Abstract
Free full text

The evolution of preclinical Alzheimer’s disease: Implications for prevention trials
Abstract
As the field begins to test the concept of preclinical neurodegenerative disease, the hypothetical stage of disease when the pathophysiological process has begun in the brain but clinical symptoms are not yet manifest, a number of intriguing questions have already arisen. In particular, in preclinical Alzheimer’s disease (AD), the temporal relationship of amyloid markers to markers of neurodegeneration and their relative utility in the prediction of cognitive decline among clinically normal older individuals remains to be fully elucidated. Secondary prevention trials in AD have already begun in both genetic-at-risk and amyloid-at-risk cohorts, with several more trials in the planning stages, that should provide critical answers about whether intervention at this very early stage of disease can truly bend the curve of clinical progression.
Introduction
The challenge in effectively treating neurodegenerative disease, and yet perhaps the greatest promise, lies in the reality that the pathophysiological process begins well more than a decade prior to the stage of clinically detectable symptoms. Recent reports from autosomal dominant forms of Alzheimer’s disease (AD) suggest that amyloid-β (Aβ) accumulation may be evident 20 years before the stage of dementia, and that there is already substantial neuronal loss by the stage of mild cognitive impairment (MCI). Indeed, this long, inexorable progression of neurodegeneration, that is well entrenched by the stage of symptomatic disease, may account, at least partially, for our failure to develop successful disease-modifying therapies (Sperling et al., 2011b). The vast majority of potential disease-modifying interventions have been tested in cohorts with clinically manifest neurological illness, when there is already substantial synaptic and neuronal damage. It is likely, as in the other fields of medicine for which we have made significant inroads, in particular, cancer, cardiovascular disease, stroke, HIV/AIDS, and diabetes, that we would have a greater chance for success targeting much earlier intervention in neurodegenerative diseases. Fortunately, recent advances in molecular neuroimaging, cerebrospinal fluid assays, and other imaging and biofluid markers have greatly facilitated our ability to detect evidence of neurodegenerative pathology in vivo, particularly in very early AD. The critical next steps will require us to elucidate the definitive links between the biomarkers of the hallmark pathologies of AD and the earliest clinical manifestations with carefully designed natural history, observational cohort studies, and ultimately with successful early intervention trials at the preclinical stages of AD.
Convergent findings from rare autosomal dominant causative mutation carriers, non-deterministic genetic-at-risk populations, and age-at-risk cohorts indicate that the pathophysiological process of AD is detectable with cerebrospinal fluid and imaging markers many years prior to the stage of dementia (Bateman et al., 2012; Knopman et al., 2012; Mormino et al., 2014b; Reiman et al., 2012; Villemagne et al., 2013; Vos et al., 2013), and the field is active with research studies aiming to improve the accuracy of prediction of cognitive decline in at-risk individuals.
Reports from autopsy cohorts, CSF and other PET amyloid imaging studies suggest that at least 1/3 of clinically normal (CN) older individuals have evidence of substantial Aβ accumulation (see Figure 1), one of the hallmark pathologies of AD (Arriagada et al., 1992b; Bennett et al., 2006; De Meyer et al., 2010; Fagan et al., 2009; Gomperts et al., 2008; Hampel, 2013; Jack et al., 2008; Jack et al., 2014; Kantarci et al., 2012; Mintun et al., 2006; Montine, 2011; Morris et al., 1996; Rowe et al., 2010; van Harten et al., 2013; Villemagne et al., 2013). It remains unknown whether the majority of CN with evidence of cerebral amyloidosis will in fact progress to the symptomatic stages of AD and over what time frame; however, the accumulating longitudinal data suggest that many of these individuals are indeed in the “preclinical stages of AD (Sperling et al., 2011a). Recent studies suggest that older individuals designated as Aβ+ are at increased risk for cognitive decline (Lim et al., 2014b; Lim et al., 2014c; Mormino et al., 2014a) and progression towards the symptomatic AD phenotype (Knopman et al., 2012; Vos et al., 2013). The challenge is that Aβ is only one piece of the puzzle, and recent findings continue to support the hypothesis that evidence of Aβ is necessary, but not sufficient in isolation, to predict imminent decline along the AD trajectory. Thus, we must continue our efforts to characterize the biomarkers or likely the combinations of biomarkers that will more accurately predict the emergence of clinical symptoms and the rate of subsequent decline at the individual level. This information is particularly important for AD prevention clinical trial design, and for future translation to clinical practice. This review will highlight recent progress in the field of preclinical AD, and the remaining gaps in knowledge.
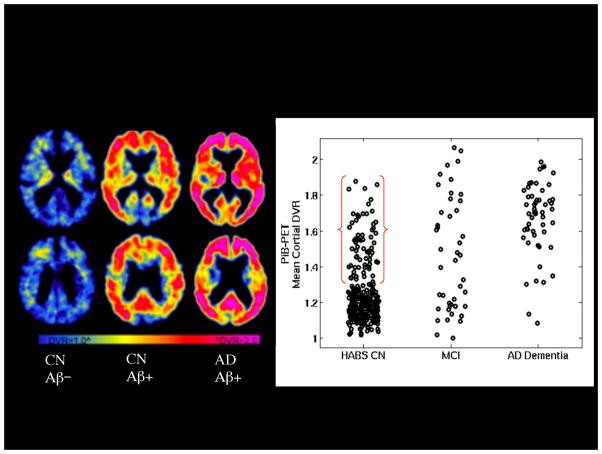
PET amyloid imaging with 11C-PiB. Left: Representative PET images from three older individuals: Clinically normal older individual without evidence of elevated Aβ accumulation (CN Aβ−), Clinically normal older individual with elevated Aβ accumulation (CN Aβ+) and patient with AD dementia with very elevated Aβ accumulation (AD Aβ+) in frontal and parietal heteromodal cortices Right: Scattergram of PiB distribution value ratios (DVR) by diagnostic group: Harvard Aging Brain Study Clinically normal older individuals (HABS CN), Mild Cognitive Impairment (MCI), and AD dementia. Approximately 30% of HABS CN demonstrate elevated Aβ accumulation in the range of MCI and AD dementia Aβ+.
The amyloid debate
The predominant model of preclinical AD that has emerged from laboratory studies and longitudinal clinical investigation places Aβ peptide accumulation as a key early event in the pathophysiological process of AD, but this remains controversial. All of the known autosomal dominant, early onset forms of AD are thought to result from alterations in amyloid-precursor protein (APP) production or cleavage (PSEN-1 and PSEN-2). Similarly, trisomy-21 invariably results in AD pathology in individuals who have three intact copies of the APP coding region located on chromosome 21, and leads to AD dementia in the majority of those who live beyond age 60. In addition apolipoprotein E (APOE), the major genetic risk factor for late onset AD, has been implicated in amyloid trafficking and plaque clearance.
Imaging studies in autosomal dominant AD have documented early accumulation of Aβ on PET scans beginning approximately 15-20 years prior to the average age of symptom onset in these families (Benzinger et al., 2013; Fleisher et al., 2012). Interestingly, however, there is some evidence of early synaptic change, prior to crossing a biomarker threshold of Aβ deposition in autosomal dominant mutation carriers (Reiman et al., 2012) and APOE ε4 carriers (Filippini et al., 2009) that may reflect toxicity of Aβ oligmeric forms. PET measurements in these high genetic-risk individuals may underestimate the accumulation of diffuse plaques, and CSF measurements indicate an early rise in Aβ1-42 levels in very young carriers that may have biological consequences in terms of synaptic function (Reiman et al., 2012). It is also possible that synaptic, mitochondrial, metabolic, or neuronal cytoskeletal alterations play an important role, in the pathogenesis of AD even in these autosomal dominant cases where Aβ peptides are overproduced since birth.
A particularly compelling finding in support of the amyloid hypothesis in late onset AD is the recent identification of a protective gene mutation (Jonsson et al., 2012). A coding mutation (A673T) adjacent to the aspartyl protease beta-site in the APP gene, which is estimated to result in approximately 40% reduction in Aβ1-42 formation of Aβ in vitro, was found to be associated with a marked reduction in the prevalence of AD in several large cohorts. Interestingly, this mutation was also found to be protective against cognitive decline in CN, which also supports the hypothesis that Aβ may influence cognitive decline in the preclinical stages of AD.
Recent evidence similarly suggests that aberrant clearance of Aβ42 may be a key etiologic event in sporadic, late-onset AD (Mawuenyega et al.). However, some researchers believe that sequestration of Aβ into fibrillar forms might serve as a protective mechanism (Lee et al., 2004; Shankar et al., 2008). Both autopsy and biomarker studies (see below), however, suggest that markers of Aβ42 accumulation increase with advanced aging, the greatest risk factor for developing AD, are often associated with other “AD-like markers of neurodegeneration, and increased risk of progression to AD dementia. It is also clear that synaptic depletion, intracellular hyperphosphorylated forms of tau and neuronal loss invariably occur in AD, and that at autopsy, these markers appear to correlate better than plaque counts or total Aβ load with clinical impairment. Although there is increasing evidence that markers of “upstream Aβ accumulation are associated with markers of “downstream pathologic change, including abnormal tau, neural dysfunction, glial activation, and neuronal loss and atrophy, it remains to be proven in sporadic, late-onset AD that Aβ accumulation is sufficient to incite the downstream pathological cascade of AD, and to ultimately result in cognitive impairment and dementia.
Staging of preclinical Alzheimer’s disease
The National Institute on Aging–Alzheimer’s Association (NIA-AA) Preclinical Workgroup developed research criteria to aid in the study of preclinical AD (Sperling et al., 2011a). The NIA-AA workgroup proposed a hypothetical staging framework specifically to categorize clinically normal (CN) individuals thought to be on the trajectory towards the symptomatic stages of AD. These stages are applicable to both genetic at-risk and age at-risk cohorts, and are based primarily on biomarker status from the Jack et al. model (Jack et al., 2010) utilizing fluid and imaging markers of amyloid-β (Aβ) accumulation and markers of neurodegeneration (ND), including CSF tau, FDG hypometabolism, and volumetric MRI metrics of atrophy. The NIA-AA Stage 1 can be operationalized as Aβ+/ND−; Stage 2 as Aβ+/ND+; and Stage 3 as Aβ+/ND+ with evidence of subtle cognitive and behavioral decline not yet sufficient to meet criteria for mild cognitive impairment (MCI; see Figure 2).
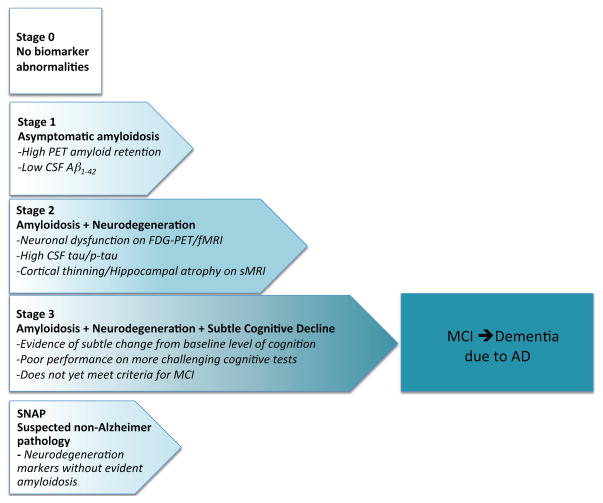
Updated staging framework for preclinical AD (adapted from Sperling et al. 2011a with updates from Jack et al. 2012). Stage 0 represents individuals without biomarker abnormalities who are not thought to be on the AD trajectory. Stage 1 begins with cerebral amyloidosis; Stage 2 is amyloidosis plus markers of neurodegeneration; Stage 3 is amyloidosis + neurodegeneration + evidence of subtle cognitive and behavioral decline that is not yet sufficient to meet criteria for mild cognitive impairment or dementia due to AD. SNAP or Suspected Non-Alzheimer Pathology has evidence of neurodegeneration without apparent amyloidosis.
Since the publication of the NIA-AA Preclinical Workgroup staging schema, several amendments and additions to the proposed classifications have been suggested (Chetelat, 2013; Dubois et al., 2014; Jack et al., 2012). A “Stage 0 group, comprised of individuals who have no evidence of abnormal biomarkers (Aβ−/ND−), was formally delineated as a control group, assuming that they are unlikely to be on the AD trajectory. Perhaps the most important concept to be considered is “Suspected Non-Alzheimer Pathology (SNAP) suggested by the Mayo Clinic (Jack et al., 2012). The SNAP category encompasses individuals with biomarker or imaging evidence of neurodegeneration without exceeding the biomarker cut point for amyloidosis, and includes up to a quarter of the older individuals in clinically normal cohorts (Jack et al., 2012; Mormino et al., 2014a). As would be predicted on the basis of selection on the basis of lower Aβ deposition, the SNAP groups tend to have a lower proportion of APOE ε4 carriers (Jack et al., 2012; Mormino et al., 2014a). Interestingly, individuals classified as SNAP tend to be older, and there is also a higher proportion of males in the SNAP groups (Jack et al., 2012; Mormino et al., 2014a), perhaps indicating the higher rates of vascular co-morbidity among males.
It should be noted that the definitions of + versus − are typically based on somewhat artificial cut points in continuously distributed data (particularly for ND markers), which may have important consequences. Some data types (e.g., amyloid PET) are noisier, due largely to spatial resolution limitations, and thus may be less sensitive to subtle changes than other imaging techniques (e.g., volumetrics); thus, the error associated with the cut point differs according to data type, and the biomarker readouts (+ or −) do not in this respect have the same meaning for all biomarkers. Moreover, the defining cut points are to some extent arbitrarily designated, and may be dependent on the specific attributes of comparison group utilized to define thresholds. Finally, the ND biomarker group includes individual biomarker types that differ from each other both theoretically and empirically (see discussion below).
Nevertheless, the proportions of CN classified across these preclinical stages, utilizing operationalized cutpoints, are remarkably similar across older cohorts, with approximately 40-50% classified as Stage 0; 10-15% as Stage 1; 15% as Stage 2 (Aβ+/ND+); and approximately 25% as SNAP (Aβ−/ND+) (Jack et al., 2012; Mormino et al., 2014a; Vos et al., 2013; Wirth et al., 2013b). These findings do indicate that a non-negligible proportion of CN have biomarker evidence of ND in the apparent absence of elevated Aβ accumulation. It is possible, that SNAP does include some individuals with sub-threshold levels on Aβ markers who are early in the process of rapid accumulation, however, recent work suggests that SNAP individuals are not more likely over short intervals to subsequently accumulate Aβ, whereas Aβ+ individuals are more likely to develop subsequent increases in ND markers (Jack et al., 2014; Knopman et al., 2013).
The question as to whether SNAP individuals manifest cognitive decline at the same rate as individuals in Stage 1 or Stage 2 Preclinical remains a matter of debate. Several recent reports have suggested that SNAP (Aβ−/ND+) individuals show a greater rate of decline than Stage 0 (Aβ−/ND−), a slightly lower rate than Stage 1 (Aβ+/ND−), and a significantly lower rate of decline than Stage 2/3 (Aβ+/ND+) (Mormino et al., 2014a; Vos et al., 2013). A subset of SNAP individuals may also be in the preclinical stages of non-AD neurodegenerative diseases. Additional large observational studies that include longitudinal markers of both Aβ and ND (ideally with more specific markers of AD-related neurodegeneration) with longer term clinical follow-up will be required to fully elucidate the temporal ordering relative contribution of these markers in predicting progression to MCI and dementia due to AD.
Relationship between markers of amyloid and neurodegeneration
Given that ND can exist in the apparent absence of elevated Aβ as highlighted by the SNAP group, it is not surprising that the results from cross-sectional studies examining the association between Aβ and ND are somewhat variable and challenging to interpret. We recently reported that Aβ+ CN were three times more likely to be classified as ND+ than Aβ− CN (Mormino et al., 2014a), which is consistent with previous work showing a similar relationship (Becker et al., 2011; Dickerson et al., 2008; Rowe et al., 2010; Storandt et al., 2009). However, other studies have not found a cross-sectional relationship between Aβ and ND (Chetelat et al., 2010; Wirth et al., 2013a). These inconsistencies may stem from differences related to cohort size and characteristics, as well as the examined measure of ND. The association between Aβ and ND is further complicated by the observation that commonly employed markers of ND are not necessarily consistent with one another (Toledo et al., 2014), and that the association between ND and Aβ status within CNs may vary depending on which measure is examined (Whitwell et al., 2013). Thus, the association between markers of Aβ and markers of ND within CN cohorts is likely subtle and influenced by methodological approaches.
Although many models suggest that neurodegenerative biology occurs downstream from Aβ dysmetabolism (Hardy and Selkoe, 2002; Jack et al., 2013a), other work suggest that pathways promoting amyloidosis and neurodegeneration might arise independently (Jack et al., 2013b; Wirth et al., 2013a). These models are not mutually exclusive, that is, Aβ and ND may initially appear to begin via separate pathways, but at some point would demonstrate an increasing biomarker association if Aβ exacerbates underlying ND (or vice versa). Longitudinal studies relating baseline levels of Aβ to change in ND biomarker over time within CNs support this claim, given that most longitudinal studies that have examined this association have revealed increased ND over time in Aβ+ CNs (Chetelat et al., 2012; Dore et al., 2013; Knopman et al., 2013; Nosheny et al., 2014; Schott et al., 2010; Storandt et al., 2009). However, there is some debate as to whether Aβ accumulation is specifically associated with atrophy of medial temporal lobe structures (Fjell et al., 2014), which may reflect the relative influence of tau rather than Aβ accumulation in this region (see below). Given the prolonged period of Aβ accumulation during preclinical AD (Villemagne et al., 2013), it is possible that different brain regions show varying degrees of vulnerability throughout the prolonged period at which Aβ accumulation occurs within CN (Mattsson et al., 2014). Finally, multiple studies have revealed that longitudinal ND is greatest in CN who have evidence of both Aβ accumulation and ND at baseline (Desikan et al., 2011; Jack et al., 2014; Knopman et al., 2013), which may be consistent with a model in which Aβ and ND initially are observed independently, but converge to accelerate further ND. However, it is also possible that ND is a marker of how long Aβ pathology has been present within Aβ+ CNs (ie. Aβ+/ND+ CNs may be further along the AD trajectory than Aβ+/ND− CNs). In addition to promoting subsequent ND, CN that are positive for both Aβ and ND are also more likely to progress to MCI (Knopman et al., 2012; Rowe et al., 2013) from Clinical Dementia Rating (CDR) 0 to 0.5 (Desikan et al., 2012; Vos et al., 2013), as well as show cognitive decline over time (Mormino et al., 2014a; Wirth et al., 2013b). Overall, studies examining the associations between Aβ and ND suggest that ND is exacerbated after the convergence of Aβ and ND pathways, and that this convergence is consequential to behavioral outcomes.
If baseline levels of ND are not exclusively associated with Aβ accumulation within older CN, what additional factors influence biomarker readouts of ND in CNs? Recent work directly investigating characteristics of SNAP (Aβ− and ND+) have found associations with markers of cerebrovascular disease (Wirth et al., 2013b) as well as a greater proportion of men (Mormino et al., 2014a). Non-AD pathologies are also likely contributors to ND within CNs. For instance, Bennett and colleagues (Bennett et al., 2006) have found that among community dwelling CN, 21.6% show infarcts, 13.4% have Lewy bodies and approximately 5% have hippocampal sclerosis at postmortem examination. TDP-43 pathology, which is associated with frontotemporal dementia, is also prevalent in older CN (Geser et al., 2010). The relevance of TDP-43 in aging was recently highlighted in a study by Wilson and colleagues (Wilson et al., 2013b). This study followed 130 individuals that were non-demented at baseline for an average of 10 years before death and found that nearly half showed some degree of TDP-43 pathology at postmortem examination. TDP-43 pathology was most common in the medial temporal lobe, suggesting that this pathology may contribute to ND in regions typically associated with AD. Furthermore, TDP-43 pathology uniquely explained a substantial portion of cognitive decline (12%, after accounting for age and NFTs), which is on par with the amount of decline uniquely explained by NFTs. Thus, TDP-43 pathology is an important contributor of decline in aging, and may contribute to ND in regions typically associated with AD. In addition to non-AD pathologies (Jack et al., 2002), hippocampal volume has also been associated with inflammation (Marsland et al., 2008), stress (Lupien et al., 1998), and estrogen replacement therapy (Eberling et al., 2004). Individuals in their 80’s have likely accumulated many more co-morbidities than those in their 60’s, and thus are more likely to demonstrate ND markers due to other etiologies, but may also be more vulnerable to developing cognitive decline in the setting of even low levels of Aβ accumulation.
It is also possible that “normal aging processes, such as synaptic alterations (Morrison and Hof, 1997), contribute to markers of ND. Along these lines, gradual and consistent associations between chronological age and gray matter (Raz et al., 2004; Sowell et al., 2003) as well as glucose metabolism (Knopman et al., 2014) are present throughout the lifespan (occurring before the age at which levels of Aβ are clearly elevated). Given that the majority of CNs above age 60 will have some degree of medial temporal lobe neurofibrillary tangle (NFT) pathology (Nelson et al., 2012), it is possible that tau pathology contributes to variance in FDG metabolism and brain volumetry (Jagust et al., 2009; Whitwell et al., 2008) (see discussion below). Finally, it is also possible that variance in markers of ND partially reflect developmental differences and early life brain reserve factors. Given the relatively large amount of variance that is present in markers of ND within young CN, it is possible that older CN with neuroimaging evidence of ND actually had smaller hippocampi and/or reduced glucose metabolism even in early mid-life. Thus, the increased risk of cognitive decline among older CN with both Aβ and ND markers could also reflect evidence that some individuals with lower reserve might be more vulnerable to Aβ toxicity.
The promise of Tau PET imaging
Given that our current markers of ND reflect multiple sources of variance and do not provide primary evidence of AD-related neurodegeneration, it may be useful to track the other hallmark pathology of AD: tau neurofibrillary tangles (NFT). Neuropathologic studies have long suggested that tau pathology correlates more strongly with synaptic and neuronal loss, as well as with the cognitive symptoms of AD (Arriagada et al., 1992a; Gomez-Isla et al., 1997). The application of tau-sensitive biomarker technology would therefore seem to be a potentially useful addition to preclinical AD trial designs. Existing CSF tau measures have been quite valuable in the prediction of cognitive decline among Aβ+ CN (Desikan et al., 2012; Fagan et al., 2007), and increases in CSF tau do correlate with Tau pathologic burden at post-mortem overall (Tapiola et al., 2009); however, some commonly measured Tau isoforms in CSF were not correlated with brain NFT pathology at postmortem (Buerger et al., 2007). It is possible that CSF tau measures may not have sufficient dynamic range to track progression through the spreading of NFT pathology associated with increasing cognitive impairment. The emergence of Tau PET as an AD biomarker should permit exploration of these issues and may prove to be a valuable addition to the biomarker armamentarium.
Brain Tau pathology in aging and typical AD accumulates and spreads anatomically in an orderly fashion that is frequently denoted with the ordinal staging scheme proposed by Braak and colleagues (Braak et al., 2006). The earliest of these stages (Braak Stages I–II) occurs in ~80% of all individuals by age 60 (Nelson et al., 2012). This early, medial temporal lobe (MTL) distribution of Tau likely precedes the emergence of fibrillar brain Aβ burden, and is followed in those individuals who are on the pathologic trajectory of AD, by propagation that extends the distribution of Tau pathology to limbic and neighboring temporal neocortex (Braak III–IV and higher) and is thought to mark the transition from asymptomatic to symptomatic pathology (Hyman et al., 2012; Nelson et al., 2012; Price and Morris, 1999). Until very recently, this critical spread and intensification of Tau pathology has been invisible to all but the neuropathologist.
Remarkable recent advances in PET imaging now allow us to image Tau pathology in vivo (Chien et al., 2013; Maruyama et al., 2013; Zhang et al., 2012). These Tau PET ligands are thought to selectively bind to tau and not to Aβ lesions. Although active research to fully validate these Tau PET tracers against histopathology is ongoing, the selectivity of at least one of the compounds (18F-T807) for Tau over Aβ was estimated to be approximately >27 fold as measured by an autoradiographic comparison of human cortical brain sections (Xia et al., 2013).
Preliminary experience with Tau PET data with 18F-T807 (see Figure 3) and other Tau tracers suggest this new technology may prove extremely valuable in the quest to elucidate the link between Aβ, Tau, and cognitive decline. Our preliminary T807 data are consistent with previous autopsy reports that medial temporal lobe Tau accumulation is very common after age 60, but it remains unknown how this pathology contributes to age-related memory change, at any level of Aβ. Despite the remarkable advances in biomarkers and cognitive research over the past decade, it remains challenging to disambiguate the trajectory of cognitive aging from the preclinical stages of Alzheimer’s disease. There is continued debate in the field as to whether AD might represent an accelerated form of brain aging. This is not to say that AD should be considered normal in any way; AD is a devastating illness that should be aggressively treated, and ultimately, prevented outright. But if tau accumulation in the MTL is indeed present in nearly all individuals over the age of 60 (Nelson et al., 2012), then the distinction between the process of brain aging and at least one of the hallmark pathologies of AD may be somewhat blurred. If tau accumulation in the entorhinal cortex is associated with worse memory performance, even in the absence of supra-threshold levels of Aβ accumulation, then should this be considered part of normal aging or one of the earliest “hits in the AD pathophysiological process?
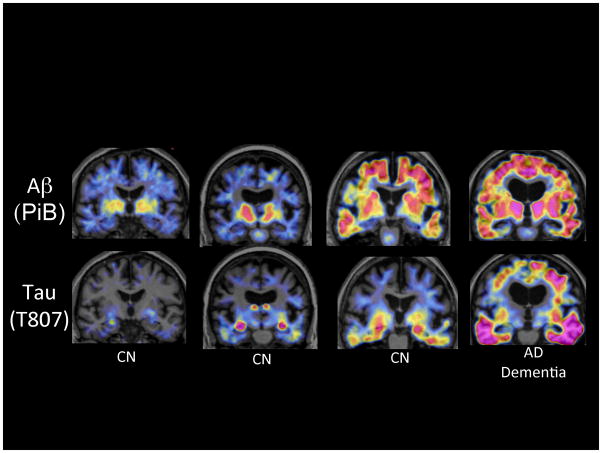
Amyloid and Tau PET imaging. Coronal PET images superimposed on structural Magnetic Resonance) of PiB Aβ (upper row) and T807 Tau (lower row) acquired on 4 participants in the Harvard Aging Brain Study. The first three columns of images are from clinically normal older individuals (CN) with the far right image acquired from a patient with AD dementia. Moving from left to right exemplifies increasing levels of Aβ in necortical regions associated with increasing levels of Tau, particularly prominent in the inferior temporal cortices.
In patients with mild cognitive impairment and mild dementia due to AD with extensive Aβ deposition, our preliminary experience indicates Tau PET binding that is evident in the neocortex, particularly in the inferior temporal cortices, lateral and medial parietal cortices (see Figure 3). The biology underlying evolution of tau pathology is not completely understood, but recent work in transgenic animals suggests that tau may propagate transynaptically (de Calignon et al., 2012; Liu et al., 2012), and that the widespread cortical pattern of tau spreading may be influenced by connectivity (Ahmed et al., 2014). However, there is also evidence of Tau spreading via diffusion to more proximal locations, such as the adjacent inferior temporal cortices, as described by Braak (Braak et al., 2006). Based on our early preliminary work in Tau imaging, evidence from laboratory studies (Choi et al., 2014; Oddo et al., 2006) and previous autopsy studies (Delacourte et al., 2002; Duyckaerts and Hauw, 1997; Price and Morris, 1999), it is likely that Aβ accelerates the spread of Tau both within and beyond the MTL, disrupting function and initiating neurodegeneration in distributed brain networks, resulting in cognitive decline. This hypothetical model (see Figure 4) remains to be tested with longitudinal Aβ and Tau PET imaging in cohorts of clinically normal and very mildly impaired older individuals, and perhaps most importantly, in clinical trials with anti-Aβ therapeutic agents.
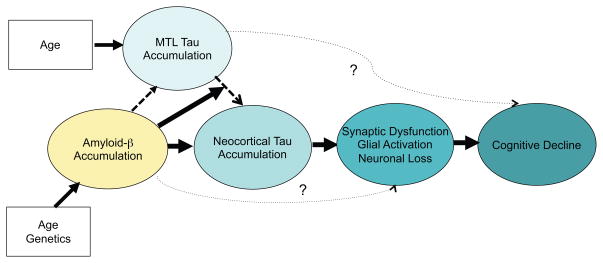
Hypothetical model of the interaction of amyloid-β (Aβ) and tau accumulation. Advancing age is nearly ubiquitously associated with the gradual accumulation of Tau aggregates in medial temporal lobe (MTL), but it remains unknown whether MTL tau in isolation is associated with “age-related cognitive change. Age and genetics influence likelihood of accumulating elevated levels of amyloid-β (Aβ) aggregates. Aβ is hypothesized to increase the accumulation of Tau aggregates and in particular to accelerate the spread of Tau out of the MTL into the neocortex through local diffusion and perhaps via transynaptic spread across neural networks. Tau accumulation leads to synaptic dysfunction, glial activation, and eventually neuronal loss. Aβ may also have direct synaptic toxicity that is not mediated through Tau. The spreading of Tau into neocortex and associated neurodegenerative processes are thought to result in cognitive impairment and further progression along the clinical trajectory of Alzheimer’s disease.
Markers of Preclinical AD, memory activity, and network dysfunction
An active line of research is the relationship of intrinsic neural networks and the “topographic evolution of the pathophysiological process of AD. It is possible, just as in real estate, that “location, location, location is key. The topography has been underutilized thus far in characterizing the dynamic temporal course of biomarker change (Jack et al., 2013a). Using PET molecular imaging, we now have the unprecedented opportunity to investigate the relationship of Aβ and Tau in vivo to the progressive disruption of functional and structural brain networks observed over the course of AD (Chhatwal et al., 2013; Chhatwal and Sperling, 2012; Greicius et al., 2004; He et al., 2009; Hedden et al., 2009; Myers et al., 2014; Petrella et al., 2011; Sorg et al., 2007).
One of the intriguing early findings was the observation that Aβ preferentially accumulates in brain regions that overlap the topology of specific brain networks, primarily the default mode network (Buckner et al., 2005). Subsequently it has been noted that Aβ deposition occurs in multiple regions of high connectivity, designated as “cortical hubs”, that include all of the nodes of the default network but also key nodes in other intrinsic networks (Buckner et al., 2009). Using a variety of paradigms with task fMRI, older individuals with evidence of amyloid accumulation on PET imaging have demonstrated impaired ability to modulate activity in the default mode network (Kennedy et al., 2012; Rami et al., 2012; Sperling et al., 2009), similar to earlier reports in AD dementia (Lustig et al., 2003). In particular, the failure to deactivate the posterior cingulate/precuneus regions during episodic memory encoding tasks has been associated with elevated Aβ accumulation in these regions. Interestingly, a very similar pattern of disrupted posterior cingulate/precuneus activity during memory encoding has been reported in presymptomatic presenilin 1 carriers (Reiman et al., 2012) and APOE ε4 carriers (Fleisher et al., 2009; Pihlajamaki et al., 2010) suggesting that failure to modulate activity in the posteromedial cortices may be an early indicator of synaptic dysfunction that underlies impending memory impairment in very early AD.
Aβ accumulation has been less consistently associated with alterations in MTL function, at least among clinically normal older individuals. Our early cross-modality imaging work suggested that alterations in hippocampal function, manifesting as hippocampal hyperactivity, became evident at the point of the earliest clinical symptoms, perhaps at the stage of early mild cognitive impairment (MCI) (Sperling et al., 2009). Interestingly, however, hippocampal hyperactivity has been reported in asymptomatic carriers of autosomal dominant mutations (Quiroz et al., 2010; Reiman et al., 2012) and younger APOE ε4 carriers (Filippini et al., 2009) suggesting that hippocampal hyperactivity might occur earlier in the setting of virulent Aβ pathology. More recently, we reported evidence of Aβ associated disruptions in entorhinal activity (Huijbers et al., 2014), specifically failure to deactivate the entorhinal cortices, similar to the reports in the neocortical regions of the default network. Of note, the early Aβ associated disruptions in task-related activity, whether in default network regions that typically deactivate during memory encoding, or in “task-positive regions that typically activate during memory processes, tend to manifest as relative increases in activity (Sperling et al., 2010). This finding is consistent with the hypothesis that Aβ might accelerate excitotoxicity through excessive or aberrant neuronal firing (Palop and Mucke, 2010). It is also possible that the increased activity may represent evidence of attempted compensation, at least early in the pathophysiological process (Elman et al., 2014). Unfortunately, hippocampal hyperactivity may also be an indicator of network degeneration (Putcha et al., 2011) and subsequent cognitive decline (O’Brien et al., 2010).
Perhaps most intriguing is the possibility that Aβ could set up a perpetuating cycle by increasing neural activity, which in turn might increase Aβ production (Cirrito et al., 2005; Jagust and Mormino, 2011). It is also conceivable that early Tau accumulation in the medial temporal lobe might be associated with transient increases in MTL neural activity, which could in turn increase Aβ production and result in deposition in the neocortical terminal fields of these neurons.
Similarly, there have been multiple reports of Aβ-related disruptions in resting state or task-free functional connectivity MRI, and the default network appears to be particularly vulnerable to amyloid-related alterations. The majority of these studies have reported decreases in default network activity associated with Aβ deposition (Brier et al., 2014a; Brier et al., 2014b; Hedden et al., 2009; Sheline et al., 2009; Wang et al., 2013). A similar pattern of default network disruption has also been reported in carriers of autosomal dominant genetic mutations (Chhatwal et al., 2013; Thomas et al., 2014). Interestingly, there have also been reports of increased connectivity associated with Aβ in clinically normal older individuals in the default network (Lim et al., 2014a), in prefrontal regions that comprise subcomponents of the DMN (Mormino et al., 2011) and in the salience network of APOE ε4 carriers (Machulda et al., 2011). Intriguingly, there is one report of increased default network connectivity associated with an increase in Aβ burden on longitudinal PET imaging (Jack et al., 2013b), again suggesting that there might be a complex interplay between network activity and Aβ pathology. Task-free functional connectivity MRI is already being implemented in several large-scale, secondary prevention AD trials (see below), as these relatively short MR sequences can be acquired on most scanners during safety MRI sessions. It should be noted, however, that all functional MRI techniques are still fraught with sources of variability, such as head movement, physiological noise, and analytic approaches, that may limit the utility of these techniques in longitudinal studies, and additional work is needed to optimize these methodologies.
In summary, it is striking that early Aβ deposition occurs in a stereotypic set of heteromodal cortical regions, largely overlapping the neocortical regions of the default network that are among the strongest “connection hubs”, whereas Tau accumulation begins in deep grey matter structures, prominently in the transentorhinal cortex and related structures in the MTL that are functionally connected to the default network (Celone et al., 2006). It is of particular interest that the two hallmark pathologies of AD seem to begin to accumulate at the opposite ends of the distributed brain networks supporting memory function, and progressively spread to eventually overlap as the disease progresses. Ongoing studies, combining functional MRI with both Aβ and Tau PET imaging should provide insights into the “chicken vs. egg questions regarding neural activity, network disruption, and the molecular pathology of preclinical AD.
Earliest cognitive changes
One of the most pressing questions remains the cognitive correlates of Aβ, and now Tau, accumulation among clinically normal older individuals. The early literature with cross-sectional Aβ PET data was quite mixed, with some studies reporting subtle associations between amyloid burden and neuropsychological tests (Rentz et al., 2010) and other studies reporting no difference in cognitive performance between older individuals with and without amyloid, particularly when using standard neuropsychological tests used to detect evidence of dementia (Aizenstein et al., 2008). Perhaps this should not be surprising as by definition, selecting a cognitively normal population substantially truncates the variance in test performance. As the field has matured with large sample sizes, there have been increasing reports of significant amyloid-associated deficits in cognitive performance, even amongst the limited range observed in clinically normal older individuals (Li et al., 2014; Lim et al., 2012 [Epub ahead of print]; Sperling et al., 2013) but the cross-sectional effects remain relatively subtle. The most convincing findings, however, stem from Aβ associated decline in longitudinal studies cognition among those who were CN at baseline (Donohue et al., 2014; Doraiswamy et al., 2012; Doraiswamy et al., 2014; Kawas et al., 2013; Lim et al., 2014b; Lim et al., 2012; Mormino et al., 2014a; Mormino et al., 2014b; Resnick et al., 2010; Storandt et al., 2009). The majority of these studies have indicated that episodic memory shows the greatest vulnerability to Aβ-associated cognitive decline, but other domains of cognition including working memory and executive function have also demonstrated Aβ-associated change (Rodrigue et al., 2012). As discussed above, however, it is likely that CN with markers of Aβ in combination with markers of neurodegeneration drive the majority of this decline. In addition, several reports have suggested that Aβ-related cognitive deficits may be accelerated in the presence of one or more APOE ε4 alleles (Kantarci et al., 2012; Mormino et al., 2014b) and other genetic variants, including BDNF (Lim et al., 2013), suggesting that there may be important interactions between Aβ accumulation and genetic background in risk of cognitive decline. More sensitive cognitive and behavioral measures are currently under development in many of these longitudinal cohorts. Recent work with challenging associative memory measures (Rentz et al., 2011) and iPad computerized testing (Rentz et al., 2013) may yield useful measures that will improve our ability to track the earliest clinical changes that appear to begin years prior to the stage of mild cognitive impairment.
Also of particular interest are several reports regarding the association of Aβ accumulation with subjective cognitive concerns. Our group found that greater PiB-PET amyloid burden was related to increased report of subjective memory concerns, even when controlling for depressive sympatology (Amariglio et al., 2012). A similar report from the Berkeley group also found that high amyloid burden was associated with greater memory complaints on self-report (Perrotin et al., 2012). A recent study from Scheltens and colleagues, recruiting participants from a subjective memory complaint clinic, revealed increased cognitive decline in Aβ+ with subjective concerns compared to Aβ− with similar levels of subjective cognitive concerns (van Harten et al., 2013). Most recently, a large study from Kentucky with autopsy confirmation also found that subjective memory complaints among individuals who died prior to diagnosis of cognitive impairment were associated with greater neuritic plaque burden (Kryscio et al., 2014).
Resilience and reserve
The observation that the extent of observable AD neuropathologic changes does not always align with the degree of clinical impairment led to the concept of “reserve”. In general, reserve might be characterized as the ability to tolerate higher levels of brain injury without exhibiting clinical symptoms. The concept of reserve has continued to evolve to include both “brain reserve and “cognitive reserve (Stern, 2009). Brain reserve generally refers to the capacity of the brain to withstand pathologic insult, perhaps due to greater synaptic density or larger number of healthy neurons, such that sufficient neural substrate remains to support normal function. Cognitive reserve is thought to represent the ability to engage alternate brain networks or cognitive strategies to withstand the effects of encroaching pathology. It is not clear, however, that the data support a sharp demarcation between these two constructs, as many factors, such as higher socio-economic status or engagement in cognitively stimulating activities, may contribute to both forms of reserve, and have been associated with lower age-adjusted incidence of clinical AD diagnosis. Recent studies suggest that high reserve may primarily influence the capability of individuals to tolerate their AD pathology for longer periods of time, but may be associated with rapid decline once a “tipping point is reached and compensatory mechanisms begin to fail (Fotenos et al., 2008; Wilson et al.). The relationship between Aβ and cognition is stronger in low reserve CNs (Rentz et al., 2010; Roe et al., 2011), suggesting that high reserve individuals may be able to maintain high levels of cognitive abilities despite underlying pathology. Furthermore, reserve has been shown to be independently associated with cognitive decline, above and beyond what is explained by AD markers such as amyloid, hippocampus volume and glucose metabolism in AD vulnerable regions (Vemuri et al., 2012), as well as common age-related pathologies as measured during post mortem examination (Wilson et al., 2013a). Thus, reserve is an important determinant of cognitive abilities in aging, and may interact with Aβ to infer additional risk of decline. However, reserve, in particular previous participation in cognitively stimulating activities, has also been associated with reduced levels of Aβ within CNs (Landau et al., 2012), which is consistent with animal work showing that an enriching environment is associated with reduced Aβ in mice (Costa et al., 2007). More recent animal data suggest that an enriched environment may also confer protection against Aβ toxicity, perhaps via beta2-adrenergic systems (Li et al., 2013). Thus, higher reserve may exert protective effects directly through influencing Aβ accumulation, and also through synaptic and neuronal capacity to influence the interaction between Aβ and downstream neurodegenerative processes.
Issues around terminology
The publication of the NIA-AA preclinical criteria raised concerns about inappropriately labeling individuals with “Alzheimer’s disease who might never progress to manifest dementia, despite multiple references to the fact that not all individuals with biomarker evidence of AD will develop clinical symptoms within their lifetime. Indeed, the prefix “pre does not require that progression to the future state, merely that the “pre state occurs prior to or “before (in time, place, order, degree or importance) [Oxford English dictionary]. It is interesting that the term “precancerous lesion has not been met with the same concern, nor has “prediabetes raised any ire. The apprehension over preclinical AD terminology likely reflects the continued stigma of the clinical syndrome of AD, and the fact that more data are needed to fully understand the risk of progression at the individual level.
Although findings from both CSF and PET amyloid imaging studies are convergent that older CN with evidence of preclinical AD, particularly those with Aβ and ND markers, have a statistically greater risk of manifesting subsequent cognitive decline and progression to the symptomatic stages of AD at a group level, there are insufficient data at this time to make accurate predictions at an individual level. Despite the accumulating data that suggest older individuals with evidence of amyloid accumulation and/or markers of neurodegeneration are at increased risk for cognitive decline and progression to AD dementia, it is becoming increasingly clear that a proportion of these individuals remain apparently resilient in the setting of early pathology. It is likely that a subset of individuals with biomarker evidence of preclinical AD will not progress to develop AD dementia during their lifetime, due to the estimated temporal lag of >15 years between the appearance of Aβ plaques sufficient to meet the neuropathological criteria for AD and the epidemiological estimates of age-adjusted dementia (Rowe et al., 2010). Recent neuropathologic studies have also highlighted specific factors that may confer resilience at the synaptic and neuronal level (Perez-Nievas et al., 2013). In addition, as noted above, there may be other protective factors, such as education and lifestyle factors, that may attenuate the progression from occult pathology to a symptomatic stage. However, the fact that there may be variable rates of progression to symptomatic AD does not negate the fact that these markers of preclinical AD are detecting evidence that the AD pathophysiological process has begun in the brain.
An international working group (Dubois et al., 2010; Dubois et al., 2007; Dubois et al., 2014), which also utilizes the overall category of preclinical AD, suggested the terminology “presymptomatic AD for individuals with autosomal dominant genetic mutations, and that “asymptomatic at-risk for Alzheimer’s disease be used to refer to individuals with biomarker evidence (CSF or PET amyloid imaging) of AD pathology but no clinical manifestations. However, this latter term is problematic as one cannot simultaneously be “at-risk for a disease”, and have “biomarker evidence that the disease has already begun”. The difficulty remains that the field uses the term “Alzheimer’s disease to refer to both the neuropathologic process in the brain and to the clinical syndrome, typically at the stage of dementia. As we move towards detecting and treating at the earliest stages of AD, the dissociation between these two connotations of “Alzheimer’s disease remains particularly salient. Both the underlying pathophysiological process of AD and its clinical symptomatology represent continua, and the evidence thus far suggests that the pathological and clinical manifestations evolve in parallel but temporally offset trajectories (Rowe et al., 2010; Sperling et al., 2011a).
The term “asymptomatic at-risk for Alzheimer’s dementia or “asymptomatic at-risk for memory decline due to AD may be preferable; however, it is also clear that a proportion of the individuals with biomarker evidence of AD pathology also demonstrate very subtle cognitive decline and subjective memory concerns as described above, thus, “asymptomatic may also be a misnomer (see section on Cognitive Studies). “Preclinical AD was originally selected by the NIA-AA workgroup as the best terminology to encompass individuals who have very subtle cognitive or behavioral changes but have not yet progressed to the point of clinically evident impairment that is diagnosed as MCI or dementia. However, as the term “preclinical also sometimes refers to the stage of animal toxicology studies in the process of drug development (also referred to as non-clinical), this term may be confusing in the setting of clinical trials. Regardless of the exact terminology, the concept that the spectrum of AD encompasses a pathophysiological phase that is detectable prior to clinically evident symptoms is important particularly for enabling clinical trials aimed at preventing the clinical expression of AD.
Implications for prevention trial design
The recognition of this long preclinical phase of AD has already enabled a number of secondary prevention trials. Secondary prevention in this context refers to testing interventions in individuals who have evidence that the disease process is already beginning in the brain aimed at preventing the onset of symptoms and progression to the clinical stages of AD. The majority of these trials are targeting presymptomatic individuals at high genetic risk for AD with anti-amyloid therapies. The Dominantly Inherited Alzheimer Network (DIAN), an international consortium trial in families with autosomal dominant mutations in PSEN1, PSEN2, and APP mutations, is currently testing two monoclonal antibodies against different forms of Aβ: solanezumab and gantereumab in a Phase 2 biomarker trial (Mills et al., 2013).The Alzheimer’s Prevention Initiative (API) trial is testing another monoclonal antibody, crenezumab, in the large Colombian PSEN1 cohort (Reiman et al., 2011). The DIAN and API PSEN-1 trials utilize biomarker outcomes in the first phase of the trials, with plans to continue with longer follow-up to assess cognitive outcomes. API also plans to begin two additional trials in apolipoprotein E ε4 homozygotes, with an active vaccination against Aβ and a BACE inhibitor over the next two years. The TOMMORROW trial is testing pioglitazone, aimed at glucose metabolism, in carriers of the high-risk variant of the TOMM-40 gene.
The Anti-Amyloid Treatment in Asymptomatic Alzheimer’s disease (A4) Trial is complementary to the genetic-at-risk trials mentioned, but identifies older individuals thought to be at high risk for cognitive decline on the basis of biomarker evidence of Aβ accumulation (see Figure 5) (Sperling et al., 2014). The A4 Study will enroll clinically normal older individuals (ages 65-85) into a Phase 3 double-blind trial of solanezumab vs. placebo. The primary outcome of the A4 Study is rate of change on a cognitive composite (Donohue et al., 2014), but also includes several novel participant reported outcomes and computerized testing on an iPad using CogState technology augmented with two episodic memory measures developed on the basis of the cognitive neuroscience and fMRI literature (Rentz et al., 2013; Stark et al., 2013). The A4 Study will acquire PET amyloid imaging, structural and functional MRI on all participants, with CSF measures and Tau PET imaging in a subset. The addition of Tau PET imaging is particularly exciting, as this will allow us to directly test the hypothesis that lowering Aβ, if implemented early enough, can prevent the spread of Tau into the neocortex. If Tau PET indeed proves to be a “theragnostic marker – that is, change in rate of tau spread is predictive of and tracks eventual clinical response, this surrogate would great enhance our ability to conduct shorter, smaller prevention trials in the future. Importantly, the A4 Study also includes an observational arm, the Longitudinal Evaluation of Amyloid Risk and Neurodegeneration (LEARN) study, that will follow 500 older CN who “screen-fail falling below the threshold for Aβ positivity on screening PET amyloid imaging. The comparison of the A4 placebo arm and the LEARN cohort should provide crucial information on the individual risk of decline related to Aβ accumulation as well as other factors that may influence decline in the absence of elevated Aβ markers.
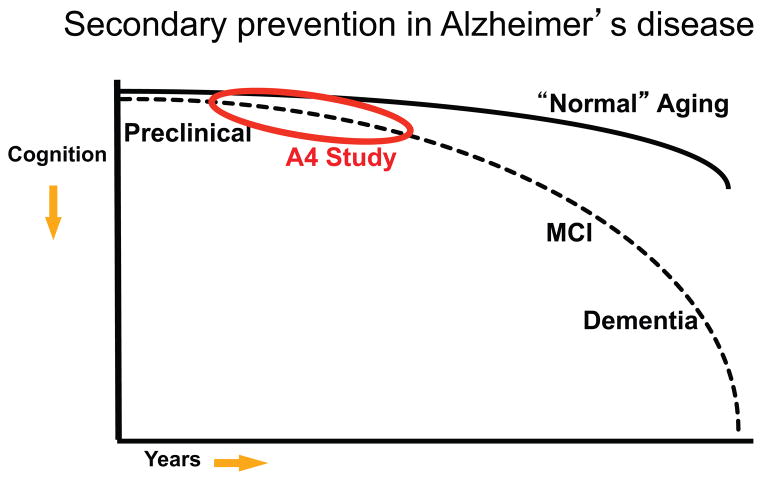
Continuum of Alzheimer’s disease (AD). Cognitive trajectory of “normal aging (starting in late life) demonstrates some decline over decades with increased deterioration in very late life. Preclinical stage of AD at the asymptomatic stage initially demonstrates similar cognitive trajectory as “normal aging but in later stages of preclinical AD demonstrates increased rate of cognitive decline towards mild cognitive impairment (MCI) and dementia due to AD. The Anti-Amyloid Treatment in Asymptomatic AD (A4) Study will test an anti-amyloid interview in preclinical AD aimed at slowing the rate of cognitive decline.
A4 is envisioned as a platform that can be adapted to test multiple potential disease-modifying therapies. The “A5 trial, which will likely test a BACE inhibitor, is currently being planned in a similar preclinical AD population. Ultimately, it is likely that we will need combination therapies (COMBAT trial) to fully halt the progress of AD, as with every other complex disease. These combinations may be multiple mechanisms to lower Aβ burden, such as a BACE inhibitor to decrease the production of Aβ1-42 monomer combined with an antibody that targets aggregated forms of Aβ. Recent animal studies suggest that these approaches might be synergistic in decreasing Aβ, and may have the added benefit in humans of allowing lower doses of each therapeutic to avoid treatment associated adverse events (Jacobsen et al., 2014). Ultimately, it would be ideal to combine anti-Aβ and anti-Tau treatments, particularly in early symptomatic patients. The hope of the secondary prevention trials discussed above is that intervention with an anti-amyloid therapy, if initiated early enough, might stem the spread of Tau, and slow progression of neurodegeneration and prevent memory decline. However, given the preliminary evidence of Tau spread that is present even prior to the stage of MCI, we may need to institute combination secondary prevention trials to fully arrest the development of cognitive decline.
The horns of the dilemma
The particular challenge in AD is that we may have the greatest success with disease-modifying therapy very early in the disease process, likely before the stage of clinical impairment when widespread, irreversible is already present. Perhaps the most frightening prospect is that for some therapeutic mechanisms, such as those aimed at decreasing production of Aβ, such as beta-secretase inhibitors or gamma-secretase modulators, we may ultimately need to intervene even prior to the stage of fulminant amyloidosis and early neurodegeneration.
Although several studies have now demonstrated that individuals with markers of both amyloidosis and neurodegeneration (NIA Stage 2) are at the greatest risk for rapid cognitive decline (Knopman et al., 2012; Mormino et al., 2014a; Vos et al., 2013), these are not necessarily the individuals who are most likely to respond to an anti-amyloid therapy. If indeed, the neurodegenerative process becomes increasingly amyloid-independent as the disease progresses (Hyman, 2011), the best opportunity for intervention with an anti-amyloid agent might be prior to tau spreading or significant hippocampal atrophy detectable on MRI. If on the other hand, Aβ accumulation continues to “fan the flames of tau pathology and neuronal loss, the individuals with evidence of both markers may comprise the ideal cohort for shortening the trial length. It is essential to define the “critical window for successful disease-modifying therapies.
One possibility would be to identify individuals with relatively low levels of Aβ accumulation who are in the rapid phase of accumulation but may still be years or more than a decade before reaching the plateau phase of Aβ deposition. This might be thought of as an “A3 trial – “Ante-Amyloid prevention of Alzheimer’s disease – if we could reliably identify people before the stage of Aβ positivity. The difficulty is that these individuals (perhaps they constitute a preclinical Stage 0.5) would be less likely to have Aβ-related neurodegeneration, and thus much less likely to demonstrate cognitive decline over the course of a 3-5 year secondary prevention trial. It is hoped that identifying reliable surrogate biomarkers, such as tau imaging or improved functional imaging markers if demonstrated to be theragnostic of eventual clinical response in secondary prevention trials, would greatly facilitate these trial designs.
The future of prevention trials
Eventually we hope to begin primary prevention trials, aimed at preventing the pathology from accumulating in the first place. These trial designs would be very challenging currently, as they might require a trial length of more than two decades and medications that are demonstrated safe enough to give to very large numbers of individuals at heterogenous risk of cognitive decline, and inexpensive enough to be given for multiple years. In addition, primary prevention trials will likely require different outcomes than those employed in the current secondary prevention trials, such as web-based iPad home assessments and very short clinical assessments that can be completed in a primary care office setting. However, if the current passive immunotherapy secondary prevention trials are successful at demonstrating that anti-Aβ therapy can bend the curve of very early cognitive decline in preclinical AD, the field will surely implement more feasible trial designs and administration routes for anti-Aβ, such as active vaccination against Aβ fragments and oral medications to decrease Aβ production. Indeed, as one of the limiting factors in AD vaccine development has been raising adequate immunologic titers in older individuals, an active immunization approach may be more successful if started in 50-year-olds with booster immunizations every few years.
Similar prevention trial efforts are beginning in other neurodegenerative diseases, in particular, Huntington’s disease, as risk can be reliably identified through genetic mutation testing, and an estimated date of symptom onset predicted through the number of trinucleotide repeats. As Parkinson’s disease does not manifest clinically until more than 90% of the substantia nigra neurons are lost, Parkinson’s also offers a tremendous opportunity for secondary prevention trials. Ongoing biomarkers efforts in Parkinson’s disease are working to identify reliable markers that can be used to select asymptomatic individuals at risk for motor progression and to track response to therapies in the preclinical stages of Parkinson’s disease.
Although the prospect of preventing neurodegenerative disease progression seems daunting at the moment, we should take encouragement from other fields of medicine that have seen remarkable success through secondary prevention approaches. In particular, the substantial reduction in cardiovascular disease morbidity and mortality achieved with lowering cholesterol levels came about after secondary prevention trials in familial hypercholesterolemia (Leitersdorf et al., 1995) and in older individuals with angina or after a single myocardial infarction, but who still had relatively preserved cardiac function (Group, 1994). One of the earliest challenges faced in that field was the reliable measurement of plasma lipids, and better understanding of the relationship of various cholesterol types to clinical outcomes. It is likely that our simplistic understanding of the AD pathophysiologic process based on current biomarker data will continue to evolve. In particular, the initiation of several large-scale secondary prevention trials in both genetic at-risk and amyloid at-risk older individuals will provide a wealth of data that should serve to disentangle these complex relationships.
Acknowledgments
The authors are supported by the National Institute on Aging: P01AG036694; R01 AG046396R01 AG027435; K24 AG035007; and F32AG044054, and the Alzheimer’s Association. The authors also wish to thank Nayiri Arzoumanian for assistance with editing the manuscript, and all of the research participants who have contributed to our findings.
References
- Ahmed Z, Cooper J, Murray TK, Garn K, McNaughton E, Clarke H, Parhizkar S, Ward MA, Cavallini A, Jackson S, et al. A novel in vivo model of tau propagation with rapid and progressive neurofibrillary tangle pathology: the pattern of spread is determined by connectivity, not proximity. Acta Neuropathol. 2014;127:667–683. [Europe PMC free article] [Abstract] [Google Scholar]
- Aizenstein HJ, Nebes RD, Saxton JA, Price JC, Mathis CA, Tsopelas ND, Ziolko SK, James JA, Snitz BE, Houck PR, et al. Frequent amyloid deposition without significant cognitive impairment among the elderly. Arch Neurol. 2008;65:1509–1517. [Europe PMC free article] [Abstract] [Google Scholar]
- Amariglio RE, Becker JA, Carmasin J, Wadsworth LP, Lorius N, Sullivan C, Maye JE, Gidicsin C, Pepin LC, Sperling RA, et al. Subjective cognitive complaints and amyloid burden in cognitively normal older individuals. Neuropsychologia. 2012;50:2880–2886. [Europe PMC free article] [Abstract] [Google Scholar]
- Arriagada PV, Growdon JH, Hedley-Whyte ET, Hyman BT. Neurofibrillary tangles but not senile plaques parallel duration and severity of Alzheimer’s disease. Neurology. 1992a;42:631–639. [Abstract] [Google Scholar]
- Arriagada PV, Marzloff K, Hyman BT. Distribution of Alzheimer-type pathologic changes in nondemented elderly individuals matches the pattern in Alzheimer’s disease. Neurology. 1992b;42:1681–1688. [Abstract] [Google Scholar]
- Bateman RJ, Xiong C, Benzinger TL, Fagan AM, Goate A, Fox NC, Marcus DS, Cairns NJ, Xie X, Blazey TM, et al. Clinical and Biomarker Changes in Dominantly Inherited Alzheimer’s Disease. N Engl J Med. 2012;367:795–804. [Europe PMC free article] [Abstract] [Google Scholar]
- Becker JA, Hedden T, Carmasin J, Maye J, Rentz DM, Putcha D, Fischl B, Greve DN, Marshall GA, Salloway S, et al. Amyloid-beta associated cortical thinning in clinically normal elderly. Ann Neurol. 2011;69:1032–1042. [Europe PMC free article] [Abstract] [Google Scholar]
- Bennett D, Schneider J, Arvanitakis Z, Kelly J, Aggarwal N, Shah R, Wilson R. Neuropathology of older persons without cognitive impairment from two community-based studies. Neurology. 2006;66:1837–1844. [Abstract] [Google Scholar]
- Benzinger TL, Blazey T, Jack CR, Jr, Koeppe RA, Su Y, Xiong C, Raichle ME, Snyder AZ, Ances BM, Bateman RJ, et al. Regional variability of imaging biomarkers in autosomal dominant Alzheimer’s disease. Proc Natl Acad Sci U S A. 2013;110:E4502–4509. [Europe PMC free article] [Abstract] [Google Scholar]
- Braak H, Alafuzoff I, Arzberger T, Kretzschmar H, Del Tredici K. Staging of Alzheimer disease-associated neurofibrillary pathology using paraffin sections and immunocytochemistry. Acta Neuropathol. 2006;112:389–404. [Europe PMC free article] [Abstract] [Google Scholar]
- Brier MR, Thomas JB, Fagan AM, Hassenstab J, Holtzman DM, Benzinger TL, Morris JC, Ances BM. Functional connectivity and graph theory in preclinical Alzheimer’s disease. Neurobiol Aging. 2014a;35:757–768. [Europe PMC free article] [Abstract] [Google Scholar]
- Brier MR, Thomas JB, Snyder AZ, Wang L, Fagan AM, Benzinger T, Morris JC, Ances BM. Unrecognized preclinical Alzheimer disease confounds rs-fcMRI studies of normal aging. Neurology 2014b [Europe PMC free article] [Abstract] [Google Scholar]
- Buckner RL, Sepulcre J, Talukdar T, Krienen FM, Liu H, Hedden T, Andrews-Hanna JR, Sperling RA, Johnson KA. Cortical hubs revealed by intrinsic functional connectivity: mapping, assessment of stability, and relation to Alzheimer’s disease. J Neurosci. 2009;29:1860–1873. [Europe PMC free article] [Abstract] [Google Scholar]
- Buckner RL, Snyder AZ, Shannon BJ, LaRossa G, Sachs R, Fotenos AF, Sheline YI, Klunk WE, Mathis CA, Morris JC, Mintun MA. Molecular, structural, and functional characterization of Alzheimer’s disease: evidence for a relationship between default activity, amyloid, and memory. J Neurosci. 2005;25:7709–7717. [Abstract] [Google Scholar]
- Buerger K, Alafuzoff I, Ewers M, Pirttila T, Zinkowski R, Hampel H. No correlation between CSF tau protein phosphorylated at threonine 181 with neocortical neurofibrillary pathology in Alzheimer’s disease. Brain. 2007;130:e82. [Abstract] [Google Scholar]
- Celone KA, Calhoun VD, Dickerson BC, Atri A, Chua EF, Miller SL, DePeau K, Rentz DM, Selkoe DJ, Blacker D, et al. Alterations in memory networks in mild cognitive impairment and Alzheimer’s disease: an independent component analysis. J Neurosci. 2006;26:10222–10231. [Abstract] [Google Scholar]
- Chetelat G. Alzheimer disease: Abeta-independent processes-rethinking preclinical AD. Nature reviews Neurology. 2013;9:123–124. [Europe PMC free article] [Abstract] [Google Scholar]
- Chetelat G, Villemagne VL, Pike KE, Baron JC, Bourgeat P, Jones G, Faux NG, Ellis KA, Salvado O, Szoeke C, et al. Larger temporal volume in elderly with high versus low beta-amyloid deposition. Brain. 2010;133:3349–3358. [Abstract] [Google Scholar]
- Chetelat G, Villemagne VL, Villain N, Jones G, Ellis KA, Ames D, Martins RN, Masters CL, Rowe CC. Accelerated cortical atrophy in cognitively normal elderly with high beta-amyloid deposition. Neurology. 2012;78:477–484. [Abstract] [Google Scholar]
- Chhatwal JP, Schultz AP, Johnson K, Benzinger TL, Jack C, Jr, Ances BM, Sullivan CA, Salloway SP, Ringman JM, Koeppe RA, et al. Impaired default network functional connectivity in autosomal dominant Alzheimer disease. Neurology. 2013;81:736–744. [Europe PMC free article] [Abstract] [Google Scholar]
- Chhatwal JP, Sperling RA. Functional MRI of mnemonic networks across the spectrum of normal aging, mild cognitive impairment, and Alzheimer’s disease. J Alzheimers Dis. 2012;31(Suppl 3):S155–167. [Europe PMC free article] [Abstract] [Google Scholar]
- Chien DT, Bahri S, Szardenings AK, Walsh JC, Mu F, Su MY, Shankle WR, Elizarov A, Kolb HC. Early clinical PET imaging results with the novel PHF-tau radioligand [F-18]-T807. J Alzheimers Dis. 2013;34:457–468. [Abstract] [Google Scholar]
- Choi SH, Kim YH, Hebisch M, Sliwinski C, Lee S, D’Avanzo C, Chen H, Hooli B, Asselin C, Muffat J, et al. A three-dimensional human neural cell culture model of Alzheimer’s disease. Nature 2014 [Europe PMC free article] [Abstract] [Google Scholar]
- Cirrito JR, Yamada KA, Finn MB, Sloviter RS, Bales KR, May PC, Schoepp DD, Paul SM, Mennerick S, Holtzman DM. Synaptic activity regulates interstitial fluid amyloid-beta levels in vivo. Neuron. 2005;48:913–922. [Abstract] [Google Scholar]
- Costa DA, Cracchiolo JR, Bachstetter AD, Hughes TF, Bales KR, Paul SM, Mervis RF, Arendash GW, Potter H. Enrichment improves cognition in AD mice by amyloid-related and unrelated mechanisms. Neurobiol Aging. 2007;28:831–844. [Abstract] [Google Scholar]
- de Calignon A, Polydoro M, Suarez-Calvet M, William C, Adamowicz DH, Kopeikina KJ, Pitstick R, Sahara N, Ashe KH, Carlson GA, et al. Propagation of tau pathology in a model of early Alzheimer’s disease. Neuron. 2012;73:685–697. [Europe PMC free article] [Abstract] [Google Scholar]
- De Meyer G, Shapiro F, Vanderstichele H, Vanmechelen E, Engelborghs S, De Deyn PP, Coart E, Hansson O, Minthon L, Zetterberg H, et al. Diagnosis-independent Alzheimer disease biomarker signature in cognitively normal elderly people. Arch Neurol. 2010;67:949–956. [Europe PMC free article] [Abstract] [Google Scholar]
- Delacourte A, Sergeant N, Wattez A, Maurage CA, Lebert F, Pasquier F, David JP. Tau aggregation in the hippocampal formation: an ageing or a pathological process? Experimental gerontology. 2002;37:1291–1296. [Abstract] [Google Scholar]
- Desikan RS, McEvoy LK, Thompson WK, Holland D, Brewer JB, Aisen PS, Sperling RA, Dale AM. Amyloid-beta-Associated Clinical Decline Occurs Only in the Presence of Elevated P-tau. Arch Neurol. 2012;69:709–713. [Europe PMC free article] [Abstract] [Google Scholar]
- Desikan RS, McEvoy LK, Thompson WK, Holland D, Roddey JC, Blennow K, Aisen PS, Brewer JB, Hyman BT, Dale AM. Amyloid-beta associated volume loss occurs only in the presence of phospho-tau. Ann Neurol. 2011;70:657–661. [Europe PMC free article] [Abstract] [Google Scholar]
- Dickerson BC, Bakkour A, Salat DH, Feczko E, Pacheco J, Greve DN, Grodstein F, Wright CI, Blacker D, Rosas HD, et al. The Cortical Signature of Alzheimer’s Disease: Regionally Specific Cortical Thinning Relates to Symptom Severity in Very Mild to Mild AD Dementia and is Detectable in Asymptomatic Amyloid-Positive Individuals. Cereb Cortex 2008 [Europe PMC free article] [Abstract] [Google Scholar]
- Donohue MC, Sperling RA, Salmon DP, Rentz DM, Raman R, Thomas RG, Weiner M, Aisen PS, et al. for the Australian Imaging B, Lifestyle Flagship Study of A. The Preclinical Alzheimer Cognitive Composite: Measuring Amyloid-Related Decline. JAMA neurology 2014 [Europe PMC free article] [Abstract] [Google Scholar]
- Doraiswamy PM, Sperling RA, Coleman RE, Johnson KA, Reiman EM, Davis MD, Grundman M, Sabbagh MN, Sadowsky CH, Fleisher AS, et al. Amyloid-beta assessed by florbetapir F 18 PET and 18-month cognitive decline: A multicenter study. Neurology 2012 [Europe PMC free article] [Abstract] [Google Scholar]
- Doraiswamy PM, Sperling RA, Johnson K, Reiman EM, Wong TZ, Sabbagh MN, Sadowsky CH, Fleisher AS, Carpenter A, Joshi AD, et al. Florbetapir F 18 amyloid PET and 36-month cognitive decline:a prospective multicenter study. Molecular psychiatry 2014 [Europe PMC free article] [Abstract] [Google Scholar]
- Dore V, Villemagne VL, Bourgeat P, Fripp J, Acosta O, Chetelat G, Zhou L, Martins R, Ellis KA, Masters CL, et al. Cross-sectional and longitudinal analysis of the relationship between Abeta deposition, cortical thickness, and memory in cognitively unimpaired individuals and in Alzheimer disease. JAMA neurology. 2013;70:903–911. [Abstract] [Google Scholar]
- Dubois B, Feldman HH, Jacova C, Cummings JL, Dekosky ST, Barberger-Gateau P, Delacourte A, Frisoni G, Fox NC, Galasko D, et al. Revising the definition of Alzheimer’s disease: a new lexicon. Lancet Neurol. 2010;9:1118–1127. [Abstract] [Google Scholar]
- Dubois B, Feldman HH, Jacova C, Dekosky ST, Barberger-Gateau P, Cummings J, Delacourte A, Galasko D, Gauthier S, Jicha G, et al. Research criteria for the diagnosis of Alzheimer’s disease: revising the NINCDS-ADRDA criteria. Lancet Neurol. 2007;6:734–746. [Abstract] [Google Scholar]
- Dubois B, Feldman HH, Jacova C, Hampel H, Molinuevo JL, Blennow K, DeKosky ST, Gauthier S, Selkoe D, Bateman R, et al. Advancing research diagnostic criteria for Alzheimer’s disease: the IWG-2 criteria. Lancet Neurol. 2014;13:614–629. [Abstract] [Google Scholar]
- Duyckaerts C, Hauw JJ. Prevalence, incidence and duration of Braak’s stages in the general population: can we know? Neurobiol Aging. 1997;18:362–369. discussion 389–392. [Abstract] [Google Scholar]
- Eberling JL, Wu C, Tong-Turnbeaugh R, Jagust WJ. Estrogen- and tamoxifen-associated effects on brain structure and function. Neuroimage. 2004;21:364–371. [Abstract] [Google Scholar]
- Elman JA, Oh H, Madison CM, Baker SL, Vogel JW, Marks SM, Crowley S, O’Neil JP, Jagust WJ. Neural compensation in older people with brain amyloid-beta deposition. Nat Neurosci. 2014;17:1316–1318. [Europe PMC free article] [Abstract] [Google Scholar]
- Fagan AM, Mintun MA, Shah AR, Aldea P, Roe CM, Mach RH, Marcus D, Morris JC, Holtzman DM. Cerebrospinal fluid tau and ptau(181) increase with cortical amyloid deposition in cognitively normal individuals: implications for future clinical trials of Alzheimer’s disease. EMBO Mol Med. 2009;1:371–380. [Europe PMC free article] [Abstract] [Google Scholar]
- Fagan AM, Roe CM, Xiong C, Mintun MA, Morris JC, Holtzman DM. Cerebrospinal fluid tau/beta-amyloid(42) ratio as a prediction of cognitive decline in nondemented older adults. Arch Neurol. 2007;64:343–349. [Abstract] [Google Scholar]
- Filippini N, MacIntosh BJ, Hough MG, Goodwin GM, Frisoni GB, Smith SM, Matthews PM, Beckmann CF, Mackay CE. Distinct patterns of brain activity in young carriers of the APOE-epsilon4 allele. Proc Natl Acad Sci U S A. 2009;106:7209–7214. [Europe PMC free article] [Abstract] [Google Scholar]
- Fjell AM, McEvoy L, Holland D, Dale AM, Walhovd KB Alzheimer’s Disease Neuroimaging I. What is normal in normal aging? Effects of aging, amyloid and Alzheimer’s disease on the cerebral cortex and the hippocampus. Prog Neurobiol. 2014;117:20–40. [Europe PMC free article] [Abstract] [Google Scholar]
- Fleisher AS, Chen K, Quiroz YT, Jakimovich LJ, Gomez MG, Langois CM, Langbaum JB, Ayutyanont N, Roontiva A, Thiyyagura P, et al. Florbetapir PET analysis of amyloid-beta deposition in the presenilin 1 E280A autosomal dominant Alzheimer’s disease kindred: a cross-sectional study. Lancet Neurol. 2012;11:1057–1065. [Europe PMC free article] [Abstract] [Google Scholar]
- Fleisher AS, Sherzai A, Taylor C, Langbaum JB, Chen K, Buxton RB. Resting-state BOLD networks versus task-associated functional MRI for distinguishing Alzheimer’s disease risk groups. Neuroimage. 2009;47:1678–1690. [Europe PMC free article] [Abstract] [Google Scholar]
- Fotenos AF, Mintun MA, Snyder AZ, Morris JC, Buckner RL. Brain volume decline in aging: evidence for a relation between socioeconomic status, preclinical Alzheimer disease, and reserve. Arch Neurol. 2008;65:113–120. [Abstract] [Google Scholar]
- Geser F, Robinson JL, Malunda JA, Xie SX, Clark CM, Kwong LK, Moberg PJ, Moore EM, Van Deerlin VM, Lee VM, et al. Pathological 43-kDa transactivation response DNA-binding protein in older adults with and without severe mental illness. Arch Neurol. 2010;67:1238–1250. [Europe PMC free article] [Abstract] [Google Scholar]
- Gomez-Isla T, Hollister R, West H, Mui S, Growdon JH, Petersen RC, Parisi JE, Hyman BT. Neuronal loss correlates with but exceeds neurofibrillary tangles in Alzheimer’s disease. Ann Neurol. 1997;41:17–24. [Abstract] [Google Scholar]
- Gomperts SN, Rentz DM, Moran E, Becker JA, Locascio JJ, Klunk WE, Mathis CA, Elmaleh DR, Shoup T, Fischman AJ, et al. Imaging amyloid deposition in Lewy body diseases. Neurology. 2008;71:903–910. [Europe PMC free article] [Abstract] [Google Scholar]
- Greicius MD, Srivastava G, Reiss AL, Menon V. Default-mode network activity distinguishes Alzheimer’s disease from healthy aging: evidence from functional MRI. Proc Natl Acad Sci U S A. 2004;101:4637–4642. [Europe PMC free article] [Abstract] [Google Scholar]
- Group SSSS. Randomised trial of cholesterol lowering in 4444 patients with coronary heart disease: the Scandinavian Simvastatin Survival Study (4S) Lancet. 1994;344:1383–1389. [Abstract] [Google Scholar]
- Hampel H. Amyloid-beta and cognition in aging and Alzheimer’s disease: molecular and neurophysiological mechanisms. J Alzheimers Dis. 2013;33(Suppl 1):S79–86. [Abstract] [Google Scholar]
- Hardy J, Selkoe DJ. The amyloid hypothesis of Alzheimer’s disease: progress and problems on the road to therapeutics. Science. 2002;297:353–356. [Abstract] [Google Scholar]
- He Y, Chen Z, Gong G, Evans A. Neuronal networks in Alzheimer’s disease. The Neuroscientist : a review journal bringing neurobiology, neurology and psychiatry. 2009;15:333–350. [Abstract] [Google Scholar]
- Hedden T, Van Dijk KR, Becker JA, Mehta A, Sperling RA, Johnson KA, Buckner RL. Disruption of functional connectivity in clinically normal older adults harboring amyloid burden. J Neurosci. 2009;29:12686–12694. [Europe PMC free article] [Abstract] [Google Scholar]
- Huijbers W, Mormino EC, Wigman SE, Ward AM, Vannini P, McLaren DG, Becker JA, Schultz AP, Hedden T, Johnson KA, Sperling RA. Amyloid Deposition Is Linked to Aberrant Entorhinal Activity among Cognitively Normal Older Adults. J Neurosci. 2014;34:5200–5210. [Europe PMC free article] [Abstract] [Google Scholar]
- Hyman BT. Amyloid-dependent and amyloid-independent stages of Alzheimer disease. Arch Neurol. 2011;68:1062–1064. [Abstract] [Google Scholar]
- Hyman BT, Phelps CH, Beach TG, Bigio EH, Cairns NJ, Carrillo MC, Dickson DW, Duyckaerts C, Frosch MP, Masliah E, et al. National Institute on Aging-Alzheimer’s Association guidelines for the neuropathologic assessment of Alzheimer’s disease. Alzheimers Dement. 2012;8:1–13. [Europe PMC free article] [Abstract] [Google Scholar]
- Jack CR, Jr, Dickson DW, Parisi JE, Xu YC, Cha RH, O’Brien PC, Edland SD, Smith GE, Boeve BF, Tangalos EG, et al. Antemortem MRI findings correlate with hippocampal neuropathology in typical aging and dementia. Neurology. 2002;58:750–757. [Europe PMC free article] [Abstract] [Google Scholar]
- Jack CR, Jr, Knopman DS, Jagust WJ, Petersen RC, Weiner MW, Aisen PS, Shaw LM, Vemuri P, Wiste HJ, Weigand SD, et al. Tracking pathophysiological processes in Alzheimer’s disease: an updated hypothetical model of dynamic biomarkers. Lancet Neurol. 2013a;12:207–216. [Europe PMC free article] [Abstract] [Google Scholar]
- Jack CR, Jr, Knopman DS, Jagust WJ, Shaw LM, Aisen PS, Weiner MW, Petersen RC, Trojanowski JQ. Hypothetical model of dynamic biomarkers of the Alzheimer’s pathological cascade. Lancet Neurol. 2010;9:119–128. [Europe PMC free article] [Abstract] [Google Scholar]
- Jack CR, Jr, Knopman DS, Weigand SD, Wiste HJ, Vemuri P, Lowe V, Kantarci K, Gunter JL, Senjem ML, Ivnik RJ, et al. An operational approach to National Institute on Aging-Alzheimer’s Association criteria for preclinical Alzheimer disease. Ann Neurol. 2012;71:765–775. [Europe PMC free article] [Abstract] [Google Scholar]
- Jack CR, Jr, Lowe VJ, Senjem ML, Weigand SD, Kemp BJ, Shiung MM, Knopman DS, Boeve BF, Klunk WE, Mathis CA, Petersen RC. 11C PiB and structural MRI provide complementary information in imaging of Alzheimer’s disease and amnestic mild cognitive impairment. Brain. 2008;131:665–680. [Europe PMC free article] [Abstract] [Google Scholar]
- Jack CR, Jr, Wiste HJ, Knopman DS, Vemuri P, Mielke MM, Weigand SD, Senjem ML, Gunter JL, Lowe V, Gregg BE, et al. Rates of beta-amyloid accumulation are independent of hippocampal neurodegeneration. Neurology. 2014;82:1605–1612. [Europe PMC free article] [Abstract] [Google Scholar]
- Jack CR, Jr, Wiste HJ, Weigand SD, Knopman DS, Lowe V, Vemuri P, Mielke MM, Jones DT, Senjem ML, Gunter JL, et al. Amyloid-first and neurodegeneration-first profiles characterize incident amyloid PET positivity. Neurology. 2013b;81:1732–1740. [Europe PMC free article] [Abstract] [Google Scholar]
- Jacobsen H, Ozmen L, Caruso A, Narquizian R, Hilpert H, Jacobsen B, Terwel D, Tanghe A, Bohrmann B. Combined treatment with a BACE inhibitor and anti-Abeta antibody gantenerumab enhances amyloid reduction in APPLondon mice. J Neurosci. 2014;34:11621–11630. [Europe PMC free article] [Abstract] [Google Scholar]
- Jagust WJ, Landau SM, Shaw LM, Trojanowski JQ, Koeppe RA, Reiman EM, Foster NL, Petersen RC, Weiner MW, Price JC, Mathis CA. Relationships between biomarkers in aging and dementia. Neurology. 2009;73:1193–1199. [Europe PMC free article] [Abstract] [Google Scholar]
- Jagust WJ, Mormino EC. Lifespan brain activity, beta-amyloid, and Alzheimer’s disease. Trends in cognitive sciences. 2011;15:520–526. [Europe PMC free article] [Abstract] [Google Scholar]
- Jonsson T, Atwal JK, Steinberg S, Snaedal J, Jonsson PV, Bjornsson S, Stefansson H, Sulem P, Gudbjartsson D, Maloney J, et al. A mutation in APP protects against Alzheimer’s disease and age-related cognitive decline. Nature. 2012;488:96–99. [Abstract] [Google Scholar]
- Kantarci K, Lowe V, Przybelski SA, Weigand SD, Senjem ML, Ivnik RJ, Preboske GM, Roberts R, Geda YE, Boeve BF, et al. APOE modifies the association between Abeta load and cognition in cognitively normal older adults. Neurology. 2012;78:232–240. [Europe PMC free article] [Abstract] [Google Scholar]
- Kawas CH, Greenia DE, Bullain SS, Clark CM, Pontecorvo MJ, Joshi AD, Corrada MM. Amyloid imaging and cognitive decline in nondemented oldest-old: the 90+ Study. Alzheimers Dement. 2013;9:199–203. [Europe PMC free article] [Abstract] [Google Scholar]
- Kennedy KM, Rodrigue KM, Devous MD, Sr, Hebrank AC, Bischof GN, Park DC. Effects of beta-amyloid accumulation on neural function during encoding across the adult lifespan. Neuroimage. 2012;62:1–8. [Europe PMC free article] [Abstract] [Google Scholar]
- Knopman DS, Jack CR, Jr, Wiste HJ, Lundt ES, Weigand SD, Vemuri P, Lowe VJ, Kantarci K, Gunter JL, Senjem ML, et al. F-fluorodeoxyglucose positron emission tomography, aging, and apolipoprotein E genotype in cognitively normal persons. Neurobiol Aging 2014 [Europe PMC free article] [Abstract] [Google Scholar]
- Knopman DS, Jack CR, Jr, Wiste HJ, Weigand SD, Vemuri P, Lowe V, Kantarci K, Gunter JL, Senjem ML, Ivnik RJ, et al. Short-term clinical outcomes for stages of NIA-AA preclinical Alzheimer disease. Neurology. 2012;78:1576–1582. [Europe PMC free article] [Abstract] [Google Scholar]
- Knopman DS, Jack CR, Jr, Wiste HJ, Weigand SD, Vemuri P, Lowe VJ, Kantarci K, Gunter JL, Senjem ML, Mielke MM, et al. Selective worsening of brain injury biomarker abnormalities in cognitively normal elderly persons with beta-amyloidosis. JAMA neurology. 2013;70:1030–1038. [Europe PMC free article] [Abstract] [Google Scholar]
- Kryscio RJ, Abner EL, Cooper GE, Fardo DW, Jicha GA, Nelson PT, Smith CD, Van Eldik LJ, Wan L, Schmitt FA. Self-reported memory complaints: Implications from a longitudinal cohort with autopsies. Neurology 2014 [Europe PMC free article] [Abstract] [Google Scholar]
- Landau SM, Marks SM, Mormino EC, Rabinovici GD, Oh H, O’Neil JP, Wilson RS, Jagust WJ. Association of lifetime cognitive engagement and low beta-amyloid deposition. Arch Neurol. 2012;69:623–629. [Europe PMC free article] [Abstract] [Google Scholar]
- Lee HG, Casadesus G, Zhu X, Takeda A, Perry G, Smith MA. Challenging the amyloid cascade hypothesis: senile plaques and amyloid-beta as protective adaptations to Alzheimer disease. Ann N Y Acad Sci. 2004;1019:1–4. [Abstract] [Google Scholar]
- Leitersdorf E, Muratti EN, Eliav O, Peters TK. Efficacy and safety of triple therapy (fluvastatin-bezafibrate-cholestyramine) for severe familial hypercholesterolemia. The American journal of cardiology. 1995;76:84A–88A. [Abstract] [Google Scholar]
- Li G, Millard SP, Peskind ER, Zhang J, Yu CE, Leverenz JB, Mayer C, Shofer JS, Raskind MA, Quinn JF, et al. Cross-sectional and longitudinal relationships between cerebrospinal fluid biomarkers and cognitive function in people without cognitive impairment from across the adult life span. JAMA neurology. 2014;71:742–751. [Europe PMC free article] [Abstract] [Google Scholar]
- Li S, Jin M, Zhang D, Yang T, Koeglsperger T, Fu H, Selkoe DJ. Environmental novelty activates beta2-adrenergic signaling to prevent the impairment of hippocampal LTP by Abeta oligomers. Neuron. 2013;77:929–941. [Europe PMC free article] [Abstract] [Google Scholar]
- Lim HK, Nebes R, Snitz B, Cohen A, Mathis C, Price J, Weissfeld L, Klunk W, Aizenstein HJ. Regional amyloid burden and intrinsic connectivity networks in cognitively normal elderly subjects. Brain 2014a [Europe PMC free article] [Abstract] [Google Scholar]
- Lim YY, Ellis KA, Ames D, Darby D, Harrington K, Martins RN, Masters CL, Rowe C, Savage G, Szoeke C, et al. Abeta amyloid, cognition, and APOE genotype in healthy older adults. Alzheimers Dement 2012 Epub ahead of print. [Abstract] [Google Scholar]
- Lim YY, Maruff P, Pietrzak RH, Ames D, Ellis KA, Harrington K, Lautenschlager NT, Szoeke C, Martins RN, Masters CL, et al. Effect of amyloid on memory and non-memory decline from preclinical to clinical Alzheimer’s disease. Brain. 2014b;137:221–231. [Abstract] [Google Scholar]
- Lim YY, Maruff P, Pietrzak RH, Ellis KA, Darby D, Ames D, Harrington K, Martins RN, Masters CL, Szoeke C, et al. Abeta and cognitive change: Examining the preclinical and prodromal stages of Alzheimer’s disease. Alzheimers Dement 2014c [Abstract] [Google Scholar]
- Lim YY, Pietrzak RH, Ellis KA, Jaeger J, Harrington K, Ashwood T, Szoeke C, Martins RN, Bush AI, Masters CL, et al. Rapid Decline in Episodic Memory in Healthy Older Adults with High Amyloid-beta. J Alzheimers Dis 2012 [Abstract] [Google Scholar]
- Lim YY, Villemagne VL, Laws SM, Ames D, Pietrzak RH, Ellis KA, Harrington KD, Bourgeat P, Salvado O, Darby D, et al. BDNF Val66Met, Abeta amyloid, and cognitive decline in preclinical Alzheimer’s disease. Neurobiol Aging. 2013;34:2457–2464. [Abstract] [Google Scholar]
- Liu L, Drouet V, Wu JW, Witter MP, Small SA, Clelland C, Duff K. Trans-synaptic spread of tau pathology in vivo. PLoS One. 2012;7:e31302. [Europe PMC free article] [Abstract] [Google Scholar]
- Lupien SJ, de Leon M, de Santi S, Convit A, Tarshish C, Nair NP, Thakur M, McEwen BS, Hauger RL, Meaney MJ. Cortisol levels during human aging predict hippocampal atrophy and memory deficits. Nat Neurosci. 1998;1:69–73. [Abstract] [Google Scholar]
- Lustig C, Snyder AZ, Bhakta M, O’Brien KC, McAvoy M, Raichle ME, Morris JC, Buckner RL. Functional deactivations: change with age and dementia of the Alzheimer type. Proc Natl Acad Sci U S A. 2003;100:14504–14509. [Europe PMC free article] [Abstract] [Google Scholar]
- Machulda MM, Jones DT, Vemuri P, McDade E, Avula R, Przybelski S, Boeve BF, Knopman DS, Petersen RC, Jack CR., Jr Effect of APOE epsilon4 status on intrinsic network connectivity in cognitively normal elderly subjects. Arch Neurol. 2011;68:1131–1136. [Europe PMC free article] [Abstract] [Google Scholar]
- Marsland AL, Gianaros PJ, Abramowitch SM, Manuck SB, Hariri AR. Interleukin-6 covaries inversely with hippocampal grey matter volume in middle-aged adults. Biol Psychiatry. 2008;64:484–490. [Europe PMC free article] [Abstract] [Google Scholar]
- Maruyama M, Shimada H, Suhara T, Shinotoh H, Ji B, Maeda J, Zhang MR, Trojanowski JQ, Lee VM, Ono M, et al. Imaging of tau pathology in a tauopathy mouse model and in Alzheimer patients compared to normal controls. Neuron. 2013;79:1094–1108. [Europe PMC free article] [Abstract] [Google Scholar]
- Mattsson N, Insel PS, Nosheny R, Tosun D, Trojanowski JQ, Shaw LM, Jack CR, Jr, Donohue MC, Weiner MW and for the Alzheimer’s Disease Neuroimaging I. Emerging beta-Amyloid Pathology and Accelerated Cortical Atrophy. JAMA neurology 2014 [Europe PMC free article] [Abstract] [Google Scholar]
- Mawuenyega KG, Sigurdson W, Ovod V, Munsell L, Kasten T, Morris JC, Yarasheski KE, Bateman RJ. Decreased clearance of CNS beta-amyloid in Alzheimer’s disease. Science. 2010;330:1774. [Europe PMC free article] [Abstract] [Google Scholar]
- Mills SM, Mallmann J, Santacruz AM, Fuqua A, Carril M, Aisen PS, Althage MC, Belyew S, Benzinger TL, Brooks WS, et al. Preclinical trials in autosomal dominant AD: implementation of the DIAN-TU trial. Revue neurologique. 2013;169:737–743. [Europe PMC free article] [Abstract] [Google Scholar]
- Mintun MA, Larossa GN, Sheline YI, Dence CS, Lee SY, Mach RH, Klunk WE, Mathis CA, DeKosky ST, Morris JC. [11C]PIB in a nondemented population: potential antecedent marker of Alzheimer disease. Neurology. 2006;67:446–452. [Abstract] [Google Scholar]
- Montine TJ. Prevalence estimates for latent neurodegenerative disease. Toxicol Pathol. 2011;39:99–102. [Europe PMC free article] [Abstract] [Google Scholar]
- Mormino EC, Betensky RA, Hedden T, Schultz AP, Amariglio RE, Rentz DM, Johnson KA, Sperling RA. Synergistic Effect of beta-Amyloid and Neurodegeneration on Cognitive Decline in Clinically Normal Individuals. JAMA neurology 2014a [Europe PMC free article] [Abstract] [Google Scholar]
- Mormino EC, Betensky RA, Hedden T, Schultz AP, Ward A, Huijbers W, Rentz DM, Johnson KA, Sperling RA, et al. Alzheimer’s Disease Neuroimaging I. Amyloid and APOE epsilon4 interact to influence short-term decline in preclinical Alzheimer disease. Neurology. 2014b;82:1760–1767. [Europe PMC free article] [Abstract] [Google Scholar]
- Mormino EC, Smiljic A, Hayenga AO, Onami SH, Greicius MD, Rabinovici GD, Janabi M, Baker SL, Yen IV, Madison CM, et al. Relationships between beta-amyloid and functional connectivity in different components of the default mode network in aging. Cereb Cortex. 2011;21:2399–2407. [Europe PMC free article] [Abstract] [Google Scholar]
- Morris JC, Storandt M, McKeel DW, Jr, Rubin EH, Price JL, Grant EA, Berg L. Cerebral amyloid deposition and diffuse plaques in “normal” aging: Evidence for presymptomatic and very mild Alzheimer’s disease. Neurology. 1996;46:707–719. [Abstract] [Google Scholar]
- Morrison JH, Hof PR. Life and death of neurons in the aging brain. Science. 1997;278:412–419. [Abstract] [Google Scholar]
- Myers N, Pasquini L, Gottler J, Grimmer T, Koch K, Ortner M, Neitzel J, Muhlau M, Forster S, Kurz A, et al. Within-patient correspondence of amyloid-beta and intrinsic network connectivity in Alzheimer’s disease. Brain 2014 [Europe PMC free article] [Abstract] [Google Scholar]
- Nelson PT, Alafuzoff I, Bigio EH, Bouras C, Braak H, Cairns NJ, Castellani RJ, Crain BJ, Davies P, Del Tredici K, et al. Correlation of Alzheimer disease neuropathologic changes with cognitive status: a review of the literature. J Neuropathol Exp Neurol. 2012;71:362–381. [Europe PMC free article] [Abstract] [Google Scholar]
- Nosheny RL, Insel PS, Truran D, Schuff N, Jack CR, Jr, Aisen PS, Shaw LM, Trojanowski JQ, Weiner MW. Variables associated with hippocampal atrophy rate in normal aging and mild cognitive impairment. Neurobiol Aging 2014 [Europe PMC free article] [Abstract] [Google Scholar]
- O’Brien JL, O’Keefe KM, LaViolette PS, DeLuca AN, Blacker D, Dickerson BC, Sperling RA. Longitudinal fMRI in elderly reveals loss of hippocampal activation with clinical decline. Neurology. 2010;74:1969–1976. [Europe PMC free article] [Abstract] [Google Scholar]
- Oddo S, Caccamo A, Tran L, Lambert MP, Glabe CG, Klein WL, LaFerla FM. Temporal profile of amyloid-beta (Abeta) oligomerization in an in vivo model of Alzheimer disease. A link between Abeta and tau pathology. J Biol Chem. 2006;281:1599–1604. [Abstract] [Google Scholar]
- Palop JJ, Mucke L. Amyloid-beta-induced neuronal dysfunction in Alzheimer’s disease: from synapses toward neural networks. Nat Neurosci. 2010;13:812–818. [Europe PMC free article] [Abstract] [Google Scholar]
- Perez-Nievas BG, Stein TD, Tai HC, Dols-Icardo O, Scotton TC, Barroeta-Espar I, Fernandez-Carballo L, de Munain EL, Perez J, Marquie M, et al. Dissecting phenotypic traits linked to human resilience to Alzheimer’s pathology. Brain. 2013;136:2510–2526. [Europe PMC free article] [Abstract] [Google Scholar]
- Perrotin A, Mormino EC, Madison CM, Hayenga AO, Jagust WJ. Subjective cognition and amyloid deposition imaging: a pittsburgh compound B positron emission tomography study in normal elderly individuals. Arch Neurol. 2012;69:223–229. [Europe PMC free article] [Abstract] [Google Scholar]
- Petrella JR, Sheldon FC, Prince SE, Calhoun VD, Doraiswamy PM. Default mode network connectivity in stable vs progressive mild cognitive impairment. Neurology. 2011;76:511–517. [Europe PMC free article] [Abstract] [Google Scholar]
- Pihlajamaki M, KOK, Bertram L, Tanzi RE, Dickerson BC, Blacker D, Albert MS, Sperling RA. Evidence of altered posteromedial cortical FMRI activity in subjects at risk for Alzheimer disease. Alzheimer Dis Assoc Disord. 2010;24:28–36. [Europe PMC free article] [Abstract] [Google Scholar]
- Price JL, Morris JC. Tangles and plaques in nondemented aging and “preclinical” Alzheimer’s disease. Ann Neurol. 1999;45:358–368. [Abstract] [Google Scholar]
- Putcha D, Brickhouse M, O’Keefe K, Sullivan C, Rentz D, Marshall G, Dickerson B, Sperling R. Hippocampal hyperactivation associated with cortical thinning in Alzheimer’s disease signature regions in non-demented elderly adults. J Neurosci. 2011;31:17680–17688. [Europe PMC free article] [Abstract] [Google Scholar]
- Quiroz YT, Budson AE, Celone K, Ruiz A, Newmark R, Castrillon G, Lopera F, Stern CE. Hippocampal hyperactivation in presymptomatic familial Alzheimer’s disease. Ann Neurol. 2010;68:865–875. [Europe PMC free article] [Abstract] [Google Scholar]
- Rami L, Sala-Llonch R, Sole-Padulles C, Fortea J, Olives J, Llado A, Pena-Gomez C, Balasa M, Bosch B, Antonell A, et al. Distinct functional activity of the precuneus and posterior cingulate cortex during encoding in the preclinical stage of Alzheimer’s disease. J Alzheimers Dis. 2012;31:517–526. [Abstract] [Google Scholar]
- Raz N, Gunning-Dixon F, Head D, Rodrigue KM, Williamson A, Acker JD. Aging, sexual dimorphism, and hemispheric asymmetry of the cerebral cortex: replicability of regional differences in volume. Neurobiol Aging. 2004;25:377–396. [Abstract] [Google Scholar]
- Reiman EM, Langbaum JB, Fleisher AS, Caselli RJ, Chen K, Ayutyanont N, Quiroz YT, Kosik KS, Lopera F, Tariot PN. Alzheimer’s Prevention Initiative: a plan to accelerate the evaluation of presymptomatic treatments. J Alzheimers Dis. 2011;26(Suppl 3):321–329. [Europe PMC free article] [Abstract] [Google Scholar]
- Reiman EM, Quiroz YT, Fleisher AS, Chen K, Velez-Pardo C, Jimenez-Del-Rio M, Fagan AM, Shah AR, Alvarez S, Arbelaez A, et al. Brain imaging and fluid biomarker analysis in young adults at genetic risk for autosomal dominant Alzheimer’s disease in the presenilin 1 E280A kindred: a case-control study. Lancet Neurol. 2012;11:1048–1056. [Europe PMC free article] [Abstract] [Google Scholar]
- Rentz DM, Amariglio RE, Becker JA, Frey M, Olson LE, Frishe K, Carmasin J, Maye JE, Johnson KA, Sperling RA. Face-name associative memory performance is related to amyloid burden in normal elderly. Neuropsychologia. 2011;49:2776–2783. [Europe PMC free article] [Abstract] [Google Scholar]
- Rentz DM, Locascio JJ, Becker JA, Moran EK, Eng E, Buckner RL, Sperling RA, Johnson KA. Cognition, reserve, and amyloid deposition in normal aging. Ann Neurol. 2010;67:353–364. [Europe PMC free article] [Abstract] [Google Scholar]
- Rentz DM, Parra Rodriguez MA, Amariglio R, Stern Y, Sperling R, Ferris S. Promising developments in neuropsychological approaches for the detection of preclinical Alzheimer’s disease: a selective review. Alzheimers Res Ther. 2013;5:58. [Europe PMC free article] [Abstract] [Google Scholar]
- Resnick SM, Sojkova J, Zhou Y, An Y, Ye W, Holt DP, Dannals RF, Mathis CA, Klunk WE, Ferrucci L, et al. Longitudinal cognitive decline is associated with fibrillar amyloid-beta measured by [11C]PiB. Neurology. 2010;74:807–815. [Europe PMC free article] [Abstract] [Google Scholar]
- Rodrigue KM, Kennedy KM, Devous MD, Sr, Rieck JR, Hebrank AC, Diaz-Arrastia R, Mathews D, Park DC. β-Amyloid burden in healthy aging: Regional distribution and cognitive consequences. Neurology. 2012;78:387–395. [Europe PMC free article] [Abstract] [Google Scholar]
- Roe CM, Fagan AM, Grant EA, Marcus DS, Benzinger TL, Mintun MA, Holtzman DM, Morris JC. Cerebrospinal fluid biomarkers, education, brain volume, and future cognition. Arch Neurol. 2011;68:1145–1151. [Europe PMC free article] [Abstract] [Google Scholar]
- Rowe CC, Bourgeat P, Ellis KA, Brown B, Lim YY, Mulligan R, Jones G, Maruff P, Woodward M, Price R, et al. Predicting Alzheimer disease with beta-amyloid imaging: results from the Australian imaging, biomarkers, and lifestyle study of ageing. Ann Neurol. 2013;74:905–913. [Abstract] [Google Scholar]
- Rowe CC, Ellis KA, Rimajova M, Bourgeat P, Pike KE, Jones G, Fripp J, Tochon-Danguy H, Morandeau L, O’Keefe G, et al. Amyloid imaging results from the Australian Imaging, Biomarkers and Lifestyle (AIBL) study of aging. Neurobiol Aging. 2010;31:1275–1283. [Abstract] [Google Scholar]
- Schott JM, Bartlett JW, Fox NC, Barnes J. Increased brain atrophy rates in cognitively normal older adults with low cerebrospinal fluid Abeta1-42. Ann Neurol. 2010;68:825–834. [Abstract] [Google Scholar]
- Shankar GM, Li S, Mehta TH, Garcia-Munoz A, Shepardson NE, Smith I, Brett FM, Farrell MA, Rowan MJ, Lemere CA, et al. Amyloid-beta protein dimers isolated directly from Alzheimer’s brains impair synaptic plasticity and memory. Nat Med. 2008;14:837–842. [Europe PMC free article] [Abstract] [Google Scholar]
- Sheline YI, Raichle ME, Snyder AZ, Morris JC, Head D, Wang S, Mintun MA. Amyloid plaques disrupt resting state default mode network connectivity in cognitively normal elderly. Biol Psychiatry. 2009;67:584–587. [Europe PMC free article] [Abstract] [Google Scholar]
- Sorg C, Riedl V, Muhlau M, Calhoun VD, Eichele T, Laer L, Drzezga A, Forstl H, Kurz A, Zimmer C, Wohlschlager AM. Selective changes of resting-state networks in individuals at risk for Alzheimer’s disease. Proc Natl Acad Sci U S A. 2007;104:18760–18765. [Europe PMC free article] [Abstract] [Google Scholar]
- Sowell ER, Peterson BS, Thompson PM, Welcome SE, Henkenius AL, Toga AW. Mapping cortical change across the human life span. Nat Neurosci. 2003;6:309–315. [Abstract] [Google Scholar]
- Sperling RA, Aisen PS, Beckett LA, Bennett DA, Craft S, Fagan AM, Iwatsubo T, Jack CR, Jr, Kaye J, Montine TJ, et al. Toward defining the preclinical stages of Alzheimer’s disease: Recommendations from the National Institute on Aging-Alzheimer’s Association workgroups on diagnostic guidelines for Alzheimer’s disease. Alzheimers Dement. 2011a;7:280–292. [Europe PMC free article] [Abstract] [Google Scholar]
- Sperling RA, Dickerson BC, Pihlajamaki M, Vannini P, LaViolette PS, Vitolo OV, Hedden T, Becker JA, Rentz DM, Selkoe DJ, Johnson KA. Functional alterations in memory networks in early Alzheimer’s disease. Neuromolecular Med. 2010;12:27–43. [Europe PMC free article] [Abstract] [Google Scholar]
- Sperling RA, Jack CR, Jr, Aisen PS. Testing the right target and right drug at the right stage. Sci Transl Med. 2011b;3:111cm133. [Europe PMC free article] [Abstract] [Google Scholar]
- Sperling RA, Johnson KA, Doraiswamy PM, Reiman EM, Fleisher AS, Sabbagh MN, Sadowsky CH, Carpenter A, Davis MD, Lu M, et al. Amyloid deposition detected with florbetapir F 18 ((18)F-AV-45) is related to lower episodic memory performance in clinically normal older individuals. Neurobiol Aging. 2013;34:822–831. [Europe PMC free article] [Abstract] [Google Scholar]
- Sperling RA, Laviolette PS, O’Keefe K, O’Brien J, Rentz DM, Pihlajamaki M, Marshall G, Hyman BT, Selkoe DJ, Hedden T, et al. Amyloid deposition is associated with impaired default network function in older persons without dementia. Neuron. 2009;63:178–188. [Europe PMC free article] [Abstract] [Google Scholar]
- Sperling RA, Rentz DM, Johnson KA, Karlawish J, Donohue M, Salmon DP, Aisen P. The A4 Study: Stopping AD before Symptoms Begin? Sci Trans Med. 2014;6:228fs213. [Europe PMC free article] [Abstract] [Google Scholar]
- Stark SM, Yassa MA, Lacy JW, Stark CE. A task to assess behavioral pattern separation (BPS) in humans: Data from healthy aging and mild cognitive impairment. Neuropsychologia. 2013;51:2442–2449. [Europe PMC free article] [Abstract] [Google Scholar]
- Stern Y. Cognitive reserve. Neuropsychologia. 2009;47:2015–2028. [Europe PMC free article] [Abstract] [Google Scholar]
- Storandt M, Mintun MA, Head D, Morris JC. Cognitive decline and brain volume loss as signatures of cerebral amyloid-beta peptide deposition identified with Pittsburgh compound B: cognitive decline associated with Abeta deposition. Arch Neurol. 2009;66:1476–1481. [Europe PMC free article] [Abstract] [Google Scholar]
- Tapiola T, Alafuzoff I, Herukka SK, Parkkinen L, Hartikainen P, Soininen H, Pirttila T. Cerebrospinal fluid {beta}-amyloid 42 and tau proteins as biomarkers of Alzheimer-type pathologic changes in the brain. Arch Neurol. 2009;66:382–389. [Abstract] [Google Scholar]
- Thomas JB, Brier MR, Bateman RJ, Snyder AZ, Benzinger TL, Xiong C, Raichle M, Holtzman DM, Sperling RA, Mayeux R, et al. Functional connectivity in autosomal dominant and late-onset Alzheimer disease. JAMA neurology. 2014;71:1111–1122. [Europe PMC free article] [Abstract] [Google Scholar]
- Toledo JB, Weiner MW, Wolk DA, Da X, Chen K, Arnold SE, Jagust W, Jack C, Reiman EM, Davatzikos C, et al. Neuronal injury biomarkers and prognosis in ADNI subjects with normal cognition. Acta Neuropathol Commun. 2014;2:26. [Europe PMC free article] [Abstract] [Google Scholar]
- van Harten AC, Smits LL, Teunissen CE, Visser PJ, Koene T, Blankenstein MA, Scheltens P, van der Flier WM. Preclinical AD predicts decline in memory and executive functions in subjective complaints. Neurology. 2013;81:1409–1416. [Abstract] [Google Scholar]
- Vemuri P, Lesnick TG, Przybelski SA, Knopman DS, Roberts RO, Lowe VJ, Kantarci K, Senjem ML, Gunter JL, Boeve BF, et al. Effect of lifestyle activities on Alzheimer disease biomarkers and cognition. Ann Neurol. 2012;72:730–738. [Europe PMC free article] [Abstract] [Google Scholar]
- Villemagne VL, Burnham S, Bourgeat P, Brown B, Ellis KA, Salvado O, Szoeke C, Macaulay SL, Martins R, Maruff P, et al. Amyloid beta deposition, neurodegeneration, and cognitive decline in sporadic Alzheimer’s disease: a prospective cohort study. Lancet Neurol. 2013;12:357–367. [Abstract] [Google Scholar]
- Vos SJ, Xiong C, Visser PJ, Jasielec MS, Hassenstab J, Grant EA, Cairns NJ, Morris JC, Holtzman DM, Fagan AM. Preclinical Alzheimer’s disease and its outcome: a longitudinal cohort study. Lancet Neurol. 2013;12:957–965. [Europe PMC free article] [Abstract] [Google Scholar]
- Wang L, Brier MR, Snyder AZ, Thomas JB, Fagan AM, Xiong C, Benzinger TL, Holtzman DM, Morris JC, Ances BM. Cerebrospinal fluid Abeta42, phosphorylated Tau181, and resting-state functional connectivity. JAMA neurology. 2013;70:1242–1248. [Europe PMC free article] [Abstract] [Google Scholar]
- Whitwell JL, Josephs KA, Murray ME, Kantarci K, Przybelski SA, Weigand SD, Vemuri P, Senjem ML, Parisi JE, Knopman DS, et al. MRI correlates of neurofibrillary tangle pathology at autopsy: a voxel-based morphometry study. Neurology. 2008;71:743–749. [Europe PMC free article] [Abstract] [Google Scholar]
- Whitwell JL, Tosakulwong N, Weigand SD, Senjem ML, Lowe VJ, Gunter JL, Boeve BF, Knopman DS, Dickerson BC, Petersen RC, Jack CR., Jr Does amyloid deposition produce a specific atrophic signature in cognitively normal subjects? Neuroimage Clin. 2013;2:249–257. [Europe PMC free article] [Abstract] [Google Scholar]
- Wilson RS, Barnes LL, Aggarwal NT, Boyle PA, Hebert LE, Mendes de Leon CF, Evans DA. Cognitive activity and the cognitive morbidity of Alzheimer disease. Neurology. 75:990–996. [Europe PMC free article] [Abstract] [Google Scholar]
- Wilson RS, Boyle PA, Yu L, Barnes LL, Schneider JA, Bennett DA. Life-span cognitive activity, neuropathologic burden, and cognitive aging. Neurology. 2013a;81:314–321. [Europe PMC free article] [Abstract] [Google Scholar]
- Wilson RS, Yu L, Trojanowski JQ, Chen EY, Boyle PA, Bennett DA, Schneider JA. TDP-43 pathology, cognitive decline, and dementia in old age. JAMA neurology. 2013b;70:1418–1424. [Europe PMC free article] [Abstract] [Google Scholar]
- Wirth M, Madison CM, Rabinovici GD, Oh H, Landau SM, Jagust WJ. Alzheimer’s disease neurodegenerative biomarkers are associated with decreased cognitive function but not beta-amyloid in cognitively normal older individuals. J Neurosci. 2013a;33:5553–5563. [Europe PMC free article] [Abstract] [Google Scholar]
- Wirth M, Villeneuve S, Haase CM, Madison CM, Oh H, Landau SM, Rabinovici GD, Jagust WJ. Associations between Alzheimer disease biomarkers, neurodegeneration, and cognition in cognitively normal older people. JAMA neurology. 2013b;70:1512–1519. [Abstract] [Google Scholar]
- Xia CF, Arteaga J, Chen G, Gangadharmath U, Gomez LF, Kasi D, Lam C, Liang Q, Liu C, Mocharla VP, et al. [(18)F]T807, a novel tau positron emission tomography imaging agent for Alzheimer’s disease. Alzheimers Dement 2013 [Abstract] [Google Scholar]
- Zhang W, Arteaga J, Cashion DK, Chen G, Gangadharmath U, Gomez LF, Kasi D, Lam C, Liang Q, Liu C, et al. A highly selective and specific PET tracer for imaging of tau pathologies. J Alzheimers Dis. 2012;31:601–612. [Abstract] [Google Scholar]
Full text links
Read article at publisher's site: https://doi.org/10.1016/j.neuron.2014.10.038
Read article for free, from open access legal sources, via Unpaywall:
http://www.cell.com/article/S0896627314009581/pdf
Citations & impact
Impact metrics
Citations of article over time
Alternative metrics
Smart citations by scite.ai
Explore citation contexts and check if this article has been
supported or disputed.
https://scite.ai/reports/10.1016/j.neuron.2014.10.038
Article citations
Blockade of brain alkaline phosphatase efficiently reduces amyloid-β plaque burden and associated cognitive impairment.
Alzheimers Res Ther, 16(1):233, 22 Oct 2024
Cited by: 0 articles | PMID: 39438925 | PMCID: PMC11494749
Graded Intensity Aerobic Exercise to Improve Cerebrovascular Function and Performance in Older Veterans: Protocol for a Randomized Controlled Trial.
JMIR Res Protoc, 13:e58316, 26 Sep 2024
Cited by: 0 articles | PMID: 39326042 | PMCID: PMC11467598
Genome-wide association analysis and admixture mapping in a Puerto Rican cohort supports an Alzheimer disease risk locus on chromosome 12.
Front Aging Neurosci, 16:1459796, 04 Sep 2024
Cited by: 0 articles | PMID: 39295643 | PMCID: PMC11408238
Classifying Unconscious, Psychedelic, and Neuropsychiatric Brain States with Functional Connectivity, Graph Theory, and Cortical Gradient Analysis.
Brain Sci, 14(9):880, 30 Aug 2024
Cited by: 0 articles | PMID: 39335376 | PMCID: PMC11430472
Sex and Gender Differences in Alzheimer's Disease: Genetic, Hormonal, and Inflammation Impacts.
Int J Mol Sci, 25(15):8485, 03 Aug 2024
Cited by: 0 articles | PMID: 39126053 | PMCID: PMC11313277
Review Free full text in Europe PMC
Go to all (389) article citations
Similar Articles
To arrive at the top five similar articles we use a word-weighted algorithm to compare words from the Title and Abstract of each citation.
Subtle Cognitive Decline and Biomarker Staging in Preclinical Alzheimer's Disease.
J Alzheimers Dis, 47(1):231-242, 01 Jan 2015
Cited by: 95 articles | PMID: 26402771 | PMCID: PMC4634634
Preclinical Alzheimer's disease therapeutics.
J Alzheimers Dis, 42 Suppl 4:S545-9, 01 Jan 2014
Cited by: 5 articles | PMID: 25079804
Review
Development of biomarkers to chart all Alzheimer's disease stages: the royal road to cutting the therapeutic Gordian Knot.
Alzheimers Dement, 8(4):312-336, 01 Jul 2012
Cited by: 72 articles | PMID: 22748938
Review
Cognitive Impairment Precedes and Predicts Functional Impairment in Mild Alzheimer's Disease.
J Alzheimers Dis, 47(1):205-214, 01 Jan 2015
Cited by: 37 articles | PMID: 26402769 | PMCID: PMC4923754
Funding
Funders who supported this work.
NIA NIH HHS (7)
Grant ID: F32AG044054
Grant ID: K24 AG035007
Grant ID: F32 AG044054
Grant ID: R01 AG027435
Grant ID: P01AG036694
Grant ID: P01 AG036694
Grant ID: R01 AG046396