Abstract
Free full text

PAcIFIC: how to dive deeper into the proteomics ocean
Abstract
Data-dependent precursor ion selection is widely used in shotgun proteomics to profile the protein components of complex samples. Although very popular, this bottom-up method presents major drawbacks in terms of detectable dynamic range. Here, we demonstrate the superior performance of a data-independent method we term Precursor Acquisition Independent From Ion Count (PAcIFIC). Our results show that almost the entire, predicted, soluble bacterial proteome can be thoroughly analyzed by PAcIFIC without the need for any sample fractionation other than the C18-based liquid-chromatograph used to introduce the peptide mixture into the mass spectrometer. Importantly, we also show that PAcIFIC provides unique performance for analysis of human plasma in terms of number of proteins identified (746 at FDR ≤ 0.5%) and achieved dynamic range (eight orders of magnitude at FDR ≤ 0.5%), without any fractionation other than immuno-depletion of the seven most abundant proteins.
Introduction
Continuous progress in mass spectrometry and polypeptide separation has made proteomics analysis more and more sensitive and comprehensive. Hundreds or thousands of proteins are routinely identified and quantified from complex mixtures such as tissue or cellular extracts, organelles, bacteria and body fluids1. In spite of this progress, full characterization of a proteome remains a challenge today. This is due to factors such as the large dynamic range of protein expression, complexity of the mixture in terms of numbers of proteins and their relative stoichiometries, as well as lack of methods to amplify proteins such as polymerase chain reaction for DNA. It is generally accepted that the dynamic range problem may be solved by sample fractionation and/or enrichment. Thus, most laboratories engage in numerous strategies to separate proteins and/or peptides prior to mass spectrometric (MS) analysis using methods such as anion or cation exchange, one- or two-dimensional polyacrylamide gel electrophoresis, isoelectric focusing and affinity capture or depletion2–4. Ideally, these methods should be orthogonal to each other to provide the best peak capacity and insure that each fraction is as unique as possible. In this perfect case MS methods would provide efficient sampling to thoroughly characterize each sample. In practice, none of the available separation techniques provide sufficient peak capacity, resulting in co-separation of the same peptides in two or more adjacent fractions, thus severely limiting the efficiency and overall sensitivity of downstream MS methods. Additionally, each separation or enrichment step introduces multiple fractions, each of which must be analyzed, and also introduces variability that renders quantitative proteomics more difficult and challenging5.
Another major problem limiting shotgun proteomics is the data-dependent acquisition mode generally used by mass spectrometers for automated ion selection. With data-dependent acquisition, the most abundant ionized species from each MS survey scan is selected for subsequent isolation, activation and tandem mass analysis1. This process greatly facilitated proteome coverage and extended dynamic range of detection for shotgun proteomics. A companion method referred to as “dynamic exclusion” that attempts to prevent reselection of the same ion over and over again6 added further benefits to coverage and dynamic range. However, this addition to the automated ion selection process also failed to provide full coverage of peptides in a complex mixture as judged by the numerous studies showing the non-reproducible nature of peptides detected in replicate analyses of the same sample7. Yet another method attempting to provide further benefit is gas-phase fractionation (GPF). With GPF, the sample is repetitively injected into the mass spectrometer and a unique m/z range is used for data-dependent precursor ion selection during each replicate LC-MS/MS analysis of a given sample8, 9. However, as we have recently shown, while optimum m/z ranges for GPF may be predicted from the genome of the considered species to maximize proteome coverage (i.e. genome-based GPF), the number of new protein identifications reaches a plateau using all three of these methods combined10. Moreover, a non-negligible fraction (up to 8%) of identified peptides has no clearly assigned precursor ion, suggested that more identifications are possible from unfractionated mixtures11.
This data-independent acquisition method described here acquires tandem mass spectra at every m/z value (i.e.m/z “channel”) without regard for whether a precursor ion is observed or not. In fact no precursor ion scans are even conducted. We therefore refer to this precursor ion independent method of tandem mass spectrometry as “Precursor Acquisition Independent From Ion Count” (PAcIFIC) which works as follows. During a first injection, the ion trap is used to perform data-independent collision induced dissociation (CID) at each of 10 continuous 1.5 m/z intervals across a range of 15 m/z per LC-MS analysis (Figure 1A) using a 2.5 m/z isolation width. In the next analysis a new 15 m/z range is examined in an identical fashion and so on until the considered precursor-ion m/z range of 400 – 1400 u is covered (Figure 1B). The acquired tandem mass spectra are then matched to peptide sequence in a database using the center of the precursor isolation window as the parent ion mass in each tandem mass spectrum file and considering charge states of 2+ and 3+. Therefore, a relatively large precursor mass tolerance of +/- 3.75 Daltons, corresponding to +/− 1.25 m/z unit for a triply charged precursor ion, is used to account for any precursor that could physically be present in the trap after isolation (Figure 1C). Much larger precursor-ion isolation windows have historically been used for data-independent analysis12–14, providing the advantage of fewer injections to cover the same precursor-ion m/z range. However, the resulting complexity of these tandem mass spectra made use of algorithms to reconstruct the precursor-ion / fragment ion lineage necessary. The narrow isolation window used for CID in PAcIFIC determines, by default, the peptide precursor mass without need for reconstruction of the lineage nor accurate mass of the precursor allowing a standard database search routine to make sequence assignments. This then makes PAcIFIC immediately available to any mass-spectrometry based proteomics laboratory with a low-resolution ion trap and standard database search engines.
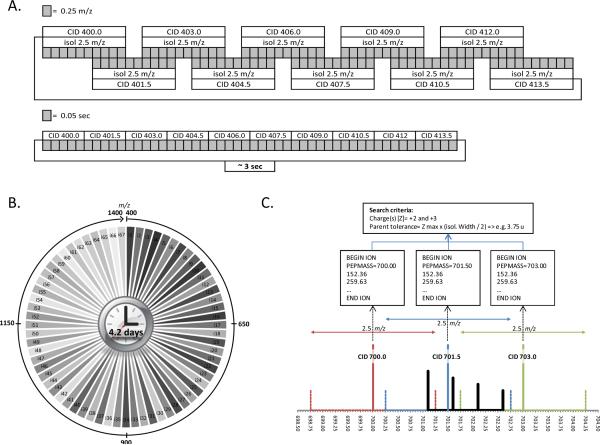
Principle of PAcIFIC. With this method, the sample is repeatedly analyzed by LCMS/MS. A) During each injection, a different precursor-ion m/z range is selected for tandem mass spectrometry independent of precursor ion measurement. B) In each narrow m/z range all precursor-ions are isolated in the ion trap and subjected to collision induced dissociation until the desired m/z range is achieved. C) Each spectra is then searched against a database using the center of the window as the precursor mass and a tolerance corresponding to: maximum charge state allowed times the isolation width divided by two, e.g. 3 × (2.5/2) = 3.75 Da.
In this report, we show that the PAcIFIC strategy, without any prior sample fractionation, provides superior protein sequence and proteome coverage compared to data-dependent acquisition methods of whole cell lysates. We also demonstrate that PAcIFIC provides unprecedented results in terms of number of proteins identified and extends detectable dynamic range by direct analysis of human plasma immuno-depleted of the top-seven most abundant proteins15.
Results and discussion
Validation on a digested complex protein mixture
First, we used the PAcIFIC strategy to analyze a soluble protein extract from the Pseudomonas aeruginosa bacteria. We chose this sample because it was already extremely well characterized in our laboratory during previous method development studies10, 11, 16. As of these most recent publications, we maximized the number of protein identifications possible from this unfractionated mixture using a genome-based GPF strategy which was by default data-dependent. A triplicate analysis of the same sample using gas-phase fractionation with eight genome-optimized m/z ranges (covering 400–2000 m/z) resulted in an average identification of 946 proteins (multiple hits) from 24 LC-MS/MS experiments. When all data acquired on this sample from genome-based GPF experiments is combined a number totaling only 1191 unique identified proteins (multiple hits) is achieved from 189 LC-MS/MS experiments. This means that the basic genome-based GPF experiment reaches quickly a plateau of proteins identified from only 24 analyses and that further attempts to capitalize on this concept are not productive. Now when we compare the results from a triplicate data-independent PAcIFIC analysis of the same sample (201 LC-MSMS experiments), covering only m/z range 400 to 1400, we reach an average total number of proteins identified equal to 1690 (multiple hits). If combined, a total of 2320 (11.5 proteins/injection) multiple hits is achieved which represents a 94% increase compared to the GPF 1191 (6.3 proteins/injection) plateau number for a nearly identical number of injections; see summary in Table 1. Additionally, by comparison to genome-based GPF, the average protein sequence coverage increased with PAcIFIC from 24% to 34%, and the average number of identified unique peptides from 5994 to 13049. Figure 2 shows how the number of identifications grows rapidly with each injection, before reaching a plateau once the entire m/z range is covered. We also note that we use extremely conservative values for determination of whether a tandem mass spectrum has matched a peptide sequence (PeptideProphet p>0.95 (FDR < 0.5%)) and for the purpose of protein identifications required two unique peptide sequences for a protein to be called “identified”.
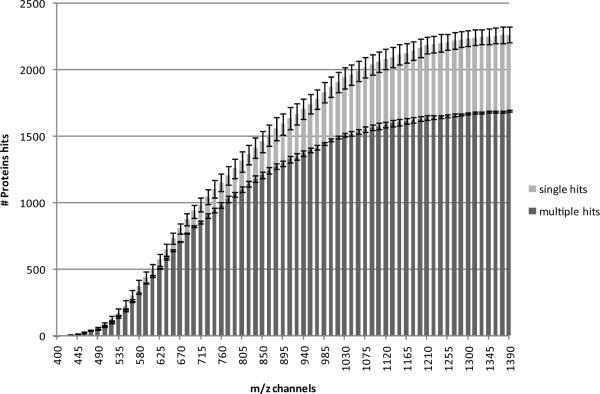
Cumulative protein identification after sequential m/z analysis with PacIFIC. The number of unique protein identifications grows rapidly with each cumulative analysis, until a plateau is reached.
Table 1
Proteins identified from the P. aeruginosa sample by GPF or PAcIFIC.
averaged DD | combined DD* | averaged DD +GPF** | combined DD +all GPF*** | averaged PAcIFIC | combined PAcIFIC | |
---|---|---|---|---|---|---|
# of runs | 3 | 9 | 24 | 189 | 67 | 201 |
Multiple hits | 394 (6) | 551 | 946 (28) | 1191 | 1690 (59) | 2320 |
Single hits | 222 (16) | 283 | 380 (4) | 393 | 576 (7) | 880 |
The numbers in parenthesis represent the standard deviation of the mean. DD: data-dependent; GPF: gas phase fractionation; PAcIFIC: peptide acquisition independent from ion count.
To explain the large difference in number of peptide and protein identifications between our prior data-dependent genome-based GPF acquisition efforts and PAcIFIC, we investigated the extent to which we observed a precursor ion for each peptide matched in confidence to a tandem mass spectrum in both the genome-based GPF data sets and the PAcIFIC generated data sets. Previously10, we noticed that close inspection of many m/z regions, where a peptide tandem mass spectrum had been acquired and a confident match made, showed no detectable trace of a precursor ion. In that prior data-dependent genome-based GPF analysis of this bacterial sample10, the rate of high confidence peptide matches to spectra without precursor ions was 8%. Normally, this would not be expected because the data-dependent process requires an ion to be detected and then selected prior to CID. However, we previously explained the absence of these ions in two ways: 1) the signal processing carried out on the fly by the FT-based instrument made it possible that an ion might be detected and then removed17 and 2) that as the m/z range used for GPF narrowed and became less complex with fewer high signal/noise ions present that the likelihood of selecting an ion obscured by noise increased18, 19. For the moment we are not able to address the data reduction issue imposed by the instrument control software. However, if the second issue is responsible for many of what we refer to as “orphan” peptide identifications (i.e. no precursor ion observed), then we would expect the rate of proteins identified via orphan peptides to increase when we conduct PAcIFIC.
In order to answer the riddle posed by the existence of proteins matches to orphan peptides, the precursor-ion m/z ratio of all PAcIFIC identified peptides was computed. In parallel, MS survey scans acquired at high sampling speed (approx. 1 scan/second) were analyzed with the feature detection and extraction software Hardklor20. Then, we verified whether a monoisotopic ion signal corresponding to an identified peptide was present within an m/z range of +/− 2.5 ppm over the entire LC-MS data set. From this experiment, we concluded that 31% of the PAcIFIC identified peptides were orphans; i.e. they had no detectable precursor ion. In contrast, only 8% of the peptides identified by data-dependent genome-based GPF acquisitions were orphans. This difference in number of orphan peptides of 8% versus 31% is in good agreement with the increase in unique peptide identifications between the two experiments of 5994 to 13049 unique peptides.
We concluded from this experiment that the increase in identifications by PAcIFIC is in large part due to the fact that these peptides were never observed during the MS survey scans of the data-dependent genome-based GPF experiments and, therefore, were never selected for tandem mass spectrometry. This group of orphan peptides then represents a category that is not typically accessible in a standard data-dependent shotgun proteomic experiment.
Next, we asked whether there were obvious differences in the physicochemical properties of orphan peptides and those with parent ions. One way to consider this question is from the perspective of differences in proteotypic peptide characteristics; i.e. those physicochemical characteristics rendering a peptide “detectable” by mass spectrometry. For this purpose, we calculated the average proteotypic score for both sets of peptide classes using previously determined empirical prototypic characteristics characteristics on the same bacterial sample and for the same instrumentation10. Surprisingly, these calculations did not show a significant difference in physicochemical proprieties between the orphan peptides and those with parent ions (supplementary figure 1). We therefore concluded that the observed difference in parent ion signals between these two groups is likely due only to peptide abundance on-column and not peptide sequence based ionization suppression events which remain poorly understood. Recently, we reported a 5% frequency for tandem mass spectra in a data-dependent genome-based gas-phase fractionation data set that were due to a combination of two or more peptides fragmented together16. We refer to those as “accidental” CID events. Given that PAcIFIC acquires tandem mass spectra using a similar isolation width for CID to data-dependent experiments; we would expect the number of accidental CID events to be similar. We checked the frequency of accidental CID events in our Pseudomonas data using the available precursor scans acquired at 60'000 resolution and modified the previously described software for precursor correction16. Thus, if multiple precursors could be found within a defined retention time window, an identical tandem mass spectrum was duplicated and the precursor mass changed accordingly. The frequency of accidental CID events was measured by calculating the number of unique peptide identifications possible from a single tandem mass spectrum. From these calculations we found a total of 5% “accidental” CID events in our Pseudomonas data set which is similar to our prior estimate from a standard data-dependent data set. Finally, we note that this number of 5% is only for the 70% of peptides for which we have detected precursor ions.
Finally, we assessed what part of the P. aeruginosa proteome was observed with the PAcIFIC technique. By merging triplicate data sets, 2320 unique proteins were identified with two or more unique peptides. The false-discovery rate (FDR) is estimated to be equal to or below 1% for each identified peptide (p > 0.95). By accepting only proteins with multiple peptide hits, the FDR of identified proteins is therefore expected to be very low. From the 5575 P. aeruginosa genes, 3417 contained neither a transmembrane domain nor a signal peptide, but contained at least two proteotypic peptides. These proteins were therefore expected to be identifiable in standard shotgun proteomic experiments. From this population of “identifiable” candidates, 1833 (54%) were indeed present in our dataset. The remaining 487 identifications corresponded to proteins containing none or only one proteotypic peptide (51), proteins with transmembrane domains (158), proteins with signal peptides (244) or proteins coded from a plasmid and/or genes not present in the P. aeruginosa PA01 genome (34). Importantly, we note that while we may not calculate copy number for the P. aeruginosa proteome, we did identify 162 proteins annotated as “transcriptional regulators” by PAcIFIC. This is approximately three times more than the 55 identified in all prior, published data-dependent GPF experiments on this sample. Finally, we assessed which proportion of so-called “housekeeping proteins”, ubiquitously expressed by the bacteria, were detected with the PAcIFIC strategy. For this purpose, a list of 721 proteins expected to be constitutively expressed and potentially identifiable by mass spectrometry was created (see materials and methods). With the PAcIFIC strategy, 81% of these were indeed identified with two or more unique peptides. In contrast, only 58% were identified using prior data-dependent acquisition mode. In composite, these results show that a large part of the P. aeruginosa proteome is now observable by PAcIFIC and this without prior sample fractionation or enrichment.
Analysis of depleted human plasma
To assess the potential of PAcIFIC on a more challenging, “timely” sample to the world of proteomic biomarker discovery, we analyzed a tryptic digest of human plasma proteins depleted of the seven most abundant proteins (100ul plasma starting material). These data were acquired in duplicate and protein identifications made using Sequest and the IPI human database (release 3.43) after which identifications were validated using PeptideProphet. We note that in an attempt to define the human plasma proteome several large projects have been conducted in the last five years and that the total number of proteins reported varies greatly with the aqueous-phase fractionation methods used either individually or in combination. Reported numbers range from 252 to approximately 3000 proteins depending on statistical filtering criteria21–25. In order to compare our dataset obtained using PAcIFIC, we performed data analysis using different stringencies ranging from 0.5% to 4.4% false discovery rate all of which are illustrated in Figure 3. With a liberal FDR, similar to that used to reach approx. 3000 by Zhang and colleagues25, set to less than 5% using PeptideProphet, an average total number of 3193 proteins were identified in our duplicate PAcIFIC dataset. This very large number is more a commentary on the pitfalls of moderate FDR use than a reflection of the number of proteins confidently identified. Thus, when a more realistic FDR of 0.5% (p > 0.95) is used, the total number of proteins identified decreased to an average of 517 proteins, 44% (n=223) of which come from multiple hits (supplementary table 1). If both lists for our duplicate set are combined instead of averaged, a total of 748 proteins are identified, 33% (n=252) of which account for multiple hits. In comparison, Whiteaker and colleagues22 who used a similar filtering cut-off (p > 0.95) with eight different aqueous-phase fractionation techniques, reached a total of 252 protein hits, 55% (n=138) of which account for multiple hits. This number is similar to our duplicate analysis (see Figure 3) with genome-based GPF (8 fractions) that yielded a total number of 234 proteins (155 multiple and 79 single hits) at an FDR of 0.4%. More impressive it the fact that they reached this number of 252 proteins by performing 160 LC-MSMS runs while our duplicate PAcIFIC set comprises 134 LC-MSMS experiments only. Thus, a 2 to 3-fold increase in proteins identified is observed using our PAcIFIC strategy with less instrument time and with only a single immuno-depletion step, by far the most effective pre-fractionation method available as pointed out clearly in the data of Whiteaker and colleagues22.
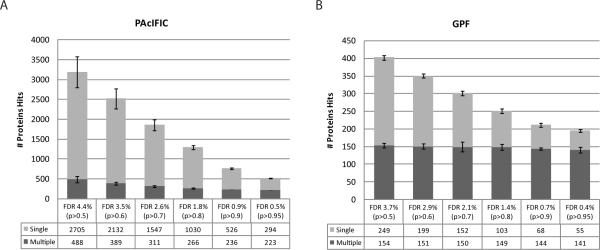
Protein results obtained from analysis of human plasma sample depleted of top-7 proteins via both acquisitions (PAcIFIC and genome-based Gas Phase Fractionation). Each dataset is represented as an average of the duplicate analysis at different filtering probabilities with their corresponding FDR. A) PAcIFIC and, B) genome-based Gas Phase Fractionation.
Considering that 31 percent of peptides in our P. aeruginosa dataset were identified as orphan peptides, we asked whether this direct analysis using PAcIFIC might have some advantage in terms of dynamic range detected over standard data-dependent analysis. To answer this question, we used literature reported plasma protein concentrations26 to compare against our set of proteins identified either by 1) PAcIFIC or 2) genome-based GPF with eight m/z ranges. Different protein lists were generated for each analytical method based on different probability cut-offs and their respective FDR. Each list was finally compared to the list of known protein concentration and observed dynamic range calculated based on the difference between the minimal and maximal concentration obtained as summarized in Figure 4 and supplementary table 2. PAcIFIC analysis detected proteins over a dynamic range of eight to mid-nine orders of magnitude depending on the FDR filtering stringency applied. This represents an increase of 1 to 3 orders of magnitude over genome-based GPF analysis of the same sample. By comparison, Zhang and colleagues25 recently published an extensive analysis of mouse plasma where they detected a logarithmic range of abundance of 7, a number similar to our GPF results. In their study, this range was achieved by depletion using a Multiple Affinity Removal System Ms-3 column (Mars-3, Agilent) and then subjected to the Intact Protein Analysis System (IPAS) methodology21 combining ion exchange and reverse phase chromatography and 566 LC-MS/MS runs to analyze all fractions from the four reference samples. By contrast our results with PAcIFIC were generated using only one fraction analyzed 134 times by LC-MS/MS with use of immuno-depletion only. The most likely explanations for this increase in dynamic range with PAcIFIC is simply that by ignoring detection of the precursor ion we do not bias our results toward high abundance signals which allows PAcIFIC to fragment barely detectable or undetectable precursor ions. This is not in itself a novel observation in that the Chait group18 reported use of an hypothesis driven tandem mass spectrometry method that looked for peptides where they expected them to be as opposed to where they were detected in precursor ion scans. This successful, but targeted approach, predicted the mass of a suspected peptide and then, regardless of parent ion signal, acquired tandem mass spectrum at that calculated m/z value. Our own prior work to maximize analytical sensitivity in an ion trap also pointed to this outcome when we were able to generate peptide tandem mass spectra from barely detectable precursor ions19.
Conclusions
In summary, we have shown that a systematic, data-independent acquisition strategy outperforms data-dependent shotgun proteomics acquisition even with previously optimized use of genome-based gas-phase fractionation for a similar number of LC-MS/MS experiments. Our data showed that we were able to observe the major fraction (70% or more) of expressed proteins from a larger prokaryotic organism (Pseudomonas aeruginosa) without prior protein fractionation or enrichment by standard liquid phase methods. Dynamic range is highly increased with our PAcIFIC strategy due to the fact that very narrow m/z ranges are concentrated in the ion trap regardless of whether a precursor ion is present thus maximizing ion concentration prior to CID. Sample consumption of ~100 g of protein utilized for a full PAcIFIC analysis in triplicate was similar to the amount commonly used by other approaches that include liquid-phase protein or peptide fractionation techniques. Similarly, less than 100μl of plasma is necessary to perform a triplicate PAcIFIC analysis making it immediately amenable to clinical studies where access to a large amount of sample is not feasible. Additionally, given the very deliberate nature of PAcIFIC in scanning every m/z channel, total analysis time is also quite similar. We note also that PAcIFIC works with standard instrumentation and software, making the method readily adaptable and easily distributed between laboratories. An immediate advantage of PAcIFIC is to reduce sample preparation to a minimum prior to fully automated LC-MS/MS operation. A secondary benefit is that all possible peptides available in a sample for multiple reaction monitoring may be mapped out in a single PAcIFIC analysis. Finally, these results show the potential outcome one could obtain with faster scanning instruments or hybrid multi-dimensional mass spectrometers27 that subject each ion in the ion trap to CID while maintaining precursor ion-fragment ion lineage. Currently a single PAcIFIC analysis takes five days for 67 injections. Our recent efforts to optimize the m/z range utilized for CID suggest that the same data discussed herein can be conducted in approx three days or 45 injections. Finally, with new ion trap instruments that incorporate ion funnels28 or ion funnel-like devices, e.g. the Velos (Thermo Fisher Scientific) which provides a 10Hz scanning rate, a single PAcIFIC analysis will easily be achieved in one to two days, making it a fast, highly-automated and reproducible strategy for complete proteome analysis.
Experimental section
Materials
All reagents and solvents were of highest available purity. The P. aeruginosa sample was prepared, digested and desalted as previously described10. The plasma sample was prepared as follows. 100ul of human plasma from a healthy donor was depleted of the 7 most abundant proteins using a MARS-7 spin cartridge (Cat N° 5188–6408 and 5188–5254, Agilent technologies, Santa Clara, CA) according to the manufacturer's instructions. Depleted plasma was then concentrated in a spin concentrator (5K MWCO, cat N° 5185–5991, Agilent technologies, Santa Clara, CA) and buffer exchanged with 100 mM ammonium bicarbonate containing 6M urea to a final volume of 170 ul. Proteins were further reduced by the addition of 4ul 200mM dithiothreitol for 1h at 37°C and then alkylated with 17 ul 200mM iodoacetamide. 400 ul of 100 mM ammonium bicarbonate buffer was added to the reduced/alkylated protein mixture before the addition of 10ug of trypsin (1ug/ul). A total of 750 ug of protein digest was then desalted on three C18 macrospin columns (30–300 ug capacity, cat N°SMM SS18V, The Nest Group, Southborough, MA) according to the manufacturer's protocol, the three eluates pooled and stored at −80°C before mass spectrometry analysis.
Mass spectrometry and HPLC
HPLC was performed on a Waters NanoAcquity (Manchester, UK) system using homemade columns and precolumns and the same chromatographic conditions as previously describe10. An estimated amount of 0.5 ug of bacterial peptides and 2.0 ug of plasma peptides was used for each sample injection. An LTQ-Orbitrap XL from ThermoFisher (San Jose, CA) was used for mass spectrometry. For precursor-ion independent acquisition (i.e. PAcIFIC), the tandem mass spectra were acquired in the LTQ (one microscan per spectrum). The maximum injection time was 100 ms and the target ion population was 104. Data-dependent ion selection across the entire available m/z range and data-dependent ion selection used with genome-based gas-phase fractionation was used as previously described10. PAcIFIC acquisition schemes were used as following: 10 consecutive MS/MS scans of m/z = 401; 402.5; 403; 404.5; 406; 407.5; 409; 410.5; 412; 413.5 with an isolation width set to 2.5 and collision energy to 35%. In the consecutive range, m/z values from 415 to 428.5 were analyzed, etc. until m/z = 1403.5 was reached (67 repeated injection in total).
Data processing
For each PAcIFIC replicate from either the bacterial sample or the plasma sample, RAW files were converted to mzXML files (67 files in total) and searched withSequest29 against the Pseudomonas protein database (http://www.pseudomonas.com/download.jsp) or the IPI human database v3.43 (http://www.ebi.ac.uk/IPI/IPIhuman.html).The precursor tolerance was set to 3.75 Da with alkylated cysteines set as fixed modification and oxidized methionine as variable. Sequest results were converted to pepXML files and probability assessments of identified peptides were computed with Peptide Prophet (ISB, Seattle, WA). For all individual searches (67 pepXML files or 1 replicate), peptides with probability scores equal or higher than 0.99 were used (estimated error rate 0.2%). When two or more technical replicates were merged, probability scores equal or higher than 0.95 were used (estimated error rate 0.5%). Typical sensitivity/error plots are shown in supplementary figure 2. Genome-based gas-phase fractionation datasets were searched as previously described10
So called “housekeeping proteins” were selected using the following rules: First, only soluble proteins (containing no transmembrane domain) with two or more protéotypic peptides were selected. Then, genes coding for non-hypothetical proteins and annotated as being part of the functional groups “Amino acid biosynthesis and metabolism”, “Carbon compound catabolism”, “DNA replication”, “Biosynthesis of cofactors, prosthetic groups and carriers”, “Energy metabolism”, “Nucleotide biosynthesis and metabolism”, “Cell division”, “Cell wall, LPS and capsule”, “Central intermediary metabolism”, “Fatty acid and phospholipid metabolism”, “Protein secretion/export apparatus” and “Transcription, RNA processing and degradation” were selected. This resulted in a list of 661 potential “housekeeping proteins”.
Supplementary Material
1_si_001
2_si_002
3_si_003
4_si_004
Acknowledgments
DRG thanks the following National Institutes of Health (NIH) agencies for support: R33CA099139-01, 1S10RR023044-01 and 1U54 AI57141-01. AP acknowledge the Swiss National Science Foundation (SNF) for support (PBLAA—119623). This work was supported in part by the University of Washington's Proteomics Resource (UWPR95794).
References
Full text links
Read article at publisher's site: https://doi.org/10.1021/ac900888s
Read article for free, from open access legal sources, via Unpaywall:
https://europepmc.org/articles/pmc3086478?pdf=render
Citations & impact
Impact metrics
Article citations
Deep Plasma Proteomics with Data-Independent Acquisition: Clinical Study Protocol Optimization with a COVID-19 Cohort.
J Proteome Res, 23(9):3806-3822, 19 Aug 2024
Cited by: 0 articles | PMID: 39159935 | PMCID: PMC11385417
Comprehensive Overview of Bottom-Up Proteomics Using Mass Spectrometry.
ACS Meas Sci Au, 4(4):338-417, 04 Jun 2024
Cited by: 2 articles | PMID: 39193565 | PMCID: PMC11348894
Review Free full text in Europe PMC
Data-Independent Acquisition: A Milestone and Prospect in Clinical Mass Spectrometry-Based Proteomics.
Mol Cell Proteomics, 23(8):100800, 15 Jun 2024
Cited by: 0 articles | PMID: 38880244 | PMCID: PMC11380018
Review Free full text in Europe PMC
Acquisition and Analysis of DIA-Based Proteomic Data: A Comprehensive Survey in 2023.
Mol Cell Proteomics, 23(2):100712, 03 Jan 2024
Cited by: 4 articles | PMID: 38182042 | PMCID: PMC10847697
Review Free full text in Europe PMC
Robust, Precise, and Deep Proteome Profiling Using a Small Mass Range and Narrow Window Data-Independent-Acquisition Scheme.
J Proteome Res, 23(3):1028-1038, 26 Jan 2024
Cited by: 2 articles | PMID: 38275131 | PMCID: PMC10913089
Go to all (131) article citations
Data
Data behind the article
This data has been text mined from the article, or deposited into data resources.
BioStudies: supplemental material and supporting data
Similar Articles
To arrive at the top five similar articles we use a word-weighted algorithm to compare words from the Title and Abstract of each citation.
Precursor ion independent algorithm for top-down shotgun proteomics.
J Am Soc Mass Spectrom, 20(11):2154-2166, 13 Aug 2009
Cited by: 31 articles | PMID: 19773183
Faster, quantitative, and accurate precursor acquisition independent from ion count.
Anal Chem, 83(6):2250-2257, 22 Feb 2011
Cited by: 44 articles | PMID: 21341720 | PMCID: PMC3217585
Multiplexed and data-independent tandem mass spectrometry for global proteome profiling.
Mass Spectrom Rev, 33(6):452-470, 26 Nov 2013
Cited by: 121 articles | PMID: 24281846
Review
Apex peptide elution chain selection: a new strategy for selecting precursors in 2D-LC-MALDI-TOF/TOF experiments on complex biological samples.
J Proteome Res, 9(11):5922-5928, 30 Sep 2010
Cited by: 1 article | PMID: 20836568
Funding
Funders who supported this work.
NCI NIH HHS (3)
Grant ID: R33CA099139-01
Grant ID: R33 CA099139-02
Grant ID: R33 CA099139
NCRR NIH HHS (3)
Grant ID: S10 RR023044
Grant ID: 1S10RR023044-01
Grant ID: S10 RR023044-010001
NIAID NIH HHS (3)
Grant ID: U54 AI057141
Grant ID: U54 AI057141-01
Grant ID: 1U54AI57141-01