Abstract
Free full text

Heterogenous populations of tissue-resident CD8+ T cells are generated in response to infection and malignancy
Abstract
Tissue-resident memory CD8+ T cells (Trm) provide host protection through continuous surveillance of non-lymphoid tissues. Using single-cell RNA-sequencing (scRNA-seq) and genetic reporter mice, we identified discrete lineages of intestinal antigen-specific CD8+ T cells, including a Blimp1hiId3lo tissue-resident effector cell population, most prominent in the early phase of acute viral and bacterial infections, and a molecularly distinct Blimp1 loId3hi tissue-resident memory population that subsequently accumulated at later infection timepoints. These Trm populations exhibited distinct cytokine production, secondary memory potential, and transcriptional programs including differential roles for transcriptional regulators Blimp1, T-bet, Id2, and Id3 in supporting and maintaining intestinal Trm. Extending our analysis to malignant tissue, we also identified discrete populations of effector-like and memory-like CD8+ T cell populations with tissue-resident gene-expression signatures that shared features of terminally-exhausted and progenitor-exhausted T cells, respectively. Our findings provide insight into the development and functional heterogeneity of Trm cells, with implications for enhancing vaccination and immunotherapy approaches.
eTOC blurb:
Tissue-resident memory CD8+ T cells (Trm) provide long-lasting immunity in non-lymphoid tissues. Milner et al. use single-cell-RNA sequencing to reveal Trm cell heterogeneity in response to infection, and identify effector-like Id3loBlimp1hi and memory-like Id3hiBlimp1lo tissue-resident populations with differential effector function, memory potential, and transcriptional programming. Analogous populations of CD8+ T cells with tissue-residency features were also identified in tumors.
Introduction
Tissue-resident memory CD8+ T cells (Trm) have emerged as critical mediators of health and disease. Trm cells remain permanently lodged in non-lymphoid tissues (and in some cases lymphoid tissues) without recirculating and are transcriptionally, epigenetically, functionally, and anatomically distinct from recirculating populations of CD8+ T cells (Masopust and Soerens, 2019; Milner and Goldrath, 2018; Szabo et al., 2019). The widespread relevance of Trm cells in preventing and precipitating disease stems from their unique localization patterns, robust inflammatory sentinel activity, potent effector function, and longevity at sites of imminent or ongoing immune responses (Ho and Kupper, 2019; Masopust and Soerens, 2019). Therefore, understanding the molecular signals controlling the fate, function, and homeostasis of Trm cells is relevant in diverse pathophysiological settings ranging from infection to cancer.
Upon infiltration into non-lymphoid sites, dynamic environmental signals converge on several key Trm cell-fate-specifying transcription factors that modulate gene-expression programs controlling tissue-retention and egress. The transcription factors Blimp1, Hobit, and Runx3 repress a transcriptional signature associated with circulating memory T cells and facilitate induction of a tissue-residency gene-expression program (Mackay et al., 2016; Milner and Goldrath, 2018). Conversely, T-bet, Eomes, and KLF2 can impede Trm cell formation (Laidlaw et al., 2014; Mackay et al., 2015; Skon et al., 2013). Despite the key regulatory roles of these transcription factors in controlling tissue-residency, the ontogeny and accompanying heterogeneity of Trm cells remains unclear.
The circulating CD8+ T cell population is heterogeneous, not only evolving over time but also composed of numerous subsets within infection timepoints (Arsenio et al., 2014; Kakaradov et al., 2017). The effector phase of infection predominantly consists of terminally-differentiated, short-lived KLRG1hiCD127lo terminal effector (TE) cells and relatively fewer multipotent KLRG1loCD127hi memory-precursor (MP) cells (Chen et al., 2018). Select effector CD8+ T cells persist following infection at memory timepoints as long-lived effector cells (LLEC) (Olson et al., 2013; Omilusik et al., 2018), while others–predominately MP cells–continue to differentiate over time into long-lived, protective memory cells that can be broadly divided into central memory (Tcm) and effector memory (Tem) subsets. Tcm cells display enhanced lymphoid homing, multipotency, expansion potential, and longevity compared to Tem cells. Conversely, Tem cells are more terminally fated, shorter-lived, have limited expansion potential, and can elicit rapid effector function upon reinfection (Chen et al., 2018). Differentiation and maintenance of circulating memory subsets are also dynamically controlled by a compendium of transcription factors, including: Id2 (Cannarile et al., 2006; Knell et al., 2013; Masson et al., 2013), T-bet (Joshi et al., 2007), Blimp1 (Kallies et al., 2009; Rutishauser et al., 2009), Zeb2 (Dominguez et al., 2015; Omilusik et al., 2015), and STAT4 (Mollo et al., 2014) that are critical for Tem cells, and Id3 (Ji et al., 2011; Yang et al., 2011), Eomes (Banerjee et al., 2010; Pearce et al., 2003), Bcl6 (Ichii et al., 2002), Foxo1 (Hess Michelini et al., 2013; Rao et al., 2012), Tcf1 (Jeannet et al., 2010; Zhou et al., 2010), Zeb1 (Guan et al., 2018), Bach2 (Roychoudhuri et al., 2016), and STAT3 (Cui et al., 2011) that support Tcm cell differentiation. It remains unclear if the Trm cell population encompasses distinct cell subsets with differing functional attributes and memory potential, analogous to circulating CD8+ T cells.
Here we used single-cell RNA-sequencing (scRNA-seq) analysis to examine Trm cell ontogeny and heterogeneity. We found significant inter- and intra-temporal molecular diversity within the small intestine intraepithelial lymphocyte (siIEL) compartment that revealed discrete tissue-resident lineages. Despite being transcriptionally distinct, these siIEL CD8+ T cell subsets shared qualities with the circulating CD8+ T cell response, and could be phenotypically, transcriptionally, and functionally distinguished based on the reciprocal expression of the effector and memory-associated transcriptional regulators, Blimp1 and Id3. Distinct Trm cell-like subsets could also be distinguished within the tumor microenvironment. Our findings provide a framework to better understand the transcriptional signals controlling Trm cell differentiation and heterogeneity.
Results
Anti-viral siIEL CD8+ T cells are inter- and intra-temporally heterogeneous
It is apparent that Trm cells are transcriptionally distinct from circulating memory populations (Mackay et al., 2013; Milner et al., 2017; Wakim et al., 2012). To expand on these findings, we profiled the transcriptome of Tcm (CD62L+) and Tem (CD62L−) cells from the spleen as well as siIEL Trm cells isolated >50 days following acute LCMV infection. Consistent with previous reports, Trm cells were transcriptionally distinct from Tcm and Tem cells, which was illustrated by hierarchical clustering analysis (Figure 1A), and were enriched with a core tissue-residency gene-expression signature (Milner et al., 2017) (Figure 1B). However, we identified four gene expression modules (clusters 2-5) commonly regulated between Trm and Tcm cells or Trm and Tem cells. Furthermore, Trm cells were found to exhibit mixed expression of both Tcm and Tem cell fate-specifying transcription factors (Figure 1A). These data highlight that although Trm cells are a distinct memory T cell subset, they also share certain transcriptional features with the more effector-like Tem cells as well as the longer-lived Tcm cell population.
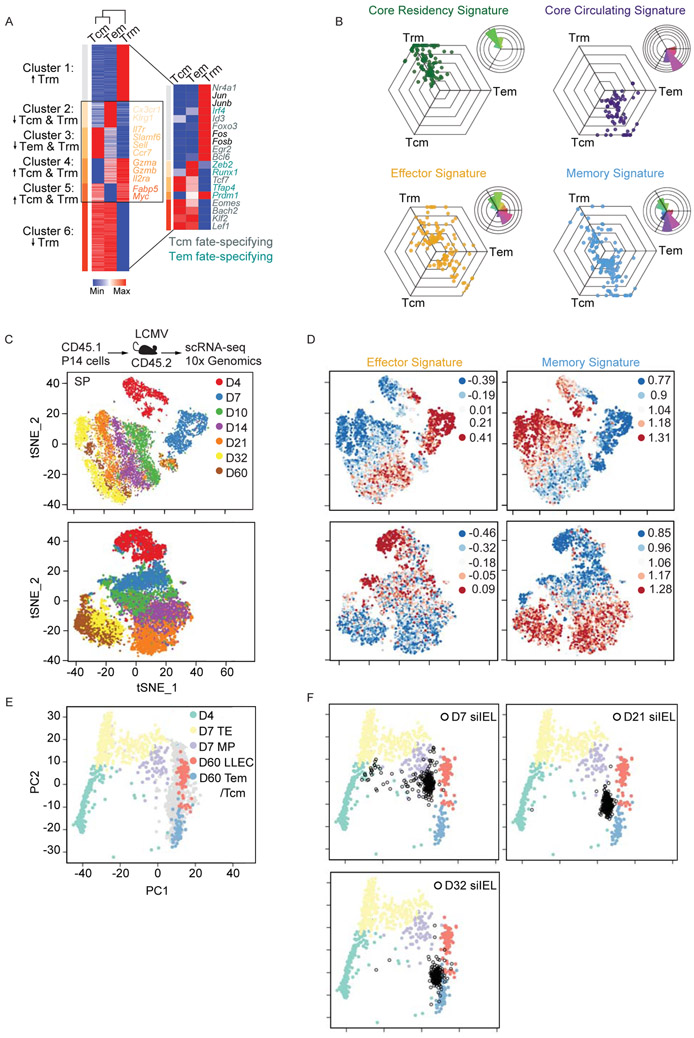
P14 CD8+ T cells were transferred into congenically distinct hosts that were subsequently infected with LCMV i.p. Donor cells from the spleen and siIEL were sorted over the course of infection for bullk RNA-seq or scRNA-seq. (A) Heatmap illustrating the relative expression of genes differentially expressed among Tcm, Tem, and Trm cell populations from bulk RNA-seq analysis; gene clusters are ordered through K-means clustering analysis. Transcriptional regulators reported as important for Tcm (gray) and Tem (teal) cell-fate are highlighted (right). (B) To visualize relative expression levels in a triwise comparison (van de Laar et al., 2016), filtered genes differentially expressed and present within the designated gene list (see Table S1,S2) were plotted in a hexagonal diagram in which distance of an individual data point represents gene expression enrichment or depletion, and the magnitude of upregulation is reflected by the distance from the origin. Rose plots (upper right corner of each hexagonal plot) indicate the percentages of genes in each orientation. Genes of the core residency (green), core circulating (purple), effector (yellow), and memory (blue) signatures are highlighted. (C-F) scRNA-seq analysis across an infection time course. tSNE plots of cells from the siIEL or spleen (SP) over all infection timepoints (C) colored by indicated timepoint or (D) shaded by intensity of effector or memory gene signatures. (E) Principal component analysis of splenic scRNA-seq samples based on expression patterns of signature effector and memory genes. Day 4 (green), day 7 TE (yellow) or MP (purple), and day 60 LLEC (pink) or Tem/Tcm cells (blue) are highlighted while samples from remaining timepoints are shaded grey. (F) The siIEL CD8+ T cell samples (black) are projected onto the 2D space according to the same principal components. See also Tables S1 and S2.
To further clarify the Trm cell population in terms of a long-lived memory or effector phenotype, we assessed expression of gene signatures in a triwise comparison of Tcm, Tem, and Trm cells (van de Laar et al., 2016). We found, as expected, that Tcm cells displayed enrichment of a long-lived memory CD8+ T cell gene-signature (Table S1,S2) relative to Tem cells (Figure 1B). However, mRNA levels of certain memory-associated genes were also increased in Trm cells relative to both Tem and Tcm cells. Conversely, Trm and Tem cells exhibited greater expression of effector-associated genes compared to Tcm cells, but Trm cells also displayed elevated expression of numerous effector-associated genes compared to Tem cells. Therefore, the non-mutually exclusive possibilities arise—does the Trm cell population exist in a unique state simultaneously balancing both effector and memory qualities, or is it comprised of distinct effector- and memory-like subsets undetectable via bulk sequencing analyses?
To investigate the apparent dual effector-memory state of the Trm cell population, we utilized scRNA-seq profiling of LCMV GP33-41-specific P14 CD8+ T cells isolated from the spleen or siIEL compartment over the course of an LCMV infection (Figure 1C). Data from multiple infection timepoints from the spleen or siIEL were integrated into an unsupervised t-distributed stochastic neighborhood embedding (tSNE) analysis (Figure 1C). Similar to circulating CD8+ T cells from the spleen, siIEL CD8+ T cells were enriched for an effector gene-expression signature early after infection, and subsequently, were enriched for a memory gene-expression signature at later infection timepoints (Figure 1D). Thus, the siIEL CD8+ T cells exhibited substantial inter-temporal heterogeneity. We also detected intra-temporal heterogeneity within the siIEL CD8+ T cell population at both early and late infection timepoints. Select siIEL CD8+ T cells enriched with a memory gene-expression signature were observed as early as day 4 following infection, and those with an enrichment of the effector gene-expression signature were evident on days 32 and 60 of infection. Furthermore, few CD8+ T cells appear to simultaneously display enrichment of both effector and memory gene-expression signatures (Figure 1D). To orient the transcriptional profile of siIEL CD8+ T cells to that of the splenic populations, principal component analysis was performed on all splenic scRNA-seq samples according to the expression of the differentially expressed genes between effector and memory T cell states. All splenic cells were plotted according to the top two principal components and day 4 effector T cells, day 7 TE and MP, day 60 LLEC, and Tem /Tcm cells were highlighted with the indicated color while samples from remaining timepoints are shaded grey (Figure 1E). Here, the bulk CD62L− population was subdivided into CD127− and CD127+ cells, which resolved LLEC and Tem cells, allowing a more refined delineation of effector and memory gene-expression signatures. Next, siIEL CD8+ T cells from the indicated time points of infection were projected into the 2D space according to the same principal components (Figure 1F; black). At day 7 of infection, siIEL CD8+ T cells clustered near the circulating effector T cell subsets (primarily MP) and LLEC. However, by day 21 of infection, the siIEL CD8+ T cells were interposed between LLEC and Tem /Tcm cells. Therefore, Figure 1F further suggests that siIEL cells exist in a range of effector and memory states analogous to circulating CD8+ T cells. In summary, we found that siIEL CD8+ T cells exhibit both inter- and intra-temporal heterogeneity, and the current broad definition of Trm cell is not necessarily reflective of this apparent diversity as effector-like siIEL CD8+ T cells also persisted into the memory phase of infection.
Key regulatory molecules distinguish effector and memory subsets of siIEL CD8+ T cells
As we noted distinct sub-populations of Trm cells, somewhat analogous to circulating CD8+ T cell subsets, we next evaluated how the current paradigm of circulating CD8+ T cell diversity parallels Trm cell heterogeneity. We first assessed the inter-temporal expression dynamics of molecules often utilized to clarify heterogeneity within the circulating CD8+ T cell compartment (Figure S1A); as anticipated, circulating CD8+ T cells exhibited an inverse expression pattern of Klrg1 and Il7r (encoding CD127, Figure 2A). Despite time-dependent changes in effector and memory gene signatures within the siIEL CD8+ T cell populations (Figure 1D), Il7r and Klrg1 expression kinetics were not as dynamic as in splenic CD8+ T cells (Figure 2A). Nonetheless, Klrg1 expression was elevated early (days 4-10) in the intestine and diminished over time, whereas Il7r expression steadily increased. This gene-expression pattern was similarly reflected at the protein level (Figure 2B). The expression of pro-effector transcriptional regulators Tbx21 (encoding T-bet), Prdm1 (encoding Blimp1), Zeb2, and Id2 as well as pro-memory factors Bcl6, Eomes, Tcf7 (encoding TCF1), and Id3 were assessed across all siIEL samples (Figure 2C). Consistent with Figure 1A, the expression pattern of pro-memory or pro-effector transcriptional regulators did not conform to an expected expression pattern (i.e. increased expression of pro-effector and pro-memory transcriptional regulators at early and later infection timepoints, respectively). Within siIEL cells, Prdm1 and Eomes expression peaked early whereas Id3 and Bcl6 expression increased over time (Figure 2C, ,D).D). Intracellular staining for T-bet, Eomes, and TCF1 in siIEL CD8+ T cells following LCMV infection was reflective of the scRNA-seq results (Figure 2E). Therefore, differential KLRG1 and CD127 expression suggests siIEL CD8+ T cell heterogeneity somewhat analogous to circulating cells, and the expression pattern of select transcription factors may be indicative of effector and memory siIEL CD8+ cell states.
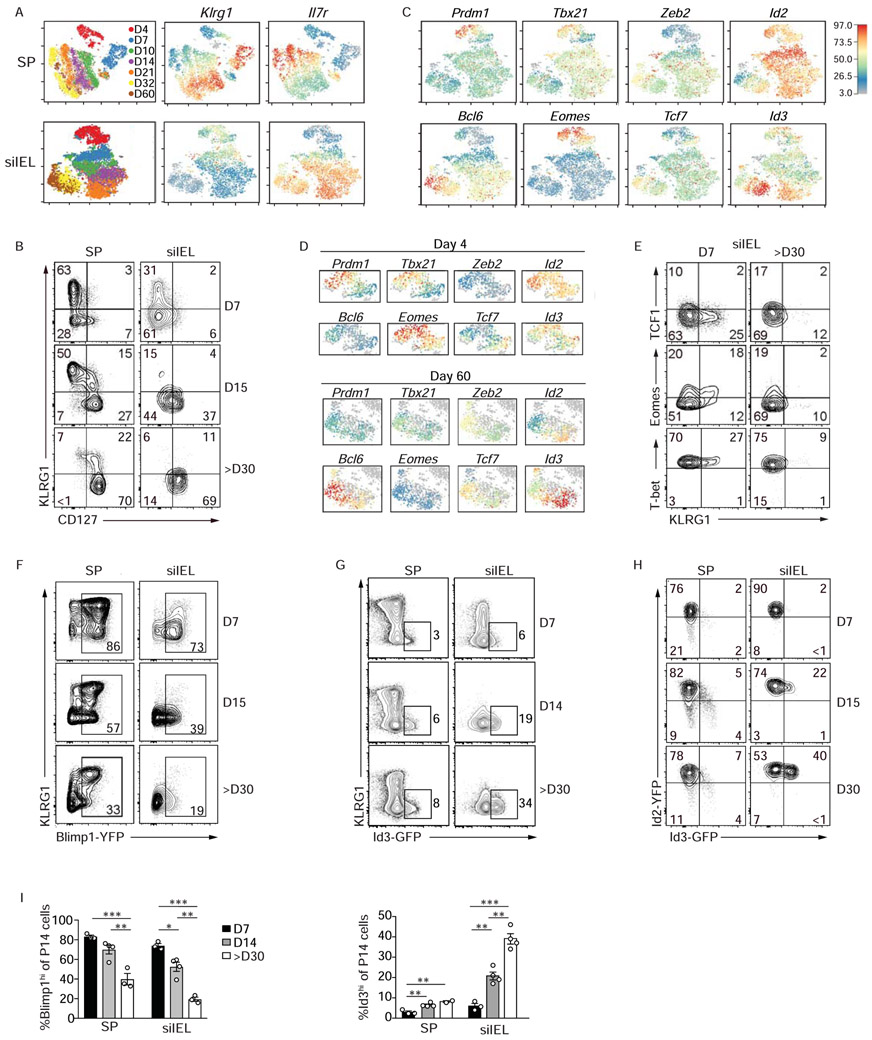
P14 CD8+ T cells were transferred into congenically distinct hosts that were subsequently infected with LCMV i.p. Donor cells from the spleen and siIEL were sorted over the course of infection for scRNA-seq or were analyzed through flow cytometry. (A) tSNE plots of cells from the spleen (top) or siIEL (bottom) over all infection timepoints colored by sample (left) and intensity of Klrg1 (middle) or Il7r (right) mRNA levels. (B) Expression levels of CD127 and KLRG1 during LCMV infection. (C) Relative gene-expression of highlighted transcriptional regulators in siIEL cells following the map from A and in the spleen (Figure S1A). (D) Expression of indicated transcriptional regulators within siIEL CD8+ T cells at day 4 (top) or 60 (bottom) of infection. (E) Expression levels of indicated transcriptional regulators. (F-H) Blimp1-YFP, Id3-GFP or Id2-YFP/Id3-GFP P14 CD8+ T cells were transferred into congenically distinct hosts that were infected with LCMV i.p. At indicated times of infection, reporter expression was analyzed in the spleen and siIEL by flow cytometry (and in Figure S1B). (I) The percentage of Blimp1-YFPhi and Id3-GFPhi P14 cells in the spleen and siIEL are quantified. Numbers in plots represent the frequency of cells in the indicated gate. All data are from one representative experiment of 2 independent experiments with n=3-5 (B,E,H) or n=2-4 (F,G). Graphs show mean ± SEM; *p<0.05, **p<0.01, ***p<0.001. See also Figure S1.
We noted intra-temporal siIEL CD8+ T cell heterogeneity at both early effector and later memory phases of infection (Figure 2D). For example, siIEL CD8+ T cells with varying expression of Prdm1, Tbx21, and Eomes were noted within early infection timepoints (e.g. day 4), whereas disparate expression of Zeb2, Id2, Bcl6, and Id3 was observed among cells at later (e.g. day 60) timepoints of infection (Figure 2D). Consistent with the scRNAseq findings, we detected a range of transcription factor expression levels at memory timepoints (Figure 2C--E).E). However, two transcriptional regulators with distinct expression patterns over the course of infection were Blimp1 (Prdm1), a transcriptional repressor, and Id3, an inhibitor of E protein transcription factors. Prdm1 expression was highest in siIEL CD8+ T cells at day 4 of infection and subsequently declined over time (Figure 2C), consistent with the Prdm1 expression patterns reported for skin Trm cells (Mackay et al., 2016). To understand if these distinct expression profiles were reflective of unique subpopulations of siIEL cells, we next utilized Blimp1-YFP-expressing P14 CD8+ T cells to assess Blimp1 expression in siIEL cells over the course of LCMV infection. Consistent with the scRNA-seq profiling, Blimp1 expression was highest early following infection corresponding with KLRG1 expression but decreased over time (Figure 2F). Within the circulation, Id3 expression marks CD8+ T cells with greater memory potential (Ji et al., 2011; Yang et al., 2011), but its expression patterns and function in tissue-resident populations has yet to be clarified. We next used Id3-GFP-reporter (Miyazaki et al., 2011) P14 CD8+ T cells to evaluate Id3 expression in siIEL CD8+ T cells over the course of LCMV infection. Similar to that observed in circulating CD8+ T cells (Ji et al., 2011) and consistent with our scRNA-seq data, Id3 expression inversely correlated with that of Blimp1 and the frequency of KLRG1loId3hi cells increased over time wherein nearly one-third of all Trm cell within the siIEL compartment at day ~30 of infection expressed Id3 (Figure 2G). Notably, the dramatically increased frequency of Id3-expressing cells was not observed in the circulating memory population, which peaked at ~8%. Id2, also a key inhibitor of E protein activity, has a recognized role in promoting survival and terminal differentiation of circulating CD8+ T cells (Cannarile et al., 2006; Knell et al., 2013; Masson et al., 2013; Omilusik et al., 2018). Therefore, we utilized Id2-YFP-expressing P14 CD8+ T cells (Yang et al., 2011), and found that Id2 expression was slightly increased in siIEL CD8+ T cells compared to splenic CD8+ T cells, but as observed in splenic populations (Yang et al., 2011), expression levels remained relatively constant over time (Figure 2H and Figure S1B). Taken together, the frequency of siIEL CD8+ T cells at effector timepoints expressing Blimp1 and KLRG1 was greater than at memory timepoints, whereas Id3hi cells were reciprocally increased in the memory phase of infection (Figure 2I).
Blimp1 and Id3 delineate distinct populations of siIEL CD8+ T cells
To examine the relationship between Blimp1 and Id3 expression in the context of the observed siIEL CD8+ T cell heterogeneity, we generated P14 Blimp1-YFP/Id3-GFP-reporter mice. In the spleen and mesenteric lymph node, Id3 expression remained relatively unchanged past day 14 of infection; however, the frequency of Id3-expressing siIEL CD8+ T cells increased over time, with a ~10-fold increase in the percentage of Id3hi siIEL CD8+ T cells from day 7 to day ~80, and a corresponding ~50-fold loss in the absolute number of Blimp1-YFP siIEL CD8+ T cells (Figure 3A,,B).B). Notably, we found that CD8+ T cell populations were transcriptionally varied across non-lymphoid sites, wherein only siIEL and lamina propria compartments had a sizeable Id3hi population of CD8+ T cells at day 90 of LCMV infection (Figure 3C). Kidney, salivary gland, and lungs contained a low frequency of Id3hi CD8+ T cells while white adipose tissue and brain populations had no detectable Id3 (Figure 3C,,D).D). However, epidermal Trm cells expressed robust levels of Id3 in a skin inflammation model (Figure 3E). Thus, while Blimp1 is expressed in a subset of CD8+ T cells across multiple non-lymphoid sites, Id3 expression may be restricted to CD8+ T cells within certain barrier tissues. We also confirmed similar Blimp1 and Id3 expression patterns in siIEL CD8+ T cells following enteric Listeria monocytogenes infection (Figure 3F).
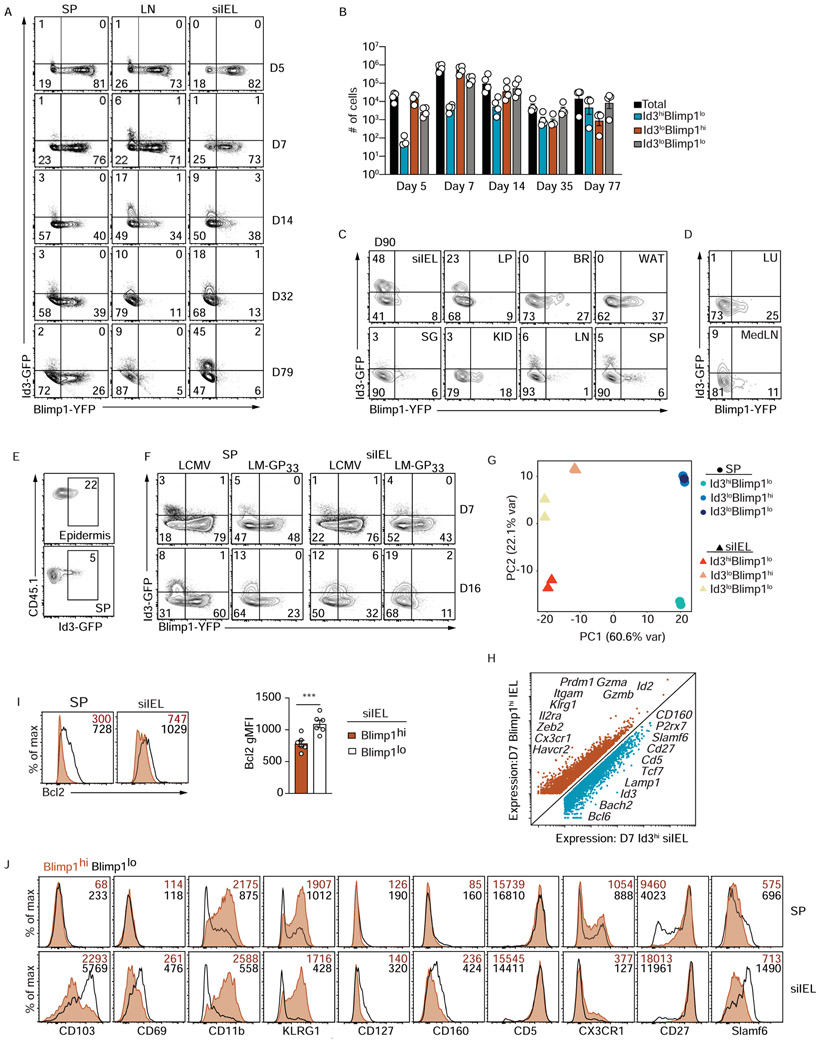
Congenically distinct Id3-GFP or Blimp1-YFP/Id3-GFP (double reporter) P14 CD8+ T cells were transferred to wild type hosts that were subsequently infected with LCMV or LM-GP33 o.g. (A) Blimp1-YFP and Id3-GFP reporter expression was assessed by flow cytometry in double reporter P14 cells from indicated host tissue over the course of the LCMV i.p. infection. (B) Quantification of the number of cells in the indicated populations from A. (C-D) Blimp1-YFP and Id3-GFP reporter expression in double reporter P14 cells from indicated host tissues on day 90 of LCMV i.p. infection (C) or from lung and draining LN (MedLN) on day 35 of an aspirated LCMV infection (D) is shown. (E) Id3-GFP P14 CD8+ T cells were adoptively transferred into recipient mice that were treated with DNFB on the left flank on day 4 of LCMV i.p. infection. The frequency of transferred P14 CD8+ T cells expressing Id3-GFP expression in the epidermis (top) and spleen (bottom) on day >30 following infection is indicated. (F) Blimp1-YFP and Id3-GFP reporter expression in double reporter P14 CD8+ T cells from host spleen and siIEL on days 7 and 16 following LCMV and LM-GP33 infection is compared. (G-H) On day 7 of infection, Id3hiBlimp1lo, Id3loBlimp1hi, and Id3loBlimp1lo P14 CD8+ T cells from the spleen and siIEL were sorted for RNA-sequencing. Principal component analysis (G) of gene expression from the sorted P14 CD8+ T cell populations is shown, or differentially expressed genes (H) between Id3hiBlimp1lo and Id3loBlimp1hi siIEL P14 CD8+ T cells are highlighted. (I-J) Blimp-YFP or Blimp1-YFP/Id3-GFP P14 CD8+ T cells transferred into congenically distinct hosts that were infected with LCMV i.p. were isolated from spleen and siIEL and analyzed on day 7 of infection for expression of Bcl2 (I) or indicated surface molecules (J) and Figure S1C-E. siIEL = small intestine intraepithelial lymphocytes; LP = small intestine lamina propria; BR= brain; WAT= white adipose tissue; SG = salivary gland; KID = kidney; LN = mesenteric lymph node; SP = spleen, LU =lung, MedLN = mediastinal lymph node. Numbers in plots represent the frequency (A,C,D,E,F) or gMFI (I,J) of cells in the indicated gate. All data are from one representative experiment of 2-3 independent experiments with n=3-5 (A-H,J) or cumulative from 2 independent experiments with a total n=6 (I). See also Figure S1.
Although the frequency of Id3-expressing cells continuously increased over time, we observed a small proportion of siIEL CD8+ T cells that expressed Id3 at the peak of LCMV infection (Figure 3A), suggesting that subset heterogeneity may be established early after infection. Principal component analysis of gene expression by Id3loBlimphi, Id3hiBlimp1lo, and Id3loBlimp1lo siIEL and splenic CD8+ T cells on day 7 of LCMV infection revealed that effector CD8+ T cells clustered based on tissue origin, but also that variation between the three siIEL populations was evident even at early effector timepoints (Figure 3G). Comparison of gene expression between the Id3loBlimphi and Id3hiBlimp1lo siIEL CD8+ T cell subsets highlighted that considerable transcriptional differences already existed by day 7 of infection, with Blimp1hi cells expressing canonical effector molecules such as Cx3cr1, Zeb2, Klrg1, Id2, Gzma, and Gzmb, whereas Id3hi cells expressed canonical memory genes including Bcl6, Bach2, Tcf7, and Cd27 (Figure 3H), suggesting this population included the long-lived Trm cell precursors. This early subset variation was further confirmed by correlating levels of key molecules with Blimp1-YFP expression by siIEL CD8+ T cells at day 7 of infection (Figure 3I,,JJ and Figure S1C-E). Thus, as early as day 7 of infection, siIEL CD8+ T cells were transcriptionally distinct from circulating effector populations and exhibited considerable subset heterogeneity, reminiscent of circulating TE and MP populations.
Id3 and Blimp1 distinguish functionally distinct memory siIEL subsets
To determine if the expression pattern of Blimp1 and Id3 observed within the siIEL CD8+ T cell population reflects molecularly distinct memory T cell populations, we next profiled the transcriptome of the Id3- and Blimp1-expressing siIEL CD8+ T cell subpopulations on day 35 of infection. As expected, principal component analysis revealed that, despite differential expression of Blimp1 and Id3, all three populations sorted from the siIEL compartment were transcriptionally similar relative to the corresponding circulating memory CD8+ T cells within the spleen and lymph node (Figure 4A). However, subtle differences between the siIEL populations were detectable in this context (Figure 4A), and closer examination revealed >2000 differentially expressed transcripts between Blimp1hiId3lo and Blimp1loId3hi siIEL CD8+ T cells (Figure 4B, Figure S1F), including increased expression of effector-associated genes (Klrg1, Zeb2, Bhlhe40 and Gzma, Gzmb) in the Blimp1hiId3lo subset and memory-associated genes (Tcf7, Eomes, Bach2) in the Blimp1loId3hi population. Further, we found that the Blimp1loId3hi siIEL gene-expression signature was enriched in cells from later memory timepoints (i.e. D21-D60) within the scRNAseq dataset (Figure S1G). Flow cytometry analysis of siIEL CD8+ T cells on days 22-25 of infection confirmed many of the findings from the RNA-seq analysis (Figure 4C, Figure S1H). Further, Blimp1 loId3lo cells exhibited an intermediate transcriptional profile between Id3hi and Blimp1hi siIEL cells (Figure 4A, S1F). Thus, while the siIEL Trm cell population as a whole was transcriptionally distinct from the circulating memory CD8+ T cell populations, Blimp1 and Id3 delineated distinct siIEL subsets.
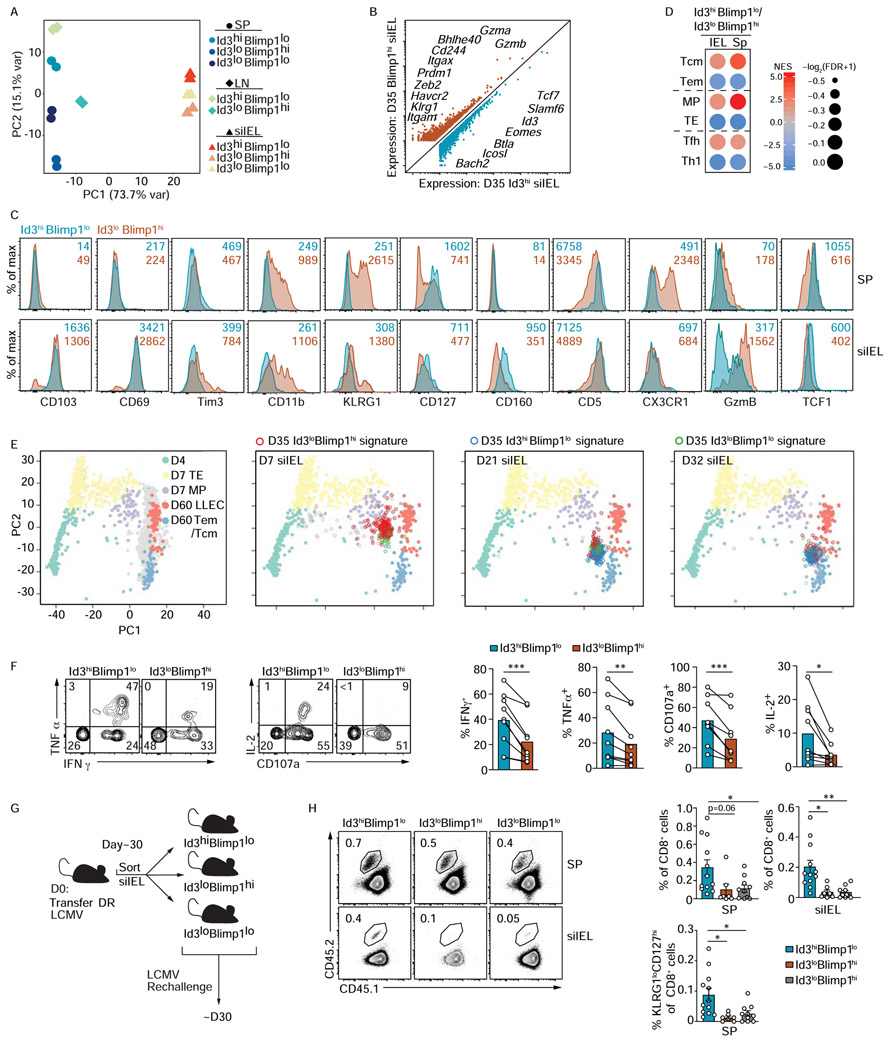
(A-D) Blimp1-YFP/Id3-GFP P14 CD8+ T cells were transferred into congenically distinct hosts that were subsequently infected with LCMV i.p. On day 35 of infection, Id3hiBlimp1lo, Id3loBlimp1hi, and Id3loBlimp1lo P14 CD8+ T cells from the spleen (SP), mesenteric lymph node (LN), and siIEL were sorted for RNA-sequencing. (A) Principal component analysis of gene expression from the sorted P14 CD8+ T cell populations is shown. (B) Genes differentially expressed by Id3hiBlimp1lo and Id3loBlimp1hi P14 siIEL CD8+ T cells are highlighted. (C) Blimp1-YFP/Id3-GFP P14 CD8+ T cells transferred into congenically distinct hosts subsequently infected with LCMV i.p. were analyzed in spleen and siIEL on day 25-27 of infection. Expression of indicated molecules were compared between Id3hiBlimp1lo (teal) and Id3loBlimp1hi (orange) subsets. Numbers in plots represent gMFI. (D) GSEA for specified gene-expression signatures enriched in Id3hiBlimp1lo and Id3loBlimp1hi siIEL CD8+ T cell subsets. (E) Principal component analysis (from scRNA-seq dataset) of the spleen samples based on expression of genes in effector and memory gene-expression signatures. Day 4 (green), day 7 TE (yellow) or MP (purple), and day 60 LLE (pink) or Tem cells/Tcm cells (blue) are highlighted. The siIEL CD8+ T cells samples are projected to the 2D space according to the same principal components and enrichment for Id3hiBlimp1lo (blue), Id3loBlimp1hi (red), and Id3loBlimp1lo (green) gene signatures is indicated on siIEL populations. (F) After 30 days of LCMV i.p. infection, Blimp1-YFP/Id3-GFP P14 siIEL CD8+ T cells were restimulated in vitro with GP33-41 peptide then the Id3hiBlimp1lo and Id3loBlimp1hi populations were analyzed by flow cytometry for the surface expression of CD107a and the production of cytokine. Representative plots (left) and quantification (right) of indicated populations are shown. Numbers in plots represent the frequency of cells in the indicated gate. (G) Schematic of experimental set-up. Congenically distinct Blimp1-YFP/Id3-GFP P14 CD8+ T cells were transferred to wild-type hosts that were infected with LCMV i.p. More than 30 days after infection, Id3hiBlimp1lo, Id3loBlimp1hi, and Id3loBlimp1lo P14 CD8+ T cells were sorted from the siIEL, and then retransferred intravenously into congenically distinct hosts subsequently infected with LCMV i.p. After 30 days of infection, donor cells in the host spleen (SP) and siIEL were analyzed by flow cytometry. (H) Frequency of transferred cells among CD8+ T cells is shown. Numbers in plots represent the frequency of cells in the indicated gate (left). Quantification of indicated populations (right). All data are from one representative experiment of 2 independent experiments with n=3-4 (C) or 3-4 independent experiments with a total n=3-12 (F,H). Graphs are cumulative of all experimental repeats and show mean ± SEM; *p<0.05, **p<0.01. See also Figure S1.
To place these Trm cell subsets in the context of other conventional T cell populations, we performed gene set enrichment analysis (GSEA). Blimp1loId3hi siIEL CD8+ T cells exhibited enrichment of gene-signatures from Tcm, MP, and CD4+ Tfh cells (i.e. cells that exhibit memory-like qualities). In contrast, the Blimp1hiId3lo siIEL population displayed enrichment with Tem, TE, and CD4+ Th1 cell gene-signatures (i.e. cells that are generally more terminally differentiated and effector-like) (Chen et al., 2018; Nguyen et al., 2019) (Figure 4D). Further, to orient the transcriptional profile of the distinct Id3 and Blimp1 expressing siIEL CD8+ T populations to that of canonical circulating T cell populations, principal component analysis was performed using all splenic scRNA-seq samples according to the expression of the differentially expressed genes between effector and memory T cell states (as in Figure 1E). Next, siIEL CD8+ T cells from the indicated time points of infection were projected into the 2D space according to the same principal components (as in Figure 1F). Then, the siIEL samples enriched for the gene-expression signatures of the distinct Id3- and Blimp1-expressing subsets (generated from day 35 bulk RNA-seq data) were highlighted as indicated within the PCA plots (Figure 4E). As expected, siIEL CD8+ T cells on day 7 of infection were enriched for the Id3loBlimp1hi signature (red) while the population at day 32 of infection was enriched with the Id3hiBlimp1lo gene-expression signature (blue). Notably, the day 21 siIEL CD8+ T cell population was heterogeneous, revealing siIEL cells with a Blimp1hi gene-expression signature exhibit an effector gene program similar to circulating effectors and LLEC, whereas siIEL cells enriched with an Id3hi signature display a memory gene-program similar to circulating memory cells.
We next assessed if differential Blimp1 and Id3 expression delineated siIEL CD8+ T cell subsets with discrete functional qualities. Blimp1loId3hi siIEL CD8+ T cells displayed elevated degranulation capacity and polyfunctionality, as evidenced by greater CD107a surface staining as well as increased IFNγ, TNFα, and IL-2 production upon cognate peptide restimulation (Figure 4F). This finding is consistent with the enhanced cytokine production observed in CD127hi siIEL CD8+ T cells relative to the CD127lo population (Kurd et al., 2020) and is a general feature of multipotent CD8+ T cells (Im et al., 2016; Joshi et al., 2007; Miller et al., 2019; Wherry et al., 2003). To examine subset-specific differences in memory potential, Blimp1hiId3lo, Blimp1loId3hi, and Blimp1loId3lo siIEL CD8+ T cell populations were sorted and transferred into recipient mice that were challenged with LCMV (Figure 4G). Notably, we found that Blimp1loId3hi siIEL donor cells yielded a greater frequency of both circulating and resident populations following rechallenge compared to the Id3lo subsets (Figure 4H). Furthermore, the progeny of Id3hiBlimp1lo siIEL cells following rechallenge generated more KLRG1loCD127hi P14 CD8+ T cells than Id3loBlimp1hi and Id3loBlimp1lo donor siIEL cells (Figure 4H). Thus, Id3hi siIEL CD8+ T cells possessed enhanced recall proliferative capacity and multipotency as they formed a larger pool of memory cells and were capable of giving rise to both circulating memory and Trm cell populations, indicating that Id3 expression marks Trm cells with heightened secondary memory potential.
Transcriptional regulation of siIEL CD8+ T cell heterogeneity
We next assessed the role of key transcriptional regulators, associated with promoting TE versus MP fates, in driving the heterogeneity of siIEL CD8+ T cells. Prdm1fl/fl-Gzmb-Cre+ or Id2f/f-CD4-Cre+ P14 CD8+ T cells were mixed 1:1 with congenically distinct wild type (WT) P14 CD8+ T cells and transferred to naive recipient mice subsequently infected with LCMV (Figure S2A,B,D,E). We previously noted that KLRG1 followed a relatively similar expression trajectory as Blimp1 and that Blimp1hi cells expressed elevated levels of KLRG1 (Figure 2F). Therefore, we utilized KLRG1 to signify Blimp1hi siIEL CD8+ T cells in order to understand the roles of Blimp1 and Id2 in regulating the fate of distinct siIEL CD8+ T cell subsets in the absence of the Blimp1-YFP and Id3-GFP reporters. On day 7 or 8 of infection, Blimp1 was generally required for optimal formation of bulk siIEL CD8+ T cells as previously reported (Mackay et al., 2016), but notably, the KLRG1hi effector subset was more dramatically impacted by loss of Blimp1 (Figure S2D,E). Further, Id2-deficiency resulted in a selective loss of the KLRG1hi siIEL CD8+ T cells, but interestingly the formation of the KLRG1lo subset was not impacted (Figure S2D,E). We also examined if T-bet regulated the KLRG1hiBlimp1hi siIEL CD8+ T subset by comparing siIEL Tbx21+/+ and Tbx21+/− P14 CD8+ T cells in a similar mixed transfer experiment (Figure S2C-E). We found that T-bet also supported the formation of KLRG1hi siIEL CD8+ T cells but was not essential for the KLRG1lo siIEL precursor population in the siIEL compartment. Further, Id2-deficiency or Tbx21-heterozygosity resulted in elevated expression levels of CD103, CD69, Slamf6, CD27, and CD127 as well as lower expression levels of KLRG1 and CX3CR1 (Figure S2F). Taken together, the effector-associated transcriptional regulators Blimp1, Id2 and T-bet direct formation of the KLRG1hi/Blimp1hi siEL CD8+ T cell subset.
Given the unique expression pattern of Id3 and its role in supporting long-lived circulating memory cells, we examined Id3-mediated regulation of siIEL maintenance by inducing deletion in established Trm cells. Id3f/f -ERCre+ P14 CD8+ T cells were mixed 1:1 with Id3+/+ P14 CD8+ T cells and transferred to congenically distinct naive mice that were subsequently infected with LCMV. Induced deletion of Id3 resulted in a minor loss of siIEL, and both CD127hi and CD127lo populations were equivalently impacted (Figure 5A,,B).B). We speculated the minimal phenotype observed by induced deletion of Id3 was due to compensation by Id2, as we find Id2 is abundantly expressed in both CD127hi and CD127lo siIEL (Figure 2H). Utilizing a similar induced deletion system, we first tested the impact of Id2 deletion alone in maintaining the distinct siIEL populations (Figure 5C). Here, we found that induced deletion of Id2 in established Trm cells dramatically impacted the CD127hi long-lived siIEL population while minimally impacting the maintenance of the CD127lo subset. Finally, Id2f/fId3f/f-ERCre+ P14 cells were studied to allow simultaneous deletion of both Id2 and Id3 in established Trm cells (Figure 5D). Notably, combined deficiency of both Id2 and Id3 resulted in an even greater loss of siIEL CD8+ T cells than deletion of Id2 alone, wherein the CD127hi siIEL CD8+ T cell population was profoundly affected with a ~3-fold greater loss of CD127hi siIEL with Id2 and Id3 deficiency compared to Id2 deficiency alone (Figure 5E). Further, deficiency of both Id2 and Id3 enhanced the frequency of CD11b+Tim3+ cells (Figure 5F). Thus, Id2 and Id3 are both critical regulators of Trm cell maintenance, especially for long-lived CD127hi siIEL cells.
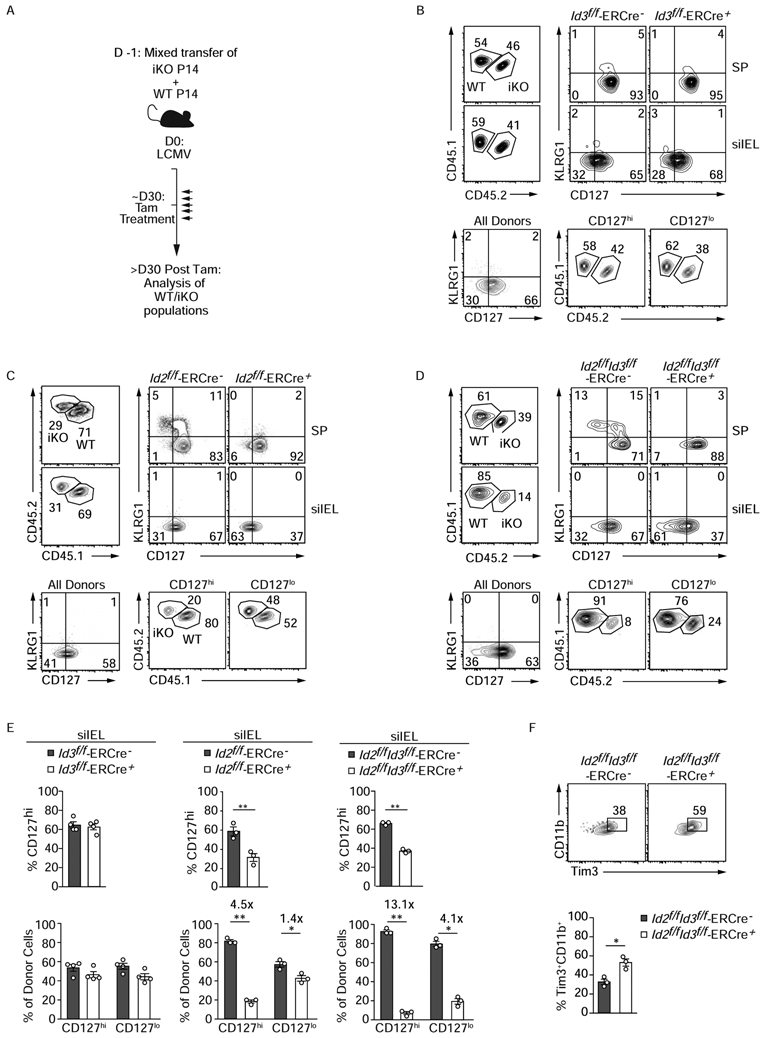
(A) Schematic of experimental set-up. A mix of congenically distinct Id2f/f-ERCre+, Id3f/f-ERCre+, or Id2f/fId3f/f-ERCre+ (iKO) and corresponding wild type ER-Cre− (WT) P14 CD8+ T cells were transferred to recipients that were subsequently infected with LCMV i.p. More than 30 days after infection, host mice were treated for 5 consecutive days with tamoxifen (Tam) to induce (B) Id3, (C) Id2 or (D) Id2 and Id3 deletion. Transferred P14 CD8+ T cells from host spleen and siIEL were analyzed by flow cytometry >30 days after the last Tam treatment. Frequency of WT and iKO cells among P14 CD8+ T cells (top left) and corresponding KLRG1 and CD127 expression (top right) is represented. The proportion of WT and iKO P14 CD8+ T cells within the CD127hi and CD127lo populations are also shown (bottom). (E) Quantification of indicated populations is displayed. (F) Phenotype of iId2/Id3 WT and DKO siIEL CD8+ T cells . Data are expressed as mean ± SEM. Numbers in plots represent frequency of cells in the indicated gate. All data are from one representative experiment of 2-3 independent experiments with n=3-5. Graphs show mean ± SEM; *p<0.05, **p<0.01. See also Figure S2 and S3.
As we detected expression of Id3 in siIEL CD8+ T cells as early as day 7 of infection (Figure 3A,,B),B), we also examined it’s contribution to Trm cell differentiation. Using the Id2f/fId3f/f-ERCre+ P14 cells transfer model, we induced deletion of Id2 and Id3 by administration of tamoxifen at days 3-6 of infection. On day 7 of infection, siIEL CD8+ T cells deficient for both Id2 and Id3 accumulated at a reduced frequency compared to their WT controls, and this defect was more pronounced when compared to loss of Id2 or Id3 alone (Figure S3), indicating that Id3 transcriptional regulation may contribute to the initial formation of siIEL CD8+ T cell populations.
Id3hi tumor-specific CD8+ T cells exhibit characteristics of Id3hi Trm and progenitor-exhausted cells
Tumor infiltrating CD8+ lymphocytes (TIL) display characteristics of Trm cells in certain settings (Ganesan et al., 2017; Milner et al., 2017; Savas et al., 2018). Here, we utilized bulk- and sc-RNA-seq analysis to expand on this finding and investigate if tumor-specific CD8+ T cells exhibited similar heterogeneity to that observed in siIEL CD8+ T cells. P14 CD8+ T cells were isolated from the spleen and tumor of B16-GP33-41 melanoma-bearing mice 7 days after adoptive transfer and were processed for scRNA-sequencing (Figure 6A). Despite TIL heterogeneity (Figure 6A), we found that TIL broadly exhibited enrichment of a tissue-residency gene-expression signature relative to tumor-specific T cells recovered from spleen (Figure 6B), and this enrichment was sustained after removal of characteristic ‘exhaustion’, ‘effector’, and ‘activation’ associated genes from the tissue-residency gene list (Figure 4SA). We next utilized our Id3-GFP/Blimp1-YFP double-reporter system to evaluate if tumor-residing P14 CD8+ T cells can be partitioned analogously to siIEL tissue-resident cells. Indeed, P14 TIL consisted of Id3loBlimp1hi, Id3loBlimp1lo, and Id3hiBlimp1lo populations (Figure 6C, Figure S4B,C). Further, RNAseq analysis revealed that Blimp1hi TIL displayed a gene-expression pattern enriched for the Blimp1hi siIEL CD8+ T cell signature, whereas Id3hi TIL shared a gene-expression pattern with Id3hi siIEL CD8+ T cells (Figure 6C). We extended this analysis by evaluating the relative enrichment of Blimp1hi and Id3hi Trm cell gene-expression signatures within individually profiled cells and limited our analysis to only TIL (Figure 6D--E).E). Discrete patterns of enrichment were observed among TIL, indicating certain tumor-specific T cells exhibited a Blimp1hi tissue-resident transcriptional profile while others had a more long-lived Id3hi tissue-resident transcriptional profile.
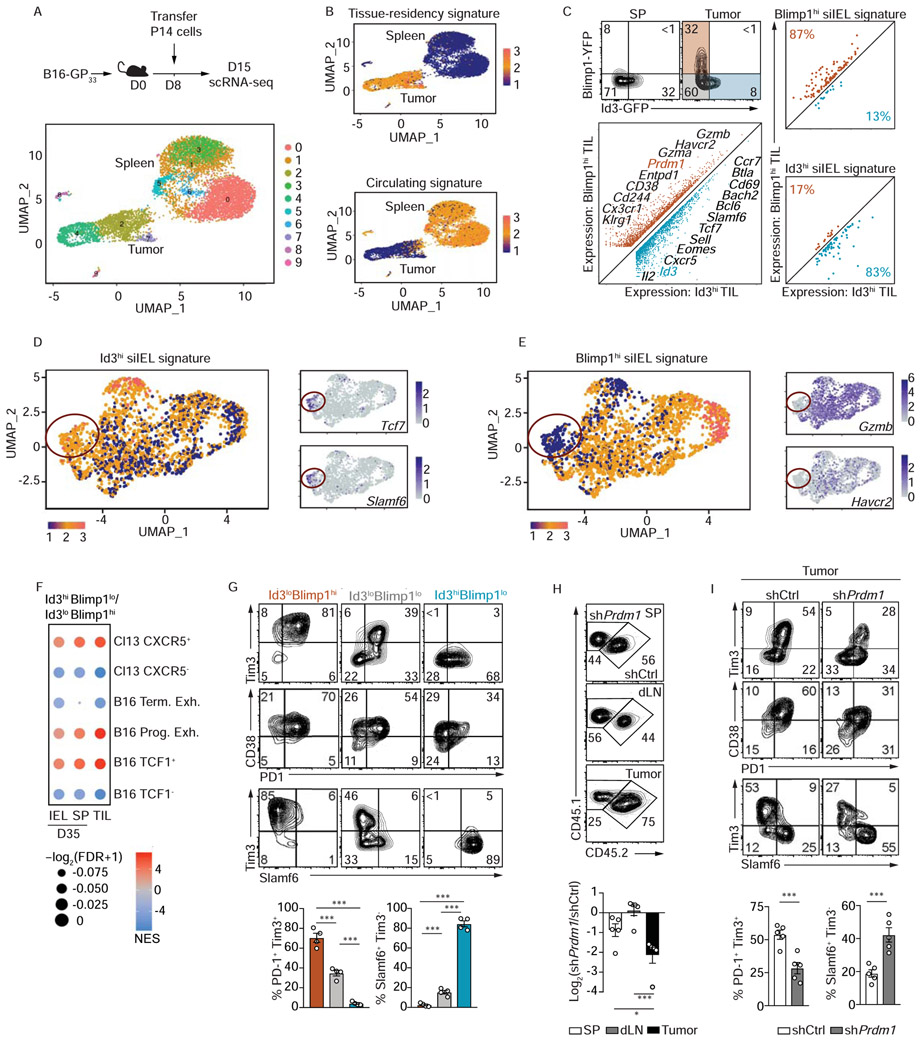
(A) Congenically distinct P14 CD8+ T cells were transferred into tumor-bearing mice, and seven days post adoptive transfer, P14 CD8+ T cells from spleens and tumors were sorted for scRNA-seq. UMAP plot of P14 CD8+ T cells from tumors and spleens. (B) UMAP plot indicating relative enrichment of a core tissue-residency signature (top) or enrichment of a core circulating signature (bottom). See also Figure S4A. (C) Representative flow cytometry plot demonstrating expression levels of Id3-GFP and Blimp1-YFP in P14 CD8+ T cells isolated from spleen or tumor (top). Expression plot from RNA-seq analysis of Blimp1hiId3lo and Blimp1loId3hi P14 CD8+ T cells from tumors (bottom left) and in Figure S4B,C. Relative expression levels of signature genes from Blimp1hi and Id3hi siIEL CD8+ T cells in Blimp1hiId3lo and Blimp1loId3hi P14 CD8+ T cells sorted from tumors. (D-E) UMAP plots (and in Figure S4A) indicating relative enrichment of the Blimp1hi (D) or Id3hi (E) siIEL CD8+ T cell signature in tumor localized P14 cells (left) as well as relative expression of highlighted genes (right). Red circle indicates distinct cluster of TIL enriched with the Id3hi Trm cell signature that also express elevated levels of genes upregulated in progenitor exhausted cells (Miller et al., 2019). (F) The CXCR5 (Im et al., 2016), B16 terminally/progenitor exhausted (Miller et al., 2019), and B16 TCF1 (Siddiqui et al., 2019) gene lists were used for gene set enrichment analyses in Id3hi cells relative to Blimp1hi cells. (G) Phenotype of Blimp1loId3hi, Blimp1loId3lo and Blimp1hiId3lo TIL. (H) Congenically distinct P14 CD8+ T cells were transduced with a Prdm1 shRNA encoding retrovirus (CD45.1+ P14 cells) or control shRNA (shCd19) encoding retrovirus (CD45.1+CD45.2+ P14 cells), mixed 1:1, and transferred into tumor bearing mice. Representative flow cytometry plots (top) and quantification (bottom) of the frequency of donor cells in the spleen, tumor draining lymph node, and tumor. Phenotype of Prdm1-deficient and control P14 CD8+ T cells from (H). Graphs show mean ± SEM of n=3-5 mice, from one representative experiment of 2 independent experiments. *p<0.05, ***p<0.005. See also Figure S4.
We noted that TIL enriched for the Blimp1hi signature expressed elevated levels of Havcr2 (encoding Tim3) and GzmB, whereas TIL enriched for the Id3hi signature expressed elevated levels of Slamf6 and Tcf7. It has previously been demonstrated that exhausted CD8+ T cells in the context of tumor or chronic infection are comprised of stem-like, progenitor exhausted (TCF1hiSlamf6hi Cxcr5hiGzBloTim3loCD38loPD-1mid) and terminally exhausted (TCF1loSlamf6loCxcr5loGzBhiTim3hiCD38hiPD-1hi) subsets (Brummelman et al., 2018; Kurtulus et al., 2019; Miller et al., 2019; Sade-Feldman et al., 2018; Siddiqui et al., 2019; Thommen et al., 2018; Vodnala et al., 2019). As our scRNA-seq analysis identified a cluster of TIL enriched for the Id3hi siIEL CD8+ T cell gene-expression signature also expressing progenitor exhausted T cell markers, we performed GSEA to place the Id3loBlimp1hi and Id3hiBlimp1lo CD8+ TIL subsets we identified within the context of already described exhausted populations (Im et al., 2016; Miller et al., 2019; Siddiqui et al., 2019). Id3hi TIL exhibited enrichment of gene-expression signatures associated with TCF1hi and progenitor exhausted T cell subsets identified in B16 tumors and CXCR5hi T cells responding to chronic LCMV infection, while Blimp1hi TIL were enriched with gene-expression signatures from TCF1lo and terminally exhausted TIL and CXCR5lo T cells of a chronic infection (Figure 6F). Flow cytometry analysis of TIL confirmed our gene-expression observations highlighting that the Id3hi TIL population expressed elevated levels of Slamf6 and lower amounts of CD38 and Tim3 compared to the corresponding Blimp1hi subset (Figure 6G). Principle component analysis and hierarchical clustering revealed Blimp1loId3lo TIL were transcriptionally more similar to Blimp1hi TIL rather than Id3hi TIL (Figure S4B,C). Last, we found that the Id3hi TIL subset expressed higher levels of CD69 relative to Blimp1loId3lo or Blimp1hi subsets, consistent with a Trm cell phenotype (Figure 6C, S5D).
Blimp1 is required for the formation of circulating TE and Tem cells (Kallies et al., 2009; Rutishauser et al., 2009), supports Trm cell differentiation (Mackay et al., 2016), and regulates PD-1 expression during LCMV Cl13 infection (Shin et al., 2009). As we found that Blimp1 is required for optimal formation of the effector-like siIEL CD8+ T cell population following LCMV infection (Figure S2), we tested if Blimp1 regulates the accumulation and differentiation of TIL. We used a mixed transfer system wherein congenically distinct P14 CD8+ T cells were transduced with a retroviral vector encoding shRNAs targeting Prdm1 or a negative control (Cd19), mixed at a 1:1 ratio and transferred into tumor bearing mice. One week following adoptive transfer, we found that the frequency of Prdm1-deficient CD8+ T cells within the tumor was 4-fold lower than control CD8+ T cells. In contrast Prdm1 deficiency did not dramatically impact the accumulation of T cells within the spleen or draining lymph node (Figure 6H), thus highlighting a specific role for Blimp1 in controlling TIL accumulation (analogous to the role of Blimp1 in regulating siIEL CD8+ T cell formation as in Figure S2A). Further, Blimp1 was crucial for instructing differentiation of the terminally exhausted CD8+ T cell population within the tumor microenvironment as Prdm1 deficiency reduced the frequency of PD-1+Tim3+ CD8+ T cells but increased the proportion of a Slamf6+Tim3− TIL (Figure 6I). These findings emphasize the transcriptional relationship between tissue-resident and tumor-resident CD8+ T cells and provide depth and understanding to the exhausted populations of tumor specific CD8+ T cells responsive to immunotherapies.
Discussion
Functional heterogeneity within the circulating CD8+ T cell compartment affords adaptability to diverse pathogens (Chen et al., 2018). Comparable diversity within the tissue-resident T cell population is less appreciated, and the key molecules often used to define residency, CD69 and CD103, are nearly uniformly expressed by siIEL Trm cell at memory timepoints (Casey et al., 2012; Mackay et al., 2013). Our data indicated that the Blimp1hiKLRG1hi/intCD127lo siIEL CD8+ T cell population that dominates the tissue early after infection ostensibly represented tissue-resident effector cells (during the effector phase of infection) or tissue-resident effector memory cells (during the memory phase of infection) as they were not only enriched for transcriptional signatures associated with more effector-like T cell populations (i.e. TE/Tem cells and Th1 cells) but also expressed elevated levels of effector molecule granzyme B. In contrast, Id3hiKLRG1loCD127hi siIEL CD8+ T cells appeared to be tissue-resident memory precursors (during the effector phase of infection) or tissue-resident memory cells (during the memory phase of infection) as they shared transcriptional signatures with other memory or memory-like populations (i.e. MP/Tcm cells and Tfh cells), exhibited greater multifunctionality, and had increased memory potential and multipotency with the capacity to generate both circulating and resident populations following re-infection. Trm cells can rapidly proliferate in situ following re-infection (Beura et al., 2018a; Park et al., 2018), and in certain contexts, may exit the tissue and rejoin the circulating memory pool (Beura et al., 2018b; Fonseca et al., 2020; Masopust et al., 2006). We speculate that it is the more stem-like Id3hi Trm cell subset that undergoes rapid local proliferation during secondary infection and differentiates into the circulating ex-Trm cells(Fonseca et al., 2020).
A number of reports have previously inferred heterogeneity exists within the tissue-resident compartment. Following Yersinia pseudotuberculosis infection, both CD103− and CD103+ Trm cell populations can be found within the lamina propria that have been suggested to contribute to immediate pathogen clearance or participate in the secondary response (Bergsbaken and Bevan, 2015; Bergsbaken et al., 2017). Diverse subsets of human Trm cells were also recently identified in multiple tissues (Kumar et al., 2018) including a population of Trm cells with features of longevity and quiescence that demonstrate a heightened proliferative capacity analogous to the Id3hi Trm cell population we report here. Furthermore, non-lymphoid residing KLRG1lo cells during early infection timepoints have previously been suggested to contain a putative Trm cell precursor population, consistent with our findings of an Id3hiBlimp1loKLRG1lo population during the effector phase of infection (Mackay et al., 2013; Sheridan et al., 2014).
Drawing comparisons from the circulating CD8+ T cell populations, we examined the requirement of previously defined T cell fate-specifying transcriptional regulators for differentiation and maintenance of the siIEL CD8+ T cell populations. We found that pro-effector transcriptional regulators (Blimp1, Id2, and T-bet) directed the differentiation of siIEL CD8+ T cells, consistent with previous studies describing that Blimp1 and T-bet control Trm cell formation (Laidlaw et al., 2014; Mackay et al., 2016; Mackay et al., 2015). However, here, we expanded on these findings in the context of the newly defined siIEL CD8+ T cell heterogeneity, and demonstrated that loss of Blimp1, Id2 or T-bet impaired formation of Blimp1hiKLRG1hi tissue-resident effector cells while the differentiation of the Id3hiKLRG1lo cells were less affected or even enhanced (Laidlaw et al., 2014; Mackay et al., 2015). Notably, both Id2 and Id3 were found to enforce siIEL CD8+ T cell homeostasis, and the CD127hi longer-lived Trm cell subset showed a greater dependency on these transcriptional regulators than CD127lo tissue-resident effector memory cells.
Conceptualization of Trm cell heterogeneity in comparison to the well-defined circulating memory T cell subsets provides context for understanding their functional differences. However, we and (Kurd et al., 2020) identified differentially expressed genes between distinct siIEL CD8+ T cell clusters within individual time points (day 4, 60 and 90) of LCMV infection beyond those described here that included transcription factors, chemokines, cytokines and associated signaling molecules, cell survival molecules, and costimulatory receptors, indicating substantially more complex heterogeneity. Thus, while parallels can be drawn with circulating memory subsets, it is clear that the siIEL CD8+ T cell population exhibits unique tissue-specific features and includes cells with a spectrum of function and memory potential.
Trm cell are present in highly diverse tissue environments so it is not surprising that tissue-specific variability also exists. Importantly, while Blimp1-expressing Trm cell were found in all tissues that we examined, Id3hi Trm cell were present within the gut and skin suggesting that these barrier tissues confer a tissue milieu that promotes and maintains Id3 expression. Trm cell cells within epithelial compartments require TGFβ for optimal differentiation and maintenance as it induces upregulation of CD103 to facilitate tissue retention (Casey et al., 2012; Mackay et al., 2013; Zhang and Bevan, 2013). TGFβ has also been shown to induce Id3 expression in various lymphoid populations (Kee et al., 2001), and thus may contribute to Id3 expression within the gut and skin Trm cell as well. This suggests an additional layer of Trm cell heterogeneity exists and that transcriptional programs within Trm cell are induced and supported by tissue-specific cues allowing Trm cell to adapt to the conditions posed by the local environment.
Accumulating evidence indicates that certain populations of TIL exhibit characteristics of tissue-resident cells, and the presence of Trm cell-like TIL is linked to positive prognoses in multiple malignancies and likely confers durable anti-tumor immunity (Clarke et al., 2019; Ganesan et al., 2017; Miller et al., 2019; Savas et al., 2018). Here, we provide evidence that despite heterogeneity, tumor-specific T cells are broadly enriched for a core tissue-residency gene-expression signature. Further, we found that sub-populations of TIL transcriptionally and phenotypically resemble Blimp1hi and Id3hi tissue-resident T cell populations. Consistent with Id3 marking multipotent or memory-like siIEL CD8+ T cells, Id3hi Trm cell-like TIL exhibited features of the recently described progenitor exhausted cells, a multipotent population of TIL that are responsive to immunotherapies such as cancer vaccination and immune checkpoint blockade (Im et al., 2016; Miller et al., 2019; Siddiqui et al., 2019). Taken together, both circulating and resident CD8+ T cells in the context of infection and cancer display phenotypic and functional heterogeneity, ranging from multipotent to terminally differentiated, endowing the immune system with flexible protection at sites of imminent pathogen exposure or ongoing disease.
STAR Methods
Resource Availability
Lead Contact
Further information and requests for resources and reagents should be directed to and will be fulfilled by the Lead Contact, Ananda W. Goldrath (ude.dscu@htardloga).
Materials Availability
This study did not generate new unique reagents
Data and Code Availability
The LCMV infection single-cell RNA sequencing data are available for download on the GEO data repository with accession number GSE131847 and all bulk RNA-seq datasets and tumor single-cell RNA-seq with the accession number GSE147502. The codes generated in this study are available at https://github.com/Arthurhe/scRNA_CD8T_Goldrath_Project.
Experimental Model and Subject Details
Mice
All mouse strains were bred and housed in specific pathogen–free conditions in accordance with the Institutional Animal Care and Use Guidelines of the University of California San Diego. Both male and female mice were used throughout the study, with sex and age matched T cell donors and recipients (or female donor cells transferred into male recipients). Blimp1-YFP mice (stock #008828;The Jackson Laboratory), Id3-GFP mice (Miyazaki et al., 2011), Id2-YFP mice (Yang et al., 2011), Prdm1fl/fl Granzyme B-Cre mice (Rutishauser et al., 2009), Tbx21+/− mice (stock #004648, The Jackson Laboratory), Id2fl/fl mice (Niola et al., 2012), Id3fl/fl mice (Guo et al., 2011), CD4-Cre mice (stock #017336; The Jackson Laboratory), Rosa26Cre-ERT2 (ERCre) (Hess Michelini et al., 2013), P14 mice (with transgenic expression of H-2Db-restricted TCR specific for LCMV glycoprotein gp333-41), OT-I mice (with transgenic expression of H-2Kb-restricted TCR specific for ovalbumin peptide 257-264; stock #003831; The Jackson Laboratory), CD45.1+, and CD45.1.2+ congenic mice were bred in house.
Cell Culture
B16 melanoma cells expressing the LCMV glycoprotein epitope amino acid 33-41 (B16-GP33-41) and PLAT-E cells were maintained in DMEM containing 5% bovine growth serum, 1% Hepes and 0.1% 2-Mercaptoethanol. Retroviral particles were generated in PLAT-E cells as previously described (Milner et al., 2017). For P14 CD8+ T cell transductions, spleens and lymph nodes were negatively enriched, activated and spinfected as previously described (Milner et al., 2017).
Method Details
Infection Studies
Wild-type, Blimp1-YFP, Id3-GFP, Id2-YFP/Id3-GFP or Blimp1-YFP/Id3-GFP P14 CD8+ T cells congenically distinct for CD45 were adoptively transferred at 5x104 cells per recipient mouse. For cotransfers, Prdm1fl/fl-granzyme B-Cre+, Id2fl/fl CD4-Cre+, Id2fl/fl ER-Cre+, Id3fl/fl ER-Cre+ or Id2fl/flId3fl/fl ER-Cre+ and corresponding control P14 CD8+ T cells were mixed in a 1:1 ratio and adoptively transferred at 5x104 total cells per recipient mouse. Alternatively, Tbx21+/− and Tbx21+/+ OT-I CD8+ T cells were mixed and similarly transferred to congenically distinct recipients. Mice were then infected with 2×105 pfu lymphocytic choriomeningitis virus-Armstrong (LCMV) or LCMV-Armstrong expressing ovalbumin (LCMV-OVA) by intraperitoneal injection or with 1x1010 Listeria monocytogenes expressing GP33 (LM-GP33) by oral gavage. For skin Trm cell studies, Id3-GFP P14 CD8+ T cells were transferred to congenically distinct recipients that were infected with LCMV by intraperitoneal injection as above. On day 4 of infection, 15μl of 0.3% 1-fluoro-2,4-dinitrobenzene (DNFB) in acetone/oil (4:1) was applied to the flank skin (Davies et al., 2017). For lung Trm cell studies, 1x105 P14 cells were transferred to congenically distinct recipients that were subsequently infected with 2x105 pfu LCMV via oropharyngeal aspiration. To distinguish vascular associated CD8+ T cells in certain non-lymphoid tissues (lung, salivary gland, kidney, brain, white adipose tissue), 3μg of CD8α (53–6.7) conjugated to APC eFlour780 was injected i.v. into mice three minutes prior to sacrifice and organ excision. Cells lacking CD8α labelling were considered to be localized within non-lymphoid tissues.
Tamoxifen Treatment
For ER-Cre-mediated deletion of floxed alleles, 1 mg tamoxifen (Cayman Chemical Company) emulsified in 100 μl of sunflower seed oil (Sigma-Aldrich) was administered by intraperitoneal injection for 4-5 consecutive days on day 3-6 of infection (for early deletion) or after 30 days of infection (for late deletion).
Tumor studies
B16-GP33 cells (5x105) were transplanted subcutaneously into the right flank of wild-type mice. After tumors became palpable, 7–8 days after transplantation, 1-2x106 in vitro expanded Blimp1-YFP/Id3-GFP P14 CD8+ T cells, WT P14 cells (for scRNAseq), or retrovirally transduced cells were transferred intravenously. For Prdm1 RNAi studies, congenically distinct shPrdm1 and shCtrl P14 cells were mixed 1:1 prior to transfer into tumor-bearing mice. Tumors were monitored daily and mice with ulcerated tumors or tumors exceeding 1500 mm3 in size were euthanized, in accordance with UCSD IACUC. Tumor infiltrating lymphocytes (TIL) were isolated as previously described (Milner et al., 2017) one week following adoptive transfer.
Preparation of Single Cell Suspensions
Single-cell suspensions were prepared from spleen or lymph node by mechanical disruption. For small intestine preparations, Peyer’s patches were excised, luminal contents were removed, and tissue was cut longitudinally then into 1cm pieces. The gut pieces were incubated while shaking in 10% HBSS/HEPES bicarbonate solution containing 15.4mg/100μL of dithioerthritol (EMD Millipore) at 37°C for 30 minutes to extract siIEL. For lamina propria lymphocyte isolation, gut pieces were further treated with 100U/ml type I collagenase (Worthington Biochemical) in RPMI-1640 containing 5% bovine growth serum, 2 mM MgCl2 and 2 mM CaCl2 at 37°C for 45 minutes. Brain, white adipose tissue, salivary gland, kidney, lung and tumor were cut with scissors into fine pieces then incubated while shaking with 100U/ml type I collagenase as above. Epidermal cells were isolated by floating skin dermis side down on 0.3% trypsin in 150mM NaCl, 6mM KCl, 6mM glucose, pH7.6 for 2hr at 37°C. The epidermis was then separated from the dermis and incubated with shaking for a further 10min at 37°C in 0.3% trypsin solution. Cells were then filtered through a sera separa column to remove any remaining tissue pieces. Lymphocytes from all tissue but skin, spleen and lymph node were purified on a 44%/67% Percoll density gradient.
Flow Cytometry and Cell Sorting
Cells were incubated for 30 min at 4°C in PBS supplemented with 2% bovine growth serum and 0.1% sodium azide. Intracellular staining was performed using the BD Cytofix/Cytoperm Solution Kit (BD Biosciences). For cytokine staining, siIEL CD8+ T cells were incubated for 3 hours at 37°C in RPMI-1640 media containing 10% (v/v) bovine growth serum with 10 nM GP33-41 peptide and Protein Transport Inhibitor (eBioscience) was added after 1 hour of incubation. CD107a (1D4B, BD Biosciences) antibody was included in the media for the entirety of the stimulation to detect surface expression as a surrogate of degranulation. Stained cells were analyzed using LSRFortessa or LSRFortessa X-20 cytometers (BD) and FlowJo software (TreeStar). All sorting was performed on BD FACSAria or BD FACSAria Fusion instruments.
Bulk RNA-Sequencing
On day 55 of LCMV infection,1x103 P14 cells from the spleen (CD62L+ Tcm cells and CD62L− Tem cells) or CD62L−CD103+ P14 cells from the siIEL were sorted into TCL buffer (Qiagen) with 1% 2-Mercaptoethanol. On day 7 or 35 day of LCMV infection, 1x103 Blimp1loId3hi , Blimp1hiid3lo Blimp1loId3lo P14 cells from the spleen, mesenteric lymph node or small intestine siIEL were sorted similarly. For tumor studies, 1x103 Blimp1loId3hi, Blimp1loId3lo, and Blimp1hiId3lo P14 cells were sorted from B16-GP33 tumors or spleens 7 days post adoptive transfer (14 days post-transplant). For all samples, polyA+ RNA was isolated and RNA-seq library preparation as well as RNA-seq analysis were carried out as described in (https://www.immgen.org/Protocols/11Cells.pdf). Heatmaps were generated using Morpheus (https://software.broadinstitute.org/morpheus). For Figure 1A, 3490 differentially expressed (D.E.) transcripts (≥2-fold, expression threshold ≥10) between all three populations of Tcm, Tem and Trm cells were identified through the Multiplot Studio module within Genepattern. The D.E. genes were then ordered through K-means clustering (set to 6 clusters) with the following modifications within Morpheus: metric=one minus pearson correlation; maximum number of iterations=1000. For the transcription factor heatmap in Figure 1A (right), transcription factors were picked from the larger heatmap (left) based on known or predicted roles in regulating the fate of Tcm or Tem cells. Heatmaps in Figures S1G and S4C were similarly generated except set to 3 clusters for K-means clustering analysis. RNA-sequencing was performed on duplicate samples as such: day 55 LCMV sorts, spleens or siIEL cells from two mice were pooled for one replicate; for day 7 LCMV and day 35 LCMV sorts, 2-5 spleens, mLNs or siIEL cells were pooled per one replicate; for TIL, spleens and tumors from two mice were pooled per replicate. For Figure S4A, the following gene lists were used: core Trm cell signature (Milner et al., 2017), ‘effector’ gene signature (Table S1, described below), ‘exhaustion’ gene signature (GSE41867_memory_vs_exhausted_CD8_Tcell_Day30_LCMV_DN), and ‘activation’ gene signature (transcripts increased in “T_8Eff_Sp_OT1-24hr_LisOva” compared to “T_8Nve_Sp_OT1” using the Population Comparison module in the Immgen database: www.immgen.org).
For triwise plots, 2-fold differentially expressed genes (between D55 Tcm, Tem and Trm cells; expression threshold >10) were filtered in Multiplot Studio based on the following gene lists: ‘Effector,’ ‘Memory,’ ‘Core Residency Signature,’ and ‘Core Circulating Signature.’ Expression values from filtered genes were then log2 transformed. Subsequently, triwise plots and rose plots were generated with the log2 transformed data using the Triwise R package (van de Laar et al., 2016) and following https://zouter.github.io/triwise/rd.html. The ‘Core Residency Signature’ and ‘Core Circulating Signature’ are from (Milner et al., 2017). The ‘effector’ and ‘memory’ signatures were generated using the ImmGen database (https://www.immgen.org/) and included as supplementary data. Specifically, utilizing default settings (expression threshold >120, 2-fold change) in the Population Comparison module, we identified differentially expressed genes between T_8Eff_Sp_OT1_d8_LisOva and T_8Mem_Sp_OT1_d100_LisOva. Genes upregulated in T_8Eff_Sp_OT1_d8_LisOva comprised the ‘effector’ signature (Table S1) and genes upregulated in T_8Mem_Sp_OT1_d100_LisOva comprised the ‘memory’ signature (Table S2).
RNAseq expression plots were generated in Multiplot Studio within GenePattern (expression threshold >10, 1.5-fold differentially expressed). In Figure 6C--E,E, the Id3hi siIEL signature is comprised of genes upregulated 1.5-fold in both D7 Id3hi IEL vs. D7 Blimp1hi IEL and D35 Id3hi IEL vs D35 Blimp1hi IEL comparisons, whereas the Blimp1hi siIEL signature is comprised of genes upregulated 1.5-fold in both D7 Id3hi IEL vs D7 Blimphi IEL and D35 Id3hi IEL vs D35 Blimp1hi IEL comparisons.
Gene set enrichment analysis was performed on each cell subset using the GSEA Preranked gene list tool with 1000 permutations and a weighted enrichment statistic. Gene sets were obtained by taking the top differentially expressed genes from microarray (GSE9650) or RNA-seq (GSE84105, GSE122713) data from the GEO database. Normalized enrichment scores (NES) and false discovery rate (FDR) were visualized using the ggplot2 package in R. For principal component analysis (PCA) plots, normalized counts were condensed to contain the top 535 differentially expressed genes between all samples with a padj < 0.05. PCA was performed using the prcomp function in R after centering and scaling the normalized counts.
10x Genomics Library Preparation and Sequencing
Activated P14 T cells (CD8+Vα2+CD45.1+CD44+) were sorted from the spleen or siIEL and resuspended in PBS+0.04% (w/v) bovine serum albumin. Approximately 10,000 cells per sample were loaded into Single Cell A chips (10x Genomics) and partitioned into Gel Bead In-Emulsions (GEMs) in a Chromium Controller (10x Genomics). Single cell RNA libraries were prepared according to the 10x Genomics Chromium Single Cell 3’ Reagent Kits v2 User Guide and sequenced on a HiSeq4000 (Illumina).
Infection studies:
Single-cell RNA-seq mapping
Reads from single-cell RNA-seq were aligned to mm10 and collapsed into unique molecular identifier (UMI) counts using the 10X Genomics Cell Ranger software (version 2.1.0). All samples had sufficient numbers of genes detected (>1000), a high percentage of reads mapped to the genome (>70%), and sufficient number of cells detected (>1000).
Cell and gene filtering
Raw cell-reads were then loaded to R using the cellrangerRkit package. The scRNA-seq dataset was then further filtered based on gene numbers and mitochondria gene counts to total counts ratio. Only cells with > 400 genes, UMI > 0, and 0.5% ~ 30% of their UMIs mapping to mitochondria genes were kept for downstream analysis. To ensure that memory requirements for all downstream analyses did not exceed 16Gb and that the samples with more cells would not dominate the downstream analysis, we randomly selected a portion of the cells that passed filtering for downstream analysis. We randomly selected 2000 cells from each library for downstream analysis. After cell filtering and sampling, we filtered genes by removing genes that did not express > 1 UMI in more than 1% of the total cells.
Single-cell RNA-seq dataset normalization and pre-processing
Five cell-gene matrices were generated:
(1) Raw UMI matrix.
(2) UPM matrix. The raw UMI matrix was normalized to get UMIs per million reads (UPM), and was then log2 transformed. All downstream differential analysis was based on the UPM matrix. The prediction models were also based on the UPM matrix, as other normalizations are very time-consuming for large datasets.
(3) MAGIC matrix. UPM matrix was further permuted by MAGIC (van Dijk et al., 2018) R package Rmagic 1.0.0 was used, and all options were kept as default. MAGIC aims to correct the drop-out effect of single-cell RNA-seq data; thus, we used MAGIC-corrected matrix for visualizing the gene expression pattern rather than using the UPM matrix. All gene expression overlaid on TSNE plots were based on the MAGIC matrix.
(4) Super cell matrix. We merged 50 cells to create a ‘super’ cell and used the super cell matrix as the input for cell type annotation analysis. This approach enabled us to bypass the issue of gene dropouts with scRNA-seq and make the data comparable to bulk RNA-seq. We first calculated the mutual nearest neighbor network with k set to 15, and then cells that were not mutual nearest neighbors with any other cells were removed as outliers. We randomly selected ‘n’ cells in the UPM matrix as the seed for super cells. The expression of each super cell was equal to the average expression of its seed and the 50 nearest neighbor cells of its seed. We derived 7400 super cells from the dataset, so each single cell was covered ~10 times.
Single-cell RNA-seq dataset dimension reduction
Top variable genes, PCA, and tSNE were calculated by Seurat version 2.3.4 functions: FindVariableGenes, RunPCA, and RunTSNE (Butler et al., 2018). Only the top 3000 genes were considered in the PCA calculation and only the top 25 principal components (PCs) were utilized in tSNE. Louvain clustering was performed by Seurat’s FindClusters function based on the top 25 PCs, with resolution set to 2. The scaled UPM matrix was used as input in the calculation.
Annotating single-cells with bulk RNA-seq signatures
The log2 TPM data from bulk RNA-seq datasets were compared with the scRNA-seq super cell matrix. Bulk cell population RNA-seq samples were first grouped into different sets according to their mutual similarities. For each bulk RNA-seq sample set, the mean expression was first calculated. The 1st correlation was calculated between all the super cells and the mean expression from the bulk RNA-seq dataset. Based on the distribution of the 1st correlation, we were able to identify a group of super cells that were most similar to the mean expression of the bulk sample. To further identify the small differences between bulk RNA-seq expression within a given set, we removed the set mean from the bulk RNA-seq and the mean from the most similar group of super cells, and then calculated the 2nd correlation between the super cells and bulk RNA-seq. Based on the 2nd correlation, we annotated the super cells with each bulk sample label.
Comparing gut and spleen CD8+ T cells
In order to compare the gut cells and spleen cells from the perspective of effector-memory differentiation, we performed a 3-step analysis. First, we calculated the differentially expressed gene between effector and memory T cells. We then performed principal component analysis on the gene expression of the DE genes of all spleen cells. Finally, we mapped both spleen cells and gut cells to the top two principle components calculated in step 2. Therefore, we can examine the heterogeneity of gut cells in a low-dimensional space that reveals the most significant difference between spleen effector and memory CD8 T cells.
Tumor studies
Analysis was performed similarly to the infection studies described above. Sequencing was performed on duplicate samples of splenocytes and TIL, wherein each replicate was pooled from 2-3 mice. Briefly, reads were aligned to mm10 and collapsed into UMI counts using the 10X Genomics Cell Ranger software. All samples had sufficient numbers of genes detected (>1000), a high percentage of reads mapped to the genome (>70%), and sufficient number of cells detected (>1000).
Raw cell-reads were then loaded to R using the Seurat package version 2.3.4 for further processing and quality control. The scRNA-seq dataset was filtered based on gene numbers and mitochondria gene counts to total counts ratio. Only cells with > 200 genes, UMI > 0, and 0%~5% of their UMIs mapping to mitochondria genes were kept for downstream analysis. Raw UMI matrix was normalized using Seurat function NormalizeData with the “LogNormalize” option, which normalized the feature expression measurements for each cell by the total expression, multiplies this by a scale factor (10,000 by default), and log-transforms the result. Normalized matrix was scaled using Seurat function ScaleData which regressed out cell-cell variation in gene expression driven by number of UMI and mitochondrial gene expression. The gene signatures used are described above in Bulk RNA-sequencing methods. For each signature, an overall score was calculated using AddModuleScore function in Seurat.
Quantification and Statistical Analysis
Statistical details of experiments can be found in the figure legends and methods. Statistical analysis was performed using GraphPad Prism software. Two-tailed paired or unpaired t-test was used for comparisons between groups. P values of <0.05 were considered significant.
KEY RESOURCES TABLE
REAGENT or RESOURCE | SOURCE | IDENTIFIER |
---|---|---|
Antibodies | ||
PE anti-CD5 (53-7.3) | BD Biosciences | Cat# 553023, RRID:AB_394561 |
APC-eFluor 780 anti-CD8α (53-6.7) | Thermo Fisher Scientific | Cat# 47-0081-82, RRID:AB_1272185 |
Brilliant Violet 785 anti-CD8α (53-6.7) | BioLegend | Cat# 100750, RRID:AB_2562610 |
PE anti-CD8β (eBioH35-17.2) | Thermo Fisher Scientific | Cat# 12-0083-82, RRID:AB_657767 |
eFluor 450 anti-CD8β (eBioH35-17.2) | Thermo Fisher Scientific | Cat# 48-0083-82, RRID:AB_11218504 |
PE anti-CD11b (M1/70) | Thermo Fisher Scientific | Cat# 12-0112-82, RRID:AB_2734869 |
PE anti-CD27 (LG.7F9) | Thermo Fisher Scientific | Cat# 12-0271-83, RRID:AB_465615 |
PerCP-eFluor 710 anti-CD27 (LG.7F9) | Thermo Fisher Scientific | Cat# 46-0271-80, RRID:AB_1834448 |
APC anti-CD38 | Thermo Fisher Scientific | Cat# 17-0381-82, RRID:AB_469382 |
PE/Cy7 anti-CD43 (1B11) | BioLegend | Cat# 121218, RRID:AB_528813 |
APC-eFluor 780 anti-CD45.1 (A20) | Thermo Fisher Scientific | Cat# 47-0453-82, RRID:AB_1582228 |
PE/Cy7 anti-CD45.1 (A20) | Thermo Fisher Scientific | Cat# 25-0453-82, RRID:AB_469629Invitrogen |
eFluor 450 anti-CD45.1 (A20) | Thermo Fisher Scientific | Cat# 48-0453-82, RRID:AB_1272189 |
Brilliant Violet 510 anti-CD45.1 (A20) | BioLegend | Cat# 110741, RRID:AB_2563378 |
FITC anti-CD45.2 (104) | Thermo Fisher Scientific | Cat# 11-0454-85, RRID:AB_465062 |
PE/Cy7 anti-CD45.2 (104) | Thermo Fisher Scientific | Cat# 25-0454-82, RRID:AB_2573350 |
PerCP/Cy5.5 anti-CD45.2 (104) | Thermo Fisher Scientific | Cat# 45-0454-82, RRID:AB_953590Invitrogen |
Brilliant Violet 510 anti-CD45.2 (104) | BioLegend | Cat# 109837, RRID:AB_2561393 |
APC/eF780 anti-CD62L | Thermo Fisher Scientific | Cat# 47-0621-82, RRID:AB_1603256 |
Brilliant Violet 510 anti-CD62L | BioLegend | Cat# 104441, RRID:AB_2561537 |
Brilliant Violet 711 anti-CD69 (H1.2F3) | BioLegend | Cat# 104537, RRID:AB_2566120 |
PE anti-CD103 (2E7) | Thermo Fisher Scientific | Cat# 12-1031-82, RRID:AB_465799 |
PE/Cy7 anti-CD103 (2E7) | BioLegend | Cat# 121426, RRID:AB_2563691 |
PE anti-CD127 (A7R34) | Thermo Fisher Scientific | Cat# 12-1271-83, RRID:AB_465845 |
PE/Cy7 anti-CD127 (A7R34) | Thermo Fisher Scientific | Cat# 25-1271-82, RRID:AB_469649 |
Brilliant Violet 421 anti-CD127 (A7R34) | BioLegend | Cat# 135027, RRID:AB_2563103 |
eFluor 660 anti-CD160 (CNX46-3) | Thermo Fisher Scientific | Cat# 50-1601-82, RRID:AB_11149495 |
APC/Fire 750 anti-CX3CR1 (SA011F11) | BioLegend | Cat# 149039, RRID:AB_2632859 |
APC anti-KLRG1 (2F1) | Thermo Fisher Scientific | Cat# 17-5893-82, RRID:AB_469469 |
eF450 anti-KLRG1 (2F1) | Thermo Fisher Scientific | Cat# 48-5893-82, RRID:AB_10852843 |
APC/eFluor 780 anti-PD1 (J43) | Thermo Fisher Scientific | Cat# 47-9985-82, RRID:AB_2574002 |
Brilliant Violet 650 anti-Ly108/Slamf6 (13G3) | BD Biosciences | Cat# 740628, RRID:AB_2740323 |
PE anti-Tim3 (RMT3-23) | Thermo Fisher Scientific | Cat# 12-5870-82, RRID:AB_465974 |
AF647 anti-Tim3 (B8.2C12) | BioLegend | Cat# 134006, RRID:AB_1626175 |
PE anti-BCL2 (3F11) | BD Biosciences | Cat# 556537, RRID:AB_396457 |
PE anti-EOMES (Dan11mag) | Thermo Fisher Scientific | Cat# 12-4875-80, RRID:AB_1603278 |
APC anti-Granzyme B (GB12) | Thermo Fisher Scientific | Cat# MHGB05, RRID:AB_10373420 |
Pacific Blue anti-IFN![]() | BioLegend | Cat# 505818, RRID:AB_893526 |
PE/Cy7 anti-IL-2 (JES6-5H4) | Thermo Fisher Scientific | Cat# 25-7021-82, RRID:AB_1235004 |
eFluor 660 anti-T-bet (4B10) | Thermo Fisher Scientific | Cat# 50-5825-82, RRID:AB_10596655 |
Pacific Blue anti-TCF1/TCF7 (C63D9) | Cell Signaling Technology | Cat# 9066, RRID:AB_2797696 |
APC anti-TNFα (MP6-XT22) | Thermo Fisher Scientific | Cat# 17-7321-82, RRID:AB_469508 |
PE anti-CD107a (1D4B) | BD Biosciences | Cat# 558661, RRID:AB_1645247 |
FITC anti-CD90.1 | Thermo Fisher Scientific | Cat# 11-0900-85, RRID:AB_465152 |
Biotin anti-B220 (RA3-6B2) | BioLegend | Cat# 103204, RRID:AB_312989 |
Biotin anti-MHCII (M5/114.15.2) | Thermo Fisher Scientific | Cat# 13-5321-82, RRID:AB_466662 |
Biotin anti-CD19 (6D5) | BioLegend | Cat# 115504, RRID:AB_313639 |
Biotin anti-CD4 (GK1.5) | BioLegend | Cat# 100404, RRID:AB_312689 |
Biotin anti-CD11b (M1/70) | Thermo Fisher Scientific | Cat# 13-0112-85, RRID:AB_466360 |
Biotin anti-CD49b (DX5) | Thermo Fisher Scientific | Cat# 13-5971-85, RRID:AB_466826 |
Biotin anti-Ter-199 (TER-199) | BioLegend | Cat# 116204, RRID:AB_313705 |
Biotin anti-GR-1 (RB6-8C5) | BioLegend | Cat# 108404, RRID:AB_313369 |
Bacterial and Virus Strains | ||
Lymphocytic choriomeningitis virus-Armstrong strain | Milner et al., 2017 | N/A |
Listeria monocytogenes-GP33 | Milner et al., 2017 | N/A |
Lymphocytic choriomeningitis virus-Armstrong strain expressing ovalbumin | Dr. E. Zuniga, UCSD | N/A |
Chemicals, Peptides, and Recombinant Proteins | ||
Collagenase Type I | Worthington Biochemicals | Cat# LS004197 |
Dithioerythritol | EMD Millipore | Cat# 233152 |
Tamoxifen | Caymen Chemicals | Cat# 13258 |
Percoll | Sigma | Cat# P1644 |
eBioscience Protein Transport Inhibitor Cocktail | Thermo Fisher Scientific | Cat# 00-4980-93 |
H-2Db -restricted peptide GP33-41 | Anaspec | Cat# AS-61296 |
MACS Streptavidin MicroBeads | Miltenyi Biotec | Cat# 130-048-101 |
1-fluor-2,4-dinitrobenzene | Sigma | Cat# D1529 |
Sunflower seed oil | Sigma | Cat# S5007 |
DMEM | Gibco | Cat# 11965-092 |
RPMI 1640 | Corning | Cat# 10-040-CV |
2-Mercaptoethanol | Gibco | Cat# 21985-023 |
HEPES | Gibco | Cat# 15630-080 |
Critical Commercial Assays | ||
BD Cytofix/Cytoperm Solution Kit | BD Biosciences | Cat# 554714 |
TransIT-LT1 Transfection Reagent | Mirus | Cat# MIR 2300 |
LS Columns | Miltenyi Biotec | Cat# 130-042 |
Deposited Data | ||
Tumor scRNA-seq | This paper | GSE147502 |
LCMV infection spleen D55 memory CD8+ T cell subsets | This paper | GSE147502 |
LCMV infection D7 and D35 spleen and siIEL CD8+ T cell subsets | This paper | GSE147502 |
Tumor D14 CD8+ T cell subsets | This paper | GSE147502 |
LCMV infection scRNA-seq | This paper with Kurd et al., 2020 | GSE131847 |
Experimental Models: Cell Lines | ||
B16- GP33-41 | Dr. A. Lamrre, INRS-institut Armand-Frappier | N/A |
PLAT-E | Cell Biolabs | Cat# RV-101, RRID:CVCL_B488 |
Experimental Models: Organisms/Strains | ||
Blimp1-YFP (B6.Cg-Tg(Prdm1-EYFP)1Mnz/J) | The Jackson Laboratory | Cat# 008828, RRID:IMSR_JAX:008828 |
Id3-GFP | Dr. C. Murre, UCSD (Miyazaki et al., 2011) | N/A |
Id2-YFP | Made in-house (Yang et al., 2011) | N/A |
Prdm1fl/fl Granzyme B-Cre | Dr. S. Kaech, Salk Institute for Biological Studies (Rutishauser et al., 2009) | N/A |
Tbx21+/− (B6.129S6-Tbx21tm1Glm/J) | The Jackson Laboratory | Cat# 004648, RRID:IMSR_JAX:004648 |
Id2fl/fl | Dr. A. Lasorella, Columbia University (Niola et al., 2012) | N/A |
Id3fl/fl | Dr. C. Murre (Guo et al., 2011) | N/A |
CD4-Cre (STOCK Tg(Cd4-cre)1Cwi/BfluJ) | The Jackson Laboratory | Cat# 017336, RRID:IMSR_JAX:017336 |
Rosa26Cre-ERT2 | Dr. S. Hedrick, UCSD | N/A |
P14 (B6.Cg-Tcratm1Mom Tg(TcrLCMV)327Sdz/TacMmjax) | The Jackson Laboratory | Cat# 037394-JAX, RRID:MMRRC_037394-JAX |
OT-1 (C57BL/6-Tg(TcraTcrb)1100Mjb/J) | The Jackson Laboratory | Cat# 003831, RRID:IMSR_JAX:003831 |
CD45.2 (C57BL/6J) | The Jackson Laboratory | Cat# 000664, RRID: IMSR_JAX:000664 |
CD45.1 (B6.SJL-Ptprca Pepcb/BoyJ) | The Jackson Laboratory | Cat# 002014, RRID:IMSR_JAX:002014 |
CD45.1.2 | Bred in-house | N/A |
Recombinant DNA | ||
shPrdm1 | Dr. M.Pipkin, The Scripps Research Institute (Milner et al., 2017) | N/A |
Software and Algorithms | ||
FlowJo v9 and v10 | Treestar Inc | RRID:SCR_008520 |
Prism 8 | Graphpad Inc | RRID:SCR_002798 |
Seurat v2.3.4 | https://satijalab.org/seurat/get_started.html | RRID:SCR_016341 |
Cell Ranger | 10X Genomics | RRID:SCR_017344 |
GenePattern | Broad Institute | RRID:SCR_003201 |
Morpheus | Broad Institute | RRID:SCR_017386 |
Triwise R | Van de Laar et al., 2016 | N/A |
Magic | Van Dijk et al., 2018 | N/A |
GSEA | Broad Institute | RRID:SCR_003199 |
Acknowledgements
This work was funded by the NIH grants AI132122 (A.W.G., G.W.Y., J.T.C.); K99 CA234430-01 (J.J.M.). Single-cell RNA-sequencing using the 10X Genomics platform was conducted at the IGM Genomics Center, University of California, San Diego, La Jolla, CA and supported by grants P30KC063491 and P30CA023100. We thank the Flow Cytometry Core at the La Jolla Institute for Allergy and Immunology for their assistance with cell sorting, and the Chang, and Goldrath laboratories for technical advice, helpful discussion, and critical reading of the manuscript.
Footnotes
Publisher's Disclaimer: This is a PDF file of an unedited manuscript that has been accepted for publication. As a service to our customers we are providing this early version of the manuscript. The manuscript will undergo copyediting, typesetting, and review of the resulting proof before it is published in its final form. Please note that during the production process errors may be discovered which could affect the content, and all legal disclaimers that apply to the journal pertain.
Declaration of Interests
A.W.G. is a member of the scientific advisory board for Pandion Therapeutics and Arsenal Biosciences.
References
- Arsenio J, Kakaradov B, Metz PJ, Kim SH, Yeo GW, and Chang JT (2014). Early specification of CD8+ T lymphocyte fates during adaptive immunity revealed by single-cell gene-expression analyses. Nat Immunol 15, 365–372. [Europe PMC free article] [Abstract] [Google Scholar]
- Banerjee A, Gordon SM, Intlekofer AM, Paley MA, Mooney EC, Lindsten T, Wherry EJ, and Reiner SL (2010). Cutting edge: The transcription factor eomesodermin enables CD8+ T cells to compete for the memory cell niche. J Immunol 185, 4988–4992. [Europe PMC free article] [Abstract] [Google Scholar]
- Bergsbaken T, and Bevan MJ (2015). Proinflammatory microenvironments within the intestine regulate the differentiation of tissue-resident CD8(+) T cells responding to infection. Nat Immunol 16, 406–414. [Europe PMC free article] [Abstract] [Google Scholar]
- Bergsbaken T, Bevan MJ, and Fink PJ (2017). Local Inflammatory Cues Regulate Differentiation and Persistence of CD8(+) Tissue-Resident Memory T Cells. Cell Rep 19, 114–124. [Europe PMC free article] [Abstract] [Google Scholar]
- Beura LK, Mitchell JS, Thompson EA, Schenkel JM, Mohammed J, Wijeyesinghe S, Fonseca R, Burbach BJ, Hickman HD, Vezys V, et al. (2018a). Intravital mucosal imaging of CD8(+) resident memory T cells shows tissue-autonomous recall responses that amplify secondary memory. Nat Immunol 19, 173–182. [Europe PMC free article] [Abstract] [Google Scholar]
- Beura LK, Wijeyesinghe S, Thompson EA, Macchietto MG, Rosato PC, Pierson MJ, Schenkel JM, Mitchell JS, Vezys V, Fife BT, et al. (2018b). T Cells in Nonlymphoid Tissues Give Rise to Lymph-Node-Resident Memory T Cells. Immunity 48, 327–338 e325. [Europe PMC free article] [Abstract] [Google Scholar]
- Brummelman J, Mazza EMC, Alvisi G, Colombo FS, Grilli A, Mikulak J, Mavilio D, Alloisio M, Ferrari F, Lopci E, et al. (2018). High-dimensional single cell analysis identifies stem-like cytotoxic CD8(+) T cells infiltrating human tumors. J Exp Med 215, 2520–2535. [Europe PMC free article] [Abstract] [Google Scholar]
- Butler A, Hoffman P, Smibert P, Papalexi E, and Satija R (2018). Integrating single-cell transcriptomic data across different conditions, technologies, and species. Nat Biotechnol 36, 411–420. [Europe PMC free article] [Abstract] [Google Scholar]
- Cannarile MA, Lind NA, Rivera R, Sheridan AD, Camfield KA, Wu BB, Cheung KP, Ding Z, and Goldrath AW (2006). Transcriptional regulator Id2 mediates CD8+ T cell immunity. Nat Immunol 7, 1317–1325. [Abstract] [Google Scholar]
- Casey KA, Fraser KA, Schenkel JM, Moran A, Abt MC, Beura LK, Lucas PJ, Artis D, Wherry EJ, Hogquist K, et al. (2012). Antigen-independent differentiation and maintenance of effector-like resident memory T cells in tissues. J Immunol 188, 4866–4875. [Europe PMC free article] [Abstract] [Google Scholar]
- Chen Y, Zander R, Khatun A, Schauder DM, and Cui W (2018). Transcriptional and Epigenetic Regulation of Effector and Memory CD8 T Cell Differentiation. Front Immunol 9, 2826. [Europe PMC free article] [Abstract] [Google Scholar]
- Clarke J, Panwar B, Madrigal A, Singh D, Gujar R, Wood O, Chee SJ, Eschweiler S, King EV, Awad AS, et al. (2019). Single-cell transcriptomic analysis of tissue-resident memory T cells in human lung cancer. J Exp Med 216, 2128–2149. [Europe PMC free article] [Abstract] [Google Scholar]
- Cui W, Liu Y, Weinstein JS, Craft J, and Kaech SM (2011). An interleukin-21-interleukin-10-STAT3 pathway is critical for functional maturation of memory CD8+ T cells. Immunity 35, 792–805. [Europe PMC free article] [Abstract] [Google Scholar]
- Davies B, Prier JE, Jones CM, Gebhardt T, Carbone FR, and Mackay LK (2017). Cutting Edge: Tissue-Resident Memory T Cells Generated by Multiple Immunizations or Localized Deposition Provide Enhanced Immunity. J Immunol 198, 2233–2237. [Abstract] [Google Scholar]
- Dominguez CX, Amezquita RA, Guan T, Marshall HD, Joshi NS, Kleinstein SH, and Kaech SM (2015). The transcription factors ZEB2 and T-bet cooperate to program cytotoxic T cell terminal differentiation in response to LCMV viral infection. J Exp Med 212, 2041–2056. [Europe PMC free article] [Abstract] [Google Scholar]
- Fonseca R, Beura LK, Quarnstrom CF, Ghoneim HE, Fan Y, Zebley CC, Scott MC, Fares-Frederickson NJ, Wijeyesinghe S, Thompson EA, et al. (2020). Developmental plasticity allows outside-in immune responses by resident memory T cells. Nat Immunol. [Europe PMC free article] [Abstract] [Google Scholar]
- Ganesan AP, Clarke J, Wood O, Garrido-Martin EM, Chee SJ, Mellows T, Samaniego-Castruita D, Singh D, Seumois G, Alzetani A, et al. (2017). Tissue-resident memory features are linked to the magnitude of cytotoxic T cell responses in human lung cancer. Nat Immunol 18, 940–950. [Europe PMC free article] [Abstract] [Google Scholar]
- Guan T, Dominguez CX, Amezquita RA, Laidlaw BJ, Cheng J, Henao-Mejia J, Williams A, Flavell RA, Lu J, and Kaech SM (2018). ZEB1, ZEB2, and the miR-200 family form a counterregulatory network to regulate CD8(+) T cell fates. J Exp Med 215, 1153–1168. [Europe PMC free article] [Abstract] [Google Scholar]
- Guo Z, Li H, Han M, Xu T, Wu X, and Zhuang Y (2011). Modeling Sjogren's syndrome with Id3 conditional knockout mice. Immunol Lett 135, 34–42. [Europe PMC free article] [Abstract] [Google Scholar]
- Hess Michelini R, Doedens AL, Goldrath AW, and Hedrick SM (2013). Differentiation of CD8 memory T cells depends on Foxo1. J Exp Med 210, 1189–1200. [Europe PMC free article] [Abstract] [Google Scholar]
- Ho AW, and Kupper TS (2019). T cells and the skin: from protective immunity to inflammatory skin disorders. Nat Rev Immunol. [Abstract] [Google Scholar]
- Ichii H, Sakamoto A, Hatano M, Okada S, Toyama H, Taki S, Arima M, Kuroda Y, and Tokuhisa T (2002). Role for Bcl-6 in the generation and maintenance of memory CD8+ T cells. Nat Immunol 3, 558–563. [Abstract] [Google Scholar]
- Im SJ, Hashimoto M, Gerner MY, Lee J, Kissick HT, Burger MC, Shan Q, Hale JS, Lee J, Nasti TH, et al. (2016). Defining CD8+ T cells that provide the proliferative burst after PD-1 therapy. Nature 537, 417–421. [Europe PMC free article] [Abstract] [Google Scholar]
- Jeannet G, Boudousquie C, Gardiol N, Kang J, Huelsken J, and Held W (2010). Essential role of the Wnt pathway effector Tcf-1 for the establishment of functional CD8 T cell memory. Proc Natl Acad Sci U S A 107, 9777–9782. [Europe PMC free article] [Abstract] [Google Scholar]
- Ji Y, Pos Z, Rao M, Klebanoff CA, Yu Z, Sukumar M, Reger RN, Palmer DC, Borman ZA, Muranski P, et al. (2011). Repression of the DNA-binding inhibitor Id3 by Blimp-1 limits the formation of memory CD8+ T cells. Nat Immunol 12, 1230–1237. [Europe PMC free article] [Abstract] [Google Scholar]
- Joshi NS, Cui W, Chandele A, Lee HK, Urso DR, Hagman J, Gapin L, and Kaech SM (2007). Inflammation directs memory precursor and short-lived effector CD8(+) T cell fates via the graded expression of T-bet transcription factor. Immunity 27, 281–295. [Europe PMC free article] [Abstract] [Google Scholar]
- Kakaradov B, Arsenio J, Widjaja CE, He Z, Aigner S, Metz PJ, Yu B, Wehrens EJ, Lopez J, Kim SH, et al. (2017). Early transcriptional and epigenetic regulation of CD8(+) T cell differentiation revealed by single-cell RNA sequencing. Nat Immunol 18, 422–432. [Europe PMC free article] [Abstract] [Google Scholar]
- Kallies A, Xin A, Belz GT, and Nutt SL (2009). Blimp-1 transcription factor is required for the differentiation of effector CD8(+) T cells and memory responses. Immunity 31, 283–295. [Abstract] [Google Scholar]
- Kee BL, Rivera RR, and Murre C (2001). Id3 inhibits B lymphocyte progenitor growth and survival in response to TGF-beta. Nat Immunol 2, 242–247. [Abstract] [Google Scholar]
- Knell J, Best JA, Lind NA, Yang E, D'Cruz LM, and Goldrath AW (2013). Id2 influences differentiation of killer cell lectin-like receptor G1(hi) short-lived CD8+ effector T cells. J Immunol 190, 1501–1509. [Europe PMC free article] [Abstract] [Google Scholar]
- Kumar BV, Kratchmarov R, Miron M, Carpenter DJ, Senda T, Lerner H, Friedman A, Reiner SL, and Farber DL (2018). Functional heterogeneity of human tissue-resident memory T cells based on dye efflux capacities. JCI Insight 3. [Europe PMC free article] [Abstract] [Google Scholar]
- Kurd NS, He Z, Milner JJ, Omilusik KD, Louis TL, Tsai MS, Widjaja CE, Kanbar JN, Olvera JG, Tysl T, et al. (2020). Molecular determinants and heterogeneity of tissue-resident memory CD8+ T lymphocytes revealed by single-cell RNA sequencing. bioRxiv, 2020.2003.2002.973578. [Europe PMC free article] [Abstract]
- Kurtulus S, Madi A, Escobar G, Klapholz M, Nyman J, Christian E, Pawlak M, Dionne D, Xia J, Rozenblatt-Rosen O, et al. (2019). Checkpoint Blockade Immunotherapy Induces Dynamic Changes in PD-1(−)CD8(+) Tumor-Infiltrating T Cells. Immunity 50, 181–194 e186. [Europe PMC free article] [Abstract] [Google Scholar]
- Laidlaw BJ, Zhang N, Marshall HD, Staron MM, Guan T, Hu Y, Cauley LS, Craft J, and Kaech SM (2014). CD4+ T cell help guides formation of CD103+ lung-resident memory CD8+ T cells during influenza viral infection. Immunity 41, 633–645. [Europe PMC free article] [Abstract] [Google Scholar]
- Mackay LK, Minnich M, Kragten NA, Liao Y, Nota B, Seillet C, Zaid A, Man K, Preston S, Freestone D, et al. (2016). Hobit and Blimp1 instruct a universal transcriptional program of tissue residency in lymphocytes. Science 352, 459–463. [Abstract] [Google Scholar]
- Mackay LK, Rahimpour A, Ma JZ, Collins N, Stock AT, Hafon ML, Vega-Ramos J, Lauzurica P, Mueller SN, Stefanovic T, et al. (2013). The developmental pathway for CD103(+)CD8+ tissue-resident memory T cells of skin. Nat Immunol 14, 1294–1301. [Abstract] [Google Scholar]
- Mackay LK, Wynne-Jones E, Freestone D, Pellicci DG, Mielke LA, Newman DM, Braun A, Masson F, Kallies A, Belz GT, and Carbone FR (2015). T-box Transcription Factors Combine with the Cytokines TGF-beta and IL-15 to Control Tissue-Resident Memory T Cell Fate. Immunity 43, 1101–1111. [Abstract] [Google Scholar]
- Masopust D, and Soerens AG (2019). Tissue-Resident T Cells and Other Resident Leukocytes. Annu Rev Immunol 37, 521–546. [Europe PMC free article] [Abstract] [Google Scholar]
- Masopust D, Vezys V, Wherry EJ, Barber DL, and Ahmed R (2006). Cutting edge: gut microenvironment promotes differentiation of a unique memory CD8 T cell population. J Immunol 176, 2079–2083. [Abstract] [Google Scholar]
- Masson F, Minnich M, Olshansky M, Bilic I, Mount AM, Kallies A, Speed TP, Busslinger M, Nutt SL, and Belz GT (2013). Id2-mediated inhibition of E2A represses memory CD8+ T cell differentiation. J Immunol 190, 4585–4594. [Europe PMC free article] [Abstract] [Google Scholar]
- Miller BC, Sen DR, Al Abosy R, Bi K, Virkud YV, LaFleur MW, Yates KB, Lako A, Felt K, Naik GS, et al. (2019). Subsets of exhausted CD8(+) T cells differentially mediate tumor control and respond to checkpoint blockade. Nat Immunol 20, 326–336. [Europe PMC free article] [Abstract] [Google Scholar]
- Milner JJ, and Goldrath AW (2018). Transcriptional programming of tissue-resident memory CD8(+) T cells. Curr Opin Immunol 51, 162–169. [Europe PMC free article] [Abstract] [Google Scholar]
- Milner JJ, Toma C, Yu B, Zhang K, Omilusik K, Phan AT, Wang D, Getzler AJ, Nguyen T, Crotty S, et al. (2017). Runx3 programs CD8(+) T cell residency in non-lymphoid tissues and tumours. Nature 552, 253–257. [Europe PMC free article] [Abstract] [Google Scholar]
- Miyazaki M, Rivera RR, Miyazaki K, Lin YC, Agata Y, and Murre C (2011). The opposing roles of the transcription factor E2A and its antagonist Id3 that orchestrate and enforce the naive fate of T cells. Nat Immunol 12, 992–1001. [Europe PMC free article] [Abstract] [Google Scholar]
- Mollo SB, Ingram JT, Kress RL, Zajac AJ, and Harrington LE (2014). Virus-specific CD4 and CD8 T cell responses in the absence of Th1-associated transcription factors. J Leukoc Biol 95, 705–713. [Europe PMC free article] [Abstract] [Google Scholar]
- Nguyen QP, Deng TZ, Witherden DA, and Goldrath AW (2019). Origins of CD4(+) circulating and tissue-resident memory T-cells. Immunology 157, 3–12. [Abstract] [Google Scholar]
- Niola F, Zhao X, Singh D, Castano A, Sullivan R, Lauria M, Nam HS, Zhuang Y, Benezra R, Di Bernardo D, et al. (2012). Id proteins synchronize stemness and anchorage to the niche of neural stem cells. Nat Cell Biol 14, 477–487. [Europe PMC free article] [Abstract] [Google Scholar]
- Olson JA, McDonald-Hyman C, Jameson SC, and Hamilton SE (2013). Effector-like CD8(+) T cells in the memory population mediate potent protective immunity. Immunity 38, 1250–1260. [Europe PMC free article] [Abstract] [Google Scholar]
- Omilusik KD, Best JA, Yu B, Goossens S, Weidemann A, Nguyen JV, Seuntjens E, Stryjewska A, Zweier C, Roychoudhuri R, et al. (2015). Transcriptional repressor ZEB2 promotes terminal differentiation of CD8+ effector and memory T cell populations during infection. J Exp Med 212, 2027–2039. [Europe PMC free article] [Abstract] [Google Scholar]
- Omilusik KD, Nadjsombati MS, Shaw LA, Yu B, Milner JJ, and Goldrath AW (2018). Sustained Id2 regulation of E proteins is required for terminal differentiation of effector CD8(+) T cells. J Exp Med 215, 773–783. [Europe PMC free article] [Abstract] [Google Scholar]
- Park SL, Zaid A, Hor JL, Christo SN, Prier JE, Davies B, Alexandre YO, Gregory JL, Russell TA, Gebhardt T, et al. (2018). Local proliferation maintains a stable pool of tissue-resident memory T cells after antiviral recall responses. Nat Immunol 19, 183–191. [Abstract] [Google Scholar]
- Pearce EL, Mullen AC, Martins GA, Krawczyk CM, Hutchins AS, Zediak VP, Banica M, DiCioccio CB, Gross DA, Mao CA, et al. (2003). Control of effector CD8+ T cell function by the transcription factor Eomesodermin. Science 302, 1041–1043. [Abstract] [Google Scholar]
- Rao RR, Li Q, Gubbels Bupp MR, and Shrikant PA (2012). Transcription factor Foxo1 represses T-bet-mediated effector functions and promotes memory CD8(+) T cell differentiation. Immunity 36, 374–387. [Europe PMC free article] [Abstract] [Google Scholar]
- Roychoudhuri R, Clever D, Li P, Wakabayashi Y, Quinn KM, Klebanoff CA, Ji Y, Sukumar M, Eil RL, Yu Z, et al. (2016). BACH2 regulates CD8(+) T cell differentiation by controlling access of AP-1 factors to enhancers. Nat Immunol 17, 851–860. [Europe PMC free article] [Abstract] [Google Scholar]
- Rutishauser RL, Martins GA, Kalachikov S, Chandele A, Parish IA, Meffre E, Jacob J, Calame K, and Kaech SM (2009). Transcriptional repressor Blimp-1 promotes CD8(+) T cell terminal differentiation and represses the acquisition of central memory T cell properties. Immunity 31, 296–308. [Europe PMC free article] [Abstract] [Google Scholar]
- Sade-Feldman M, Yizhak K, Bjorgaard SL, Ray JP, de Boer CG, Jenkins RW, Lieb DJ, Chen JH, Frederick DT, Barzily-Rokni M, et al. (2018). Defining T Cell States Associated with Response to Checkpoint Immunotherapy in Melanoma. Cell 175, 998-+. [Europe PMC free article] [Abstract] [Google Scholar]
- Savas P, Virassamy B, Ye C, Salim A, Mintoff CP, Caramia F, Salgado R, Byrne DJ, Teo ZL, Dushyanthen S, et al. (2018). Single-cell profiling of breast cancer T cells reveals a tissue-resident memory subset associated with improved prognosis. Nat Med 24, 986–993. [Abstract] [Google Scholar]
- Sheridan BS, Pham QM, Lee YT, Cauley LS, Puddington L, and Lefrancois L (2014). Oral infection drives a distinct population of intestinal resident memory CD8(+) T cells with enhanced protective function. Immunity 40, 747–757. [Europe PMC free article] [Abstract] [Google Scholar]
- Shin H, Blackburn SD, Intlekofer AM, Kao C, Angelosanto JM, Reiner SL, and Wherry EJ (2009). A role for the transcriptional repressor Blimp-1 in CD8(+) T cell exhaustion during chronic viral infection. Immunity 31, 309–320. [Europe PMC free article] [Abstract] [Google Scholar]
- Siddiqui I, Schaeuble K, Chennupati V, Fuertes Marraco SA, Calderon-Copete S, Pais Ferreira D, Carmona SJ, Scarpellino L, Gfeller D, Pradervand S, et al. (2019). Intratumoral Tcf1(+)PD-1(+)CD8(+) T Cells with Stem-like Properties Promote Tumor Control in Response to Vaccination and Checkpoint Blockade Immunotherapy. Immunity 50, 195–211 e110. [Abstract] [Google Scholar]
- Skon CN, Lee JY, Anderson KG, Masopust D, Hogquist KA, and Jameson SC (2013). Transcriptional downregulation of S1pr1 is required for the establishment of resident memory CD8+ T cells. Nat Immunol 14, 1285–1293. [Europe PMC free article] [Abstract] [Google Scholar]
- Szabo PA, Miron M, and Farber DL (2019). Location, location, location: Tissue resident memory T cells in mice and humans. Sci Immunol 4. [Europe PMC free article] [Abstract] [Google Scholar]
- Thommen DS, Koelzer VH, Herzig P, Roller A, Trefny M, Dimeloe S, Kiialainen A, Hanhart J, Schill C, Hess C, et al. (2018). A transcriptionally and functionally distinct PD-1(+) CD8(+) T cell pool with predictive potential in non-small-cell lung cancer treated with PD-1 blockade. Nat Med 24, 994–1004. [Europe PMC free article] [Abstract] [Google Scholar]
- van de Laar L, Saelens W, De Prijck S, Martens L, Scott CL, Van Isterdael G, Hoffmann E, Beyaert R, Saeys Y, Lambrecht BN, and Guilliams M (2016). Yolk Sac Macrophages, Fetal Liver, and Adult Monocytes Can Colonize an Empty Niche and Develop into Functional Tissue-Resident Macrophages. Immunity 44, 755–768. [Abstract] [Google Scholar]
- van Dijk D, Sharma R, Nainys J, Yim K, Kathail P, Carr AJ, Burdziak C, Moon KR, Chaffer CL, Pattabiraman D, et al. (2018). Recovering Gene Interactions from Single-Cell Data Using Data Diffusion. Cell 174, 716–729 e727. [Europe PMC free article] [Abstract] [Google Scholar]
- Vodnala SK, Eil R, Kishton RJ, Sukumar M, Yamamoto TN, Ha NH, Lee PH, Shin M, Patel SJ, Yu Z, et al. (2019). T cell stemness and dysfunction in tumors are triggered by a common mechanism. Science 363. [Europe PMC free article] [Abstract] [Google Scholar]
- Wakim LM, Woodward-Davis A, Liu R, Hu Y, Villadangos J, Smyth G, and Bevan MJ (2012). The molecular signature of tissue resident memory CD8 T cells isolated from the brain. J Immunol 189, 3462–3471. [Europe PMC free article] [Abstract] [Google Scholar]
- Wherry EJ, Teichgraber V, Becker TC, Masopust D, Kaech SM, Antia R, von Andrian UH, and Ahmed R (2003). Lineage relationship and protective immunity of memory CD8 T cell subsets. Nat Immunol 4, 225–234. [Abstract] [Google Scholar]
- Yang CY, Best JA, Knell J, Yang E, Sheridan AD, Jesionek AK, Li HS, Rivera RR, Lind KC, D'Cruz LM, et al. (2011). The transcriptional regulators Id2 and Id3 control the formation of distinct memory CD8+ T cell subsets. Nat Immunol 12, 1221–1229. [Europe PMC free article] [Abstract] [Google Scholar]
- Zhang N, and Bevan MJ (2013). Transforming growth factor-beta signaling controls the formation and maintenance of gut-resident memory T cells by regulating migration and retention. Immunity 39, 687–696. [Europe PMC free article] [Abstract] [Google Scholar]
- Zhou X, Yu S, Zhao DM, Harty JT, Badovinac VP, and Xue HH (2010). Differentiation and persistence of memory CD8(+) T cells depend on T cell factor 1. Immunity 33, 229–240. [Europe PMC free article] [Abstract] [Google Scholar]
Full text links
Read article at publisher's site: https://doi.org/10.1016/j.immuni.2020.04.007
Read article for free, from open access legal sources, via Unpaywall:
http://www.cell.com/article/S1074761320301655/pdf
Citations & impact
Impact metrics
Citations of article over time
Alternative metrics
Smart citations by scite.ai
Explore citation contexts and check if this article has been
supported or disputed.
https://scite.ai/reports/10.1016/j.immuni.2020.04.007
Article citations
Higher frequency of peripheral blood CD103<sup>+</sup>CD8<sup>+</sup> T cells with lower levels of PD-1 and TIGIT expression related to favorable outcomes in leukemia patients.
Front Immunol, 15:1437726, 25 Sep 2024
Cited by: 0 articles | PMID: 39391310 | PMCID: PMC11465237
Longitudinal Intravascular Antibody Labeling Identified Regulatory T Cell Recruitment as a Therapeutic Target in a Mouse Model of Lung Cancer.
J Immunol, 213(6):906-918, 01 Sep 2024
Cited by: 1 article | PMID: 39082930
Epithelial organoid supports resident memory CD8 T cell differentiation.
Cell Rep, 43(8):114621, 15 Aug 2024
Cited by: 0 articles | PMID: 39153200 | PMCID: PMC11401477
Protective function and differentiation cues of brain-resident CD8+ T cells during surveillance of latent Toxoplasma gondii infection.
Proc Natl Acad Sci U S A, 121(24):e2403054121, 05 Jun 2024
Cited by: 2 articles | PMID: 38838017
Resident memory T cells and cancer.
Immunity, 57(8):1734-1751, 01 Aug 2024
Cited by: 0 articles | PMID: 39142275
Review
Go to all (124) article citations
Data
Data behind the article
This data has been text mined from the article, or deposited into data resources.
BioStudies: supplemental material and supporting data
GEO - Gene Expression Omnibus (2)
- (1 citation) GEO - GSE147502
- (1 citation) GEO - GSE131847
RRID - Resource Identification Portal (5)
- (1 citation) RRID - RRIDAB_11218504
- (1 citation) RRID - RRIDAB_10596655
- (1 citation) RRID - RRIDAB_10852843
- (1 citation) RRID - RRIDAB_11149495
- (1 citation) RRID - RRIDAB_10373420
Similar Articles
To arrive at the top five similar articles we use a word-weighted algorithm to compare words from the Title and Abstract of each citation.
Single-Cell Transcriptomics Reveals Core Regulatory Programs That Determine the Heterogeneity of Circulating and Tissue-Resident Memory CD8+ T Cells.
Cells, 10(8):2143, 20 Aug 2021
Cited by: 10 articles | PMID: 34440912 | PMCID: PMC8392357
Cutting edge: resident memory CD8 T cells occupy frontline niches in secondary lymphoid organs.
J Immunol, 192(7):2961-2964, 05 Mar 2014
Cited by: 131 articles | PMID: 24600038 | PMCID: PMC3965619
Id3 Controls Cell Death of 2B4+ Virus-Specific CD8+ T Cells in Chronic Viral Infection.
J Immunol, 195(5):2103-2114, 31 Jul 2015
Cited by: 10 articles | PMID: 26232435
Exhausted CD8+ T cells face a developmental fork in the road.
Trends Immunol, 44(4):276-286, 11 Mar 2023
Cited by: 11 articles | PMID: 36907685
Review
Funding
Funders who supported this work.
NCI NIH HHS (2)
Grant ID: P30 CA023100
Grant ID: K99 CA234430
NIAID NIH HHS (2)
Grant ID: R37 AI067545
Grant ID: P01 AI132122
NIDDK NIH HHS (1)
Grant ID: P30 DK063491