/reports/10.1002/jmv.26427
Article citations
A prospective cohort study of SARS-CoV-2 infection-induced seroconversion and disease incidence in German healthcare workers before and during the rollout of COVID-19 vaccines.
PLoS One, 19(1):e0294025, 30 Jan 2024
Cited by: 2 articles | PMID: 38289913 | PMCID: PMC10826949
Nirmatrelvir-remdesivir association for non-hospitalized adults with COVID-19, point of view.
Inflammopharmacology, 30(5):1927-1931, 18 Aug 2022
Cited by: 6 articles | PMID: 35980509 | PMCID: PMC9386652
COVID-19 mRNA Vaccines: A Retrospective Observational Pharmacovigilance Study.
Clin Drug Investig, 42(12):1065-1074, 23 Oct 2022
Cited by: 9 articles | PMID: 36274082 | PMCID: PMC9589581
COVID-19 mortality is associated with pre-existing impaired innate immunity in health conditions.
PeerJ, 10:e13227, 06 May 2022
Cited by: 3 articles | PMID: 35547187 | PMCID: PMC9083528
Assessment of SARS-CoV-2 Immunity in Convalescent Children and Adolescents.
Front Immunol, 12:797919, 17 Dec 2021
Cited by: 10 articles | PMID: 34975908 | PMCID: PMC8718543
Go to all (6) article citations
Data
Data behind the article
This data has been text mined from the article, or deposited into data resources.
BioStudies: supplemental material and supporting data
Similar Articles
To arrive at the top five similar articles we use a word-weighted algorithm to compare words from the Title and Abstract of each citation.
Tackling obesity during the COVID-19 pandemic.
Diabetes Metab Res Rev, 37(3):e3393, 29 Aug 2020
Cited by: 1 article | PMID: 32797666 | PMCID: PMC7435354
Risk factors for Covid-19 severity and fatality: a structured literature review.
Infection, 49(1):15-28, 28 Aug 2020
Cited by: 267 articles | PMID: 32860214 | PMCID: PMC7453858
Review Free full text in Europe PMC
Differentiating characteristics of patients with asthma in the severe acute respiratory syndrome coronavirus 2 infection.
Ann Allergy Asthma Immunol, 126(1):92-93, 07 Sep 2020
Cited by: 6 articles | PMID: 32911055 | PMCID: PMC7476503
HIV/SARS-CoV-2 coinfection: A global perspective.
J Med Virol, 93(2):726-732, 28 Jul 2020
Cited by: 51 articles | PMID: 32692406 | PMCID: PMC7404432
Review Free full text in Europe PMC