Abstract
Background
The antiviral efficacy of remdesivir in COVID-19 hospitalized patients remains controversial.Objectives
To estimate the effect of remdesivir in blocking viral replication.Methods
We analysed nasopharyngeal normalized viral loads from 665 hospitalized patients included in the DisCoVeRy trial (NCT04315948; EudraCT 2020-000936-23), randomized to either standard of care (SoC) or SoC + remdesivir. We used a mathematical model to reconstruct viral kinetic profiles and estimate the antiviral efficacy of remdesivir in blocking viral replication. Additional analyses were conducted stratified on time of treatment initiation (≤7 or >7 days since symptom onset) or viral load at randomization (< or ≥3.5 log10 copies/104 cells).Results
In our model, remdesivir reduced viral production by infected cells by 2-fold on average (95% CI: 1.5-3.2-fold). Model-based simulations predict that remdesivir reduced time to viral clearance by 0.7 days compared with SoC, with large inter-individual variabilities (IQR: 0.0-1.3 days). Remdesivir had a larger impact in patients with high viral load at randomization, reducing viral production by 5-fold on average (95% CI: 2.8-25-fold) and the median time to viral clearance by 2.4 days (IQR: 0.9-4.5 days).Conclusions
Remdesivir halved viral production, leading to a median reduction of 0.7 days in the time to viral clearance compared with SoC. The efficacy was larger in patients with high viral load at randomization.Free full text

Effect of remdesivir on viral dynamics in COVID-19 hospitalized patients: a modelling analysis of the randomized, controlled, open-label DisCoVeRy trial
Abstract
Background
The antiviral efficacy of remdesivir in COVID-19 hospitalized patients remains controversial.
Objectives
To estimate the effect of remdesivir in blocking viral replication.
Methods
We analysed nasopharyngeal normalized viral loads from 665 hospitalized patients included in the DisCoVeRy trial (NCT 04315948; EudraCT 2020-000936-23), randomized to either standard of care (SoC) or SoC + remdesivir. We used a mathematical model to reconstruct viral kinetic profiles and estimate the antiviral efficacy of remdesivir in blocking viral replication. Additional analyses were conducted stratified on time of treatment initiation (≤7 or >7 days since symptom onset) or viral load at randomization (< or ≥3.5 log10 copies/104 cells).
Results
In our model, remdesivir reduced viral production by infected cells by 2-fold on average (95% CI: 1.5–3.2-fold). Model-based simulations predict that remdesivir reduced time to viral clearance by 0.7 days compared with SoC, with large inter-individual variabilities (IQR: 0.0–1.3 days). Remdesivir had a larger impact in patients with high viral load at randomization, reducing viral production by 5-fold on average (95% CI: 2.8–25-fold) and the median time to viral clearance by 2.4 days (IQR: 0.9–4.5 days).
Conclusions
Remdesivir halved viral production, leading to a median reduction of 0.7 days in the time to viral clearance compared with SoC. The efficacy was larger in patients with high viral load at randomization.
Introduction
Remarkable progress has recently been made in finding effective antiviral treatments in SARS-CoV-2-infected patients that can prevent disease progression and hospitalization. These treatments, which can rely either on monoclonal antibodies1,2 or small molecules,3,4 need to be administered early in the infection, typically in the first week after symptom onset, to be fully effective. Although the use of monoclonal antibodies has shown some encouraging results in the Recovery trial,5 the role of antiviral treatments in hospitalized patients to prevent mechanical ventilation and death remains unclear. Among these drugs, remdesivir has received an emergency use authorization for the treatment of COVID-19 hospitalized patients in several countries, and is approved for hospitalized patients in the United States.6 However, its clinical efficacy remains controversial, with some randomized clinical trials pointing to efficacy in preventing disease worsening, and others finding no efficacy.7–10
Remdesivir is a nucleoside analogue prodrug inhibiting RNA polymerase activity of several viruses11 that has shown antiviral activity against SARS-CoV-2 both in vitro and in animal models.12–14 An important element to precisely evaluate remdesivir is to analyse its effect on viral dynamics, which is likely a prerequisite to clinical efficacy. However, results from the literature are still scarce. In three randomized and controlled clinical trials, no difference in viral load levels were found between hospitalized patients receiving remdesivir and those that did not receive remdesivir.7,10,15 In two of these studies,7,8 the analysis of the effect was hampered by limited number of patients (with 237 and 181 patients included) and by the design of the analysis, which compared viral load at different timepoints and not the overall effect on viral dynamics.7 Moreover, the results remained limited by the heterogeneity in the biological samples and the molecular techniques used. In the larger DisCoVeRy trial, which used normalized viral loads,15 no effect of remdesivir on viral dynamics was found, but the variability of the time interval between the onset of symptoms and treatment initiation, which is a key factor for antiviral drug evaluation,16,17 was not considered. One potential approach to address this issue is to use a model-based approach to reconstitute the precise effect of treatment on the course of viral dynamics, as we previously showed in a cohort of hospitalized patients.18
Here, we developed this approach to estimate the effect of remdesivir in inhibiting viral replication. We used normalized and centralized data on viral kinetics from the European randomized controlled DisCoVeRy trial that compared the efficacy of remdesivir plus standard of care (SoC) with SoC alone in COVID-19 hospitalized patients.15,19
Patients and methods
Study design and data collection
Hospitalized patients with a laboratory-confirmed SARS-CoV-2 infection were enrolled in the DisCoVeRy trial (NCT 04315948; EudraCT 2020-000936-2315), sponsored by the Institut national de la santé et de la recherche médicale (Inserm, France). Written informed consent was obtained from all included participants (or their legal representatives if unable to consent). We analysed the results obtained from patients allocated to receive either SoC alone or SoC plus remdesivir between March 2020 and January 2021, hospitalized at 48 different sites in France, Belgium, Portugal, Austria and Luxembourg, and for whom nasopharyngeal swabs were available. Given that patients who were reported to arrive very late in their disease could be indicative of a poor reporting of the time of symptom onset, we removed from our analysis the N = 17 patients whose delay between the reported time of symptom onset and the randomization was larger than 20 days. Remdesivir was administered intravenously at a loading dose of 200 mg on day 1 followed by 100 mg infusions once-daily for up to 10 days. More details on study design, ethics approval and inclusion/exclusion criteria can be found in the initial trial report.15
Viral load measurements
Normalized viral load in samples was measured at randomization and at days 3, 5, 8, 11, 15 ± 2 and 29 ± 3 after randomization, in nasopharyngeal swabs collected through validated devices containing flocked swabs and virus transport medium.
To allow the comparison of samples of different qualities (cell richness) we followed the same methodology of normalized viral load developed previously in the context of influenza virus infection.20 In brief, the normalized SARS-CoV-2 viral load was determined by RT-PCR blinded to treatment arm, divided by the number of cells measured (quantification of HPRT-1 housekeeping gene) and expressed in copies per 104 cells. All samples were centralized and analysed in the same laboratory at the National Centre for Viral Respiratory Infections (Hospices Civils de Lyon, France). We estimated the limit of detection (LoD) as 1 log10 copies/104 cells and all viral loads strictly below LoD were considered as censored.15
Viral load was also assessed in broncho-alveolar lavage (BAL) fluids for a subset of patients, at the clinician’s discretion (see Discussion).
Viral dynamics model
We used a mathematical model to reconstruct the viral kinetics from the time of infection (t = 0) until viral clearance. The model incorporates an effect of remdesivir, denoted as , that reduces viral production after a pharmacological delay, denoted as τ. The estimation of both viral kinetic and treatment parameters was obtained through fitting of the model to the virological data from both remdesivir-treated and -untreated patients from the Discovery trial.
Model equations
Given the small number of patients hospitalized in the first days after symptom onset,15 we used a target-cell limited model with an eclipse phase to characterize viral dynamics,18 and did not consider more complex models.18 The model includes target cells (T) and infected cells, that are initially non-productive (I1)during the eclipse phase and become subsequently productively infected (I2). The model assumes that target cells are infected at a constant infection rate β, and the mean duration of the eclipse phase is noted 1/k. Productively infected cells have a constant loss rate, denoted δ, that differs between patients above or below 65 years old.18 Each infected cell produces p viral particles per day, but only a fraction of these particles, μ are infectious. We note V the sum of infectious viral particles, Vi and of non-infectious viral particles, Vni and both are cleared at rate c. The model can be written as:
At the time of infection, we assumed that there is exactly one productively infected cell I2 in the entire nasopharyngeal tract, thus: T = T0; I1 = 0; I2 = 1; Vi = 0; Vni = 0. The basic reproductive number R0, defined as the number of secondary infected cells resulting from one infected cell in a population of fully susceptible cells, is equal to βpT0μ/cδ where T0, the total number of susceptible cells, is equal to 4 × 106 cells.21 We used a scaling factor, f, to convert V into a normalized viral load and we note Vobs = f × V. As only the parameter p×f can be estimated, we assumed without loss of generality that the proportion of susceptible cells in the biological sample was on average 10-fold lower than in the nasopharyngeal compartment, i.e. 0.1%.21 Thus, we fixed f to 10−3 × 104/4 × 106 = 2.5 × 10−6. Changing the value of the scaling factor would affect the estimate of p, but not the value of the other parameters, including the estimation of R0 and the treatment efficacy (see below).
We fixed c to 10 days−1, k to 4 days−1 and μ to 10−4 as previously published.16,18,22 Further we fixed the time of infection to 5 days prior to symptom onset in all individuals, which corresponds to the mean duration of the incubation phase.18 We estimated p and δ as well as their interindividual variabilities. Given the lack of data on the viral upslope, we also fixed the standard deviation of the random effect associated to R0, denoted ωR0 to 0.5 (which corresponds to assuming a coefficient of variation of ~50% for this parameter).
Modelling remdesivir effect and pharmacological delay
The initiation of remdesivir reduces viral production p by a factor , a parameter comprised between 0 (no activity) and 1 (full viral suppression), leading to:
Active forms of remdesivir have a long mean terminal elimination half-life (t½) of about 24 h, and in non-human primates reaches steady-state concentrations at day 4.23 Therefore, we assumed that treatment was only effective after a certain delay after treatment initiation τ, and we considered different possible values for τ, ranging from τ = 0 (model M0) to τ = 5 (model M5).
We used a model averaging approach24 to account for the uncertainty in τ. Thus, the median parameter estimates and their 95% CIs were obtained by sampling in the mixture of the parameter estimate asymptotic distribution of each model, with weights of model Mi calculated as follows:
where AICi is the Akaike Information Criterion (AIC) of model Mi. We computed the model averaged likelihood using weights Mi and tested the significance of the effect of remdesivir in both models Mi and model averaging using Likelihood Ratio Test (LRT).25
To quantify and visualize the effect of remdesivir on the time to viral clearance, 5000 individual profiles were sampled for each candidate model Mi using the population parameters estimated in Table S1, and each potential trajectory was selected with a probability wi. Times of treatment initiation were sampled from the fitted Gamma distribution of observed times of randomization in the population. For each simulated individual, we calculated the AUC of viral load and time to reach viral clearance, and computed the cumulated incidence of viral clearance. The same individual parameters were used assuming a null treatment efficacy , to simulate 5000 untreated individuals and compute the difference between a treated individual and its own control. Finally, we evaluated the effects of earlier treatment initiation, assuming a fixed time to treatment initiation of 3, 5, 7 or 9 days after symptom onset.
Sub-analyses according to viral load at admission and time since symptom onset
We used the same modelling strategy in two distinct populations, i.e. in patients in whom treatment was initiated early (≤7 days since symptom onset) and those initiating the treatment >7 days since symptom onset. We chose the cutoff of 7 days from symptom onset, consistent with the initial analysis.15 Similarly, we conducted the same analysis in patients with high or low viral load at admission, taking the cutoff of 3.5 log10 copies/104 cells, which is the threshold level below which the virus cannot be cultured.26
Parameter estimation
Parameters were estimated by maximum likelihood estimation using the stochastic approximation expectation–maximization (SAEM) algorithm implemented in Monolix2020R1 (http://www.lixoft.eu), which provides estimations of the fixed effects and the standard deviation of their random effect (see Supplementary data, available at JAC Online). These estimates were then used to perform model averaging and results were reported as the median and 95% CI of these parameters.
Results
Baseline characteristics and viral load data of analysed population
A total of 832 patients were evaluable for the primary intention-to-treat analysis,15 among whom 684 had at least one nasopharyngeal viral load available (Figure 1 and Figure S1). Two patients randomized in the remdesivir arm who did not receive treatment were excluded, as well as 17 patients who were randomized more than 20 days after onset of symptoms (see Methods), leaving a total of 665 patients (SoC alone, N = 329; SoC + remdesivir, N = 336) (Table 1; Figures S1 and S2). Patients were mostly male (N = 457, 68.7%), younger than 65 years (N = 349, 52.5%) and randomized more than 7 days after symptom onset (N = 455, 68.4%). The median viral load at randomization was 3.2 log10 copies/104 cells. Overall, 15% (61/420) of patients had undetectable viral load at symptom onset; this proportion was equal to 18% (49/272) in patients randomized >7 days after symptom onset, and it was equal to 8% (12/148) in patients randomized ≤7 days after symptom onset.
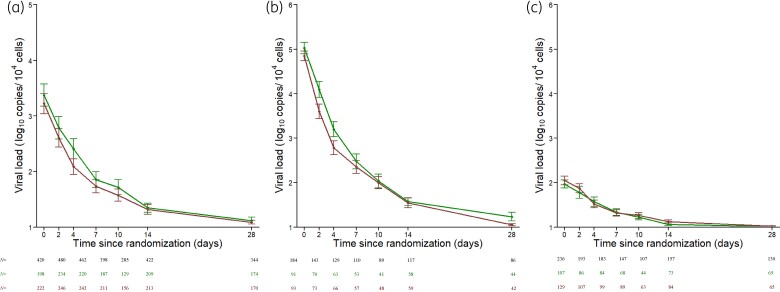
Nasopharyngeal normalized viral load data in 665 patients from DisCoVeRy trial analysed in the present study. (a) SARS-CoV-2 nasopharyngeal viral load according to the time since randomization. (b and c) SARS-CoV-2 nasopharyngeal viral load according to the time since randomization in patients (N = 184) with ≥3.5 log10 copies/104 cells (b) and patients (N = 236) with <3.5 log10 copies/104 cells (c). Data are presented as means (95% CI). Red lines, patients receiving remdesivir + SoC; green lines, patients receiving SoC only. Tables below show the number of samples available at each time.
Table 1.
Baseline characteristics of the analysed population at randomization
Characteristics | Standard of care (N = 329) | Standard of care + remdesivir (N = 336) |
---|---|---|
Male gender | 222 (67.5%) | 235 (69.9%) |
Age (years) | 64 (53–72) | 63 (55–73) |
Age ≥65 years | 160 (48.6%) | 156 (46.4%) |
Time from symptom onset to hospitalization (days) | 9 (7–11) | 9 (7–11) |
Patients admitted within 7 days of symptom onset | 109 (33%) | 101 (30%) |
Duration of hospital stay (days) | 12 (8–24) | 13 (10–25) |
Deaths | 30 (9%) | 30 (9%) |
Normalized viral load at randomization (log10 copies/104 cells)a | 3.2 (1.9–4.5) | 3.2 (1.8–4.5) |
Number of viral samples under LOQ at randomization (% of available samples) | 24 (12%) | 37 (17%) |
Results shown are median (IQR) or n (%) depending on the data type.
Viral dynamics parameters
We used a target-cell limited model with an effect of age on the loss rate of infected cells, δ, (see Methods) which was estimated to 0.88 days−1 (95% CI: 0.80–0.96) in individuals aged <65 years and 0.75 days−1 (95% CI: 0.67–0.84) in those ≥65 years, respectively (P value <10−3; Table 2). R0 was estimated at 10.6 (95% CI: 8.53–12.7; Table 2). The viral production p was estimated at 1.20×106 viruses per day (95% CI: 0.66 × 106 – 1.7 × 106), leading to a burst size of infectious viruses, given by p × μ/δ of 136 and 160 in individuals aged <65 years and those aged ≥65 years, respectively.
Table 2.
Parameter distribution using model averaging
Parameter estimates, median (95% CI) | ||
---|---|---|
Parameter | Fixed effects | SD of the random effect ω |
R0 | 10.60 (8.53–12.68) | 0.50 |
δ<65 (days−1) | 0.88 (0.80–0.96) | 0.46 (0.41–0.51) |
δ≥65 (days−1) | 0.75 (0.67–0.84) | |
p (106 virus cell−1 days−1)a | 1.20 (0.66–1.72) | 0.38 (0.14–0.63) |
![]() | 52 (35–69) | 0.77 (0.18–1.37) |
σ (log10 RNA copies/104 cells) | 1.14 (1.09–1.19) | – |
R0, basic reproductive number; δ, loss rate of infected cells; p, rate of viral production; , remdesivir effect; σ, residual variability.
Estimation of remdesivir effect on viral dynamics
Remdesivir effect, , was estimated for different putative values of the pharmacological delay ranging from 0 to 5 days (see Methods). Using model averaging, we estimated remdesivir to decrease the viral production p by a factor of 2 (
= 52%, 95% CI: 35%–69%; Table 2) and this effect was statistically significant in model averaging (P value = 0.0031). The antiviral effect of remdesivir,
, was statistically significant for τ = 0 to τ = 3, with values equal to 49%, 50%, 53% and 52%, respectively (P value = 0.020, 0.033, 0.0026, 0.0024, respectively, Figure 2; Table S1).
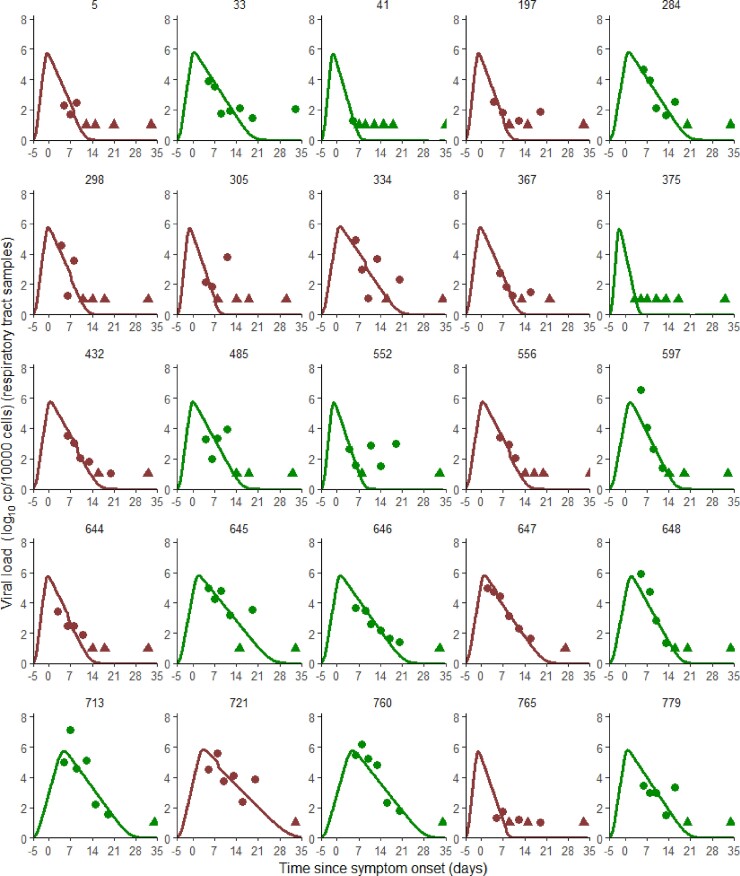
Model-based individual fits for τ = 3. Patients represented here are the patients with seven data points collected within one week of symptom onset. Circles represent detectable viral load, triangles represent data below the limit of quantification. Results obtained by using the individual predictions of the model τ = 3. Red lines and symbols, patients receiving remdesivir + SoC; green lines and symbols, patients receiving SoC only.
To get a sense of the effect size, we simulated 5000 in silico virological profiles using the estimated parameter distributions and we calculated the exact time to viral clearance for each simulated individual. Remdesivir shortened the time to viral clearance by 0.7 days (IQR: 0.0–1.3), with a median time to viral clearance of 14.5 days (IQR: 10.4–20.2 days) and 15.3 days since symptom onset (IQR: 10.6–21.5 days) in treated and untreated individuals, respectively (Figure 3a and andb).b). Because patients older than 65 years had a slower clearance of infected cells (P value <10−3), they cleared virus less rapidly (15.6 versus 16.6 days in treated and untreated individuals, respectively, for patients ≥65 years and 13.3 versus 14.1 days in treated and untreated individuals, respectively, for patients <65 years). In terms of time since randomization, remdesivir led to a time of viral clearance also reduced by 1 day, with a median time equal to 5.5 days (IQR: 1.0–12.0 days) and 6.5 days (IQR: 1.0–13.0 days) in treated and untreated individuals, respectively (Figure S3a and c), with initial undetectability at time of randomization slightly overestimated as compared with the observations (Table 1). Overall, the maximum difference in median viral loads between treated and untreated individuals was obtained at day 11.5 post symptom onset, and equal to 0.24 log10 copies/104 cells (IQR: 0.06–0.39), corresponding to a 2-fold reduction in viral load levels (Figure 3a).
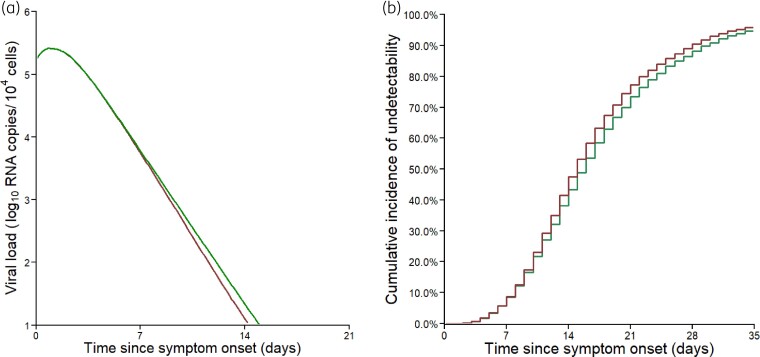
Viral dynamics predicted by the model. (a) Median predicted nasopharyngeal viral dynamics according to the time since symptom onset. (b) Cumulative incidence of the predicted time to viral clearance. Red line, patients receiving remdesivir + SoC; green lines, patients receiving SoC only. Results obtained by sampling 5000 individuals in the estimated parameter distributions.
We repeated the same estimation procedure in subpopulations stratified by the delay since symptom onset (Figure S4) and by the viral load at admission. In patients initiating treatment in the first 7 days following symptom onset, remdesivir effect was larger than 0 only for τ = 3 (=36%; P value = 0.041, Table S2a), but it was not statistically significant by model averaging approach (P value = 0.07, Table S2b). In patients initiating treatment >7 days after symptom onset, remdesivir effect was no greater than 0 for any of the models considered (Tables S3a and S3b). In patients with a high viral load at admission (see Methods, N = 184, median time of admission = 8 days since symptom onset, IQR: 6–10, Figure 1b), remdesivir reduced viral production by 80% (95% CI: 65%–96%), i.e. a reduction by 5-fold compared with SoC, and this effect was statistically significant in model averaging (P value <10−5, see Methods, Table S4a). In line with the analysis on the whole population, remdesivir effect was statistically significant for values of τ ranging from 0 to 3 days after treatment initiation, with values equal to 80%, 82%, 50% and 55%, respectively (P value <10−3 for all values of τ, Table S4b). In patients admitted with a low viral load (N = 236, median time of admission = 9 days since symptom onset, IQR: 7–12, Figure 1c), remdesivir effect was lower and was estimated at 49%, with a poor precision of the estimation (95% CI: 0–100, P value = 0.013, Table S5a).
Using parameter distributions estimated in the population with high viral load at randomization, simulations showed that remdesivir led to a time to viral clearance shortened by 2.4 days (IQR: 0.9–4.5, Figure 4). Further, remdesivir led to a maximum difference between median viral loads of treated and untreated patients of 0.7 log10 copies/104 cells, reached at 12 days after symptom onset. Similar effects were obtained when looking at data in terms of time since randomization, with a median time equal to 10.5 days (IQR: 6.5–14.6 days) and 13.5 days (IQR: 10.0–18.0 days) in treated and untreated individuals, respectively (Figure S3b and d).
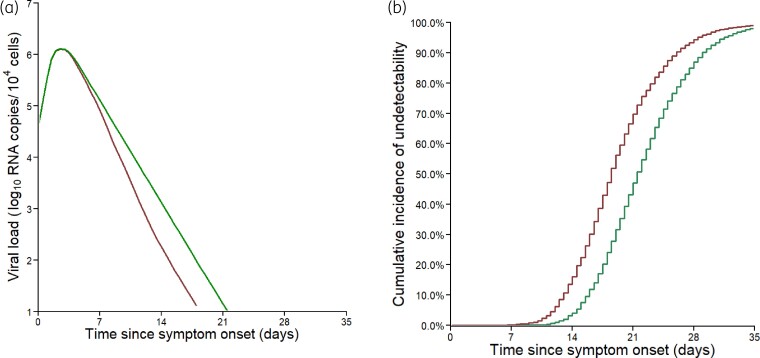
Viral dynamics predicted by the model in the subpopulation of viral load at admission ≥3.5 log10 copies/104 cells. (a) Median predicted nasopharyngeal viral dynamics according to the time since symptom onset. (b) Cumulative incidence of the predicted time to viral clearance. Red lines, patients receiving remdesivir + SoC; green lines patients receiving SoC only. Results obtained by sampling 5000 individuals in the estimated parameter distributions.
Discussion
We found that remdesivir had a statistically significant antiviral efficacy, reducing viral production from infected cells by 52% (95% CI: 35%–69%), which corresponds to a 2-fold reduction in viral production. The impact on viral dynamics remained modest, with an average 0.7 days reduction in the time to viral clearance and large interindividual variability. This explains why less sophisticated approaches did not find any effect of remdesivir on viral dynamics.7,10,15
The overall limited antiviral effect of remdesivir may be due to factors that mitigate a potential effect, in particular the heterogeneity in the time between infection and treatment initiation, as well as variability in viral load levels at the time of treatment initiation. To explore this question, we here relied on a very rich dataset of 665 patients, for which viral loads were centralized and normalized, and we used a model previously developed on a large cohort of hospitalized patients to disentangle the effects of remdesivir from the natural clearance of viral load. To identify factors associated with the effects of remdesivir on viral kinetics, we performed exploratory analyses by stratifying the population on timing of treatment initiation in days since symptom onset and on viral load at randomization, the latter being an exploratory analysis not included in the initial trial protocol. We did not identify a significant effect of remdesivir in patients treated early, consistent with the results from descriptive models.15 This may be due to the fact that symptom onset may not be a very good proxy of active viral replication and/or to the lack of statistical power due to the fact that viral load at admission was missing in about 40% of individuals arriving within 7 days of symptom onset (Table 1). However, remdesivir effect in reducing viral production was larger in patients with a high viral load at admission, leading to a median reduction of 2.4 days (IQR: 0.9–4.5) in the time to viral clearance compared with untreated patients. These results therefore suggest that remdesivir could be more effective in patients with high viral load, at a stage of the infection where viral replication is very active. This hypothesis is consistent with the results of the Recovery trial,5 which identified a significant effect of monoclonal antibody combination REGN-CoV-2 on clinical outcome in hospitalized patients with a seronegative status at baseline, a factor associated with high viral load.2,27 The relationship between treatment effect and viral load was also reported in outpatients receiving monoclonal antibodies within 7 days of symptom onset.2,27 Simulations conducted with our model suggested no dramatic difference in viral shedding when administration is initiated between 3–7 days (Figure S5), but our model did not account for immune mechanisms that could act, in concert with early antiviral treatment, to accelerate viral clearance.22 Consistent with the interpretation that it could be beneficial to administrate remdesivir as early as possible, results from the Pinetree study showed that administration of remdesivir in outpatients within 7 days of symptom onset led to a significant reduction of the risk of hospitalization.3 However there was no effect of remdesivir on the average change in nasopharyngeal viral load, but more detailed analyses taking into account interpatient variability will be needed to estimate the precise effect of remdesivir on viral dynamics.
How does remdesivir antiviral efficacy compare with other antiviral drugs now available? Our estimated effect of 52% in the whole population is below the pharmacodynamic target of 90% that we determined previously to achieve clinical efficacy in hospitalized patients and/or to prevent disease acquisition in prophylactic setting.16,18,28 It is also probably lower than what has been shown in outpatients treated with monoclonal antibodies. For instance, 7 days after treatment initiation, the viral load in ambulatory individuals receiving casirivimab + imdevimab or bamlanivimab + etesevimab was reduced by 5–10-fold compared with patients receiving placebo, as compared to 2-fold in our hospitalized population here.2,29 The lower efficacy of remdesivir could nonetheless be relevant in a combination setting to increase the genetic barrier to resistance due to the emergence of variants of concern.30,31 This could be even more relevant in patients with high viral load, to reduce the risk of mutation emergence in a context where huge quantities of viral progeny are still produced upon treatment initiation.
We acknowledge some important limitations to our study. First a more complete evaluation of remdesivir would involve analysing viral dynamics in the lower respiratory tract, as was done in non-human primates.13,14 In our study, viral loads in lower respiratory tracts were available in a subset of 120 individuals. However, the number of samples was limited and these individuals had very severe disease (Table S6), making it impossible to provide an unbiased and precise estimate for remdesivir. Also, our model did not incorporate any time-dependent effects of the immune response and underestimates the response of the host in clearing the virus. Further, this model predicts that a modest antiviral effect reduces the growth rate of virus and therefore requires more time to deplete all target cells. Consequently, in this basic model treatment may have the paradoxical effect of lowering the viral load but extending the period of shedding and the time to viral clearance.3 This phenomenon explains why our model predicts that nearly 25% of patients would have no benefit of remdesivir in terms of viral clearance. Second, we could not evaluate the association between remdesivir drug concentration and viral decay, which would be important to obtain more definitive evidence on remdesivir antiviral activity. Here, drug concentrations were available for only a limited number of patients (N = 61), and no significant association between drug concentrations and the time to viral clearance (Figure S6) could be found. Moreover, given that symptom onset is often after the peak viral load,18,32 a potential bias in the estimation of early viral dynamic parameters cannot be ruled out (see a discussion on this aspect18). Finally, the use of adjuvant drugs such as corticosteroids or any immunosuppressive treatment that might promote viral replication, as well as the intrinsic immune competency of each treated individual have not been considered.
In conclusion, the use of a within-host model of the infection allowed us to estimate the in vivo antiviral efficacy of remdesivir using the nasopharyngeal swabs of hospitalized patients. Overall, we showed that remdesivir had a modest antiviral activity in reducing viral production, leading to a reduction by 0.7 days of the time to viral clearance. In exploratory analyses, remdesivir had a higher antiviral efficacy in patients with high viral load at randomization (≥3.5 log copies/104 cells), leading to a reduction of time to viral clearance of 2.4 (IQR: 0.9–4.5) days between treated and untreated patients. The limited effect of remdesivir is consistent with the lack of clinical efficacy in hospitalized patients reported by the DisCoVeRy trial.15
Acknowledgements
Members of the DisCoVeRy Study Group
Sponsor: Sandrine Couffin-Cadièrgues, Christelle Delmas, Hélène Esperou (Pôle de Recherche Clinique, Inserm, Paris, France).
Investigators
Austria: Bernd Lamprecht (Kepler Universitätsklinikum Linz, Linz), Michael Joannidis (Medizinische Universität Innsbruck, Innsbruck), Alexander Egle, Richard Greil (Paracelsus Medical University Salzburg, SCRI-CCCI and AGMT). Belgium: Antoine Altdorfer, Vincent Fraipont (Centre Hospitalier Régional de la Citadelle, Liège), Leila Belkhir (Cliniques Universitaires de Saint Luc, Bruxelles), Maya Hites, Gil Verschelden (Hôpital Erasme, Cliniques Universitaires de Bruxelles). France: Violaine Tolsma, David Bougon (Centre Hospitalier Annecy-Genevois); Agathe Delbove, Marie Gousseff (Centre Hospitalier Bretagne-Atlantique, Vannes); Nadia Saidani, Guilhem Wattecamps (Centre Hospitalier Cornouaille, Quimper); Félix Djossou, Loïc Epelboin (Centre Hospitalier de Cayenne Andrée Rosemon); Jean-Philippe Lanoix, Pierre-Alexandre Roger, Claire Andrejak, Yoann Zerbib (Centre Hospitalier Universitaire de Amiens); Kevin Bouiller, Catherine Chirouze, Jean-Christophe Navellou (Centre Hospitalier Universitaire de Besançon); Alexandre Boyer, Charles Cazanave, Alexandre Duvignaud, Didier Gruson, Denis Malvy (Centre Hospitalier Universitaire de Bordeaux); Henry Lessire, Martin Martinot (Hospices Civils de Colmar); Pascal Andreu, Mathieu Blot, Lionel Piroth, Jean Pierre Quenot (Centre Hospitalier Universitaire de Dijon); Olivier Epaulard, Nicolas Terzi (Centre Hospitalier Universitaire de Grenoble-Alpes); Karine Faure, Emmanuel Faure, Julien Poissy, Saad Nseir (Centre Hospitalier Régional Universitaire de Lille); Florence Ader, Laurent Argaud, Tristan Ferry, Thomas Perpoint, Vincent Piriou, Jean-Christophe Richard, Julien Textoris, Florent Valour, Florent Wallet (Hospices Civils de Lyon); André Cabié, Jean- Marie Turmel, Cyrille Chabartier (Centre Hospitalier Universitaire de Martinique, Fort-de-France); Rostane Gaci, Céline Robert (Centre Hospitalier Régional de Metz-Thionville); Alain Makinson, Vincent Le Moing, Kada Klouche (Centre Hospitalier Universitaire de Montpellier); Olivier Hinschberger, Joy Mootien (Centre Hospitalier de Mulhouse Sud-Alsace); Sébastien Gibot, François Goehringer, Antoine Kimmoun, Benjamin Lefevre (Centre Hospitalier Régional Universitaire de Nancy); David Boutoille, Emmanuel Canet, Benjamin Gaborit, Paul Le Turnier, François Raffi, Jean Reignier (Centre Hospitalier Universitaire de Nantes); Johan Courjon, Jean Dellamonica, Sylvie Leroy, Charles-Hugo Marquette (Centre Hospitalier Universitaire de Nice), Paul Loubet, Claire Roger, Albert Sotto (Centre Hospitalier Universitaire de Nîmes); Cédric Bruel, Benoît Pilmis (Groupe Hospitalier de Paris Saint-Joseph); Guillaume Geri, Elisabeth Rouveix-Nordon (Hôpital Ambroise Paré, Assistance Publique - Hôpitaux de Paris); Olivier Bouchaud (Hôpital Avicenne, Assistance Publique - Hôpitaux de Paris); Samy Figueiredo, Stéphane Jaureguiberry, Xavier Monnet (Hôpital Bicêtre, Assistance Publique - Hôpitaux de Paris); Lila Bouadma, François-Xavier Lescure, Nathan Peiffer-Smadja, Jean-François Timsit, Yazdan Yazdanpanah (Hôpital Bichat - Claude Bernard, Assistance Publique - Hôpitaux de Paris); Solen Kernéis, Marie Lachâtre, Odile Launay, Jean-Paul Mira (Hôpital Cochin, Assistance Publique - Hôpitaux de Paris); Julien Mayaux, Valérie Pourcher (Hôpital de la Pitié- Salpêtrière, Assistance Publique - Hôpitaux de Paris); Jérôme Aboab, Flora Crockett, Naomi Sayre (Hôpital Delafontaine, Saint-Denis), Clément Dubost, Cécile Ficko (Hôpital d'Instruction des Armées Bégin, Saint Mandé); David Lebeaux (Hôpital Européen Georges-Pompidou, Assistance Publique - Hôpitaux de Paris); Sébastien Gallien, Armand Mekontso-Dessap (Hôpital Henri-Mondor, Assistance Publique - Hôpitaux de Paris); Jérôme Le Pavec, Francois Stefan (Hôpital Marie Lannelongue, Le Plessis-Robinson); Hafid Ait-Oufella, Karine Lacombe (Hôpital Saint-Antoine, Assistance Publique - Hôpitaux de Paris); Jean-Michel Molina (Hôpital Saint-Louis, Assistance Publique - Hôpitaux de Paris); Murielle Fartoukh, Gilles Pialoux (Hôpital Tenon, Assistance Publique - Hôpitaux de Paris); Firouzé Bani-Sadr, Bruno Mourvillier (Centre Hospitalier Universitaire de Reims); François Benezit, Fabrice Laine, Bruno Laviolle, Yves Le Tulzo, Matthieu Revest (Centre Hospitalier Universitaire de Rennes); Elisabeth Botelho-Nevers, Amandine Gagneux-Brunon, Guillaume Thiery (Centre Hospitalier Universitaire de Saint-Étienne); Raphaël Clere-Jehl, François Danion, Yves Hansmann, Ferhat Meziani, Walid Oulehri, Yvon Ruch, Charles Tacquard (Centre Hospitalier Universitaire de Strasbourg); Fanny Bounes-Vardon, Guillaume Martin-Blondel, Marlène Murris-Espin, Béatrice Riu-Poulenc (Centre Hospitalier Universitaire de Toulouse); Vanessa Jean-Michel, Eric Senneville (Centre Hospitalier de Tourcoing); Louis Bernard, Denis Garot (Centre Hospitalier Universitaire de Tours). Luxembourg: Jean Reuter, Thérèse Staub (Centre Hospitalier de Luxembourg); Marc Berna (Hôpitaux Robert Schuman). Portugal: Sandra Braz, Joao Miguel Ferreira Ribeiro (Centro Hospital Universitário de Lisboa Norte, Hospital de Santa Maria); José-Artur Paiva, Roberto Roncon-Albuquerque (Centro Hospitalar Universitário São João de Porto).
Laboratory support: Maude Bouscambert-Duchamp, Alexandre Gaymard, Benjamin Leveau, Bruno Lina (Hospices Civils de Lyon); Minh-Patrick Lê, Gilles Peytavin, Sarah Tubiana (Hôpital Bichat - Claude Bernard, Assistance Publique - Hôpitaux de Paris).
Trial management: Aline Dechanet (Hôpital Bichat - Claude Bernard, Assistance Publique - Hôpitaux de Paris); Juliette Saillard (ANRS-MIE), Marina Dumousseaux, Assia Ferrane (Pôle de Recherche Clinique, Inserm), Claire Fougerou-Leurent (Centre Hospitalier Universitaire de Rennes); Marion Noret (Réseau national de recherche clinique en infectiologie).
Pharmacovigilance: Alpha Diallo, Noémie Mercier, Vida Terzić (ANRS-MIE).
Statistics and data management: Drifa Belhadi, Charles Burdet, Jérémie Guedj, France Mentré, Priyanka Velou (Hôpital Bichat - Claude Bernard, Assistance Publique - Hôpitaux de Paris); Dominique Costagliola (Institut Pierre-Louis d'Épidémiologie et de Santé Publique).
Funding
This work was supported by: European Commission (EU-Response, Grant 101015736); Programme Hospitalier de Recherche Clinique (PHRC-20-0351, Ministry of Health); Domaine d’intérêt majeur One Health Île-de-France (R20117HD); REACTing, a French multi-disciplinary collaborative network working on emerging infectious diseases; Fonds Erasme-COVID-Université Libre de Bruxelles; Belgian Health Care Knowledge Centre; Austrian Group Medical Tumor; European Regional Development Fund; Portugal Ministry of Health; Portugal Agency for Clinical Research and Biomedical Innovation.
Supplementary data
Additional Methods, Tables S1 to S6 and Figures S1 to S6 are available as Supplementary data at JAC Online.
References
Full text links
Read article at publisher's site: https://doi.org/10.1093/jac/dkac048
Read article for free, from open access legal sources, via Unpaywall:
https://academic.oup.com/jac/article-pdf/77/5/1404/43480506/dkac048.pdf
Citations & impact
Impact metrics
Citations of article over time
Alternative metrics

Discover the attention surrounding your research
https://www.altmetric.com/details/123919080
Article citations
Antiviral effect of Evusheld in COVID-19 hospitalized patients infected with pre-Omicron or Omicron variants: a modelling analysis of the randomized DisCoVeRy trial.
J Antimicrob Chemother, 79(11):2887-2895, 01 Nov 2024
Cited by: 0 articles | PMID: 39236218 | PMCID: PMC11531825
SARS-CoV-2 viral load is linked to remdesivir efficacy in severe Covid-19 admitted to intensive care.
Sci Rep, 14(1):20825, 06 Sep 2024
Cited by: 0 articles | PMID: 39242658 | PMCID: PMC11379941
SARS-CoV-2 RNA and Nucleocapsid Antigen Are Blood Biomarkers Associated With Severe Disease Outcomes That Improve in Response to Remdesivir.
J Infect Dis, 230(3):624-634, 01 Sep 2024
Cited by: 2 articles | PMID: 38657001 | PMCID: PMC11420797
COVID-19 therapeutics.
Clin Microbiol Rev, 37(2):e0011923, 21 May 2024
Cited by: 3 articles | PMID: 38771027
Review
Modeling the emergence of viral resistance for SARS-CoV-2 during treatment with an anti-spike monoclonal antibody.
PLoS Pathog, 20(4):e1011680, 18 Apr 2024
Cited by: 1 article | PMID: 38635853 | PMCID: PMC11060554
Go to all (21) article citations
Data
Data behind the article
This data has been text mined from the article, or deposited into data resources.
Clinical Trials
- (1 citation) ClinicalTrials.gov - NCT04315948
European Clinical Trials
- (2 citations) EU Clinical Trials Register - 2020-000936-23
Similar Articles
To arrive at the top five similar articles we use a word-weighted algorithm to compare words from the Title and Abstract of each citation.
Evaluation of the Effects of Remdesivir and Hydroxychloroquine on Viral Clearance in COVID-19 : A Randomized Trial.
Ann Intern Med, 174(9):1261-1269, 13 Jul 2021
Cited by: 66 articles | PMID: 34251903 | PMCID: PMC8279143
Cardiac Adverse Events and Remdesivir in Hospitalized Patients With COVID-19: A Post Hoc Safety Analysis of the Randomized DisCoVeRy Trial.
Clin Infect Dis, 79(2):382-391, 01 Aug 2024
Cited by: 0 articles | PMID: 38552208 | PMCID: PMC11327784
A real-life setting evaluation of the effect of remdesivir on viral load in COVID-19 patients admitted to a large tertiary centre in Israel.
Clin Microbiol Infect, 27(6):917.e1-917.e4, 09 Mar 2021
Cited by: 20 articles | PMID: 33705849 | PMCID: PMC7939997
Remdesivir: A Closer Look at Its Effect in COVID-19 Pandemic.
Pharmacology, 106(9-10):462-468, 10 Aug 2021
Cited by: 10 articles | PMID: 34515227 | PMCID: PMC8450841
Review Free full text in Europe PMC
Funding
Funders who supported this work.
Austrian Group Medical Tumor
Belgian Health Care Knowledge Centre
European Commission (3)
Grant ID: R20117HD
EU-RESPONSE European Research and Preparedness Network for Pandemics and Emerging Infectious Diseases
INSTITUT NATIONAL DE LA SANTE ET DE LA RECHERCHE MEDICALE France, INSTITUT NATIONAL DE LA SANTE ET DE LA RECHERCHE MEDICALE France
Grant ID: 101015736
Grant ID: PHRC-20-0351