Abstract
Background
Severe acute respiratory syndrome (SARS) is an emerging infectious disease caused by a new coronavirus strain, SARS-CoV. Specific proteomic patterns might be present in serum in response to the infection and could be useful for early detection of the disease.Methods
Using surface-enhanced laser desorption/ionization (SELDI) ProteinChip technology, we profiled and compared serum proteins of 39 patients with early-stage SARS infection and 39 non-SARS patients who were suspected cases during the SARS outbreak period. Proteomic patterns associated with SARS were identified by bioinformatic and biostatistical analyses. Features of interest were then purified and identified by tandem mass spectrometry.Results
Twenty proteomic features were significantly different between the 2 groups. Fifteen were increased in the SARS group, and 5 were decreased. Their concentrations were correlated with 2 or more clinical and/or biochemical variables. Two were correlated with the SARS-CoV viral load. Hierarchical clustering analysis showed that a majority of the SARS patients (95%) had similar serum proteomic profiles and identified 2 subgroups with poor prognosis. ROC curve analysis identified individual features as potential biomarkers for SARS diagnosis (areas under ROC curves, 0.733-0.995). ROC curve areas were largest for an N-terminal fragment of complement C3c alpha chain (m/z 28 119) and an internal fragment of fibrinogen alpha-E chain (m/z 5908). Immunoglobulin kappa light chain (m/z 24 505) positively correlated with viral load.Conclusions
Specific proteomic fingerprints in the sera of adult SARS patients could be used to identify SARS cases early during onset with high specificity and sensitivity.Free full text

Serum Proteomic Fingerprints of Adult Patients with Severe Acute Respiratory Syndrome
Abstract
Background: Severe acute respiratory syndrome (SARS) is an emerging infectious disease caused by a new coronavirus strain, SARS-CoV. Specific proteomic patterns might be present in serum in response to the infection and could be useful for early detection of the disease.
Methods: Using surface-enhanced laser desorption/ionization (SELDI) ProteinChip technology, we profiled and compared serum proteins of 39 patients with early-stage SARS infection and 39 non-SARS patients who were suspected cases during the SARS outbreak period. Proteomic patterns associated with SARS were identified by bioinformatic and biostatistical analyses. Features of interest were then purified and identified by tandem mass spectrometry.
Results: Twenty proteomic features were significantly different between the 2 groups. Fifteen were increased in the SARS group, and 5 were decreased. Their concentrations were correlated with 2 or more clinical and/or biochemical variables. Two were correlated with the SARS-CoV viral load. Hierarchical clustering analysis showed that a majority of the SARS patients (95%) had similar serum proteomic profiles and identified 2 subgroups with poor prognosis. ROC curve analysis identified individual features as potential biomarkers for SARS diagnosis (areas under ROC curves, 0.733–0.995). ROC curve areas were largest for an N-terminal fragment of complement C3c α chain (m/z 28 119) and an internal fragment of fibrinogen α-E chain (m/z 5908). Immunoglobulin κ light chain (m/z 24 505) positively correlated with viral load.
Conclusions: Specific proteomic fingerprints in the sera of adult SARS patients could be used to identify SARS cases early during onset with high specificity and sensitivity.
The outbreak of severe acute respiratory syndrome (SARS) 1 has affected 32 countries and regions. SARS has infected more than 8000 people and killed more than 700 (1). Since the outbreak, many efforts have been made to understand the nature of the disease. The causative agent for SARS was identified to be a human SARS coronavirus (SARS-CoV) (2)(3)(4). High fever, dry cough, myalgia, and lymphopenia were the most distinctive characteristic symptoms seen in patients with SARS. The SARS-CoV has a RNA genome of 30 Kb encoding the replicase, spike glycoprotein, envelop protein, membrane protein, and nucleocapsid (2)(3)(4). Early diagnosis is necessary to prevent the spread of the disease through isolation of infected patients. Diagnostic tests to detect the presence of either SARS antibody or SARS-CoV RNA have been developed (5)(6). Quantitative real-time viral RNA reverse transcription-PCR assays allow the detection of SARS-CoV within the first week of illness, whereas antibody tests are reliable around 20 days after disease onset (5)(6).
Recently, advances in proteomics have provided new strategies to identify biomarkers and therapeutic targets and to study the pathology of diseases. Surface-enhanced laser desorption/ionization (SELDI) ProteinChip technology is a proteomic tool that has been applied to the discovery of diagnostic proteomic fingerprints for various diseases, including cancer and infectious diseases (7)(8)(9). Recent studies have shown that this technology could also be used to identify potential biomarkers for early diagnosis of SARS (7)(10)(11). In these studies, the control cases were either healthy persons or persons with non-SARS viral infections. Unfortunately, the degree of similarity of the symptoms between the SARS and control groups and the time point of blood collection were not considered in these studies. From the perspective of infectious disease diagnosis, one is not trying to differentiate healthy persons from infected patients, but rather is trying to identify the disease causing the symptoms in patients presenting with similar symptoms (12).
Bearing in mind the above issues, in the present study we attempted to profile and compare the serum proteomes between SARS patients and non-SARS patients. The non-SARS patients were those who had symptoms similar to those in SARS patients. They were admitted to the same hospital as the SARS patients and were later shown to be negative for SARS-CoV infection. Furthermore, for both SARS and non-SARS patients, sera were collected within 1 week after fever onset.
Materials and Methods
patient materials
The SARS group included 13 males and 26 females (mean age, 42 years; range, 21–88 years); the non-SARS group included 18 males and 21 females (mean age, 44.4 years; range, 20–88 years). The serum samples from both SARS and non-SARS groups represented the first time point after hospitalization (3–7 days after onset of fever); no medications, including steroids, ribavirin, traditional Chinese medicine, or intravenous immunoglobulins, had been given to the above patients before blood collection. All of the SARS cases were positive in the anti-SARS-CoV IgG antibody serology test. Anti-SARS-CoV IgG antibody was detected by an indirect immunofluorescence assay using Vero cells that were infected with a strain of CoV (GenBank accession no. AY278554) isolated from a patient with SARS. Both the sensitivity and the specificity of this serology test for SARS were 100%.
The serum SARS-CoV RNA test was performed on 31 patients, and 22 of them (71%) showed detectable SARS-CoV RNA in their serum. For the control group, the 39 non-SARS patients (21 with bacterial pneumonia, 1 with aspiratory pneumonia, 2 with acute pulmonary edema, 2 with upper respiratory tract infection, 2 with influenza, 1 with acute bronchitis, 1 with bronchiectasis, 1 with secondary lung cancer, 1 with gastrointestinal bleeding, 1 with acute cholangitis, 1 with infectious mononucleosis, 1 with viral gastroenteritis, 1 with liver abscess, 1 with peritonitis and end stage renal failure, 1 with cellulites, and 1 with thalamic hemorrhage) were those who had symptoms similar to those for the SARS patients. They were admitted to the same hospital as the SARS patients and were later shown to be negative for SARS-CoV antibody by a serology test after at least 6 weeks of the onset of symptoms. All biochemical information was collected from the same blood samples. All samples were stored as aliquots at −70 °C until ProteinChip array profiling analysis was performed.
serum proteomic profiling
In the SELDI ProteinChip analysis, the serum samples from the diseased and control groups were randomized, and the investigator was blinded to their identity. The SELDI ProteinChip analysis was performed as described previously (8)(10)(13). For each set of binding conditions, samples were analyzed in duplicate. Serum samples (2 μL) were denatured by addition of 4 μL of U9 buffer (9 mol/L urea, 20 g/L CHAPS, 50 mmol/L Tris-HCl, pH 9.0) and incubated on ice for 20 min. Denatured serum samples were diluted with 34 μL of T4 (50 mmol/L sodium acetate, 1 mL/L Triton X-100, pH 4.0) or T9 (10 mmol/L Tris, 1 mL/L Triton X-100, pH 9.0) binding buffer, respectively, to give a final dilution of 20-fold. CM10 ProteinChip arrays (Ciphergen Biosystems) were used in this study. After dilution, 5 μL of the diluted sample was applied to a preequilibrated ProteinChip array in duplicate in a bioprocessor and incubated with shaking for 90 min at room temperature. After incubation, the ProteinChip arrays were washed 5 times with the same binding buffer and rinsed twice with deionized water. After air-drying, sinapinic acid matrix in 500 mL/L acetonitrile–5 mL/L trifluoroacetic acid was added to each array. The ProteinChip arrays were read on the ProteinChip PBS II reader of a ProteinChip Biomarker System (Ciphergen Biosystems) to determine the masses and intensities of all peaks over the range m/z 1000 to 250 000. For each box (12 pieces containing 96 assay spots) of ProteinChip arrays, 1 array (8 assay spots) was used for calibration. Mixtures of peptide/protein calibrators [angiotensin (m/z 1296.51), corticotropin (clip 1–17; m/z 2093.46), corticotropin (clip 18–39; m/z 2465.72), doubly charged horse apomyoglobin (m/z 8475.8), Escherichia coli thioredoxin (m/z 11 673.5), horse apomyoglobin (m/z 16 951.6), bovine serum albumin (m/z 66 430), and bovine serum albumin dimer (m/z 132 861); Applied Biosystems Ltd.] were added to all 8 assay spots of the calibration array. Intensities of peaks between m/z 1000 and 20 000 were obtained at a laser setting of 183 and a optimized range of m/z 1000 to 20 000; intensities of peaks between m/z 20 000 and 250 000 were obtained at a laser setting of 188 and a optimized range of m/z 20 000 to 150 000. The spectra were smoothed, baseline-subtracted, and externally calibrated. The common peaks among the SELDI mass spectra were identified and quantified by use of Biomarker Wizard software (Ciphergen Biosystems). The peak intensities were normalized with the total ion current and, subsequently, with the total peak intensities. Before data mining, the normalized peak intensities of the duplicate measurements were averaged and log2-transformed. The intraassay CVs of the normalized intensities of various peaks were <15%.
bioinformatic analysis
To identify proteomic features associated only with disease, we used 2 criteria: (a) the normalized peak intensities had to be significantly higher/lower in SARS patients than in non-SARS individuals; and (b) the normalized peak intensities had to correlate with 2 or more clinical/biochemical variables, indicating their biological meaningfulness.
The Significance Analysis of Microarray (SAM) algorithm (Stanford University) (8)(10)(13) was used to identify proteomic features with concentrations significantly different between the SARS and non-SARS patient groups. During SAM analysis, “two classed, unpaired data” were selected as the data type, and 5000 permutations were performed. The false significant discovery rate was set to zero to avoid the identification of falsely significant proteomic features caused by multiple comparisons. Correlations between the differential proteomic features and various clinical/biochemical features were examined by the Spearman rank-order correlation test.
The significant differential proteomic features correlated with various clinical/biochemical correlations were subjected to two-way hierarchical clustering analysis, as described previously (8). Before clustering analysis, the data of each proteomic feature were subjected to zero-mean unit-variance normalization. The processed proteomic data and the serum samples were subjected to two-way hierarchical clustering analysis by the Cluster and TreeView (14). Spearman rank correlation was used to calculate the distance, and complete linkage clustering was performed.
protein purification
For protein identification, proteins corresponding to the SEDLI peaks were purified by cation-exchange chromatography with the use of CM10 ceramic beads (BioSepra) under binding conditions similar to those for CM10 ProteinChip arrays. Briefly, pooled serum samples were first denatured with U9 buffer and diluted with T4 or T9 sample binding buffer, respectively. After incubation for 120 min and subsequent washing, the bound proteins were eluted from the CM10 ceramic beads with 1 mol/L NaCl solution. C18 ZipTips were used to desalt the eluted proteins according to the manufacturer’s instructions (Millipore). The desalted protein preparations were spotted on the gold-coated ProteinChip arrays and examined with the ProteinChip reader to confirm that the purified proteins had the same masses as the targeted SEDLI proteomic features. After confirmation, the purified proteins were resolved by 2-dimensional gel electrophoresis in the absence of reducing agents. Proteins on gels were visualized by either colloidal blue (Invitrogentrade;) or silver staining (GE Healthcare). The gel images were then digitized with a densitometer and analyzed by the PDQuest gel analysis software (Ver. 7.3.0; Bio-Rad). Protein spots with masses matched with the differential proteomic features were excised and subjected to mass spectrometric (MS) analysis.
protein identification by tandem ms
Protein spots of interests were removed from the gel and subjected to trypsin digestion as described previously (15). The trypsin digests were then extracted and subjected to tandem MS (MS/MS) analysis in the ABI 4700 system (Applied Biosystems). Trypsin peaks, possible keratin contamination, and matrix cluster peaks were excluded from subsequent collision-induced dissociation. The MS/MS spectra were then processed with Data Explorer software (Ver. 4.4; Applied Biosystems). The spectra were subjected to gaussian smoothing with a filter width of 5 points, and the baselines were corrected with default settings. Peaks were detected based on a signal-to-noise threshold of 15. The fragment masses and intensities of each MS/MS mass spectrum were subjected to an online Mascot MS/MS ion search (http://www.matrixscience.com/) to obtain the protein identities. For the search parameters, the maximum allowed missed cleavage in trypsin digestion was 1; partial oxidation of methionine, phosphorylation of serine/threonine/tyrosine, and iodoacetamide modification of cysteine residues were selected. The error tolerance values of the parent peptides and the MS/MS ion masses were 0.1 and 0.3 Da, respectively. A protein identification result was considered significant when the MS/MS ion profile matched a known protein in the NCBInr (2005/06/01) database with a P value <0.05. For each identified protein, an accession number in the UniProt protein database (Ver. 48.0) was reported when available.
Results
differential serum proteomic features in sars patients
More than 800 common proteomic features were identified and compared between the SARS and non-SARS patient groups. Using SAM at a median false discovery rate of zero, we identified 107 proteomic features that were significantly differentially expressed between the 2 groups. Fifty-two were increased and 55 were decreased in the SARS group.
To avoid identification of falsely significant proteomic features caused by systematic bias, we considered only 20 differential proteomic features (Fig. 11 and Table 11 ) that were significantly correlated with at least 2 biochemical/clinical variables as SARS-specific (Table 22 ). These potential biomarkers were found to be significantly associated with SARS-CoV viral load (2 correlated with SARS-CoV RNA), acute-phase reaction [10 correlated with C-reactive protein (CRP)], lung damage [12 correlated with lactate dehydrogenase (LD)], liver function (15 correlated with albumin and/or total protein), immune response (11 correlated with neutrophil count; 3 correlated with total leukocyte count), and age (3 correlated), respectively. Thus, these biomarkers could reflect different physiologic conditions of the body after infection with SARS-CoV.
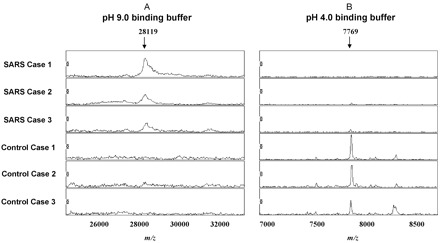
Two representative mass spectra of serum samples in the SARS and non-SARS groups, respectively.
(A), proteomic feature m/z 28 119 was specific to SARS cases, whereas proteomic feature m/z 7769 (B) was specific to the non-SARS control group.
Table 1.
Summary of the proteomic features differentially expressed between the SARS and non-SARS control groups.
Mean (minimum–maximum) m/z | Area under ROC curve1 (95% confidence interval) | Mean (SD) intensity of proteomic feature relative to total sum of proteomic features, % | ||
---|---|---|---|---|
Non-SARS control | SARS cases | |||
4154 (4149–4159) | 0.867 (0.781–0.953) | 0.145 (0.102) | 0.534 (0.586) | |
4303 (4300–4309) | 0.914 (0.842–0.985) | 0.110 (0.115) | 0.397 (0.204) | |
4469 (4466–4475) | 0.898 (0.828–0.968) | 0.230 (0.130) | 0.664 (0.359) | |
4482 (4476–4489) | 0.819 (0.723–0.915) | 0.102 (0.079) | 0.245 (0.184) | |
4680 (4676–4683) | 0.802 (0.702–0.903)2 | 0.153 (0.075) | 0.087 (0.034) | |
5908 (5903–5915) | 0.995 (0.985–0.996)2 | 1.895 (0.716) | 0.255 (0.244) | |
6634 (6626–6643) | 0.745 (0.636–0.854)2 | 0.276 (0.144) | 0.167 (0.091) | |
7769 (7761–7776) | 0.733 (0.621–0.846)2 | 0.058 (0.054) | 0.027 (0.019) | |
8606 (8600–8612) | 0.923 (0.859–0.988) | 0.239 (0.298) | 1.054 (0.570) | |
8619 (8613–8623) | 0.923 (0.861–0.985) | 0.148 (0.178) | 0.670 (0.424) | |
8635 (8630–8641) | 0.915 (0.851–0.979) | 0.074 (0.072) | 0.331 (0.227) | |
8814 (8808–8820) | 0.850 (0.764–0.935) | 0.013 (0.020) | 0.060 (0.051) | |
8831 (8827–8836) | 0.835 (0.741–0.929) | 0.015 (0.017) | 0.049 (0.036) | |
8865 (8856–8874) | 0.762 (0.656–0.868) | 0.100 (0.071) | 0.170 (0.076) | |
8937 (8930–8946) | 0.901 (0.833–0.970) | 0.604 (0.335) | 1.612 (0.755) | |
8956 (8946–8968) | 0.925 (0.868–0.982) | 0.277 (0.168) | 0.869 (0.455) | |
17 878 (17 860–17 902) | 0.846 (0.759–0.934) | 0.005 (0.004) | 0.014 (0.009) | |
24 505 (24 465–24 553) | 0.733 (0.621–0.845) | 1.598 (0.828) | 2.302 (0.988) | |
28 119 (28 064–28 171) | 0.987 (0.966–1.007) | 0.787 (0.496) | 3.904 (1.370) | |
88 637 (88 474–88 736) | 0.851 (0.766–0.936)b | 1.300 (0.316) | 0.920 (0.207) |
Table 2.
Summary of the differentially expressed proteomic features and their correlations to various clinical/biochemical features.
Feature, m/z | Concentration in SARS vs non-SARS control | Clinical/Biochemical features1 |
---|---|---|
4154 | Higher | Age (r = 0.344; P = 0.035); neutrophil count (r = 0.395; P = 0.016) |
4303 | Higher | Neutrophil count (r = 0.045; P = 0.005); LD (r = 0.495; P = 0.003); total protein (r = −0.325; P = 0.046); albumin (r = −0.332; P = 0.042) |
4469 | Higher | LD (r = 0.417; P = 0.013); CRP (r = 0.513; P = 0.003); total protein (r = −0.383; P = 0.018); albumin (r = −0.451; P = 0.004) |
4482 | Higher | Neutrophil count (r = 0.353; P = 0.032); LD (r = 0.463; P = 0.005); CRP (r = 0.600; <0.0005); total protein (r = −0.358; P = 0.027); albumin (r = −0.339; P = 0.037) |
4680 | Lower | Viral load (r = −0.374; P = 0.042); bilirubin (r = −0.403; P = 0.012) |
5908 | Lower | Neutrophil count (r = −0.39; P = 0.017); total leukocyte count (r = −0.373; P = 0.023); largest change in chest x-ray (r = −0.377; P = 0.04) |
6634 | Lower | CRP (r = −0.384; P = 0.033); total protein (r = 0.402; P = 0.012); albumin (r = 0.388; P = 0.016) |
7769 | Lower | Bilirubin count (r = −0.405; P = 0.012); LD (r = −0.374; P = 0.027) |
8606 | Higher | Neutrophil count (r = 0.364; P = 0.027); LD (r = 0.539; P = 0.001); CRP (r = 0.425; P = 0.017); age (r = 0.321; P = 0.049); total protein (r = −0.432; P = 0.007); albumin (r = −0.446; P = 0.017) |
8619 | Higher | Neutrophil count (r = 0.394; P = 0.016); LD (r = 0.511; P = 0.002); CRP (r = 0.445; P = 0.012); age (r = 0.362; P = 0.026); total protein (r = −0.437; P = 0.006); albumin (r = −0.440; P = 0.006) |
8635 | Higher | Neutrophil count (r = 0.408; P = 0.012); LD (r = 0.509; P = 0.002); CRP (r = 0.484; P = 0.006); total protein (r = −0.472; P = 0.003); albumin (r = −0.459; P = 0.004) |
8814 | Higher | Neutrophil count (r = 0.391; P = 0.017); LD (r = 0.510; P = 0.002); albumin (r = −0.335; P = 0.04) |
8831 | Higher | Neutrophil count (r = 0.339; P = 0.040); LD (r = 0.483; P = 0.003); CRP (r = 0.379; P = 0.036); total protein (r = −0.333; P = 0.041); albumin (r = −0.360; P = 0.027) |
8865 | Higher | Neutrophil count (r = 0.383; P = 0.019); total leukocyte count (r = 0.330; P = 0.046) |
8937 | Higher | LD (r = 0.448; P = 0.007); CRP (r = 0.521; P = 0.003); total protein (r = −0.415; P = 0.010); albumin (r = −0.459; P = 0.004) |
8956 | Lower | LD (r = 0.465; P = 0.005); CRP (r = 0.623; <0.0005); total protein (r = −0.459; P = 0.004); albumin (r = −0.500; P = 0.001) |
17 878 | Higher | Neutrophil count (r = 0.584; <0.0005); total leukocyte count (r = 0.373; P = 0.023); LD (r = 0.694; <0.0005); CRP (r = 0.502; P = 0.004); total protein (r = −0.434; P = 0.006); albumin (r = −0.481; P = 0.002) |
24 505 | Higher | Viral load (r = 0.467; P = 0.0009); total protein (r = −0.481; P = 0.002); albumin (r = −0.488; P = 0.002) |
28 119 | Higher | LD (r = 0.375; P = 0.038); CRP (r = 0.375; P = 0.038); total protein (r = −0.375; P = 0.020); albumin (r = −0.416; P = 0.009) |
88 637 | Lower | Total protein (r = 0.348; P = 0.032); initial change in chest x-ray (r = −0.394; P = 0.031) |
differentiation of sars by two-way hierarchical clustering analysis of serum proteomic fingerprints
In the dendrogram (Fig. 22 ), majority of SARS cases (95%) were grouped under 4 clusters. There were significantly more cases with poor prognosis [required treatment in the intensive care unit (ICU) and/or supplemental oxygen during treatment] in SARS clusters 2 and 3.
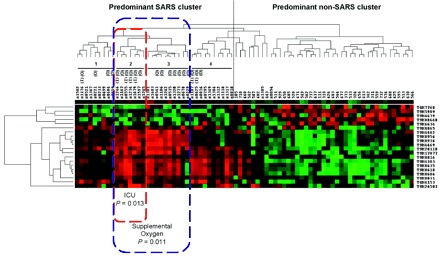
Two-way hierarchical clustering analysis (complete linkage) of the serum proteomic features among the SARS cases (case number starting with s) and non-SARS control cases (case numbers not starting with s).
The intensity of the red or green color indicates that the relative protein concentration is higher than or lower than the mean value, respectively. The column labels indicate the individual cases; the row labels indicate the differential proteomic features found in the serum samples. I, case required care in the ICU; O, case required supplemental oxygen.
diagnostic values of individual proteomic features
The areas under the ROC curves for most of the SARS-specific proteomic features were between 0.733 and 0.995. The 2 biomarkers at m/z 28 119 and 5908 gave the largest ROC curve areas. For the biomarker at m/z 28 119, the ROC curve area was 0.987 (95% confidence interval, 0.966–1.007; Fig. 3A3A ). At 97% specificity, the sensitivity was 97%. For the biomarker at m/z 5908, the ROC curve area of 1/peak intensity was 0.995 (95% confidence interval, 0.985–1.004; Fig. 3B3B ). At 95% specificity, the sensitivity was 100%.
protein identities of the potential biomarkers
Attempts were made to purify and identify the 2 biomarkers with the highest diagnostic values and the 2 biomarkers correlated with viral load. The CM10 ceramic beads captured proteomic features similar to those of the CM10 ProteinChip array. The proteins eluted from the CM10 ceramic beads were separated and concentrated as protein spots by 2-dimensional gel electrophoresis. We successfully identified the protein spots with masses corresponding to the proteomic features with m/z values of 5908, 24 505, and 28 119, which were internal fragment of fibrinogen α-E chain, immunoglobulin κ light chain, and N-terminal fragment of complement C3c α-chain, respectively (Fig. 44 ).
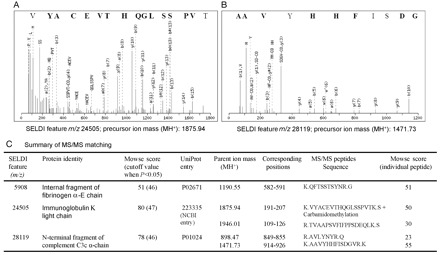
MS/MS identification of the representative biomarkers.
(A), MS/MS spectrum of one of the tryptic peptides from biomarker m/z 24 505; (B), MS/MS spectrum of one of the tryptic peptides from biomarker m/z 28 119. Amino acids in bold indicate y-series or b-series fragment ions identified from the spectra. Positions of the amino acids are indicated according to the b-series ions. (C), summary of the MS/MS matching, the Mowse score, and the required score for significant matching (P <0.05).
Discussion
This study demonstrates that the SELDI ProteinChip technology can identify serum proteins or protein fragments that are differentially regulated in adult SARS patients. To avoid identification of falsely significant differential proteomic features (16), we used a data-mining strategy similar to that used in our previous studies (10)(13). We considered only the differential proteomic features that were correlated with at least 2 biochemical/clinical features considered SARS-specific. This strategy was adopted to ensure that the identified disease-specific proteomic features had biological meaning and were not the product of systematic bias. We successfully identified potential biomarkers reflecting various physiologic or pathologic responses of the body to SARS infection, including acute-phase reaction (7)(17), lung damage (18)(19)(20), impairment of liver function (21)(22)(23), neutrophil activation (24)(25)(26), and viral load (5)(10)(27)(28).
The most sensitive marker, the proteomic feature at m/z 5908, which was negatively correlated with neutrophil count and with the largest chest radiographic changes, was found to be an internal fragment of fibrinogen α-E chain. On the one hand, we previously reported that a high neutrophil count was a risk factor associated with clinical deterioration in SARS (26). On the other hand, intravascular fibrin deposition has been observed in SARS patients (29). Vascular fibrin thrombi are often associated with pulmonary infarcts. Specific interactions between neutrophils and fibrin thrombi are well recognized (30). In addition, neutrophils produce a neutral peptide–generating protease that can cleave fibrinogen into peptide fragments (31). It is possible that fibrinogen and/or its fragments are involved in the pathophysiologic mechanism linking neutrophil activation and lung damage.
The next most sensitive biomarker is the proteomic feature at m/z 28 119. This feature was identified as the N-terminal fragment of complement C3c α-chain. Complement 3 (C3), which is composed of an α chain (Mr 115 000) and a β chain (Mr 75 000), is the central molecule in complement systems comprising the classic, alternative, or lectin pathways. On activation and subsequent inactivation of C3, several physiologic protein fragments, such as C3c, are produced. When examined by sodium dodecyl sulfate–polyacrylamide gel electrophoresis, C3c separates into a β-chain (Mr 75 000) and 2 fragments of α-chain (Mr 27 000 N-terminal fragment and Mr 43 000 C-terminal fragment) (32). The presence of free C3c α-chain N-terminal fragment in the blood circulation might be the result of degradation of C3c. In the SARS patients, the concentration of this C3c fragment was positively correlated with CRP, suggesting its positive association with the acute-phase reaction and with the activation of the complement system. It is worth nothing that this C3c fragment contains a binding domain for complement receptor type 1 (33). Activation of complement receptor type 1 enhances phagocytosis of the neutrophils (34) and activates B-cell differentiation (35)(36). To date, information about the activation of the complement pathway in SARS patients has been limited. Liao et al. (17) reported that there was no significant difference in C3 concentrations between SARS and control patients. Another group demonstrated that SARS-CoV could trigger complement activation through the lectin pathway (37). The biological relevance of C3 in SARS remains unknown.
The proteomic feature at m/z 24 505, which was increased in SARS patients and was positively correlated with viral load, was found to be immunoglobulin κ light chain. This finding is consistent with our recent finding of anti-SARS-CoV IgG in 93% of SARS cases at the time of sampling. IgG was first detected on day 4 of illness (38). Higher IgG concentrations were detected in patients with poor outcome (i.e., requiring supplemental oxygen for hypoxia or treatment in the ICU).
Aside from using individual differential proteomic features as biomarkers, one could combine all of the differential features to form a SARS-specific fingerprint. The SARS-specific fingerprint not only could differentiate SARS from non-SARS diseases with similar symptoms, but also could be useful in identifying patients with poor prognosis. The prognostic capability could be explained by the fact that the identified differential proteomic features were correlated with clinical and biochemical features having prognostic values, including viral load (27)(28), neutrophil counts (26), LD (26)(39)(40)(41), and age (40)(41).
Previously, 2 research teams, using the SELDI ProteinChip technology, reported potential biomarkers in the sera of adult SARS patients (7)(11). In the present study, the intensity of the proteomic feature at m/z 7769 was significantly lower in SARS patients (Mann–Whitney test, P <0.001); in their study, Yip et al. (7) also reported that it is significantly lower in SARS patients (Mann–Whitney test, P = 4.9 × 10−8). Except for this proteomic feature, the SARS-associated proteomic features in these other studies were different from our findings. These differences might be attributable to different selection criteria for the controls. In the previous studies, the control cases were either healthy persons or persons with viral infections from other clinics. The extent of similarities of symptoms between the SARS and control groups and the time point of blood collection were not considered. In the present study, the control group comprised suspected SARS patients who were admitted to the same hospital as the SARS patients but were later shown to be negative for SARS-CoV infection. The symptoms in the SARS and control groups and the time point of blood collection were very similar. The biomarkers identified in the present study may thus have an advantage in an actual diagnostic setting compared with those identified in the previous studies.
The difference in the findings reported by Yip et al. (7) and the present study could be also attributable to the use of different profiling methodologies. In their study, Yip et al. (7) used a comprehensive profiling approach. After being denatured with urea and detergent, the serum proteins were first fractionated with anion-exchange beads to give 6 fractions, which were later analyzed with copper ProteinChip arrays and weak cation-exchange CM10 ProteinChip arrays. Use of the comprehensive profiling approach would increase the chance of identification of more potential protein markers (8). In the present study, we analyzed the serum proteins directly after purification with only CM10 ProteinChip arrays at 2 different binding conditions (pH 4 and pH 9). We chose the CM10 ProteinChip arrays (previously called WCX2) because Kang et al. (11) showed that this chip type gives the best profiling result when analyzing serum samples from SARS patients by a direct binding approach (11). Although the direct binding approach might lead to the discovery of fewer biomarkers, such direct binding assays have higher potential to be modified for use as a clinical assay that can be used even when the protein identities of the disease-specific SELDI peaks are not known.
The most commonly used assays for detecting SARS are based on the detection of viral RNA (6) or antibodies against the SARS-CoV (5). Detection of viral RNA is useful in the early phase of the disease, whereas the serology test for antibodies against SARS-CoV is useful from 21 days onward. The current study has demonstrated that within the first week after onset of fever, similar to viral RNA concentration, the serum proteome contains both diagnostic and prognostic information. The SELDI ProteinChip assay could be used for first-line detection of SARS, followed by the quantitative viral RNA assay for confirmation. Once the disease is confirmed, the treatment strategy could be adjusted according to the prognosis based on the SELDI ProteinChip profiling result and the viral RNA concentration.
In conclusion, we have demonstrated that disease-specific proteomic fingerprints are present in the sera of adult SARS patients. They could be used to identify SARS cases during the early stage of the disease with high specificity and sensitivity. These markers may provide information about the patient’s physiologic status as well as prognostic information. The 2 proteomic features having the highest diagnostic value were the N-terminal fragment of complement C3c α-chain and an internal fragment of fibrinogen α-E chain. The proteomic feature (m/z 24 505) positively correlated with viral load was identified as immunoglobulin κ light chain.
Acknowledgments
The project team is supported by the Research Fund for the Control of Infectious Diseases (RFCID) from the Health, Welfare and Food Bureau of the Hong Kong SAR Government.
Footnotes
References
Full text links
Read article at publisher's site: https://doi.org/10.1373/clinchem.2005.061689
Read article for free, from open access legal sources, via Unpaywall:
https://academic.oup.com/clinchem/article-pdf/52/3/421/32686901/clinchem0421.pdf
Citations & impact
Impact metrics
Citations of article over time
Alternative metrics
Smart citations by scite.ai
Explore citation contexts and check if this article has been
supported or disputed.
https://scite.ai/reports/10.1373/clinchem.2005.061689
Article citations
Complement and complement regulatory proteins are upregulated in lungs of COVID-19 patients.
Pathol Res Pract, 247:154519, 08 May 2023
Cited by: 3 articles | PMID: 37244049 | PMCID: PMC10165854
Complement and COVID-19: Three years on, what we know, what we don't know, and what we ought to know.
Immunobiology, 228(3):152393, 11 May 2023
Cited by: 8 articles | PMID: 37187043 | PMCID: PMC10174470
Review Free full text in Europe PMC
Highly pathogenic coronavirus N protein aggravates inflammation by MASP-2-mediated lectin complement pathway overactivation.
Signal Transduct Target Ther, 7(1):318, 14 Sep 2022
Cited by: 78 articles | PMID: 36100602 | PMCID: PMC9470675
Complement Inhibition and COVID-19: The Story so Far.
Immunotargets Ther, 10:273-284, 26 Jul 2021
Cited by: 13 articles | PMID: 34345614 | PMCID: PMC8323860
Review Free full text in Europe PMC
A SARS-CoV-2 antibody curbs viral nucleocapsid protein-induced complement hyperactivation.
Nat Commun, 12(1):2697, 11 May 2021
Cited by: 45 articles | PMID: 33976229 | PMCID: PMC8113585
Go to all (56) article citations
Data
Data behind the article
This data has been text mined from the article, or deposited into data resources.
BioStudies: supplemental material and supporting data
Nucleotide Sequences
- (1 citation) ENA - AY278554
Similar Articles
To arrive at the top five similar articles we use a word-weighted algorithm to compare words from the Title and Abstract of each citation.
Proteomic fingerprints for potential application to early diagnosis of severe acute respiratory syndrome.
Clin Chem, 51(1):56-64, 18 Nov 2004
Cited by: 36 articles | PMID: 15550479 | PMCID: PMC7108460
Proteomic analysis reveals platelet factor 4 and beta-thromboglobulin as prognostic markers in severe acute respiratory syndrome.
Electrophoresis, 33(12):1894-1900, 01 Jul 2012
Cited by: 18 articles | PMID: 22740477 | PMCID: PMC7163558
Proteomic profiling in SARS: diagnostic and prognostic applications.
Hong Kong Med J, 15 Suppl 8:15-18, 01 Dec 2009
Cited by: 2 articles | PMID: 20393206
Application of ProteinChip array profiling in serum biomarker discovery for patients suffering from severe acute respiratory syndrome.
Methods Mol Biol, 382:313-331, 01 Jan 2007
Cited by: 7 articles | PMID: 18220240 | PMCID: PMC7120874