Abstract
Free full text

Variations in the progranulin gene affect global gene expression in frontotemporal lobar degeneration
Abstract
Frontotemporal lobar degeneration is a fatal neurodegenerative disease that results in progressive decline in behavior, executive function and sometimes language. Disease mechanisms remain poorly understood. Recently, however, the DNA- and RNA-binding protein TDP-43 has been identified as the major protein present in the hallmark inclusion bodies of frontotemporal lobar degeneration with ubiquitinated inclusions (FTLD-U), suggesting a role for transcriptional dysregulation in FTLD-U pathophysiology. Using the Affymetrix U133A microarray platform, we profiled global gene expression in both histopathologically affected and unaffected areas of human FTLD-U brains. We then characterized differential gene expression with biological pathway analyses, cluster and principal component analyses, and subgroup analyses based on brain region and progranulin (GRN) gene status. Comparing 17 FTLD-U brains to 11 controls, we identified 414 upregulated and 210 downregulated genes in frontal cortex (P-value < 0.001). Moreover, cluster and principal component analyses revealed that samples with mutations or possibly pathogenic variations in the GRN gene (GRN+, 7/17) had an expression signature that was distinct from both normal controls and FTLD-U samples lacking GRN gene variations (GRN-, 10/17). Within the subgroup of GRN+ FTLD-U, we found >1300 dysregulated genes in frontal cortex (P-value < 0.001), many participating in pathways uniquely dysregulated in the GRN+ cases. Our findings demonstrate a distinct molecular phenotype for GRN+ FTLD-U, not readily apparent on clinical or histopathological examination, suggesting distinct pathophysiological mechanisms for GRN+ and GRN- subtypes of FTLD-U. In addition, these data from a large number of human brains provide a valuable resource for future testing of disease hypotheses.
INTRODUCTION
Frontotemporal lobar degeneration (FTLD) is the second most common cause of dementia in individuals below the age of 65 years (1), with devastating effects on patients and families. Several clinical classification schemes for FTLD exist (2–4), but in general individuals suffer progressive atrophy of their frontal and temporal lobes, with corresponding deficits in the domains of behavior, social and executive function, and/or language. Pathologically, FTLD can be divided into those cases which have tau-positive inclusions (FTLD-tau) and those which are tau- and alpha-synuclein-negative but show ubiquitinated inclusions (FTLD-U), with rare cases exhibiting neither tau-positive nor ubiquitin-positive inclusions (5,6).
FTLD-U is the most commonly seen pathology (7), and recent advances have led to a greater understanding of FTLD-U pathophysiology at a protein and gene level. In 2006, two groups demonstrated that mutations in the gene progranulin (GRN) were associated with many cases of FTLD-U (8,9). Most of the GRN mutations found thus far result in premature termination (8–10), and protein haploinsuffiency has been suggested as a mechanism of disease (8–11). The major protein in the hallmark ubiquitinated inclusions of FTLD-U was subsequently identified as TAR DNA binding protein 43 (TDP-43), encoded by the gene TARDBP (12). Very recently, progranulin depletion has been shown to increase caspase-dependent cleavage of TDP-43 in an in vitro model, offering a possible mechanism to link these two disease-associated abnormalities (13). Strikingly, TDP-43 appears to be the major protein within the pathological inclusions of frontotemporal dementia with motor neuron disease (FTD-MND) and sporadic amyotrophic lateral sclerosis (ALS) as well (12,14–17), lending support to the view that these diseases are part of a clinicopathological spectrum (18).
With the advent of TDP-43 immunostaining, different histopathological subtypes of FTLD-U are increasingly being recognized (6,17,19). Within these subtypes, GRN mutations are found mainly, possibly exclusively, among a subtype (Sampathu Type 3) characterized by numerous neuronal cytoplasmic inclusions, short dystrophic neurites and a variable number of neuronal intranuclear inclusions in the superficial cortical layers (19). However, while GRN mutations are found in Type 3 cases, not all Type 3 cases carry GRN mutations (6,17). Clinically, GRN mutation cases present heterogeneously within the FTLD spectrum (10,20,21).
Although the function of the disease-associated protein TDP-43 is largely unknown, several lines of evidence point to a role in transcriptional regulation. First, TDP-43 is known to bind DNA (22,23), having first been identified in its role as a protein that binds to HIV transactive response DNA (24). Second, TDP-43 is known to bind RNA, regulating through this interaction the splicing of the cystic fibrosis transmembrane conductance regulator (25) and other genes (26). Finally, physiologic expression of TDP-43 appears to be nuclear (12,27,28), where it colocalizes with other nuclear bodies believed to function in transcription and splicing (27), and TDP-43 binds a number of heterogeneous ribonucleoproteins with well-known splicing activities (28).
The confluence of permissive technology and what is known about the biology of this disease sets the stage for a genome-wide analysis of gene expression in FTLD-U. In this study, we used microarray technology to evaluate the global gene expression of multiple regions of human FTLD-U brain, in comparison with neurologically normal controls. Our aims were (i) to identify dysregulated genes and pathways in this disease, (ii) to compare gene expression changes in neuropathologically affected (frontal cortex, hippocampus) and unaffected (cerebellum) areas of brain and (iii) to compare gene expression changes in FTLD-U cases with (GRN+) and without (GRN−) progranulin gene alterations.
We found that mRNA changes are extensive in FTLD-U. Moreover, GRN+ cases had a global gene expression signature that was distinct from both GRN- cases and controls, with over 1300 genes dysregulated in frontal cortex. Gene Ontology (GO) and Kyoto Encyclopedia of Genes and Genomes (KEGG) pathway analyses implicated some biological pathways as common to both GRN+ and GRN− cases (e.g. MAPK signaling), while others (e.g. TGF-beta signaling, cell cycle regulation) were unique to GRN+ cases. Taken together, our findings reveal major transcriptional changes in FTLD-U as a whole, as well as a previously unrecognized molecular signature for GRN+ FTLD-U.
RESULTS
Identification of genes differentially expressed in FTLD-U cases compared to controls
We examined global gene expression in 17 human FTLD-U brains and 11 age- and sex-matched controls (Table 1). Analyses included tissue dissected from the frontal cortex and hippocampus (regions showing significant histopathology in disease) and cerebellum (region with little histopathology in disease). We compared FTLD-U cases with controls, treating each brain region separately and controlling for age and gender. FTLD-U frontal cortex exhibited a greater number of differentially expressed transcripts relative to controls, while FTLD-U cerebellum had many fewer differentially expressed genes, consistent with patterns of histopathology. Because of a paucity of hippocampal control samples, we could not make a statistically meaningful comparison between disease and control among the hippocampal samples.
Table 1.
Human brain samples analyzed by microarray
Control | All FTLD-U | GRN+ FTLD-U | GRN− FTLD-U | Total | |
---|---|---|---|---|---|
Total cases | 11 | 17 | 7 | 10 | 28 |
![]() | 7 | 7 | 3 | 4 | 14 |
![]() | 4 | 10 | 4 | 6 | 14 |
![]() | 67 (54–75) | 69 (59–75) | 71 (67–77) | 64 (53–72) | |
![]() | 7 (5–14.5) | 6 (4–9.5) | 6 (5–7) | 7.5 (3–11) | |
Total samples | 18 | 42 | 16 | 26 | 60 |
![]() | 8 | 13 | 5 | 8 | 21 |
![]() | 8 | 16 | 6 | 10 | 24 |
![]() | 2 | 13 | 5 | 8 | 15 |
PMI, postmortem interval; IQR, interquartile range (25th–75th percentile).
Age, sex and PMI were not significantly different between cases and controls by a Mann–Whitney test. GRN+ FTLD-U includes all cases with progranulin gene abnormalities (mutations and variants), while GRN− FTLD-U denotes cases without progranulin gene abnormalities.
In FTLD-U frontal cortex (16 FTLD-U, 8 controls), 624 probe sets representing 536 genes demonstrated significant differential expression at a P-value of 0.001 (Table (Table2).2). Of these, 414 probe sets were increased in disease, while 210 were decreased in disease. In FTLD-U cerebellum (10 FTLD-U, 7 controls), in contrast, only 70 probe sets representing 75 genes demonstrated significant differential expression at a P-value of 0.001. (Some probes recognized more than one gene.) To correct for multiple hypothesis testing, false discovery rates (FDR) were calculated for each comparison (Table (Table2).2). Of the 624 probe sets differentially expressed in FTLD-U frontal cortex, only 4% are expected to be false positives. In addition, to focus on genes likely to have a more significant biological effect, we imposed a minimum fold-change cutoff of two to identify genes with greater magnitudes of dysregulated expression. Applying this fold-change cutoff still resulted in our finding 185 probe sets representing 159 genes dysregulated in FTLD-U frontal cortex (Table (Table2).2). Of these, 142 transcripts were increased in disease, and 43 transcripts were decreased in disease (Supplementary Material, Table S1); the top 20 transcripts showing differential expression are summarized in Table Table33.
Table 2.
mRNA changes in human FTLD-U brain—by brain region and progranulin status
Samples | Overall probes | Increased in disease | Decreased in disease | FDR (%) | |
---|---|---|---|---|---|
FTLD-U versus normal | |||||
![]() | 16 versus 8 | 624 (185) | 414 (142) | 210 (43) | 4 |
![]() | 10 versus 7 | 70 (8) | 28 (3) | 42 (5) | 27.2 |
GRN+ FTLD-U versus Normal | |||||
![]() | 6 versus 8 | 1311 (772) | 579 (284) | 732 (488) | 1.9 |
![]() | 4 versus 7 | 50 (15) | 25 (3) | 25 (12) | 39.4 |
GRN- FTLD-U versus Normal | |||||
![]() | 10 versus 8 | 154 (40) | 94 (33) | 60 (7) | 13.8 |
![]() | 6 versus 7 | 218 (86) | 171 (60) | 47 (26) | 8.7 |
Numbers reflect probe sets with differential expression (overall probes), increased expression in FTLD-U (increased in disease) or decreased expression in FTLD-U (decreased in disease) at P < 0.001. The total number meeting this statistical threshold is shown, while the number of probes meeting both a P < 0.001 criterion and fold change >2 criterion appears in parentheses.
FDR, false discovery rate. Determined by class permutation analysis (similar results were obtained with calculations based on nominal P-value). GRN+ FTLD-U=FTLD-U cases with progranulin gene abnormalities. GRN− FTLD-U=FTLD-U cases without progranulin gene abnormalities.
Table 3.
Top 20 transcripts showing differential expression in FTLD-U
Probe ID | P-value | FC | Symbol | Gene |
---|---|---|---|---|
Genes showing greatest increases in expression | ||||
209292_at | 4.36E−06 | 4.5806 | ID4 | Inhibitor of DNA binding 4 |
204719_at | 1.36E−05 | 4.5518 | ABCA8 | ATP-binding cassette, sub-family A (ABC1), member 8 |
221841_s_at | 1.92E−05 | 4.2471 | KLF4 | Kruppel-like factor 4 (gut) |
206101_at | 4.23E−04 | 4.1557 | ECM2 | Extracellular matrix protein 2 |
209047_at | 3.76E−04 | 4.1472 | AQP1 | Aquaporin 1 (Colton blood group) |
205856_at | 4.33E−04 | 4.0672 | SLC14A1 | Solute carrier family 14 (urea transporter) |
208451_s_at | 3.50E−05 | 4.0529 | C4A/C4B | Complement component 4A |
205907_s_at | 4.59E−04 | 4.0307 | OMD | Osteomodulin |
211896_s_at | 1.05E−04 | 3.8509 | DCN | Decorin |
205608_s_at | 2.47E−06 | 3.6276 | ANGPT1 | Angiopoietin 1 |
209335_at | 5.73E−04 | 3.4846 | DCN | Decorin |
214428_x_at | 1.81E−04 | 3.3938 | C4A/C4B | Complement component 4A |
211813_x_at | 2.04E−04 | 3.1688 | DCN | Decorin |
207542_s_at | 3.87E−04 | 3.1580 | AQP1 | Aquaporin 1 (Colton blood group) |
218418_s_at | 1.36E−05 | 3.1493 | ANKRD25 | Ankyrin repeat domain 25 |
204457_s_at | 9.84E−04 | 3.1478 | GAS1 | Growth arrest-specific 1 |
201893_x_at | 2.23E−04 | 3.1145 | DCN | Decorin |
217678_at | 4.70E−04 | 3.0599 | SLC7A11 | Solute carrier family 7 |
213110_s_at | 5.25E−05 | 3.0167 | COL4A5 | Collagen, type IV, alpha 5 (Alport syndrome) |
213592_at | 8.44E−04 | 3.0154 | AGTRL1 | Angiotensin II receptor-like 1 |
Genes showing greatest decreases in expression | ||||
213479_at | 7.64E−04 | 0.1994 | NPTX2 | Neuronal pentraxin II |
207768_at | 5.95E−05 | 0.2053 | EGR4 | Early growth response 4 |
206115_at | 2.67E−05 | 0.2189 | EGR3 | Early growth response 3 |
219697_at | 3.09E−04 | 0.2375 | HS3ST2 | Heparan sulfate (glucosamine) 3-O-sulfotransferase 2 |
216086_at | 1.08E−04 | 0.2523 | SV2C | Synaptic vesicle glycoprotein 2C |
211616_s_at | 3.67E−04 | 0.2825 | HTR2A | 5-hydroxytryptamine (serotonin) receptor 2A |
218625_at | 3.67E−04 | 0.3156 | NRN1 | Neuritin 1 |
220794_at | 1.34E−04 | 0.3343 | GREM2 | Gremlin 2, Cysteine knot superfamily |
210090_at | 9.41E−04 | 0.3535 | ARC | Activity-reg cytoskeleton-associated protein |
207135_at | 8.85E−04 | 0.3617 | HTR2A | 5-hydroxytryptamine (serotonin) receptor 2A |
205586_x_at | 1.51E−04 | 0.3690 | VGF | VGF nerve growth factor inducible |
201693_s_at | 9.81E−04 | 0.3815 | EGR1 | Early growth response 1 |
201694_s_at | 4.98E−04 | 0.3888 | EGR1 | Early growth response 1 |
210227_at | 1.49E−04 | 0.3997 | DLGAP2 | Discs, large (Drosophila) homolog-associated protein 2 |
206161_s_at | 5.14E−04 | 0.4151 | SYT5 | Synaptotagmin V |
204506_at | 9.89E−04 | 0.4154 | PPP3R1 | Protein phosphatase 3 (formerly 2B), regulatory subunit B, 19 kDa, alpha isoform |
205721_at | 2.08E−06 | 0.4201 | GFRA2 | GDNF family receptor alpha 2 |
204945_at | 6.75E−04 | 0.4223 | PTPRN | Protein tyrosine phosphatase, receptor type, N |
207548_at | 5.98E−04 | 0.4246 | GRM7 | Glutamate receptor, metabotropic 7 |
214078_at | 1.63E−04 | 0.4254 | PAK3 | P21 (CDKN1A)-activated kinase 3 |
Sixteen FTLD-U cases were compared to 8 normal controls on Affymetrix U133A microarrays. A statistical cutoff of P < 0.001 was used to identify genes with differential expression, which were then ranked based on fold change (FC, disease/normal). The 20 genes with largest increases (top) and decreases (bottom) in expression, identified by GeneSymbol and name, are shown for frontal cortex samples.
To further evaluate the overall structure of transcriptional variation, we performed a cluster analysis of gene expression levels. Using Spearman correlation coefficients, we clustered all microarray samples across all regions and disease states in an unbiased fashion to create a hierarchical tree. With one exception, all 17 cerebellar samples regardless of disease status fell into one branch, demonstrating that these samples show different global expression from other brain regions (Fig. 1). Samples from frontal cortex and hippocampus, however, were admixed, despite the fact that cytoarchitecture in these regions is different.
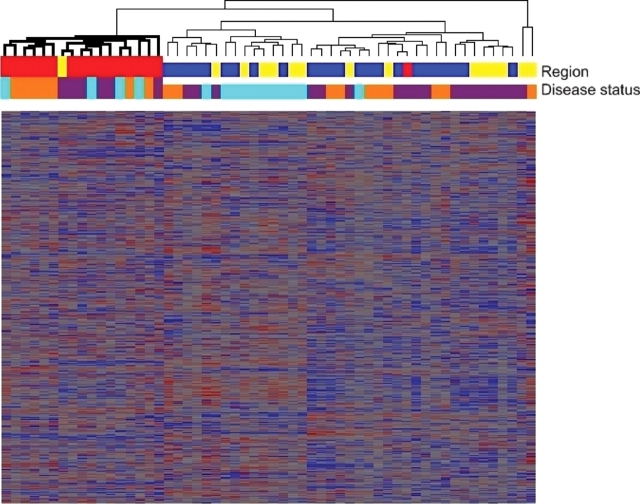
Cluster analysis of all samples shows a distinct expression signature for cerebellum. Hierarchical dendrogram produced by clustering of expression levels for 56 brain samples (columns) and 22 277 transcripts (rows), using Spearman correlation coefficients. With one exception, all 17 cerebellar samples (red) regardless of disease status fell into one branch (bold), while samples from frontal cortex (blue) and hippocampus (yellow) were admixed. Disease status for each sample is shown as well, with orange denoting normal cases, purple denoting FTLD-U cases without progranulin gene abnormalities (GRN− FTLD-U) and teal denoting FTLD-U cases with progranulin gene abnormalities (GRN+ FTLD-U). Heatmap tiles show standardized expression levels of individual genes with red denoting high expression, grey denoting medium expression and blue denoting low expression levels.
If transcriptional dysregulation is a key feature of FTLD-U, one might expect more changes in gene expression in this disease than in others because of the putative nuclear transcriptional functions of TDP-43. We therefore compared the results of our study with microarray studies of other neurodegenerative diseases. Comparisons made across microarray datasets are hampered, however, by the fact that different platforms, statistical conditions and experimental conditions are used, leading to differences in statistical power and noise. To control for some of these conditions, we compared our study only with those studies using postmortem human cortical samples, with sample sizes comparable to or larger than our own frontal cortex dataset (16 disease, 8 control). We chose three representative comparison studies, one evaluating 22 disease and 9 control hippocampal samples in Alzheimer’s Disease (AD, 29) one evaluating 15 disease and 5 control frontal cortex samples in sporadic Creutzfeld-Jacob Disease (sCJD, 30) and one evaluating 11 disease and 9 control motor cortex samples in sporadic ALS (31). In each case, we re-evaluated our dataset using the statistical conditions used in the comparison study, to obtain the number of genes that would be deemed to be significantly changed in a comparable analysis (Supplementary Material, Table S2). As shown in Figure Figure2,2, many more gene changes were found in the present FTLD-U study than in studies of AD or ALS; the number of dysregulated genes in FTLD-U was comparable to that seen in cases of sCJD.
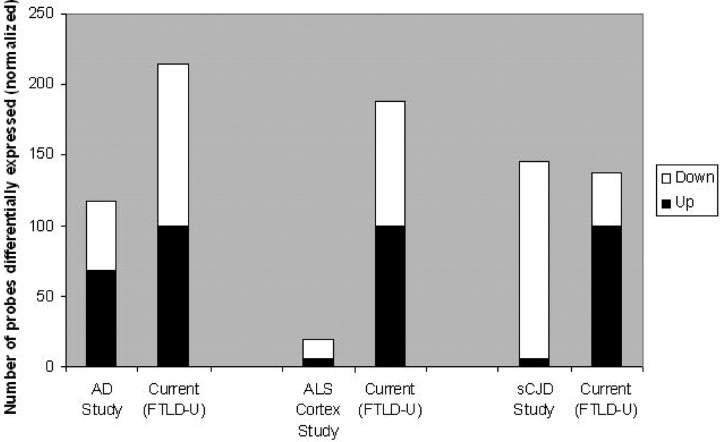
Number of dysregulated genes identified in human cortex microarray studies of various neurodegenerative diseases. Data from present study (FTLD-U) was re-analyzed using the same statistical conditions as each comparison study (29–31). See Supplementary Material, Table S3 for details of other studies. The black portion of each bar represents the number of upregulated genes in disease compared to control, while the white portion represents the number of downregulated genes in disease compared to control. Many more gene changes were found in the present FTLD-U study than in studies of AD or ALS.
In summary, many genes are differentially expressed in FTLD-U cases compared to controls. In addition, regional patterns of gene expression changes are consistent with patterns of histopathology, with frontal cortex being much more affected than cerebellum. Finally, cerebellar gene expression carries a signature distinct from that of frontal cortex or hippocampus.
Effect of progranulin gene status on global gene expression
Genetic screening of our FTLD-U cases revealed 7 cases containing progranulin gene abnormalities (GRN+). Of these, four possessed truncation mutations previously associated with disease and believed to be pathogenic, while the remaining three cases exhibited new variants of unknown significance (VUS, Table Table4).4). Preliminary studies suggest that at least some of these VUS are pathogenic, and this avenue of investigation is currently ongoing. Using Spearman correlation coefficients, we clustered all frontal cortex and hippocampal samples into a hierarchical dendrogram to look for any naturally arising subsets of samples. We found that almost all GRN+ samples (9/11), regardless of region of origin, clustered together into one branch without interspersion of GRN− cases of FTLD-U or control samples (Fig. (Fig.3A).3A). This clustering result was robust to statistical methodology, seen in dendrograms produced using Pearson correlation coefficients as well.

Cluster analysis and principal components analysis of samples from histopathologically affected brain regions show a distinct expression signature for GRN+ FTLD-U. (A) Hierarchical dendrogram produced by clustering of expression levels for 39 brain samples from frontal cortex or hippocampus (columns) and 22 277 transcripts (rows), using Spearman correlation coefficients. With two exceptions, all 11 GRN+ FTLD-U samples (yellow) fell into one branch (bold), while samples from GRN− FLTD-U (red) and normal controls (blue) were admixed. Brain region for each sample is shown as well, with orange denoting frontal cortex samples and teal denoting hippocampal samples. Heatmap tiles show standardized expression levels of individual genes with red denoting high expression, grey denoting medium expression and blue denoting low expression levels. (B) Principal components analysis of frontal and hippocampal samples using 22 277 transcripts also revealed a distinct expression signature for GRN+ FTLD-U. GRN+ FTLD-U samples (yellow) were characterized by a low score along the first principal component (X-axis) and a high score along the second principal component (Y-axis), separating them from GRN− FTLD-U samples (red) and normal samples (blue). Arrows indicate the two samples that were more distant in global gene expression by cluster analysis. Within GRN+ FTLD-U cases, samples bearing truncation mutations (squares) and samples bearing variants of unknown significance (triangles) were admixed. R493X=predicted protein change for indicated truncation mutant. R418X=predicted protein change for indicated truncation mutant.
Table 4.
Cases bearing progranulin gene alterations
Case | cDNA | Protein | Truncation/variant | Reference |
---|---|---|---|---|
1 | c.1252C>T | R418X | Truncation | (9) |
2 | c.1477C>T | R493X | Truncation | (10) |
3 | c.348A>C | S116S | Silent variant near splice site | New |
4 | c.911G>A | W304X | Truncation | (10) |
5 | IVS708+1-+4 del GTGA | Intronic | Intronic variant at splice site | New |
6a | c.1009C>T | Q337X | Truncation | (53) |
c.903G>A | S301S | |||
c.1070C>A | P357R | |||
7 | c.1058G>A | S353 N | Missense variant | New |
FTLD-U cases bearing progranulin gene abnormalities (GRN+ FTLD-U) included 4 cases with truncation mutations previously associated with disease and believed to be pathogenic, as well as 3 cases with new variants of unknown significance (VUS) that are being investigated further.
aCase 6 possesses three separate abnormalities within the progranulin gene. In each case, cDNA numbering is based on GenBank NM_002087.2, and predicted protein numbering is based on GenPept NP_0020278.1.
cDNA, complementary DNA; New, new variant which has not been previously reported.
Principal component analysis was performed as a second methodology for looking in an unbiased manner at overall data structure (Fig. (Fig.3B).3B). Within histopathologically affected regions of brain, GRN+ FTLD-U cases again grouped together, defined by a low score along the first principal component (X-axis) and a high score along the second principal component (Y-axis).
Based on the observation from unbiased cluster and principal component analyses that GRN+ FTLD-U cases have a molecular expression signature which is distinct from GRN− FTLD-U cases and control subjects, we performed subgroup analyses of our gene expression data. Comparing 6 GRN+ frontal cortex samples to 8 normal frontal cortex samples, we found 1311 probe sets representing 1131 genes with differential expression at a P-value of 0.001, with an FDR of 1.9% (Table (Table2).2). Of these, 579 probe sets were increased in disease, and 732 were decreased in disease. After imposing a minimum fold change cutoff of 2, 772 probe sets representing 657 genes were still found to be differentially expressed at a P-value of 0.001. Of these, 284 probe sets were increased in disease, and 488 were decreased in disease (Supplementary Material, Table S3); the top 20 transcripts showing differential expression are summarized in Table Table5.5. In contrast, comparing 10 GRN- frontal cortex samples to 8 normal frontal cortex samples, we found only 154 probe sets representing 147 genes showing differential expression at a P-value of 0.001 (FDR 13.8%, Table Table2).2). In fact, when GRN+ and GRN− frontal cortex samples were compared directly, more genes were found to be differentially expressed (208 probe sets representing 214 genes—several probes recognize more than one gene) between these molecular subtypes of disease than between the GRN− cases and normals (Supplementary Material, Table S4).
Table 5.
Top 20 transcripts showing differential expression in GRN+ FTLD-U
Probe ID | P-value | FC | Symbol | Gene |
---|---|---|---|---|
Genes showing greatest increases in expression | ||||
204719_at | 9.67E−05 | 6.3989 | ABCA8 | ATP-binding cassette, sub-family A (ABC1), member 8 |
205907_s_at | 1.84E−04 | 6.0441 | OMD | Osteomodulin |
221841_s_at | 1.12E−04 | 5.6157 | KLF4 | Kruppel-like factor 4 (gut) |
211896_s_at | 6.57E−05 | 5.3074 | DCN | Decorin |
202409_at | 2.05E−04 | 5.3061 | C11ORF43 | Chromosome 11 open reading frame 43 |
209335_at | 2.45E−04 | 5.1019 | DCN | Decorin |
209292_at | 1.28E−04 | 5.0969 | ID4 | Inhibitor of DNA binding 4 |
206101_at | 1.83E−04 | 5.0700 | ECM2 | Extracellular matrix protein 2 |
206373_at | 8.05E−05 | 4.5235 | ZIC1 | ZIC family member 1 |
211742_s_at | 8.28E−05 | 4.4841 | EVI2B | Ecotropic viral integration site 2B |
206100_at | 3.58E−05 | 4.3504 | CPM | Carboxypeptidase M |
204457_s_at | 9.77E−04 | 4.2875 | GAS1 | Growth arrest-specific 1 |
211813_x_at | 5.88E−05 | 4.2649 | DCN | Decorin |
201893_x_at | 3.97E−05 | 4.2531 | DCN | Decorin |
213975_s_at | 9.53E−04 | 4.2301 | LILRB1/LYZ | Leukocyte immunoglobulin-like receptor, subfamily B; lysozyme (renal amyloidosis) |
205608_s_at | 2.48E−07 | 4.2236 | ANGPT1 | Angiopoietin 1 |
218418_s_at | 1.60E−06 | 4.2142 | ANKRD25 | Ankyrin repeat domain 25 |
202403_s_at | 4.28E−04 | 4.2031 | COL1A2 | Collagen, type I, alpha 2 |
208451_s_at | 1.34E−04 | 4.1915 | C4B/C4A | Complement component 4A |
203042_at | 2.14E−05 | 4.1637 | LAMP2 | Lysosomal-associated membrane protein 2 |
Genes showing greatest decreases in expression | ||||
221805_at | 3.57E−04 | 0.0837 | NEFL | Neurofilament, light polypeptide 68 kDa |
205825_at | 3.40E−04 | 0.0838 | PCSK1 | Proprotein convertase subtilisin/kexin type 1 |
206935_at | 2.05E−05 | 0.0860 | PCDH8 | Protocadherin 8 |
214432_at | 2.21E−04 | 0.1106 | ATP1A3 | ATPase, NA+/K+ transporting, α3 polypeptide |
204337_at | 4.79E−05 | 0.1146 | RGS4 | Regulator of G-protein signaling 4 |
203998_s_at | 8.59E−04 | 0.1191 | SYT1 | Synaptotagmin I |
203000_at | 1.18E−04 | 0.1280 | STMN2 | Stathmin-like 2 |
201939_at | 2.83E−05 | 0.1330 | PLK2 | Polo-like kinase 2 (Drosophila) |
206115_at | 2.22E−06 | 0.1342 | EGR3 | Early growth response 3 |
204339_s_at | 1.24E−04 | 0.1399 | RGS4 | Regulator of G-protein signaling 4 |
206552_s_at | 4.88E−04 | 0.1414 | TAC1 | Tachykinin, precursor 1 (substance K) |
203999_at | 4.34E−05 | 0.1420 | SYT1 | Synaptotagmin I |
220359_s_at | 6.21E−05 | 0.1433 | ARPP-21 | Cyclic AMP-regulated phosphoprotein, 21 kDa |
206678_at | 1.63E−04 | 0.1443 | GABRA1 | GABA A receptor, alpha 1 |
214230_at | 4.53E−05 | 0.1454 | CDC42 | Cell division cycle 42 (GTP binding protein, 25 kDa) |
203001_s_at | 3.02E−04 | 0.1476 | STMN2 | Stathmin-like 2 |
201340_s_at | 3.92E−04 | 0.1477 | ENC1 | Ectodermal-neural cortex |
204035_at | 1.68E−04 | 0.1490 | SCG2 | Secretogranin II (chromogranin C) |
221916_at | 1.94E−04 | 0.1507 | NEFL | Neurofilament, light polypeptide 68 kDa |
204722_at | 7.20E−05 | 0.1540 | SCN3B | Sodium channel, voltage-gated, type III, beta |
Six FTLD-U cases with progranulin gene abnormalities (GRN+ FTLD-U) were compared to 8 normal controls on Affymetrix U133A microarrays. A statistical cutoff of P < 0.001 was used to identify genes with differential expression, which were then ranked based on fold change (FC, disease/normal). The 20 genes with largest increases (top) and decreases (bottom) in expression, identified by GeneSymbol and name, are shown for frontal cortex samples.
The finding that there are many more dysregulated genes among the GRN+ cases is striking given the fact that the smaller number of GRN+ cases offers less statistical power. The large number of dysregulated genes common to this subset of cases corroborates evidence from our cluster and principal component analyses that the GRN+ cases have a distinct expression signature.
As with the full dataset, cerebellar samples exhibited many fewer dysregulated genes (Table (Table22).
Quantitative real-time QRT–PCR validation of gene changes detected on microarrays
To corroborate gene changes detected on our microarrays, we performed QRT–PCR for a subset of upregulated and downregulated genes. For all genes tested, microarray and QRT–PCR results were consistent in both GRN+ and GRN− FTLD-U cases (Fig. (Fig.4).4). We used ß-actin as our reference gene for normalizing gene expression levels based on microarray data showing stable expression; comparable results were obtained using cyclophilin A as an alternate reference gene (data not shown). In general, relative to QRT–PCR, microarray results tended to underestimate the magnitude of gene changes. This has been observed in other studies as well (30,32), and likely reflects the greater amount of noise around microarray measurements, with their subsequent need for normalization.
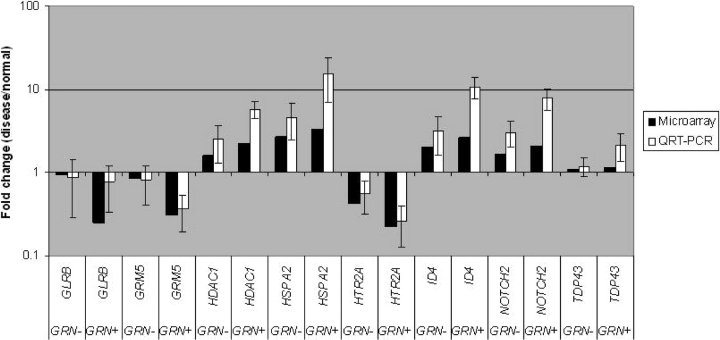
Validation of microarray results by quantitative reverse transcription real-time PCR (QRT–PCR). Relative expression levels of genes are indicated by the fold changes in expression level for FTLD-U samples with progranulin gene abnormalities (GRN+), and FTLD-U samples without progranulin gene abnormalities (GRN−). For QRT–PCR, average fold changes and standard deviations of three cases in each group are shown. PCR reactions were performed in duplicate. GLRB, glycine receptor beta subunit; GRM5, metabotropic glutamate receptor 5; HDAC1, histone deacetylase 1; HSPA2, heat shock protein 2 (70 kD); HTR2A, serotonin receptor 2A; ID4, inhibitor of DNA binding 4; NOTCH2, Notch homolog (Drosophila) 2.
Biological pathway analysis
Using the Database for Annotation, Visualization, and Integrated Discovery (DAVID) database mining tool (Materials and Methods), we identified biological process categories significantly over-represented in GRN+ and GRN− cases of FTLD-U. We queried both the GO database and the KEGG database using all probe sets showing differential expression in frontal cortex between disease and normal at P < 0.001. We analyzed GRN+ and GRN- FTLD-U cases separately because of our observation that GRN+ cases have a distinct signature and because the strong effect of the GRN+ cases tended to drive overall findings within a mixed FTLD-U disease group.
The top GO and KEGG biological process categories showing over-representation in genes dysregulated in GRN- FTLD-U cases (P < 0.05) are shown in Table Table6.6. Genes involved in the MAPK signaling pathway, which has been implicated in a number of neurodegenerative diseases (33–35), are downregulated, as are genes involved in ion transport and cell localization. Genes involved in lipid metabolism, on the other hand, appear to be upregulated in GRN− FTLD-U, as has been shown for AD and HD as well (29,35).
Table 6.
Dysregulated biological processes in GRN− FTLD-U
Database | Biological process | P-value | Biological process subgroups |
---|---|---|---|
Biological processes enriched in upregulated genes | |||
GO | Lipid metabolism group | 2.39E−02 | Cellular lipid metabolism, lipid metabolism, lipid biosynthesis, membrane lipid metabolism |
Biological processes enriched in downregulated genes | |||
KEGG | MAPK signaling pathway | 6.23E−04 | |
GO | Transport group | 3.26E−02 | Di-, tri-valent inorganic cation transport, calcium ion transport, metal ion transport, cation transport, transport, ion transport |
GO | Localization group | 4.78E−02 | Establishment of localization, transport, localization |
Frontal cortex samples from 10 FTLD-U cases without progranulin gene abnormalities (GRN− FTLD-U) were compared to 8 normal controls on Affymetrix U133A microarrays to identify genes with differential expression at P < 0.001. Biological pathway analysis using the KEGG and GO databases was then performed as described in Materials and Methods, and biological processes over-represented (P < 0.05) in genes upregulated in disease (top) and downregulated in disease (bottom) are shown. Biological process categories from the GO database were combined into functionally related groups, for which the median P-value of the subgroups is shown.
Many more biological pathways were over-represented in GRN+ FTLD-U (Table (Table7).7). Of these, the majority of the biological process categories were made up of genes downregulated in disease. These included all three categories downregulated in GRN− FTLD-U (MAPK signaling, ion transport, localization), as well as many pathways which are unique to GRN+ FTLD-U. Among the downregulated pathways unique to GRN+ FTLD-U were pathways identified in other neurodegenerative disease microarray studies—synaptic transmission, calcium signaling, neurotransmitter secretion, microtubule-based movement (29,35)—as well as pathways such as axon guidance identified in proteomics studies of neurodegeneration (34).
Table 7.
Dysregulated biological processes in GRN+ FTLD-U
Database | Biological Process | P-value | Biological process subgroups |
---|---|---|---|
Biological processes enriched in upregulated genes | |||
KEGG | Focal adhesion | 1.20E−02 | |
KEGG | TGF-beta signaling | 1.32E−02 | |
KEGG | Regulation of actin cytoskeleton | 1.60E−02 | |
KEGG | ECM-receptor interaction | 4.53E−02 | |
GO | Negative regulation of biological process group | 2.16E−03 | Negative regulation of cellular process, negative regulation of cellular physiological process, negative regulation of biological process, negative regulation of physiological process |
GO | Cell communication group | 7.96E−03 | Intracellular signaling cascade, signal transduction, cell communication |
GO | Cell cycle group | 2.69E−02 | Negative regulation of progression through cell cycle, regulation of progression through cell cycle, regulation of cell cycle |
GO | Small GTPase mediated signal transduction group | 4.30E−02 | Regulation of Ras protein signal transduction, Ras protein signal transduction, regulation of small GTPase mediated signal transduction |
Biological processes enriched in downregulated genes | |||
KEGG | Calcium signaling pathway | 1.48E−09 | |
KEGG | Long-term potentiation | 2.32E−09 | |
KEGG | Gap junction | 3.43E−05 | |
KEGG | Axon guidance | 1.15E−03 | |
KEGG | Long-term depression | 8.81E−03 | |
KEGG | MAPK signaling pathway | 1.02E−02 | |
GO | Organismal physiological process/synaptic transmission group | 7.24E−08 | Transmission of nerve impulse, synaptic transmission, cell–cell signaling, neurophysiological process, organismal physiological process |
GO | Localization group | 4.68E−05 | Establishment of localization, localization, transport |
GO | Secretion/transport group | 1.60E−04 | Neurotransmitter secretion, regulated secretory pathway, regulation of neurotransmitter levels, synaptic vesicle transport, vesicle-mediated transport, secretion, secretory pathway, synaptic vessel exocytosis, exocytosis |
GO | Ion transport group | 1.60E−04 | Metal ion transport, cation transport, calcium ion transport, ion transport, monovalent inorganic cation transport, di-,tri-valent inorganic cation transport, potassium ion transport |
GO | Cytoskeleton organization/protein polymerization group | 1.56E−02 | Microtubule-based movement, protein polymerization, cytoskeleton organization and biogenesis, cytoskeleton-dependent intracellular transport, microtubule-based process |
Frontal cortex samples from 6 FTLD-U cases with progranulin gene abnormalities (GRN+ FTLD-U) were compared to 8 normal controls on Affymetrix U133A microarrays to identify genes with differential expression at P < 0.001. Biological pathway analysis using the KEGG and GO databases was then performed as described in Materials and Methods, and biological processes over-represented in genes upregulated in disease (top, P < 0.05) and downregulated in disease (bottom, P < 0.02) are shown. Biological Process categories from the GO database were combined into functionally related groups, for which the median P-value of the subgroups is shown.
We found eight biological processes categories upregulated in GRN+ FTLD-U (Table (Table7).7). These did not include lipid metabolism, which was the only biological pathway upregulated in the GRN− cases. As with the downregulated pathways, many biological processes found in this study had been identified in previous studies of neurodegenerative disease. These include signal transduction (including small GTPase-mediated signal transduction) and focal adhesion, identified to be dysregulated in HD (35) and AD (29), respectively, as well as regulation of actin cytoskeleton, implicated in a proteomics study of multiple neurodegenerative diseases (34). In addition, we found several specific biological pathways upregulated in this subset which may be unique to GRN+ FTLD-U; they have not been identified in previous microarray-based neurodegenerative disease studies or in our GRN− FTLD-U cases. These include the TGF-ß signaling pathway and regulation of the cell cycle.
DISCUSSION
Global mRNA expression profiling with the Affymetrix U133A microarray platform interrogated over 22 000 transcripts and detected a large number of differentially expressed genes in FTLD-U postmortem frontal cortex samples. The vast majority of these dysregulated genes were not found in cerebellum samples. This aspect of our analysis echoes previous histopathological studies, which have shown significant pathology, both by traditional methods and by TDP-43 staining, in frontal cortex but not in cerebellum (6). With respect to GRN status, however, this molecular phenotyping approach revealed differences which are not captured clinically or histopathologically.
A distinct expression signature for GRN+ FTLD-U
By cluster analysis, principal component analysis and biological pathway analysis, we found that GRN+ FTLD-U cases had a distinct expression signature. While there have been suggestions of clinical and pathological differences in some FTLD-U cases with GRN mutations (36,38,39), the overall heterogeneity of presentations makes it difficult or nearly impossible to make distinctions on clinical or histopathological grounds alone (10,20,21). The ability to detect distinct molecular phenotypes, however, may be important in identifying patient groups more responsive to targeted therapies.
It is important to note that cluster and principal component analyses revealing the distinct expression signature for GRN+ FTLD-U cases were performed in an unbiased manner; we did not pre-specify subgroups but rather evaluated the overall pattern of similarity in global gene expression among all samples. The fact that the GRN truncation mutants (four cases) formed a group with the three cases carrying GRN gene VUS was somewhat surprising. This finding has implications for the pathogenicity of these variants, which are under investigation now.
Notably, 2 of the 11 GRN+ samples were more distant in global gene expression from the other 9 samples (Fig. (Fig.3B,3B, labeled arrows). Of these, one (Fig. (Fig.3A,3A, arrow) was particularly distant and remained so regardless of clustering methodology. This frontal cortex sample came from a case bearing the truncation mutation R493X. The corresponding hippocampal sample from this case did cluster with the other GRN+ cases. On reviewing this case, we noted significant alpha-synuclein pathology in addition to TDP-43 pathology in the frontal cortex sample, which may explain the status of this case as an outlier. The R493X mutation has previously been found in multiple FTLD-U families (10). Frontotemporal dementia and primary progressive aphasia have been the associated clinical diagnoses; pathologically, cases bearing this mutation have been characterized by ubiquitin-positive intranuclear inclusions, consistent with a Sampathu Type 3 classification (10,19). Coexisting alpha-synuclein pathology has not been previously described; thus, along with the cases bearing GRN gene VUS, this case is also being investigated further. The other sample clustering separately from the majority of the GRN+ FTLD-U cases is a frontal cortex sample also bearing a truncation mutation (R418X). This sample, however, appeared more related to the other GRN+ FTLD-U samples, with distance decreasing with the use of a parametric correlation coefficient. The corresponding hippocampal sample from this case fell into the main GRN+ FTLD-U cluster.
We considered various possibilities to explain our finding of a distinct GRN+ FTLD-U expression signature. First, this finding could be the spurious result of different treatment conditions for these samples. However, the 56 brain samples used in this study were collected in random order, with the operator blinded to disease and gene status. Processing of all samples after collection was performed on two consecutive days, using the same batch of microarray platforms, and the GRN+ samples were divided between the 2 days. Second, this finding could be the result of differing RNA quality in this subset. However, review of RNA quality parameters for these selected cases did not reveal any obvious differences from the rest of the samples. Third, while all cases in this study bearing GRN gene abnormalities exhibited Sampathu Type 3 histopathology (19), not all of the GRN− FTLD-U cases were Type 3, raising the possibility that the non-Type 3 cases drove this apparent difference in global gene expression. We therefore re-analyzed our data excluding the four GRN− FTLD-U cases that were not Sampathu Type 3. Excluding these cases did not materially change results (Supplementary Material, Fig. S1). Thus, we believe that the distinct global expression signature observed for GRN+ FTLD-U cases in this study carries biological significance.
Comparison to previous FTLD and ALS gene expression studies
While our study corroborated the findings of a previous FTLD gene expression study (40) that synapse-related genes are downregulated and cytoskeleton-associated genes are upregulated, both our experimental design and overall findings exhibited several important differences from the previous study. Because we compared affected and unaffected brain regions and also cases with and without GRN mutations, we were able to detect significant differences in expression levels in frontal cortex versus cerebellum, and in GRN+ cases versus GRN- cases relative to each other and controls. In addition, compared to the previous study, we found many more genes, involving many more biological pathways, to be dysregulated in both our FTLD-U overall group and in our GRN+ subset (Supplementary Material, Table S2). Fluorescent signal was present for TARDBP and GRN gene expression in all of our samples, which was not the case in the previous study (40). Finally, we found approximately equal numbers of up- and down-regulated genes for FTLD-U overall, as well as our GRN+ and GRN- subsets. In contrast, the previous study found almost all of the genes showing significant differential expression to be downregulated in disease (40).
The differing results of these two studies could reflect the fact that our study included many more samples, with greater pathological homogeneity, both of which increase statistical power.
In addition, we compared our results to a study of gene expression changes in sporadic ALS motor cortex (31) and found many more genes with differential expression (Fig. (Fig.2).2). In sporadic ALS, TDP-43-positive inclusions have been found in lower motor neurons (16) as well as motor cortex (37,41). These regional differences in TDP-43 histopathology and gene expression changes in FTLD-U and ALS are worthy of further examination.
Genes implicated in previous neurodegenerative disease studies
Several of the individual genes found to be dysregulated in our study have been implicated in previous studies of neurodegenerative disease. The gene found to be most downregulated in our GRN+ FTLD-U frontal cortex samples was the 68 kD neurofilament (NF) light polypeptide (NEFL), which has been associated with motor neuron disease on pathological, mouse model and human genetic grounds. Pathologically, aberrant NF accumulation has long been considered a hallmark of ALS (42). In addition, mice overexpressing NEFL (43) and mice carrying a point mutation in NEFL (44) show selective dysfunction and degeneration of spinal motor neurons. More recently, it has been argued that TDP-43 acts physiologically as a stabilizer of NEFL mRNA, with loss of this normal function involved in the pathology of FTLD-U (45). Because NFs are abundant structural components of neurons, the apparent loss of NF mRNA from postmortem samples of brain or spinal cord might be attributable simply to neuronal loss. Arguing against this, however, is the fact that we found NEFL mRNA expression to be downregulated only in our GRN+ cases. The GRN− FTLD-U cases, which have as much neuronal loss, did not show any differences in levels of NEFL mRNA expression.
GRN mutations are associated with FTLD-U, but we did not find dysregulated GRN mRNA expression in either our GRN+ or GRN− subsets. We considered several possible explanations. First, this result could be an artifact of combining GRN truncation mutants (four cases) with cases containing GRN gene VUS (three cases). We therefore evaluated these two categories separately but were still unable to find a statistically significant difference in GRN gene expression between either group and normal controls. Second, although total mRNA and protein levels of progranulin have been shown to be decreased in FTLD-U cases with GRN mutations (8,9), most of these studies have focused on mRNA isolated from lymphoblastoid cells rather than brain. In addition, sequencing of cDNA from brain and lymphoblastoid cells has demonstrated preferential expression of the normal allele (8,9), but this does not exclude the possibility that there is a compensatory increase in expression of the normal allele in the affected organ (brain), resulting in little difference in total mRNA levels. Third, in one case of documented decreased total mRNA expression in brain (46), GRN mRNA levels were decreased by ~20% in frontal cortex and ~10% in cerebellum; by microarray standards, such changes are relatively small and may have been below the detection level of our experimental design. Finally, the P-values for differential expression for the three GRN probe sets on our microarray platform were 0.006, 0.048 and 0.005. In an effort to detect the most robust gene changes, we may have set our statistical threshold too stringently (at P < 0.001) to identify this gene as significantly dysregulated. Of note, we are systematically evaluating progranulin transcript levels in different regions of human brain with QRT–PCR now and will report these findings separately.
TDP-43, the major protein found in the characteristic inclusions of FTLD-U and ALS, has been the subject of intense study recently. Initial genetic studies of TDP-43, encoded by the gene TARDBP, have not revealed obvious mutations within FTLD-U patients (47); mRNA expression studies are lacking. In our microarray analysis, TARDBP mRNA levels were not significantly changed among either GRN+ or GRN− cases, compared to controls. There was a suggestion (Fig. (Fig.4)4) of slightly higher mRNA levels in the three GRN+ cases selected for QRT–PCR testing, but this observation remains preliminary.
Novel biological pathways in GRN+ FTLD-U
The present study of RNA expression profiles in human FTLD-U brain provides evidence for the commonality of some biological pathways in multiple neurodegenerative diseases. This accords with previous work implicating mechanisms such as oxidative stress (48), mitochondrial dysfunction (49), aberrant protein folding and accumulation (50), synaptic dysfunction (51) and others in several neurodegenerative diseases. Indeed, some have argued that neurodegenerative disorders are linked not just by final common pathways of cell dysfunction and death but by an actual network of interacting proteins in which abnormalities in one or a few proteins may cause dysfunction of the entire network (52,53). One recent study constructed an interacting proteomic map, with nodes representing various proteins implicated in AD, PD, ALS, HD, dentatorubropallidoluysian atrophy and prion disease (34); this map was then used to identify KEGG biological pathways enriched in disease-associated proteins. Of the 8 KEGG pathways identified in this manner, 3 (axon guidance, focal adhesion, regulation of actin cytoskeleton) were identified in our study as well, with an additional 2 (adherens junction, P = 0.07, Wnt signaling pathway, P = 0.08) falling just short of our P-value cutoff for significance.
Although these common pathways are important in understanding neurodegeneration as a whole, biological pathways specific to a particular disease may be key to developing targeted therapies. To that end, our study identified two biological pathways which may be specific to GRN+ FTLD-U. We found the categories of TGF-ß signaling and the cell cycle to be significantly enriched in genes upregulated in GRN+ FTLD-U. These pathways did not emerge from human brain gene expression studies of HD (35) or AD (29), general proteomics studies of neurodegeneration (34) or from analyses of our GRN− FTLD-U subset of cases.
Limitations of postmortem brain studies
One limitation of studies using postmortem tissue is that observed changes in gene expression may reflect either primary events in pathogenesis or secondary responses such as inflammation, cell death and neuronal loss. In addition, we used a regional sampling approach which by definition involves sampling of mixed populations of glial and neuronal cells. We chose this approach to minimize the amount of RNA degradation, which is greater with microdissection techniques. However, in doing so, we considered the possibility that primary pathophysiological changes might be masked by secondary changes of less biological importance.
Two lines of evidence from our present study support the idea that most of the genes and pathways identified have biological relevance. First, we found a distinct molecular phenotype for the GRN+ cases, which is unlikely to be due simply to non-specific inflammation and cell death, since the GRN− cases were clinically and histopathologically similar and handled the same way. Similarly, the presence of a distinct GRN+ FTLD-U expression signature made it unlikely that our findings are simply the result of neuronal cell loss, causing a more ‘glial’ signature in diseased brains, since both GRN+ and GRN− cases exhibited comparable amounts of neuronal cell loss. Second, many microarray studies have found genes involved in stress or immune response and apoptosis to be upregulated in their datasets (29,31), raising the issue of whether microarrays on postmortem tissue simply reflect the end-stage events of vulnerable cells dying. While categories of response to stress and apoptosis did appear in our biological pathway analysis, they were much less significantly enriched and did not meet our stringent statistical criterion for inclusion.
Conclusions and implications for future studies
We used a global mRNA expression profiling approach to characterize human postmortem FTLD-U brain. Our study was large, with 56 brain samples, and designed to evaluate the effect of brain region and progranulin gene status. We found many dysregulated genes within the regions of the brain affected histopathologically in disease, but not in areas which are relatively histopathologically spared. In addition, we found a distinct molecular phenotype for GRN+ FTLD-U, with associated biological pathways which may be specific for this subtype of disease. This finding has implications for future therapeutic strategies.
Although our study did not focus exclusively on one histopathological subtype of FTLD-U, Sampathu Type 3 FTLD-U cases (19) comprised the majority of our disease samples. We emphasized this type in order to create a balanced comparison between GRN+ and GRN− cases, since GRN mutations are found primarily within Type 3 cases. Future studies investigating whether the gene changes seen extend to all histopathological subtypes of FTLD-U, and to FTD-MND and ALS, would be a valuable addition to the data presented here.
Finally, the rationale for performing an RNA expression analysis in FTLD-U came from the observation that a DNA- and RNA-binding protein, TDP-43, may be involved in pathogenesis. The fact that a vast number of genes show dysregulated expression in brain regions characterized by TDP-43 pathology lends support to the idea that gene expression changes seen in FTLD-U may be more than bystander effects or lead-generating ‘hits’ for further analysis. If transcriptional dysregulation is a key disease mechanism in FTLD-U, these changes may be central to its pathophysiology.
MATERIALS AND METHODS
Human brain samples
Seventeen FTLD-U cases and 11 neurologically normal controls were sampled from the University of Pennsylvania Center for Neurodegenerative Disease Research Brain Bank (Table (Table1).1). Informed consent was obtained for postmortem studies. Age, sex and postmortem interval (PMI) to autopsy were not significantly different between cases and controls. All FTLD-U cases were reviewed by a board-certified neuropathologist. In order to facilitate comparison of GRN+ and GRN− cases, the majority of the FTLD-U cases used (13/17) met criteria for FTLD-U Type 3 according to Sampathu (19). All FTLD-U cases were evaluated for abnormalities in the progranulin gene, as previously described (54). Among the FTLD-U cases, seven had progranulin gene alterations (GRN+), with four cases carrying known pathogenic mutations and three cases carrying VUS (Table (Table4).4). Preliminary evidence indicates that at least two of the VUS may be pathogenic by affecting mRNA splicing. Studies of these variants are in progress. All seven GRN+ cases met criteria for FTLD-U Type 3. Control brains had no evidence of neurological disease either clinically or neuropathologically. For each brain used, a 50–100 mg sample of frozen tissue was collected from frontal cortex grey matter anterior to the genu of the corpus callosum, cerebellum and hippocampus (where available), while keeping the brain on dry ice. Samples were either processed immediately or stored at −80°C.
RNA extraction and hybridization
Frozen tissue samples were homogenized for 30–60 s using a PowerGen 35 (Fisher Scientific). RNA was isolated using TRIzol (Invitrogen) and RNeasy Mini columns (Qiagen), according to standard manufacturer protocols. All subsequent steps were conducted as described in the Affymetrix GeneChip Expression Analysis Technical Manual (Affymetrix). Briefly, 3 µg of total RNA from each sample were used to prepare biotinylated fragmented cRNA with products from Affymetrix according to manufacturer protocols. Microarrays (Affymetrix Human Genome U133A) assessing expression of 22 277 transcripts were hybridized for 16 h at 45°C. Chips were washed and stained, and hybridization signals were collected with a GeneChip 3000 7G scanner (Affymetrix).
Microarray quality control
Measures were taken both prior to microarray hybridization and after hybridization to ensure quality control. After RNA isolation, RNA purity and integrity were assessed by spectrophotometric measurement of 260/280 nm OD ratios and by capillary electrophoresis on an Agilent 2100 Bioanalyzer. All samples used for further analysis had a 260/280 ratio of >1.9, with sharp ribosomal RNA peaks (Supplementary Material, Fig. S2), virtually undetectable low molecular weight RNA, and RIN numbers >8 (55). After microarray hybridization, 3′/5′ ratios of GAPDH were calculated, and only chips with ratios <3 were retained. Finally, we used a quality assessment algorithm based on weights and residuals from robust regression models of gene expression to identify outlier chips (56), which were excluded from analysis. Four samples were eliminated from analysis after all quality control measures; of these, two were identified by all three methods, implying that initial RNA quality may be the most important factor.
Microarray data analysis
Statistical analyses of gene expression were performed using open source R software packages available from http://www.bioconductor.org (57). Fluorescence intensity measures of gene expression were normalized and quantified by robust multi-array analysis (58), using the affy package (59). To identify transcripts differentially expressed between disease (all FTLD-U, GRN+ subset, GRN− subset) and controls for each brain region, we used the limma package (60), which adopts a Bayesian approach. Our model corrected for gender and age. FDR were calculated based on nominal P-values as described (35) and by using a class permutation test to adjust for co-expressed genes. R-scripts for these analyses are available on request. In addition, principal components analysis and hierarchical cluster analysis using Spearman and Pearson correlation coefficients was performed using Partek software, version 6.3 Copyright © 2007 (Partek, Inc., St Louis, MO, USA).
Biological pathway analysis
We used the DAVID 2007 program available at http://david.abcc.ncifcrf.gov/ (61) to assign differentially expressed genes to biological pathways and processes annotated in the GO and KEGG databases, available at http://www.geneontology.org (62) and http://www.genome.jp/kegg/ (63), respectively. DAVID uses a Fisher’s exact test to find overrepresentation of differentially expressed genes within each biological pathway represented in the GO and KEGG databases. In addition, because of the hierarchical organization of GO categories, simple lists tend to result in similar, redundant categories. DAVID curates these GO categories into related groups based on this hierarchical structure, allowing for a more biologically meaningful analysis. We retained those pathways with a P-value of 0.05 or less (or, in the case of GO categories, functional groups with a median P-value of 0.05 or less) as statistically significant.
Quantitative RT–PCR
QRT–PCR was performed using the Applied Biosystems 7900HT Fast Real-Time PCR system. Briefly, total RNA isolated from postmortem brain samples was treated with DNAse (DNA-free kit; Ambion) according to manufacturer protocols, and 1.75 µg of DNAse-treated RNA was used to make single-stranded cDNA (Superscript III; Invitrogen) according to the manufacturer’s protocols. One microliter of the resulting cDNA then underwent further PCR cycles, using the Taqman Gene Expression System (Applied Biosystems). PCR conditions used were 95°C for 10 min, followed by 40 cycles of denaturing at 95°C for 15 s and annealing/extension at 60°C for 1 min, in a 20 µl reaction volume. Detailed information on primer sets used is given in Supplementary Material, Table S5. The delta–delta method (64) was used for relative quantification of gene expression. We used ß-actin and cyclophilin A as our reference genes. For QRT–PCR validation of microarray results, three frontal cortex samples from each group were selected to represent the controls, GRN+ cases and GRN− cases (9 total samples).
FUNDING
These studies were supported by grants from the National Institutes of Health (NIH, AG17586, AG10124, AG15116, AG023501, AG19724 and NS44266). A.S.C.-P. is a fellow of the NINDS Morris K. Udall Parkinson’s Disease Research Center of Excellence and the NIH Research Fellowship Training Program for Clinicians in Translational Research in Neurobiology of Disease. J.B.P. is supported by a Burroughs Wellcome Fund Career Award at the Scientific Interface. V.M.-Y.L. is the John H. Ware, 3rd Professor of Alzheimer’s disease research. J.Q.T. is the William Maul Measey-Truman G. Schnabel, Jr Professor of Geriatric Medicine and Gerontology.
ACKNOWLEDGEMENTS
We are grateful to the Penn Microarray Facility and Dr Don Baldwin for advice and technical assistance with array samples. We thank Dr Bruce Miller for recruiting patients who were studied. Finally, we thank our patients and their families whose generosity has made this work possible.
Conflict of Interest statement: None declared.
REFERENCES
Articles from Human Molecular Genetics are provided here courtesy of Oxford University Press
Full text links
Read article at publisher's site: https://doi.org/10.1093/hmg/ddn023
Read article for free, from open access legal sources, via Unpaywall:
https://academic.oup.com/hmg/article-pdf/17/10/1349/17244318/ddn023.pdf
Free to read at hmg.oxfordjournals.org
http://hmg.oxfordjournals.org/cgi/content/abstract/17/10/1349
Free after 12 months at hmg.oxfordjournals.org
http://hmg.oxfordjournals.org/cgi/content/full/17/10/1349
Free after 12 months at hmg.oxfordjournals.org
http://hmg.oxfordjournals.org/cgi/reprint/17/10/1349.pdf
Citations & impact
Impact metrics
Citations of article over time
Smart citations by scite.ai
Explore citation contexts and check if this article has been
supported or disputed.
https://scite.ai/reports/10.1093/hmg/ddn023
Article citations
Association of cortical morphology, white matter hyperintensity, and glymphatic function in frontotemporal dementia variants.
Alzheimers Dement, 20(9):6045-6059, 11 Aug 2024
Cited by: 1 article | PMID: 39129270 | PMCID: PMC11497707
Preclinical translational platform of neuroinflammatory disease biology relevant to neurodegenerative disease.
J Neuroinflammation, 21(1):37, 31 Jan 2024
Cited by: 2 articles | PMID: 38297405 | PMCID: PMC10832185
RNA-binding deficient TDP-43 drives cognitive decline in a mouse model of TDP-43 proteinopathy.
Elife, 12:RP85921, 11 Oct 2023
Cited by: 4 articles | PMID: 37819053 | PMCID: PMC10567115
Testing for Allele-specific Expression from Human Brain Samples.
Bio Protoc, 13(19):e4832, 05 Oct 2023
Cited by: 0 articles | PMID: 37817908 | PMCID: PMC10560631
Huntingtin and Other Neurodegeneration-Associated Proteins in the Development of Intracellular Pathologies: Potential Target Search for Therapeutic Intervention.
Int J Mol Sci, 23(24):15533, 08 Dec 2022
Cited by: 4 articles | PMID: 36555175 | PMCID: PMC9779313
Review Free full text in Europe PMC
Go to all (91) article citations
Data
Data behind the article
This data has been text mined from the article, or deposited into data resources.
BioStudies: supplemental material and supporting data
RefSeq - NCBI Reference Sequence Database
- (1 citation) RefSeq - NM_002087.2
Similar Articles
To arrive at the top five similar articles we use a word-weighted algorithm to compare words from the Title and Abstract of each citation.
Brain progranulin expression in GRN-associated frontotemporal lobar degeneration.
Acta Neuropathol, 119(1):111-122, 02 Aug 2009
Cited by: 48 articles | PMID: 19649643 | PMCID: PMC3104467
Expression of TMEM106B, the frontotemporal lobar degeneration-associated protein, in normal and diseased human brain.
Acta Neuropathol Commun, 1:36, 11 Jul 2013
Cited by: 29 articles | PMID: 24252750 | PMCID: PMC3893524
A distinct clinical, neuropsychological and radiological phenotype is associated with progranulin gene mutations in a large UK series.
Brain, 131(pt 3):706-720, 29 Jan 2008
Cited by: 147 articles | PMID: 18234697 | PMCID: PMC2577762
Mutations in progranulin (GRN) within the spectrum of clinical and pathological phenotypes of frontotemporal dementia.
Lancet Neurol, 7(10):965-974, 02 Sep 2008
Cited by: 127 articles | PMID: 18771956
Review
Funding
Funders who supported this work.
NIA NIH HHS (8)
Grant ID: AG023501
Grant ID: AG19724
Grant ID: P01 AG017586
Grant ID: P01 AG019724
Grant ID: AG17586
Grant ID: AG10124
Grant ID: AG15116
Grant ID: R01 AG015116
NINDS NIH HHS (2)
Grant ID: NS44266
Grant ID: R01 NS044266