Abstract
Free full text

Emerging patterns of somatic mutations in cancer
Abstract
The advance in technological tools for massively parallel, high-throughput sequencing of DNA has enabled the comprehensive characterization of somatic mutations in large number of tumor samples. Here, we review recent cancer genomic studies that have assembled emerging views of the landscapes of somatic mutations through deep sequencing analyses of the coding exomes and whole genomes in various cancer types. We discuss the comparative genomics of different cancers, including mutation rates, spectrums, and roles of environmental insults that influence these processes. We highlight the developing statistical approaches used to identify significantly mutated genes, and discuss the emerging biological and clinical insights from such analyses as well as the challenges ahead translating these genomic data into clinical impacts.
Introduction
While it is recognized that cancer is a collection of complex pathological entities with diverse biological capabilities1, much of our current understanding of cancer genetics is grounded on the principle that cancer arises from a clone that has accumulated the requisite somatically-acquired genetic aberrations, leading to malignant transformation2. Progression of such a transformed clone to disseminated disease has previously been thought to be a linear process driven by serially acquired new mutations, including base pair substitutions, small insertions and deletions (indels) of bases, chromosomal rearrangements, and gains and losses in gene copy number3. However, recent insights have emerged from comprehensive genomic characterization using next-generation sequencing (NGS) technologies, which has allowed for the sequencing of the coding portion of the genome through hybrid-capture whole-exome sequencing (WES) or nearly all base pairs in a tumor-normal pair by whole-genome sequencing (WGS) (Box 1) (reviewed in4,5), revealing unanticipated complexities in the patterns of somatic alterations in cancers.
Prior to the more widespread use of NGS technologies, studies using PCR-amplification and Sanger-based capillary sequencing methodology were limited by cost and throughput, dictating either one of two study designs: a limited number of genes sequenced in a large cohort or all coding genes sequenced in a small number of samples. Mutations in many of the known cancer genes, so-called ‘mountains’ (genes altered in a high percentage of tumors), were discovered by focused sanger sequencing or cytogenetic analyses, some of which express mutated proteins that have become successful drug targets2,4. The first efforts to interrogate the complete protein coding sequence of the cancer genome of colon, breast and glioblastoma necessitated the generation of up to 208,311 primer pairs6,7 for Sanger-based sequencing. These pioneering studies led to the identification of a highly recurrent mutation in a novel oncogene, isocitrate dehydrogenase 1 (IDH1)6, involved in both cell metabolism8,9 and DNA methylation10, reinforcing the promise of unbiased genomic sequencing in the identification of novel genetic driving events in human cancers.
The advancement in NGS technology has allowed the cancer research community to employ systematic sequencing to identify additional ‘mountains’, which include the discovery of frequent mutations in epigenetic regulators and pre-mRNA splicing machinery in many cancers (Figure 1 and described in detail below), and so-called ‘hills’ (genes altered less frequently in cancer). Furthermore, these efforts have uncovered various mutational mechanisms underlying tumorigenesis and progression, such as chromothripsis11,12, chromoplexy13,14 and kategeis15-17. Following the launch of the Cancer Genome Project (CGP) in the United Kingdom in 2000 and The Cancer Genome Atlas (TCGA) in the United States in 2006, the International Cancer Genome Consortium (ICGC) was created in 2007 to coordinate the generation of comprehensive catalogues of genome alterations from 52 different cancers18. To date, disease working groups from TCGA and ICGC have released comprehensive genomic analyses for a number of cancer types19-33. In addition, a major ongoing effort is to molecularly characterize rare malignancies and cancers that are common in diverse geographical regions in different populations, such as gastric cancer34,35, viral hepatitis (B or C) associated hepatocellular carcinoma (HCC)27,36-38, and parasite-induced cholangiocarcinoma (CCA)39.
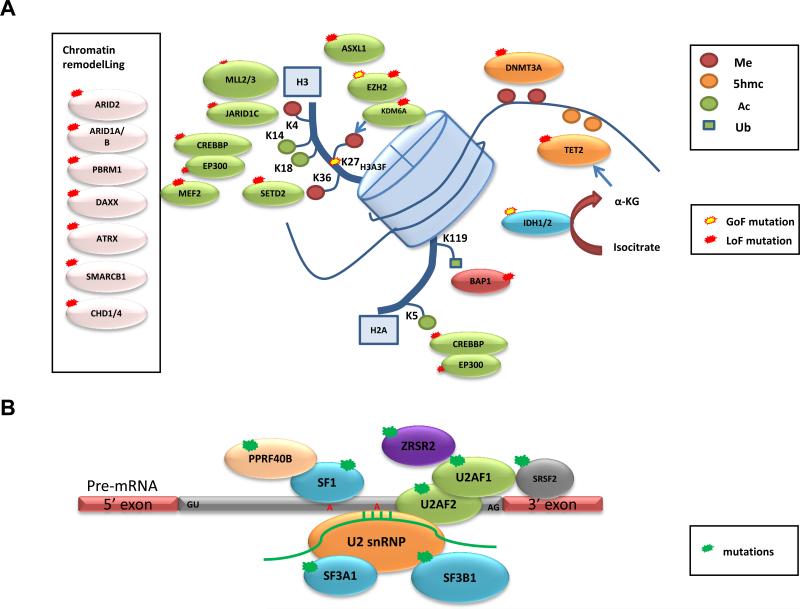
Representative genes involved in (A) chromatin remodelling, histone and DNA methylation (Me), acetylation (Ac), and ubiquitination (Ub), and (B) pre-mRNA splicing that were identified to be recurrently mutated in various types of cancers by whole-exome and whole-genome sequencing are displayed. Predicted consequences of mutations, either gain of function (GOF) or loss of function (LOF), are also shown.
The general approach to sequencing and bioinformatics analysis, as well as the potential of large-scale cancer genomics by NGS, has been discussed in depth in recent review articles2,4,5,40,41. Here, we attempt to keep pace with the explosion of NGS studies by summarizing a selection of findings from recently published genomic studies (2008 to July 2013), with a focus on analysis of genes targeted by point mutations (base pair substitutions) and indels. We review WES and WGS studies that have provided the clearest landscape of somatic mutations in major adult tumor types (majority of which involve deep sequencing analyses of the coding exomes and whole genomes of 20 or more samples per study), TCGA and ICGC publications, as well as a few examples of rare malignancies and cancers more prevalent in diverse geographical regions (a selection of studies is summarized in Table 1). We discuss our current understanding of the mutational landscapes of these different tumor types and underscore the biological insights into the etiology of cancer gained through integrative and pathway analysis with various genomic platforms, while highlighting the challenges of identifying driver mutations and functional validation of significantly mutated genes (SMGs). While structural rearrangement can also be identified through NGS, the analytics for defining such structural alterations are much less matured, thus we will not be covering this important aspect of genomic alterations in detail in this review.
Table 1
Overview of example cancer genomics studies for different cancer types.
Cancer type | Mutation analysis: # of samples | Highlighted or novel mutated genes | References |
---|---|---|---|
Glioblastoma | PCR amplify and sequenced 20,661 genes: 22 | IDH1 • † | 6 |
WES: 48 (6 matched normal DNA) *pediatric GBM | H3F3A•†, ATRX/DAXX#, TP53 | 118 | |
ccRCC | WES: 7 | PBRM1# | 47 |
WGS: 1 | BAP1# | 53 | |
WES: 106 WGS: 14 | TCEB1•#, KEAP1#, TET2, mTOR† | 55 | |
WES: 417 WGS: 22 | TCEB1•#, NFE2L2†, ARID1A#, mTOR† (Confirmed PBRM1#, SETD2#, KDM5C#, PTEN#, BAP1#, TP53, PIK3CA•†) | 19 | |
HNSCC | WES: 32 | NOTCH1# | 56 |
WES: 74 | NOTCH1# | 57 | |
HGS-OvCa | WES: 316 | TP53 (universal) | 25 |
Melanoma | WGS: 25 | PREX2a (see Box 4) | 61 |
WES: 121 | RAC1•†, PPP6C•, STK19•, ARID2# | 43 | |
WES: 147 (99 matched normal DNA) | RAC1•†, PPP6C•#, ARID2# | 62 | |
Lung AC | WES: 159 WGS/WES: 23 WGS : 1 | U2AF1•†, RBM10#, ARD1A# | 64 |
Lung SCC | WES: 178 | HLA-A#, NFE2L2†, KEAP1(#) | 26 |
Small-cell lung cancer | WES: 53 | SOX family (+22 SMGs identified) | 65 |
Prostate | WES: 112 | SPOP, FOXA1, MED12• | 68 |
WES: 50 | CHD1#, MLL2, FOXA1† | 69 | |
Colorectal | WES: 224 | ARID1A#, SOX9#, FAM123B# | 22 |
WES: 72 WGS: 2 | TET family, ERBB3†, ATM# | 70 | |
Gastric | WES: 22 | ARID1A# | 34 |
WES: 15 | FAT4#, ARID1A#, MLL3, MLL | 35 | |
Breast | WES: 54 WES/WGS: 4 WGS:15 | Confirmed TP53, PIK3CA•†, RB1#, PTEN# | 75 |
WES: 31 WGS: 46 | MAP3K1#, CDKN1B#, TBX3, RUNX1, LDLRAP1, STNM2, MYH9, AGTR2, STMN2, SF3B1• and CBFB# | 73 | |
WES: 100 | AKT2†, ARID1B#, CASP8#, CDKN1B#, MAP3K1#, MAP3K13#, NCOR1#, SMARCD1#, TBX3 | 31 | |
WES: 86 WES/WGS: 17 WGS: 5 | CBFB# | 72 | |
WES: 510 | GATA3•, PIK3CA•†, MAP3K1#, MAP2K4# (BOX3) | 23 | |
PDAC | WES: 142 | EPC1, ARID2, ATM, ZIM2, MAP2K4, NALCN, SLC16A4, MAGEA6, axon guidance pathway genes (SLIT/ROBO signaling) | 21 |
HCC | WES: 10 | ARID2# | 37 |
WES: 24 | ARID1A#, RPS6KA3#, NFE2L2†, IRF2# | 36 | |
WGS: 27 | ARID1A#, ARID1B#, ARID2#, MLL#, MLL3# | 27 | |
WES: 10 | ARID1A# | 38 | |
CCA | WES: 8 | TP53, KRAS†, SMAD4#, MLL3#, ROBO2#, RNF43#, PEG3#, GNAS•† | 39 |
AML | WGS: 24 | SMC3#, SMC1A#, STAG2#, RAD21# | 88 |
WGS: 50 WES: 150 | Confirmed 23 SMGs; FLT3•†, NPM1, DNMT3A•#, IDH1/2•†, TET2#, RUNX1*#, TP53#, NRAS*†, CEBPA#, WT1#, PTPN11†, KIT*†, U2AF1, KRAS•†, SMC1A#, SMC3#, PHF6#, STAG2#, RAD21#, FAM5C, EZH2•#, HNRNPK | 33 | |
MDS | WES: 29 | SF3B1•, SRSF2•, U2AF1•, ZRSR2, SF3A1, PRPF40B, U2AF2, SF1 | 89 |
WES: 9 | SF3B1• | 28 | |
CLL | WES: 5 | NOTCH1 • † | 94 |
WGS: 4 | NOTCH1•†, MYD88•†, XPO1•†, KLHL6 | 29 | |
WES: 88 WGS: 3 | SF3B1• | 92 | |
WES: 105 | SF3B1• | 30 | |
DLBCL | WES: 6 | MLL2#, CREBBP#, EP300# | 100,101 |
WES or WGS: 13 | MLL2#, MEF2B • † | 98 | |
WES: 55 | MEF2B•†, MLL2#, BTG1, GNA13, ACTB, P2RY8, PCLO, TNFRSF14#, BCL2# | 99 | |
MM | WES: 16 WGS: 23 WES/WGS: 1 | DIS3#, FAM46C, LRRK2, BRAF•†, IRF4†, 11 NFκB pathway genes | 103 |
Summarized information of whole-exome (WES) and whole-genome sequencing (WGS) studies of selected publications with highlighted novel mutated genes indicated. ccRCC = clear cell renal cell carcinoma, HNSCC = head-and-neck squamous cell carcinoma, HGS-OvCa = high grade serous ovarian carcinoma, HCC = hepatocellular carcinoma, AML = acute myeloid leukemia, MDS = myelodysplastic syndromes, CLL = chronic lymphocytic leukemia, DLBCL = diffuse large B cell lymphoma, MM = multiple myeloma, MSI = microsatelite instable, MSS = microsatelite stable. Predicted or reported consequences of mutations are shown: •hotspot identified, †activating, or likely activating #inactivating, or likely inactivating.
Features of somatic mutation genomic studies
Mutation rates and spectrum across cancer types
A meta-analysis of 2,957 whole exomes and 126 whole genomes from 27 cancer types performed at the Broad Institute, recently illustrated the mutational heterogeneity in diverse cancer types42. First, the variation in mutation frequency can partly be explained by cancer type. For example, the mutation rates for pediatric and haematological cancers possess the lowest mutation rates (~1 mutations/Mb for chronic lymphocytic leukemia (CLL))30, compared to cancers where environmental mutagens are known to increase the mutation burden, such as melanoma and lung cancer (~15 mutations/Mb for melanoma)43. In addition, mutation rates can vary tremendously within a cancer type, often due to the degree of exposure to an environmental mutagen, or dependent on which genes are mutated (e.g. tumors possessing mutations in mismatch repair genes). Second, the mutation spectrums also vary across cancer types. For example, clustering analysis on all possible mutations (considering context of flanking residues) demonstrated natural groupings of mutation spectrum and cancer types consistent with known signatures of carcinogenesis mechanisms: lung tumors possess a high fraction of G>T transversions, attributable to exposure of polycyclic aromatic hydrocarbons from tobacco smoke; melanomas possess a high fraction of C>T transitions in dipyrimidines caused by UV-induced DNA damage and misrepair; gastrointestinal tumors (oesophageal, colorectal and gastric) possess a high frequency of transition mutations at CpG dinucleotides that may be a reflection of elevated methylation levels in these tumors22; cervical, bladder, some head-and-neck and breast cancers possess frequent mutations at Cs in the context of TpC, characteristic mutations caused by the APOBEC family of cytidine deaminases15-17; and leukemic samples (acute myeloid leukaemia (AML) and CLL) possess A to T mutations in the TpA context42. Finally, analysis of WGS confirmed mutational heterogeneity across the genome that is heavily influenced by two factors: gene expression level and DNA replication time. In light of this known mutational heterogeneity, correcting for such factors has been shown to be important in determining SMGs42,43.
Approaches to identify cancer-associated genes
Identifying which mutations are likely to be ‘drivers’ in pathogenesis and elucidating how mutated genes affect the biology of a given tumor, are fundamental challenges in cancer genomics. Statistical tools to identify SMGs that possess a higher mutation rate than the expected (calculated/estimated) background mutation rate (BMR), indicating positive selection during tumorigenesis have been developed by a number of groups (Box 2). Following the recent surge of WES and WGS studies, a more comprehensive census of human cancer genes has emerged. However, this is just the first step in the translation of these findings to clinical benefits for patients. Additionally, many of the published studies have collected data on other genomic dimensions (e.g. DNA copy number, DNA methylation, mRNA and miRNA expression profiles), which can be used for integrative analysis (Box 3) and for elucidating mechanisms of disease pathogenesis; we highlight a few informative examples of such integration in the sections on specific cancers.
Landscape of somatic mutations in solid tumors
Glioblastoma
The Sanger-sequencing of 20,661 protein coding genes from 22 glioblastoma samples mentioned above revealed a recurrent heterozygous IDH1 mutation targeting amino acid R132 in 12% of samples, which correlated with improved patient survival6. In parallel, glioblastoma was the first cancer to undergo comprehensive genomic characterization by TCGA Research Network, which used a targeted approach consisting of Sanger-based capillary sequencing of 601 selected genes in 91 glioblastoma tumor–normal pairs to identify somatic mutations, which revealed frequent mutations in the phosphatidylinositol 3-kinase (PI3K) regulatory subunit, PIK3R124. The targeted sequencing was complemented by analyses of DNA copy number, mRNA expression and DNA methylation from 206 glioblastomas. Integrative analysis demonstrated statistically significant deregulation of the RTK–RAS–PI3K, p53, and RB signaling pathways in glioblastoma24. This study set the framework for comprehensive multi-dimensional genomic characterization in large-scale studies (Box 3). Investigation of RNA sequencing (RNA-seq) data from glioblastomas also revealed translocations involving fibroblast growth factor receptor (FGFR) genes to the transforming acidic coiled-coil (TACC) coding domains of TACC1 or TACC3, in approximately 3% (3/97) of cases44. Glioblastoma cells expressing the fusion demonstrated sensitivity to FGFR kinase inhibition in preclinical assays44. The examples above demonstrate the power of unbiased genomic sequencing in the identification of clinically relevant genetic changes.
Clear-cell renal cancer
Somatic or germline inactivating mutations in the tumor suppressor gene, von Hippel–Lindau (VHL), a master regulator of HIF transcription factors and the hypoxia response, are found in most clear-cell renal-cell carcinoma (ccRCC) cases (somatic mutations reported in >55% of sporadic ccRCC)45,46. However, studies from model organisms have pointed to the requirement for additional genetic alterations to drive tumor development. Indeed, focused Sanger sequencing revealed mutations in neurofibromin 2 (NF2) and in genes involved in histone methylation and demethylation: SETD2, JARID1c (also known as KDM5C) and UTX (also known as KMD6A)46 (Box 3 and Figure 1a). A follow-up WES analysis led to the identification of the second most frequently mutated gene in ccRCC, polybromo 1 (PBRM1)47, which possessed truncating mutations in 41% (92/227) of cases. PBRM1 encodes for the chromatin-targeting subunit of the PBAF SWI/SNF chromatin remodeling complex, which regulates transcription and has essential roles in maintenance of stem pluripotency (reviewed in48) (Figure 1a). The importance of the PBAF complex was initially suggested from studies that found inactivating mutations in its core component, SMARCB1 (SNF5), in rhabdoid tumors49,50. PBRM1 mutations were found in the context of loss of heterozygosity, and functional data supported its role as a tumor suppressor, suggesting PBRM1 represents the second major genetic event that cooperates with VHL in ccRCC47. A second WES study identified frequent mutations in the two-hit tumor suppressor BRCA1-associated protein-1 (BAP1) in ccRCC, previously found to be frequently mutated in uveal melanoma51 and pleural mesothelioma52, that functions as a deubiquitinating enzyme and regulator of histone H2A lysine 119 ubiquitination53 (Figure 1a). Interestingly, mutations in BAP1 and PBRM1 were anti-correlated, and tumors possessing BAP1 mutations were associated with high tumor grade53,54. Recent efforts from a larger WES/WGS study55 and TCGA19 confirmed PBRM1, SETD2, KDM5C and BAP1 to be significantly mutated in a cohort of 417 ccRCC tumors, as well as a novel hotspot mutation in a component the VHL E3 ligase complex, TCEB119,55. Importantly, TCGA was able to show widespread DNA hypomethylation in SETD2-mutant tumors and transcriptional network analysis suggest mutations in the chromatin remodeling complex (PBRM1, ARID1A, and SMARCA4) are linked to RAS signaling, immune function, DNA repair, β-catenin and TGF-β signaling. RNA-seq analysis also revealed recurrent SFPQ–TEF3 fusions found by in 5/416 samples, all five lacking a VHL mutation19.
Head-and-neck squamous cell carcinomas (HNSCCs)
A first global view of the somatic mutations in HNSCC was published in two studies in 2011, based on WES characterization of 32 and 74 HNSCC tumor–normal pairs, respectively56,57. Epidemiologically, tobacco use, alcohol consumption, and infection with human papilloma virus (HPV) are known major risk factors for HNSCC. These studies reported that tumors isolated from patients with a history of tobacco use possessed higher mutation rates than those from non-smokers, that HPV-associated tumors possessed far fewer mutated genes, and that mutations in TP53 and HPV infection were mutually exclusive56,57. These observations demonstrate how environmental causes of cancer leave footprints in the genome, and these may offer hints to the molecular mechanism of pathogenesis.
In terms of genes that are frequently mutated in HNSCC, Agarwal et al.56 identified 6 recurrently mutated genes based on the frequency of mutations, and Stransky et al.57 defined 39 SMGs using the MutSig algorithm (Box 2; Supplementary Figure 1). Stransky et al. also looked for enrichment in functional gene sets among the list of SMGs and discovered that the highest scoring set was involved in epidermal development, particularly in squamous cell differentiation, pointing to disruption of the stratified squamous differentiation program as a candidate route to HNSCC.
Both studies identified previously unrecognized NOTCH1 mutations in HNSCC in approximately 10-15% of samples. Although oncogenic activating NOTCH1 mutations have been observed in a number of hematological malignancies29, the mutations in NOTCH1 identified in HNSCC possessed characteristics indicative of loss of function (LOF) mutations, hence suggesting a tumor-suppressive role in this cancer. Phenotypes observed in mice are consistent with the idea that Notch1 can function as a tumor suppressor58. γ-secretase inhibitors target NOTCH downstream signaling, but the clinical development of an inhibitor in humans has been halted, partly due to an increased association with skin cancer risk59. This example highlights the increasingly recognized context-dependent nature of cancer gene functions and that different mutations of the same gene likely confer different cancer-relevant biological activities (Box 4), adding to the complexity of applying genomic information in therapeutic decisions.
Ovarian cancer
Integrated analysis of high-grade serous ovarian adenocarcinoma (HGS-OvCa) by TCGA included mRNA, miRNA, promoter methylation and DNA copy number analyses from 489 tumors as well as WES data on 316 of these samples25. Although 9 SMGs were identified using two algorithms (MutSig and MuSiC), the landscape of somatic mutations in this cancer type was dominated by near universal presence of TP53 mutations (found in 96% of samples). However, there was also evidence for substantial complexity, with multiple infrequently SMGs identified (Supplementary Figure 1). The HGS-OvCa genomes had many somatic copy-number aberrations (SCNAs), with 113 statistically significant recurrent SCNAs as defined by the statistical approach, GISTIC. This level of SCNAs was greater than that for other reported TCGA studies (for glioblastoma, colon, breast and lung cancers)22-26.
Melanoma
The discovery of the BRAF V600E mutation in over 50% of melanomas in 200260 and the subsequent development of an inhibitor to treat patients with BRAF-mutant metastatic disease is the proof-of-concept for genomics-informed personalized therapy. It is known that an additional 20% of melanomas are characterized by recurrent NRAS hotspot mutations; however, the driver mutations in the remaining melanoma cases remain poorly understood.
The search for additional driver mutations in melanoma has been complicated by the fact that melanoma has the highest basal mutation rate of any cancer sequenced to date43,61,62, which can be almost entirely attributable to the abundance of UV-induced C>T transitions in dipyrimidines. As a result, identifying SMGs is highly susceptible to discovery cohort bias. To overcome this challenge, statistically powered discovery cohorts of many patients and modified analytics that take into account this high basal mutation rate have been needed to make sense of the mutation data for this tumor type, as demonstrated by two recent studies that analyzed WES data of cohorts of >100 tumor–normal pairs43,62 (Supplementary Figure 1). For example, an algorithm called InVEx43 was developed to identify SMGs (Box 2). This method led to the identification of several novel SMGs, many of which harbored hotspot mutations, a pattern of mutation that signifies strong biological selection. One of these was a hotspot mutation in the Rho GTPase, RAC1, identified in both studies at a frequency of 4–9%43,62. This example illustrates that different numbers of samples and analytical algorithms are required for different tumor types based on the status of the cancer genome.
Lung cancer
Lung cancer is classified into two major histological types: small-cell lung cancer (SCLC) and non-small-cell lung cancer (NSCLC). NSCLC is further subdivided into squamous cell carcinoma (SCC), adenocarcinoma (AC) and large-cell carcinoma subtypes. Significant progress in personalized treatment for this disease has been made in recent years with the demonstration that epidermal growth factor receptor (EGFR)-activating mutations or gene fusion events involving the receptor tyrosine kinase gene ALK identify NSCLC patients who are responsive to inhibitors of these tyrosine kinases (reviewed in63).
Recently, WES and WGS data have provided a more detailed view of the somatic mutational landscape of various lung cancer subtypes: a combination of WES and WGS on 183 lung adenocarcinoma tumor–normal DNA pairs64, an integrated analysis of 178 lung SCCs26, and WES of 53 SCLC samples65. These studies showed that lung cancer possesses the second highest reported mutation rate after melanoma (mean mutation rate as high as 12.9 mutations/Mb for smokers), with strong mutation signatures associated with tobacco smoke exposure (i.e. G>T transversions and C>T transitions in the setting of CpG dinucleotides)26,64-66. In one study, the InVEx algorithm was used to address the challenge of this high mutation rate and 25 SMGs were identified64 (Supplementary Figure 1). The authors found that mutations in U2 small nuclear RNA auxiliary factor 1 (U2AF1) (Figure 1b) and TP53 correlated negatively with progression-free survival. Together with frequent mutations in RNA binding motif protein 10 (RBM10), the mutations in U2AF1 point towards a role for RNA splicing deregulation in lung cancer as seen in a number of hematological malignancies (Figure 1b and described in detail below).
A modified version of MutSig was used26, which took into account gene expression level, GC content, local gene density, and local relative replication time, to identify 10 SMGs in the lung SCC TCGA study (Box 2; Supplementary Figure 1). These included previously unreported LOF mutations in HLA-A and widespread TP53 mutations in nearly all samples analyzed26. Integrative analysis identified pathways frequently deregulated in lung SCC, which included confirmation of the oxidative stress response due to frequent mutations and SCNAs in the CUL3 and KEAP1 components of an E3 ubiquitin ligase and its target substrate, NFE2L2, in 34% of samples26 (Box 3). Finally, Rudin et al. took into account gene expression levels in their significance analysis to identify 22 SMGs (Supplementary Figure 1), including frequent mutations and SCNAs in SOX family members not previously recognized in SCLC65. This series of analyses nicely illustrates the genomically distinct nature of these three subtypes of lung cancer despite their common organ site involvement, heralding the need for genomic classification to complement traditional histopathological diagnosis.
Prostate cancer
Frequent chromosomal rearrangements of ETS transcription factor genes with androgen-responsive promoters, commonly through TMPRSS2–ERG fusions, were discovered as driver events in up to 50% of prostate cancers in 200567. Recurrent somatic point mutations were thought to play a less important role in prostate tumorigenesis, until two WES studies shed light on novel SMGs in this disease. An analysis68 of WES data from 112 treatment-naїve prostate adenocarcinoma–normal pairs identified 12 SMGs (Supplementary Figure 1); notably, SPOP, a substrate-binding subunit of a Cullin-based E3 ubiquitin ligase complex, was mutated in ~13% of samples. A WES analysis69 on 50 lethal, heavily pre-treated castration-resistant prostate cancers (CRPCs) and 11 treatment-naїve, high-grade localized prostate cancers identified 9 SMGs. Integrating WES and copy-number data found CHD1, an ATP-dependent chromatin-remodeling enzyme, to be frequently altered (Figure 1a). Interestingly, both SPOP-mutant and CHD1-deleted tumors lacked ETS-family gene rearrangements, thus demonstrating how molecular characterization of sizeable tumor cohorts can help identify novel genetic events defining a molecularly distinct subset of a tumor type.
Colorectal and gastric cancer
A molecular characterization of colorectal carcinoma (CRC) by TCGA analyzed WES, DNA copy number, promoter methylation, mRNA and miRNA expression from >200 samples22. Interestingly, the somatic mutation rates varied considerably among samples. Approximately 16% of tumors were designated as hypermutated (having >12 mutations/Mb). These were characterized by high levels of microsatellite instability (MSI), frequent epigenetic silencing of the DNA mismatch-repair pathway gene, MLH1, mutations in other mismatch repair genes or a DNA polymerase catalytic subunit, POLE, providing molecular insights into the underlying causes for the elevated mutation rate. Interestingly, the hypermutated samples possessed few SCNAs and harbored frequent concurrent BRAF V600E mutations. After removal of non-expressed genes, MutSig and prior biological knowledge were used to identify 15 mutated genes in the hypermutated cancers, and 17 in the non-hypermutated samples, thought to be important for CRC (Supplementary Figure 1). The two signature genes of CRC, TP53 and the WNT signaling pathway antagonist APC, were found to be more frequently mutated in the non-hypermutated tumors. Across the hypermutated and non-hypermutated groups it was found that there was near universal deregulation (92–97%) of the WNT signaling pathway and the PI3K pathways (~50%). However, deregulation of the transforming growth factor-β (TGFβ) and RTK–RAS signaling pathway was observed more frequently in the hypermutated subtype.
In another study, WES analysis70 of 15 MSI and 57 microsatellite stable (MSS) colorectal cancer samples identified 23 SMGs in the MSS cohort. Interestingly, RNA-seq analysis discovered recurrent gene fusions predicted to produce functional proteins involving R-spondin family members, RSPO2 and RSPO3, in 3% (2/68) and 8% (5/68) of samples, respectively. The RSPO fusions were found to be mutually exclusive with APC mutations, and exogenous expression of plasmids encoding fusions were shown to activate WNT signaling in a human colon cancer cell line. In a separate WGS study71, a recurrent fusion of VTI1A and TCF7L2 (encodes a WNT signaling effector, TCF4 transcription factor) was found in 3/97 of colorectal cancer samples, and a colorectal carcinoma cell line harboring the fusion was shown to be dependent on its expression for anchorage-independent growth. These multiple findings of frequent deregulation of WNT signaling is consistent with other evidence of APC as an initiating event in CRC and points to various components of WNT signaling as drivers of this disease, particularly in the hypermutated subtype.
Gastric cancer has high prevalence in East Asia and WES analysis on >15 gastric tumors was performed in two studies34,35. Both studies identified cell adhesion/junction organization and chromatin modification as the most enriched pathways affected by SMGs (Supplementary Figure 1). Interestingly, a member of the SWI/SNF chromatin remodeling family, ARID1A, was found to be mutated more frequently and had decreased expression in MSI (83%) and Epstein–Barr virus (EBV)-infected MSS (73%) gastric cancers, compared to non-EBV-infected MSS cancers (11%)34. Alterations in ARID1A were also predictive of improved disease-free survival, suggesting deregulation of the SWI/SNF complex represents a unique mechanism of carcinogenesis associated with a distinct clinical behavior.
Breast cancer
Clinically, breast cancer is categorized into three basic groups: estrogen receptor (ER) and progesterone receptor (PR) positive; ERBB2 (also known as HER2) amplified; and triple-negative breast cancer (TNBC), which lacks ER, PR and ERBB2 overexpression. Recently, a number of large-scale WES and WGS studies of breast cancer have developed new algorithms to reconstruct the clonal evolution of the tumors (Box 5), shedding light on the mutational processes responsible for the generation of somatic mutations in breast cancer in addition to identifying SMGs that correlated with well-established clinically relevant subtypes16,23,31,72-75.
For example, WES/WGS analysis of 65 TNBC cases75 identified 6 SMGs, confirming TP53 as the most frequently mutated gene in this subtype. Clonal frequency analysis provided evidence that somatic mutations in TP53, PIK3CA and PTEN are clonally dominant in most tumors in which they are found, consistent with a founder mutation status role in most, but not all TNBCs.
The largest sequencing studies to date for breast cancer include WES of 79 ER+ and 21 ER-breast tumors31, WES of 103 and WGS of 22 breast tumors from diverse subtypes72, and the TCGA WES analysis of 510 breast tumors from 507 patients23. Although these studies used diverse approaches to understand the mutational landscape of breast cancer, they shared remarkable overlap in the SMGs that were identified (Supplementary Figure 1)23,31,72, including nearly all genes previously associated with breast cancer, as well as novel SMGs in the transcription factors TBX331 and CBFB72, and a recurrent, potentially druggable MAGI3–AKT3 fusion in 3.4% (8/235) of samples72.
Finally in a study of the responsiveness of ER+ breast cancers (of both the luminal A and luminal B expression subtypes) to estrogen deprivation, WES and WGS were performed on pretreatment tumor biopsies from patients who were subsequently treated with the neoadjuvant aromatase inhibitors73. The authors identified 18 SMGs by MuSiC, and found that GATA3 mutations correlated with response to aromatase inhibitor treatment. Furthermore, integrative analysis of mutations, mRNA expression and clinical attributes suggested that for patients with MAP3K1-mutant luminal A tumors, neoadjuvant aromatase inhibitors would prove a favorable option, but not for patients with TP53-mutant luminal B tumors73.
Pancreatic cancer
Recently, the ICGC published a WES and copy-number analysis from a prospectively accrued cohort of 142 early (stage I and II) sporadic pancreatic ductal adenocarcinoma (PDAC) samples21. MuSiC identified 16 SMGs (Supplementary Figure 1) and pathway analysis of these genes using GeneGO ascertained known cancer pathways (such as the G1/S checkpoint, apoptosis, and TGFβ signaling) as mechanisms important for PDAC development, as well as a novel pathway involved in axon guidance. Importantly, expression levels of two axon guidance genes, ROBO2 and ROBO3, were associated with patient survival21. Forward genetics (in the form of a Sleeping Beauty transposon mutagenesis screen in a mouse model of PDAC) and functional genomics (in the form of a shRNA screen in pancreatic cell lines) were also leveraged to explore the functional relevance of SMGs identified by sequencing. Thus, large-scale genomic analysis coupled with forward genetics and functional genomics can provide insights into pathways not previously linked to cancer.
Liver cancer
Hepatocellular carcinoma (HCC) has a strong association with chronic liver disease such as viral hepatitis (B or C) and aflatoxin B exposure, and is more prevalent outside of North America and Europe. Four recent genomic studies27,36-38 using WES and WGS data from discovery tumor cohorts varying from 4 to 27 samples have provided insights into the genetic drivers in HCC arising from various etiologies. All the studies confirmed previously known mutations in TP53 in HCC, but they also shed light on the importance of deregulation by somatic mutations of genes involved in chromatin remodeling, the WNT–β-catenin pathway, cell cycle control, the PI3K pathway, and oxidative and endoplasmic reticulum stress pathway27,36-38 (Supplementary Figure 1). Although most of the mutations were not associated with a specific type of chronic liver disease, in one study, mutations in interferon regulatory factor 2 (IRF2) were exclusively found in hepatitis B virus (HBV)-related tumors36. WGS has also revealed that the number of HBV integration sites in HCC tumors was associated with poor survival, and identified recurrent integration events in the TERT76, MLL4 and CCNE1 loci, which resulted in concurrent increase in gene expression27,77, reaffirming the powerful analytical capacity of NGS to investigate the potential role of pathogens in human cancers5.
One form of fatal hepatobiliary cancer that is highly prevalent in certain parts of Southeast Asia, is cholangiocarcinoma (CCA) associated with ingestion of the Opisthorchis viverrini parasite present in raw or undercooked fish. Interestingly, the biliary tree contains stem cell compartments for the liver, pancreas and bile duct, and analysis of WES from 8 Opisthorchis viverrini-related CCAs identified a mutational landscape that appeared more similar to PDAC than HCC (Supplementary Figure 1)39. This reinforces the importance of genomic classification in diagnosis, as we begin to understand cancers on a molecular level in addition to their organ-site and pathological features.
Landscape of somatic mutations in hematological malignancies
Myeloid malignancies
Genomic characterization of hematological malignancies has been at the forefront in the field of cancer genetics, and the most active in terms of clinical translation. Identification of gene fusions as the predominant drivers of certain leukemias has led to the development and clinical success of targeted therapies, including imatinib in chronic myeloid leukemia (CML) and acute lymphocytic leukemia (ALL) cases with the BCR–ABL1 fusion and all-trans-retinoic acid (ATRA) in acute promyelocytic leukemia (APL) cases with PML–RARA fusion. However, substantial numbers of leukemias do not possess such gene fusions, and the search for other genetic drivers led to the identification of somatic mutations in genes such as FLT3, RAS, CEBPA, KIT, JAK2, RUNX1, TET2, ASXL1, EZH2, and TP53 prior to the era of NGS-based studies78-82. The first WGS of a patient with French-American-British (FAB) classification M1 acute myeloid leukemia (AML) not only confirmed mutations in previously known genes, but led to the subsequent identification of new somatic mutations in genes involved in DNA methylation, such as DNA methyltransferase 3A (DNMT3A), IDH1, and IDH2 (Figure 1a)83-86.
Although there is some inter-patient heterogeneity, frequency of somatic mutations identified in myeloid luekemias is generally lower than that of solid tumors. The recent TCGA WES and WGS analysis of 200 patients of de novo acute myeloid leukemia (AML) revealed that an average of 13 coding mutations per sample (range: 0-51), and the mutation rate was even lower in cases that harbor known fusion variants, such as MLL-X or PML-RARA fusion33. In this study, MuSiC identified 23 SMGs that included known mutations in AML, as well as mutations in the mRNA splicing machinery (U2AF1) (Figure 1b) or cohesin complex (SMC1A, SMC3. STAG2, and RAD21) that were recently identified in myeloid leukemias (Supplementary Figure 2)87,88.
WES analyses of myelodysplastic syndromes (MDS) have shown that the mutation rate is similar to that of AML (median 9 mutations per sample), and share a similar spectrum of mutated genes (Supplementary Figure 2)28,89. However, some genes and pathways are overrepresented in MDS compared to AML and vice versa. For example, mutations in spliceosome complex genes are more abundant in MDS than AML and approximately 40% of MDS cases are found to have mutations in one of the spliceosome complex genes (SF3B1, SRSF2, U2AF1, ZRSR2, SF3A1, PRPF40B, U2AF2, and SF1) in a mutually exclusive manner, suggesting that deregulation in pre-mRNA splicing plays a crucial role in MDS pathogenesis (Figure 1b)89,90. Recently, sequencing of longitudinal samples from MDS patients identified hotspot mutations in SET binding protein 1 (SETBP1) that was acquired during leukemic evolution to AML91. This data has set the framework for elucidating the genomic basis of transformation from MDS to AML.
Lymphoid malignancies
The pattern of driver mutations identified in lymphoid malignancies differs from that of myeloid malignancies, although there is some overlap of mutated genes found in CLL29,30,92-94, acute lymphoblastic leukemia (ALL)95-97, diffuse large-B-cell lymphoma (DLBCL)98-101, mantle-cell lymphoma (MCL)102, and multiple myeloma (MM)103,104 (Supplementary Figure 2). Examples of findings for CLL include three independent WES and WGS studies29,30,92 that revealed known mutations in TP53105,106 and ATM107, and previously unknown SMGs in NOTCH1, myeloid differentiation primary response gene 88 (MYD88), and the splicing factor SF3B1 (Figure 1b)29,30,92. In addition, WES and WGS analysis from 91 CLL cases92 defined five core molecular pathways crucial for disease pathogenesis: DNA damage repair and cell cycle control, NOTCH signaling, inflammatory pathways, WNT signaling, and RNA splicing and processing pathways.
Some shared features have been reported in other lymphoid malignancies. For example, RNA-seq has identified hotspot mutations in NOTCH1 in some cases of MCL, suggesting a common role of NOTCH signaling deregulation in B cell malignancies102. MYD88 mutations were also observed in DLBCL99 and Waldenstrom macroglobulinemia108. The landscape of somatic mutations has also been characterized in multiple myeloma in two recent WES/WGS studies, confirming known deregulation of RAS, NF-κB, and histone methyltransferase activity, while revealing previously unknown mutations in genes involved in RNA processing and protein homeostasis103,104. The pattern of somatic mutations in DLBCL is more complex99, but a significant number of cases harbor mutations in regulators of histone and chromatin modification including MLL2, CREBBP, EP300 and activating mutation in EZH2 (Figure 1a)98,100,101. Of interest, WGS of pediatric early T cell precursor ALL revealed a similar somatic mutation landscape to that of myeloid leukemia, suggesting that therapies effective for patients with myeloid leukemia might also be effective in this aggressive form of pediatric leukemia97.
NGS also has the potential to reveal major actionable genetic alterations in rare cancers, such as the discovery of NOTCH2 mutations in 25% of splenic marginal zone lymphoma (SMZL)109, mutations in signal transducer and activator of transcription 3 (STAT3) in 40% of large granular lymphocytic leukemia (LGL)110, and BRAF V600E mutation present in 100% of hairy-cell leukemia (HCL) samples tested to date111. The BRAF inhibitor, vemurafenib, has already shown efficacy in the case of an individual with refractory HCL, and Phase II clinical trials are ongoing to validate these findings (clinicaltrials.gov identifier: NCT01711632)112 [link to http://clinicaltrials.gov/show/NCT01711632] These examples show how systematic integration of data from diverse tumor types has the potential to transform the diagnostic and treatment paradigm for a rare disease.
Genomically defined cancer subtypes
Cancer genomics has shown that histopathologically distinct cancer subtypes of the same organ site often have divergent underlying genomic alterations. In addition to the examples described above, TP53 is mutated in 96% of HGS-OvCa samples, but clear-cell and endometrioid ovarian cancer tumors have lower rates of TP53 mutations and instead possess frequent recurrent mutations in PIK3CA113 and ARID1A114,115, which were identified using NGS technology. Most cutaneous melanomas are driven by BRAF or NRAS mutations; by contrast ocular melanomas, have frequent hotspot mutations in the G-proteins GNA11, GNAQ, and LOF mutations in deubiquitinating enzyme, BAP151,116,117. Recent glioblastoma studies support the notion that pediatric and adult cancers need to be characterized separately at the molecular level, as pediatric and adult glioblastoma tumors possess distinct genetic driving events, which includes mutations in ATRX, DAXX, and the replication-independent histone variant, H3F3A, which were much more prevalent in the pediatric setting (Figure 1a and Supplementary Figure 1)118.
Genomically defined cancer subtypes have been shown to carry diagnostic and/or prognostic significance. One example of this is the V617F mutation in Janus kinase 2 (JAK2) in the diagnosis of the myeloproliferative neoplasm, polycythemia vera (PV), the incidence of which is estimated at 95% and is currently incorporated as one of the diagnostic criteria for PV119. Based on recent findings, the BRAF V600E mutation might be used as a diagnostic tool for HCL, a disease for which morphological diagnosis has been a challenge, but further validation is required111. Classically, genetic information has been actively incorporated into the diagnosis and prognostication of AML and MDS120,121. Chromosomal alterations remain the strongest prognostic factor in both AML and MDS, but recent efforts incorporating somatic mutations have shown promise in creating more sophisticated prognostic models122-124. For instance, in intermediate-risk AML identified by the conventional prognostic model, by incorporating information of additional genetic alterations, which include the internal tandem duplication in fms-related tyrosine kinase 3 gene (FLT3-ITD) and mutations in NPM1, CEBPA, and MLL genes, physicians are able to identify patients that will benefit from stem cell transplant during the first complete remission125. Such prognostication studies help not only to identify “biological drivers” but also to identify “clinically relevant drivers”.
Conclusions and future directions
A number of important future directions have emerged from the genomic studies described above. First, the characterization of rare cancers and clinically important genetic subtypes, such as NSCLCs that lack genetic aberrations in EGFR, KRAS and ALK or melanomas that are wild-type for BRAF and NRAS will undoubtedly provide valuable information into the genetic etiology of these cancers. Second, the analysis of somatic mutations has focused almost entirely on the protein coding regions of the genome. However, projects such as the Encyclopedia of DNA elements (ENCODE) are elucidating the functional elements encoded in the roughly 80% of the genome that is non-coding, including promoter and enhancer regions, providing a significant opportunity to understand the landscape of somatic mutation across the entire genome126. In this regard, two groups have recently shown recurrent mutations in the TERT promoter in approximately 70% of melanomas127,128, which highlights the discovery potential in examining somatic mutations in non-coding regions in cancer. Third, we need to begin to systematically explore the interaction of host genome variation with the somatic genome of the tumor in ultimately influencing outcomes. Fourth, recent studies have brought forward the important issue of tumor heterogeneity and the clinical implications for targeted therapy129 (Box 5). In this respect, in addition to extending genomic study design to include multi-region and longitudinal sampling of tumors, linking of the complex genomic data to dynamic clinical history of that specific patient will be most informative in guiding the analyses and extracting the most clinically relevant insights from the cancer genomes. Such approaches will undoubtedly be essential for the study of drug resistance mechanisms of cancer, which remains an important area of future focus. Lastly, we must not underestimate the challenge and necessity of deep biological investigation in the search for efficient and effective translation of new genomic discoveries into clinically impactful endpoints.
Acknowledgements
We sincerely apologize for omission of any pertinent work related to this review. We thank Denise Spring and members of the Chin lab for their helpful comments and feedback.
GLOSSARY TERMS
Next generation sequencing (NGS) | All post-Sanger sequencing methods, most commonly referring to massively parallel sequencing technology. |
Hybrid-capture sequencing | A target enrichment approach wherein custom oligonucleotides (bait set) are designed and optimized to hybridize to specific regions of the genome so specific fragments of DNA can be enriched by hybridization for NGS sequencing. |
Whole-exome sequencing (WES) | Sequence by NGS all protein-coding exomes after capture utilizing hybridization to a whole-exome bait set designed to enrich DNAs in all protein coding portion of the genome. The most common implementation targets miRNA genes in addition. The size of the captured DNA is approximately 40 Mb. |
Whole-genome sequencing (WGS) | Sequencing of the entire genome, usually via a random fragment (shotgun) and to sufficient coverage to ensure adequate representation of all alleles. A variation specifically utilizing low-coverage WGS is sometimes leveraged to assess rearrangements in the genome. |
Significantly mutated genes (SMGs) | A gene that possesses a somatic mutation rate above the calculated background mutation rate (BMR) as determined by a given statistical calculation. |
Driver mutations | Somatic mutations in a gene that confer a selective advantage to cancer cells as reflected in statistical evidence of positive selection. This is not a definition based on functional activity. |
Passenger mutations | Neutral mutations in a gene that do not provide a selective advantage for cancer cells as reflected in lack of statistical evidence for positive or negative selection. This is not a definition based on functional activity. |
Hotspot mutations | Recurrent mutations resulting in the same amino acid change in a gene observed in cancer, signifying strong positive selection. |
Cancer gene | A gene is considered a cancer gene if it harbors a cancer driving genetic aberration (note: cancer genes may possess both driver and passenger somatic alterations) as defined by criteria that can include statistical evidence of selection, recurrence pattern or by functional activity. |
Two-hit tumor suppressor | The Knudson two-hit hypothesis was proposed to explain the early onset of cancer in hereditary syndromes whereby inheritance of one germline copy of a mutated gene in all cells substantially increases the likelihood any cell undergoing mutation of the other allele, thus giving rise to earlier onset disease compared to sporadic forms of the disease. It specifically relates to the necessity to inactivate both alleles of a recessive cancer gene. |
Epstein-Barr Virus (EBV) | Member of the Herpes virus family associated with the development of particular forms of cancer. |
Background mutation rate (BMR) | The rate of mutation in a tumor sample as a consequence of exposure to environmental mutagens (e.g. UV exposure) and/or random generation and misrepair processes. |
CpG island methylator phenotype | A classification of cancers by their degree of methylation at CpG rich promoter regions, first characterized in human colorectal cancers, and often associated with distinct epidemiology, histological and molecular distinct features. |
Triple negative breast cancer | One of the subtypes of breast cancer that is defined by the absence of staining for estrogen receptor (ER), progesterone receptor (PR) and HER2 (ERBB2) by immunohistochemistry. |
Neoadjuvant aromatase inhibitors | Aromatase inhibitors used to treat estrogen receptor positive breast cancer patients prior to surgical resection. This approach is applied in cases where tumor size needs to be reduced for breast conserving surgery. Neoadjuvant aromatase inhibitor treatment is not considered a standard of care at this point and is conducted under clinical trials. |
Sleeping Beauty Transposon System | A genetically engineered insertional mutagenesis system involving synthetic DNA transposons, which can be applied to various model systems to ascertain gene function. |
Aflatoxin B | Aflatoxin B is one of the mycotoxins that are produced by Aspergillus Flavus. High-level exposure to aflatoxins is known to cause acute liver necrosis or cirrhosis resulting in the development of hepatocellular carcinoma (HCC). |
French-American-British (FAB) classification M1 | FAB classification of acute leukemias was first proposed in 1976 by French-American-British cooperative group. It classified acute myeloid leukemia (AML) into 8 different categories (M0-M7) and acute lymphoblastic leukemia (ALL) into 3 different categories (L1-L3) based on their morphological findings. The classification was updated in 1989. |
RNAsequencing (RNAseq) | Whole-transcriptome shotgun sequencing of cDNA to determine the sequence of RNA used for expression analysis and the identification of gene-gene fusions. |
Microsatellite instability (MSI): | Is a hypermutable phenotype caused from germline, somatic, or epigenetic inactivation in DNA Mismatch Repair (MMR) activity |
Chromothripsis | Greek for chromosome “shattering”, whereby up to hundreds of genomic rearrangements take place in a single cellular crisis event that develop from errors in mitosis that occur in approximately 2-3% of cancers. |
Chromoplexy | Greek for chromosome “weave” or “braid”. Analysis of prostate cancer genomes revealed copy-neutral rearrangements consisting of between 4-12 distinct breakpoint junctions, which tend to occur at transcriptionally active portions of chromatin, forming a closed chain called chromoplexy. |
Kategeis | Greek for “shower” or “thunderstorm”. A phenomenon, identified in breast cancers, of localized hypermutations almost exclusively involving C base pair substitutions at TpC dinucleotides. This mutation pattern has been linked to the APOBEC family of cytidine deaminases. |
Actionable genetic alterations | A genetic alteration with sufficient scientific evidence supporting its use to inform a treatment decision |
Biography
Lynda Chin, M.D., is a Professor and Chair of the Department of Genomic Medicine and the Scientific Director of the Institute of Applied Cancer Science at The University of Texas MD Anderson Cancer Center in Houston. Dr. Chin is a Principal Investigator of The Cancer Genome Atlas (TCGA) Genome Data Analysis Center at the Broad Institute and co-Principal Investigator of the TCGA Genome Characterization Center at Harvard Medical School. She is also a member of the Scientific Steering Committee of the International Cancer Genome Consortium (ICGC). Dr. Chin received her M.D. from the Albert Einstein College of Medicine in New York and conducted her clinical and research training at Albert Einstein College of Medicine and Columbia Presbyterian Medical Center. Her research program focuses on mining and translating complex multi-dimensional genomic data through comparative oncogenomics — the comparison of mouse and human cancers — and the integration with functional genomics to identify novel cancer targets and diagnostic biomarkers.
Andrew Futreal, Ph.D., is a Professor in the Department of Genomic Medicine at The University of Texas, MD Anderson Cancer Center in Houston and Honorary Faculty member at the Wellcome Trust Sanger Insitute. He received his Ph.D. in Pathology from the University of North Carolina at Chapel Hill. He was a faculty member at Duke University until 2000, when he moved to the Wellcome Trust Sanger Institute to become a Co-Founder/Director of the Cancer Genome Project and Head of Cancer Genetics and Genomics. His work has focused on identifying somatic mutations in human cancers and discoverying susceptibility genes for breast and ovarian cancers, which includes his pioneering work on BRCA1 and BRCA2. Dr. Futreal has characterized a number of somatic alterations driving cancer, such as the discovery of somatic BRAF mutations in melanoma and other cancers; ERBB2 intragenic mutations in lung cancer; FBXW7 and PTEN mutations in T-cell leukemia and frequent mutations in genes encoding epigenetic regulators in clear cell renal cell carcinoma.
Ian Watson, Ph.D., is a Postdoctoral Fellow in the laboratory of Dr. Lynda Chin at the Department of Genomic Medicine at The University of Texas, MD Anderson Cancer Center in Houston. He currently holds a Canadian Institute of Health Research (CIHR) Postdoctoral Fellowship. He trained at the Dana Farber Cancer Institute in Boston from 2010-2011 and was a visiting scientist at the Broad Institute prior to moving to MD Anderson Cancer Center. His current area of research focuses on characterizing the genetic driving events in melanoma through integration of computational approaches and functional genomics.
Koichi Takahashi, M.D., received his M.D. from Niigata University, Niigata, and completed his internship and residency in medicine at both Toranomon Hospital, Tokyo, and at Beth Israel Medical Center, New York. He is currently a Clinical Fellow in Hematology and Oncology at The University of Texas, MD Anderson Cancer Center in Houston and is a research scholar at Department of Hematology and Oncology at Kyoto University, Kyoto, Japan. He is currently training in the laboratory of Dr. Lynda Chin. His research focus involves uncovering genomic mechanisms of disease progression and transformation in hematologic malignancies.
Footnotes
Competing interests statement
The authors have no competing interests to declare.
Weblinks:
International Cancer Genome Consortium (ICGC) Data Portal: http://dcc.icgc.org/web/
The Cancer Genome Atlas (TCGA) data portal: https://tcga-data.nci.nih.gov/tcga/
Broad Institute Cancer Portals http://www.broadinstitute.org/scientific-community/science/programs/cancer/cancer-portals-overview
Broad Institute Genome Data Analysis Center (GDAC) https://confluence.broadinstitute.org/display/GDAC/Home
Wellcome Trust Sanger Institute Scientific Cancer Genome Project http://www.sanger.ac.uk/research/projects/cancergenome/
Catalogue of Somatic Mutations in Cancer (COSMIC) http://cancer.sanger.ac.uk/cancergenome/projects/cosmic/
Memorial Sloan-Kettering Cancer Center cBioPortal: http://www.cbioportal.org/public-portal/
Institute for Systems Biology and MD Anderson Cancer Center web portal http://www.cancerregulome.org/cancerstudies.html
UCSC Cancer Genomics Browser https://genome-cancer.ucsc.edu/
UCSC Encyclopedia of DNA Elements http://genome.ucsc.edu/ENCODE/
ClinicalTrials.gov: http://clinicaltrials.gov NCT01711632
Subject categories:
Biological sciences / Cancer / Cancer genomics [URI /631/67/69]
Biological sciences / Genetics / Genomics / Genome evolution [URI /631/208/212/2304]
Biological sciences / Genetics / Functional genomics / Mutagenesis [URI /631/208/191/1908]
Biological sciences / Genetics / Sequencing / Next-generation sequencing [URI /631/208/514/2254]
References
Full text links
Read article at publisher's site: https://doi.org/10.1038/nrg3539
Read article for free, from open access legal sources, via Unpaywall:
https://www.ncbi.nlm.nih.gov/pmc/articles/PMC4014352
Citations & impact
Impact metrics
Article citations
Prostate Cancer Progression Modeling Provides Insight into Dynamic Molecular Changes Associated with Progressive Disease States.
Cancer Res Commun, 4(10):2783-2798, 01 Oct 2024
Cited by: 0 articles | PMID: 39347576 | PMCID: PMC11500312
Network analysis of driver genes in human cancers.
Front Bioinform, 4:1365200, 08 Jul 2024
Cited by: 0 articles | PMID: 39040139 | PMCID: PMC11260686
Pan-cancer discovery of somatic mutations from RNA sequencing data.
Commun Biol, 7(1):619, 23 May 2024
Cited by: 0 articles | PMID: 38783092 | PMCID: PMC11116503
Identifying somatic fingerprints of cancers defined by germline and environmental risk factors.
Genet Epidemiol, 30 Apr 2024
Cited by: 0 articles | PMID: 38686586
Integrative genomic and transcriptomic profiling of pulmonary sarcomatoid carcinoma identifies molecular subtypes associated with distinct immune features and clinical outcomes.
Cancer Innov, 3(3):e112, 15 Apr 2024
Cited by: 0 articles | PMID: 38947760
Go to all (312) article citations
Other citations
Wikipedia
Data
Data behind the article
This data has been text mined from the article, or deposited into data resources.
BioStudies: supplemental material and supporting data
Clinical Trials
- (3 citations) ClinicalTrials.gov - NCT01711632
Similar Articles
To arrive at the top five similar articles we use a word-weighted algorithm to compare words from the Title and Abstract of each citation.
Beyond the exome: the role of non-coding somatic mutations in cancer.
Ann Oncol, 27(2):240-248, 23 Nov 2015
Cited by: 22 articles | PMID: 26598542
Review
Pan-cancer analysis of whole genomes.
Nature, 578(7793):82-93, 05 Feb 2020
Cited by: 1327 articles | PMID: 32025007 | PMCID: PMC7025898
Next-Generation Sequencing.
Adv Exp Med Biol, 943:119-148, 01 Jan 2017
Cited by: 18 articles | PMID: 27910067
Review
Advances in understanding cancer genomes through second-generation sequencing.
Nat Rev Genet, 11(10):685-696, 01 Oct 2010
Cited by: 649 articles | PMID: 20847746
Review
Funding
Funders who supported this work.
CIHR
NCI NIH HHS (2)
Grant ID: P30 CA016672
Grant ID: U24CA143845