Abstract
Free full text

Methylome-wide analysis of chronic HIV infection reveals five-year increase in biological age and epigenetic targeting of HLA
SUMMARY
HIV-infected individuals are living longer on antiretroviral therapy, but many patients display signs that in some ways resemble premature aging. To investigate and quantify the impact of chronic HIV infection on aging, we report a global analysis of the whole blood DNA methylomes of 137 HIV+ individuals under sustained therapy along with 44 matched HIV− individuals. First, we develop and validate epigenetic models of aging that are independent of blood cell composition. Using these models, we find that both chronic and recent HIV infection lead to an average aging advancement of 4.9 years, increasing expected mortality risk by 19%. In addition, sustained infection results in global deregulation of the methylome across >80,000 CpGs and specific hypomethylation of the region encoding the human leukocyte antigen locus (HLA). We find that decreased HLA methylation is predictive of lower CD4/CD8 T cell ratio, linking molecular aging, epigenetic regulation and disease progression.
Graphical abstract
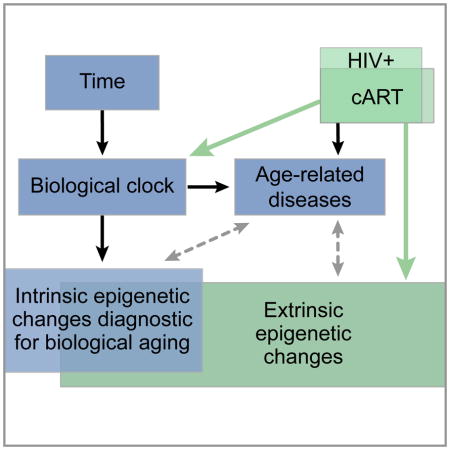
Gross et al. investigate the impact of chronic HIV infection by profiling the DNA methylomes of HIV+ individuals and matched HIV− controls. Using epigenetic models of aging they observe that HIV+ individuals show an age advancement of 4.9 years in whole blood and validate these results in pure cell samples.
INTRODUCTION
It is an open question why some people show early or delayed onset of aging-associated disorders (Kennedy et al., 2014). Recent studies have found that aging is associated with epigenetic changes (Christensen et al., 2009; Day et al., 2013; Heyn et al., 2012; Numata et al., 2012; West et al., 2013), and based on this work we (Hannum et al., 2012) and others (Horvath, 2013; Weidner et al., 2014) have built models capable of predicting a person’s age using DNA methylation patterns across a large number of CpG sites. Although these models are fairly accurate, errors of prediction — differences between the chronological and predicted age — serve as a quantitative readout of the relative advancement or retardation of the ‘biological age’ of an individual. Biological age advancement has been correlated with factors such as gender, genetic polymorphisms and diseases including cancer and diabetes, and it may influence the onset of other age-associated disorders (Day et al., 2013; Hannum et al., 2012). A recent longitudinal study validated the clinical utility of these models by demonstrating a link between biological age advancement and increased mortality rates (Marioni et al., 2015).
Biological aging has become of particular interest in treatment of HIV, in which the development of combination Anti-Retroviral Therapy (cART) now enables infected individuals to live many decades (Deeks, 2011; Deeks et al., 2013; Maartens et al., 2014). Several studies have suggested links between chronic HIV infection and early onset of neurodegeneration (Nightingale et al., 2014), liver or kidney failure (Joshi et al., 2011; Kovari et al., 2013), cancer (Dubrow et al., 2012), cardiovascular disease (Freiberg et al., 2013), and telomere shortening (Leeansyah et al., 2013; Pathai et al., 2013), leading to the hypothesis that HIV+ patients might experience advanced or accelerated aging (Appay and Rowland-Jones, 2002; Guaraldi et al., 2011; Smith et al., 2012). While these studies report rough estimates of HIV-mediated age advancement in the range of 0–20 years, it has been difficult to accurately quantify this number due to sampling effects, co-morbidities, and relatively low incidence rates of any single age-associated disease. To this effect, the existence, extent, and molecular basis of a bona-fide increase in aging have been unclear (Althoff et al., 2014; Solomon et al., 2014), in part due to lack of an objective biological clock or aging biomarker.
In parallel with such epidemiological observations, a number of studies report age effects using blood-based biomarkers. Analysis of cell surface markers in T cells has shown HIV+ subjects to show phenotypes of older cells (Cao et al., 2009). Other studies have observed shortened telomeres in certain cell populations (Rickabaugh et al., 2011) as well as whole blood (Zanet et al., 2014), indirectly linking HIV to aging via the well-studied connection between telomere length and age (Lindsey et al., 1991; Cawthon et al, 2003). Furthermore, a recent analysis of untreated HIV+ individuals found DNA methylation sites that are associated with both HIV infection and age (Rickabaugh et al., 2015). Together, these results raise the possibility that HIV infection results in an increase in biological age. Many questions remain, however: Are the epigenetic changes associated with HIV the same as those previously identified (Hannum et al., 2012; Horvath, 2013) in normal individuals as markers of ‘biological age’, and how complete is the correspondence between these two responses? What is the quantitative effect on aging in years, and is it fixed age advancement or continuous acceleration? What is the impact on aging of chronic HIV infection and sustained cART treatment? Are there other impacts of HIV on the methylome that are unrelated to aging?
Here we begin to address these questions by analyzing the methylomes of HIV-infected, cART-treated subjects, in which we observe a strong shared phenotype of HIV and age. To understand this signal, we develop models of biological age that allow us to establish a clear quantitative link between HIV infection and aging as observed in the general population. We identify both global and targeted epigenomic effects of HIV, including specific hypomethylation of the HLA locus. Together, these results shed light on the epigenetic consequences and/or gerontological aspects of chronic HIV infection.
RESULTS
Genome-wide DNA methylation profiling
To determine whether HIV is associated with signs of aberrant biological aging, samples of whole blood DNA were obtained from 137 HIV-infected, cART-treated but otherwise healthy non-Hispanic white males (no hepatitis C co-infection, no diabetes, and high adherence to therapy) and 44 healthy non-Hispanic white male controls (Table S1, Figure S1). Genome-wide methylation profiles of each sample were determined using the Illumina Infinium HumanMethylation450 BeadChip array. Data were normalized and controlled for quality using standard techniques, resulting in removal of two control patients due to poor signal (Experimental Procedures).
Unsupervised analysis shows shared phenotypes of HIV and age
As a preliminary exploration of this dataset, we ran an unsupervised analysis to identify age-associated methylation sites and their relation to HIV infection. Analysis of a previous methylome-wide screen of 538 healthy subjects (Hannum et al., 2012) identified as many as 61,592 methylation sites associated with age at a 1% false-discovery rate (FDR, likelihood ratio test in multivariate regression model with Benjamini-Hochberg correction). Validation of these sites in whole blood from a second control cohort from the European Prospective Investigation into Cancer and Nutrition (Riboli et al., 2002) (EPIC, N = 662) confirmed 26,927 of these sites as strongly associated with age (Figure 1A, Table S2).
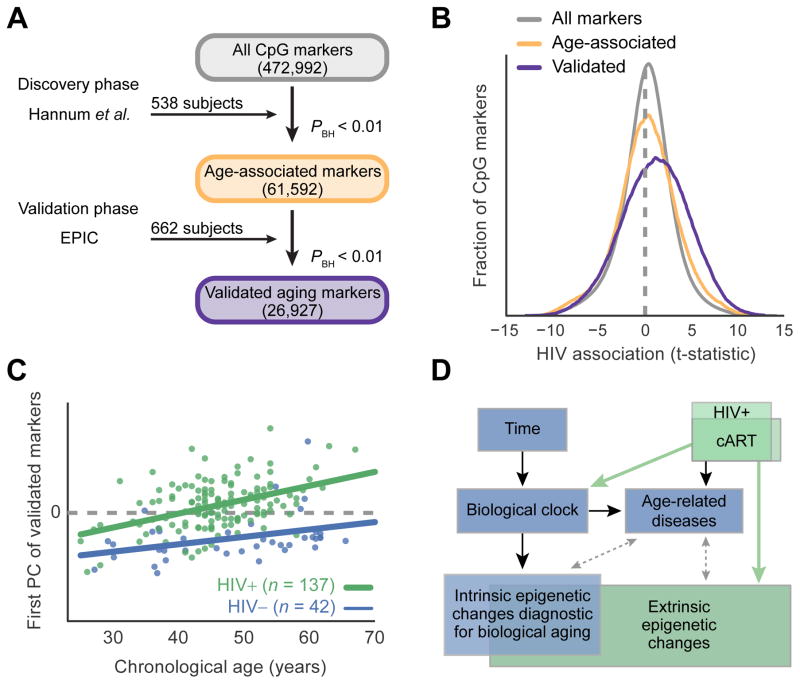
A, Discovery and validation of CpG methylation markers associated with age. B, Distribution of t-statistics measuring association of each methylation marker with HIV status. Colors indicate groups of markers identified in (A): Gray, all markers; yellow, age-associated markers from discovery phase; violet, subset of age-associated markers confirmed in validation. C, Principal component (PC) analysis of the validated age-associated markers, in which the first PC (y-axis) is positively associated with both age (x-axis) and disease status (HIV+, green; HIV−, blue). D, Potential relationships among HIV infection, epigenetics, disease, and aging. Black: known; Dashed gray: potential; Green: connections explored in this study. See also Table S2 and Table S3.
Among these validated age-associated sites, we found a striking association with methylation in the HIV+ patients relative to healthy controls (P < 10−100, Figure 1B). Further analysis of these sites found a positive association of the first principal component with both age and HIV status (Figure 1C, Table S3, association by multivariate linear model P < 10−8). These findings support a link between HIV infection and aging (Rickabaugh et al., 2015), as quantitatively measured by epigenomic profiling (Figure 1D).
Benchmarking and refinement of epigenetic aging models
Given the shared effects of HIV and aging, we sought to determine whether HIV causes the same biological aging signature as previously found in cohorts of uninfected individuals (Hannum et al., 2012; Horvath et al., 2013, Marioni et al., 2015). We tested aging models from both our group (Hannum et al., 2012) and Horvath (Horvath, 2013) in three independent datasets derived from whole blood samples (Hannum et al., 2012; Riboli et al., 2002; this study; Table S4). Although the Hannum and Horvath modeling efforts were based on different methodologies and training data, we found they made very similar predictions (r = 0.9, Pearson’s correlation, Figure 2A) and furthermore that a consensus of the two models outperformed either model individually (Figures 2B and 2C, Table S4). For this reason, we used this consensus model for all remaining analyses.
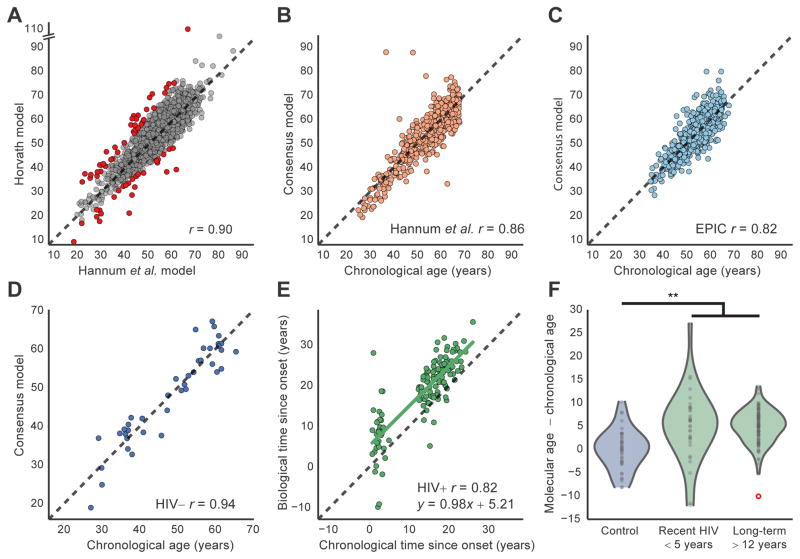
A, Scatter plot comparing the ages predicted using the Hannum et al. and Horvath models on healthy controls (n = 1,246 from HIV−, Hannum et al. and EPIC datasets). Red points indicate patients that were discarded due to disagreement between the two aging models (n = 66). B–C, Accuracy of the consensus model (y-axis) to predict true chronological age (x-axis) in datasets from Hannum et al. (n = 497, B) or EPIC (n = 637, C). Panels (A–C) show patients between 25 and 68 years old. D, Scatter plot of predicted biological age (consensus aging model) versus chronological age for HIV− healthy controls. E, Scatter plot of biological time versus chronological time since HIV onset for infected subjects. F, Violin plots showing the distribution of residuals from regression of biological versus chronological age. Three groups are shown: HIV− controls, short-term HIV+ infected individuals, and long-term HIV+ infected individuals. Note that the red circle indicates an outlier, which is not used to fit the violin profile, but is used in all statistical assessments. A–E, Black dashed lines indicate diagonal (y = x). r, Pearson’s correlation coefficient. ** indicates P < 10−5. See also Table S4.
A potential issue with these models arises in the fact that methylation profiles from whole blood are influenced by cell composition, and different cell types have different methylation states (Jaffe and Irizarry, 2014). These differences might be particularly pronounced in HIV-infected patients, some of whom have low CD4+ T cell counts (Trono et al., 2010). To understand the sensitivity of epigenetic aging models to cell type composition, we downloaded two datasets profiling sorted cells across shared sets of individuals (Absher et al., 2013, GSE59250; Reynolds et al., 2014, GSE56046). Among these sorted cell datasets, we saw good concordance of epigenetic age predictions with chronological age (Figure S2A–F). Epigenetic age was reproducible across different cell types profiled from the same patients, with high agreement of age estimates (r > 0.77–0.88) and moderate but very significant agreement of age advancement (Pearson’s r > 0.45–0.68; P < 0.0001 for all associations, Figure S2G–J).
While we therefore expect the contribution of cell composition to be minimal, we nonetheless developed an algorithm to individually normalize each methylation profile using methylation-derived cell type information. In brief, we used a previously reported method (Jaffe and Irizarry, 2014) to reliably predict blood composition (Figure S3) and adjust out the expected contribution of cell-type specific effects. This procedure greatly limited the effects of age and HIV induced blood composition changes in downstream analyses (Experimental Procedures, Figure S4).
HIV+ individuals have advanced DNA methylation age
We next used this consensus aging model to calculate the ‘biological age’ of each individual in our cohort (Table S4). For uninfected controls, the calculated biological age had a very high concordance with chronological age (Figure 2D, Pearson’s r = 0.94). In contrast, the HIV+ patients had a biological age advancement of 4.9 years on average (P < 10−8 by Student’s t-test, 95% confidence interval 3.4 – 7.1 years, Figures 2E and 2F). These results were consistent with our previous unsupervised analysis (Figures 1B and 1C) in suggesting that HIV infection leads to advanced aging. Furthermore, we found that the age advancement of HIV+ individuals was negatively correlated with the ratio of CD4+/CD8+ T lymphocytes (Spearman’s rho = −0.2, P < 0.02). CD4+ T cells are a major indicator of immune integrity (Leung et al., 2013; Serrano-Villar et al., 2014) and are inversely associated with morbidity and mortality, including from non-AIDS defining diseases (El-Sadr et al., 2006); similarly, the CD4/CD8 ratio predicts non-AIDS morbidity (Leung et al., 2013; Serrano-Villar et al., 2014). This finding links biological aging of HIV-infected individuals to a clinical measure of disease progression, and it raises the possibility that patients with stable immune responses may be less affected by the advanced aging phenotype. Taking into account a recently estimated 4.2% increase in mortality risk per year of biological age advancement using the Hannum model (Marioni et al., 2015), the changes observed in HIV+ patients result in an expected total mortality risk increase of 19%.
Age advancement is independent of HIV duration
Notably, patients more recently infected with HIV (< 5 years) had no significant difference in age advancement from those patients with chronic (>12 years) infection (P > 0.5, Mann-Whitney U Test; Figure 2F). Similar findings emerged from a regression analysis of the chronological versus biological time since infection: the slope did not differ from one (0.98 ± 0.06, standard error) whereas the y-intersect was significantly positive (5.2 ± 0.9; Figures 2E and 2F). These findings lend support to the theory that age advancement occurs early in the course of disease as a consequence of acute infection or reaction to drug treatment (Guaraldi et al., 2011; Smith et al., 2012). The lack of an increase of age advancement with disease duration seems to contradict alternative views that HIV-mediated aging occurs through cumulative effects of latent virus (Appay and Rowland-Jones, 2002) or chronic therapeutic intervention (Torres and Lewis, 2014). We did however observe less variation in age advancement within the chronically infected HIV+ individuals (Figure 2F, P < 0.002, Bartlett’s test relative to recently infected group), perhaps reflecting the comparative stability of infection and immune response on long-term cART therapy (Luz et al., 2014; Rosenblatt et al., 2005).
Age advancement is independent of cellular composition
While the direct effects of cell type composition on the whole blood methylome were corrected by the adjustment described above (also see Experimental Procedures), we considered that it was still possible that changes in cell-type composition could lead to downstream, indirect changes in the epigenomes of all blood cells. If this were the case, cell-type associated changes could be responsible for the observed increase in biological age in the HIV+ cases. To assess this possibility, we constructed a multivariate linear model in which cell-type composition variables and HIV status were used to predict biological age as measured by the methylome (Table 1). In this model, the presence of HIV was associated with an age advancement of 3.8±1.1 years, while the presence of natural killer cells accounted for additional increases in biological age. In an even more conservative test, we modeled age advancement with cell-type composition variables alone and found that the unexplained variation in this model still had a significant association with HIV infection (P = 0.02, Likelihood Ratio Test, Table 1). Thus, even in a very conservative analysis, HIV infection still has association with advanced aging that is independent of cell composition.
Table 1
Multivariate linear models of biological age based on chronological age, HIV and cellular composition.
Model 1. Dependent variable: Biological age | |||||
---|---|---|---|---|---|
Independent variable | Effect | StdErr | t | P | |
HIV | 3.76 | 1.14 | 3.3 | 0.003 | |
Chronological age | −0.12 | 0.04 | −3.0 | 0.001 | |
Cell composition (%) | NK cell | 0.21 | 0.08 | 2.6 | 0.011 |
CD4 T cell | −0.07 | 0.07 | −1.1 | 0.293 | |
CD8 T cell | 0.13 | 0.06 | 0.2 | 0.812 | |
B cell | −0.17 | 0.13 | −1.4 | 0.174 | |
Monocyte | −0.09 | 0.14 | −0.6 | 0.521 | |
Model 2. Dependent variable: Biological age | |||||
Independent variable | Effect | StdErr | t | P | |
Chronological age | −0.22 | 0.07 | −3.1 | 0.002 | |
Cell composition (%) | NK cell | 0.15 | 0.07 | 2.1 | 0.041 |
CD4 T cell | −0.23 | 0.08 | −2.9 | 0.004 | |
CD8 T cell | 0.15 | 0.08 | 1.8 | 0.076 | |
B cell | −0.10 | 0.07 | −1.3 | 0.190 | |
Monocyte | −0.05 | 0.07 | −0.7 | 0.492 | |
Model 3. Dependent variable: Model 2 residuals | |||||
Independent variable | Effect | StdErr | t | P | |
HIV | 0.18 | 0.08 | 2.4 | 0.019 |
We also sought to experimentally assess if the observed age advancement due to HIV infection was also observed in purified cell populations. Using standard calculations of statistical power, we estimated that a sample of 48 patients, balanced approximately between cases and controls, would have 81% power to detect the same aging advancement effect as our primary screen at p < 0.01. Accordingly, this number of subjects was prospectively recruited from the University of Nebraska Medical Center (Experimental Procedures, Table S5). Whole blood was separated immunomagnetically to isolate pure populations of neutrophils and CD4+ T cells.
As in whole blood, unsupervised analysis showed a clear effect of HIV in age-associated methylation markers (Figure 3A–B). Application of epigenetic models of aging in these pure-cell datasets showed good concordance of predicted age with chronological age in both cell types (Figure 3C–F). In neutrophils, the Hannum model predicted a 2.5 year increase in age due to HIV infection (P < 0.03, 95% CI 0.6–5.0 years, Figure 3E) whereas the Horvath model showed a smaller effect of 0.4 year (P > 0.05). In contrast, CD4+ T-cells had a much stronger and more consistent HIV response in both models, with the consensus aging model showing an increase of 5.7 years in the HIV+ subjects (P < 10−5, 95% CI 3.4–7.9 years, Figure 3F). These data indicate that the effect of epigenetic age advancement is not merely an artifact of changing blood composition, but likely reflects true aging signals. The stronger effect size within CD4+ T-cells (Figure 3G–H) suggests that these cells may be exposed to more age-like stress than neutrophils, although further work is needed to understand how disease may affect aging rates across different cell types and tissues.
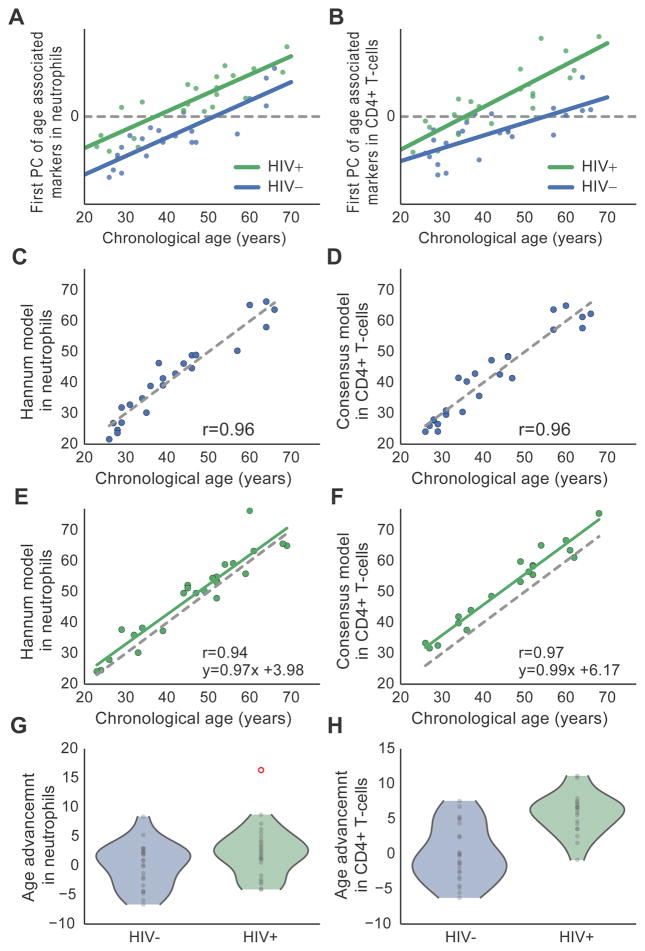
A–B, Unsupervised principal component (PC) analysis of methylation patterns in purified blood cell types, in which the first PC is positively associated with both age (x-axis) and disease status (HIV+, green; HIV- blue). (A) New CD4+ T-cell cohort across 5999 markers that are age-associated in CD4+ T-cells (GSE59250). (B) New neutrophil cohort across markers probes that are age-associated in neutrophils (GSE65097). C–F, Control (C–D) and HIV+ (E–F) subjects for sorted cell validation datasets comparing chronological age to the Hannum et al. epigenetic aging model in neutrophils (C, E) and consensus aging model in CD4+ T-cells (D, F). G–H, Violin plots showing age advancement in the two sorted cell datasets. For B, in initial analysis the first PC heavily reflected an outlier point, which was removed for this analysis after which the PC was recalculated. See also Figure S2.
HIV and aging have shared and distinct methylation patterns
Having identified a large effect of HIV infection on age associated methylation signals, we then sought to better understand the wider changes instigated by HIV. We identified 81,361 CpG markers associated with HIV infection (Benjamini-Hochberg corrected P < 0.01; likelihood ratio test using a multivariate linear model, Table S2). Of these, 2569 upregulated markers and 1769 downregulated markers were also associated with aging, a 3.2 and 1.4-fold enrichment over random expectation, respectively (Figure 4A, Fisher’s Exact Test P < 10−30, Table S6). We found that markers associated with both HIV and aging were enriched in DNAse hypersensitivity sites and transcriptional start sites, suggesting methylation changes in DNA regions under active regulation. These CpG markers were also enriched in binding sites for polycomb repressive complex (PRC2) (Figure 4B), a switch that tightly regulates genes required for differentiation and renewal, and in Drosophilia is linked to longevity (Siebold et al., 2010). These findings reinforce previous reports that PRC2 targets are irreversibly repressed by methylation during the aging process (Beerman et al., 2013; Deaton and Bird, 2011; Teschendorff et al., 2010). Interestingly, markers associated with HIV but not aging had a very different functional enrichment profile (Figure 4B), indicating additional mechanism(s) for epigenetic alteration associated with HIV.
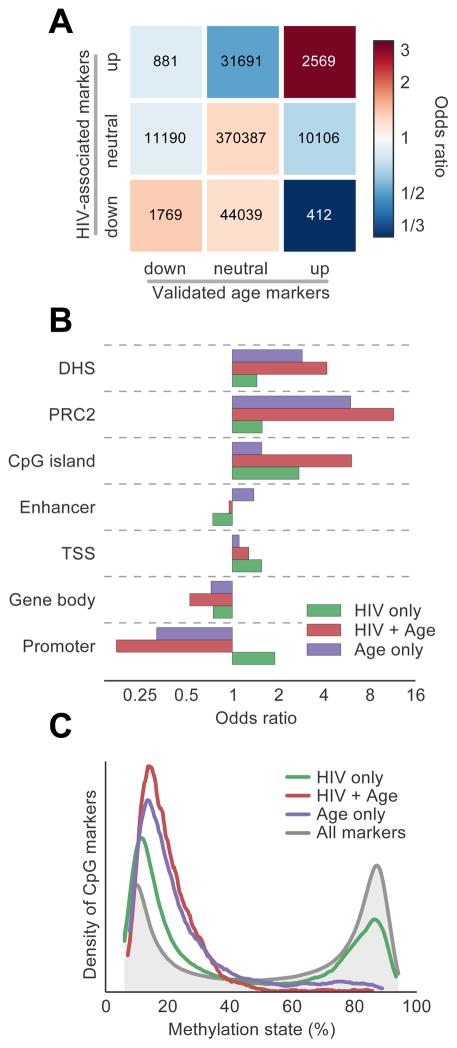
A, Overlap table comparing the set of CpG markers associated with HIV and the set of validated age-associated markers (see Figure 1A). Numbers indicate probe counts in each overlap, colors correspond to odds ratio of overlap compared to background. B, Odds ratios of enrichment for a panel of genomic features, evaluated in sets of markers associated with age, HIV, or both. PRC2, polycomb repressive complex 2 binding sites; DHS, DNase hypersensitivity sites; TSS, transcription start sites. C, Distribution of methylation states for the CpG marker sets defined in (A). See also Table S2 and Table S6.
We have previously reported that age-associated markers in older subjects tend away from a fully methylated or unmethylated state and instead move towards disorder (with a methylation fraction of 50% representing complete disorder) (Hannum et al., 2012). We found that HIV-infected patients displayed a similar trait: among markers associated with HIV, 66% tended towards disorder, compared with 70% of age-associated markers (Experimental Procedures, Figure S5). Furthermore, whereas age-associated markers tended to have a low methylation fraction that increased with age, HIV-associated markers were more equally balanced between low and high methylation states (Figure 4C).
HIV is associated with hypomethylation of the HLA locus, independent of aging
Thus far, we had observed multiple effects of HIV on the methylome, including changes in cellular composition, age advancement, and a general increase in methylome disorder. We next sought to determine whether there are specific genomic regions for which the methylation state is particularly associated with HIV infection, independent of aging or other factors (Experimental Procedures). Towards this aim, we conducted an analysis of HIV-associated CpG markers independent of disorder or age, controlling for the effects of cellular composition. Analysis of the whole blood data identified a single genomic region that was enriched in CpG markers associated with HIV; this region, consisting of 10 megabases on chromosome 6 including histone gene cluster 1 and the entire HLA locus, had particularly reduced methylation levels in HIV+ cases as compared to HIV− controls (P < 10−10, Figure 5A, Experimental Procedures). HLA genes encode the Major Histocompatibility Complexes (MHC), the key antigen-presenting molecules that govern the acquired immune response and impact innate immunity (Figure 5B) (Goulder and Walker, 2012). We found that the differentially methylated markers surround the rs2395029 variant, for which common genetic variation has been repeatedly implicated in HIV host control (Figures 5C, D) (Fellay et al., 2007; International HIV Controllers Study et al., 2010). Examination of this locus in the validation samples of purified neutrophils and CD4+ T cells identified the HCP5 gene body as particularly differentially methylated in neutrophils (Figures 5E, F, Figure S6). As further evidence that the observed changes are functional, we found that the amount of methylation at this gene was correlated with a patient’s CD4+/CD8+ T cell ratio (Figure S6). Taken together, these results indicate that the HLA locus is likely differentially methylated across blood cell types and also changes within individual cell types in response to HIV. An intriguing interpretation of our results is that some of the previously reported changes in HLA expression and corresponding HIV control (Apps et al., 2013) are attributable to methylation dynamics.
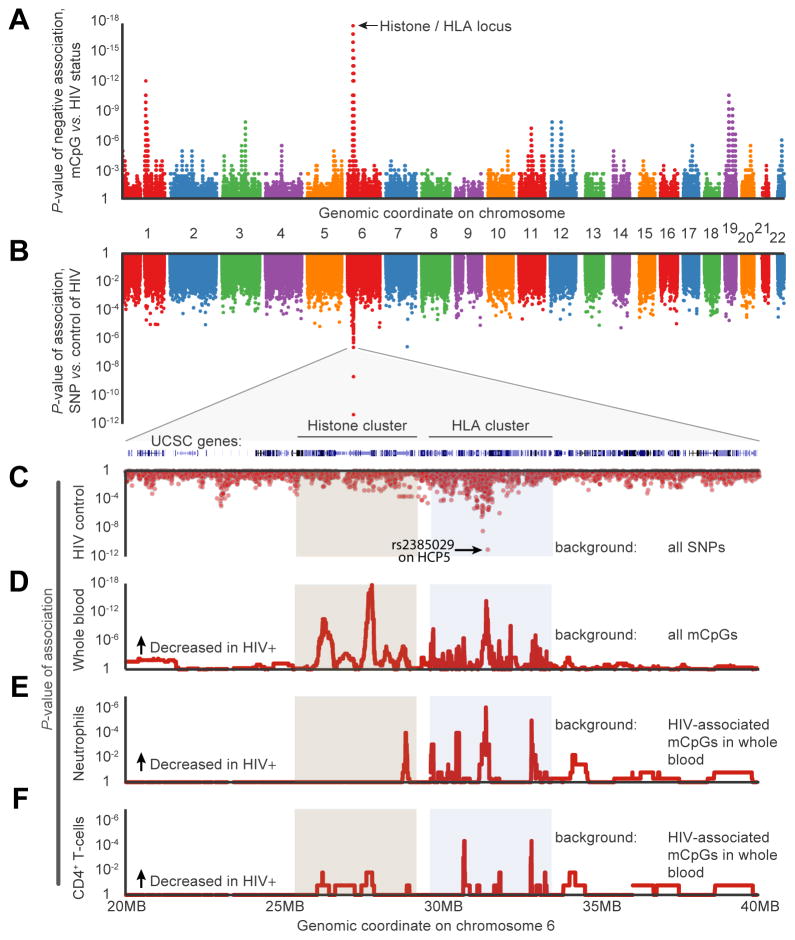
A, Epigenome-wide association of CpG methylation (mCpG) with HIV status (presence or absence). Each point represents the P-value of enrichment for differentially methylated CpG markers within a bin of ±100 consecutive markers along the genome. B–C, P-values of genome-wide association of single nucleotide polymorphisms (SNP) with host control of HIV, reproduced from Fellay et al. D, Epigenome-wide association of mCpG with HIV status (presence or absence), zoomed in to target histone/HLA locus. E–F, Validation screen of HIV-downregulated markers in purified populations of neutrophils (E) and CD4+ T-cells (F). See also Table S2 and Figure S6.
DISCUSSION
We have shown that methylome-wide changes previously ascribed to aging are also induced by HIV (Figures 1 and and3).3). By using highly accurate, externally trained and validated models of biological aging, our study provides a robust estimate of a five-year age advancement in HIV/cART individuals (Figure 2). These results, in combination with the link between molecular age advancement and increased mortality risk (Marioni et al., 2015), support the idea that chronic HIV infection is accompanied by a tangible gerontological phenotype. In addition to an aggregate estimate of HIV age advancement, the methylation aging model allows for patient-by-patient estimates. Patients deemed more likely to suffer from HIV-mediated aging effects might be placed on alterative schedules for preventative care, including early screening and further testing if warranted.
While epidemiological studies have attempted to measure age acceleration and increased mortality rates in HIV+ individuals (Appay and Rowland-Jones, 2002; Guaraldi et al., 2011; Smith et al., 2012), such measurements are made difficult by the myriad co-factors associated with HIV infection. For instance, metabolic disorders such as diabetes, HCV infection, and medication adherence are important factors of HIV infection that are also suspected to significantly affect mortality rates. Most previous studies have not attempted to control for these factors; in contrast, our study has focused specifically on well-characterized subjects. Nonetheless, our estimate of HIV age advancement of 4.9 years, calculated from a quantitative analysis of the methylome, falls within the range of the previous epidemiological studies. Further work will be needed to understand if the observed epigenetic age advancement is generalizable to broader slices of the HIV+ population, i.e. patients with complex co-morbidities such as drug use or additional viral infections.
This study is based on the same epigenetic model of biological aging as many others, including recent reports associating epigenetic aging with Down’s Syndrome (Horvath et al., 2015), traumatic stress (Boks et al., 2015), and even all-cause mortality (Marioni et al., 2015). Here, we implement key data-processing and analysis steps to improve the application of these models, which should aid in future applications. By minimizing the effects of cell type composition, we find better calibration of our control samples (Figure 2B–D and Table S2), and the model is less affected by confounding associations such as the changing blood composition that occurs in HIV+ individuals (Figure S4). Furthermore, integration of both the Hannum et al. and Horvath models of epigenetic aging serves to limit biases in model training and allows us to filter samples that are of low quality or ill-suited for use in aging studies (Experimental Procedures, Table S4).
Our finding of a 5-year age advancement in cART-treated subjects (Figure 2E) is similar to one recent report (Horvath and Levine, 2015) but contrasts with another study in untreated patients, in which shared effects of age and HIV on the methylome were used to report an age advancement of 14 years (Rickabaugh et al., 2015). Although this discrepancy could be due to a beneficial effect of cART, we believe it is more likely due to differing statistical approaches. The previous number is based on comparison of the effects of HIV and age in a single cohort, rather than an epigenetic model of aging built for normal individuals, as performed here. Moreover, the authors derive their estimate from the ratio of linear coefficients for HIV and age, which are themselves highly correlated; such co-linearity is a well-known cause of instability in such estimates (Farrar and Glauber, 1967).
The discovery of HLA hypomethylation as a targeted consequence of HIV infection (Figure 5) has compelling synergy with the earlier discoveries of HLA genotype and expression level as major determinants of HIV control. Common genetic variation in HLA has been identified as the major contributing factor to host control of HIV infection (Fellay et al., 2007; International HIV Controllers Study et al., 2010), and HLA has been reported as a hotspot for integration of HIV provirus (Ambrosi et al., 2011). HIV infection has also been associated with decreased expression of some HLA genes but not others (Bonaparte and Barker, 2004; Cohen et al., 1999), and higher HLA-C expression is associated with HIV control (Apps et al., 2013; Kulkarni et al., 2011; Thomas et al., 2009). Our result suggests an epigenetic component to the regulation of HLA expression in this region. It also raises the possibility that the ability to control HIV infection could be acquired through epigenetic modification, as well as inherited through genotype.
In summary, we have shown that an extrinsic perturbation to a human population, driven by HIV infection and cART, is capable of inducing changes in the epigenomic state of affected individuals. This perturbation may influence regulation of HLA gene expression and also encompasses signatures of aging. Our findings help address a long-standing debate regarding the effects of HIV infection on biological aging in cART-treated individuals, in a manner that can be assessed numerically using an epigenome-based readout. Taken together, our findings show that the epigenome adds a quantitative means of assessing the interaction of HIV with normal and pathogenic processes associated with aging, and they shed light on the underlying mechanisms by which acute and chronic viral infection impact the host.
EXPERIMENTAL PROCEDURES
Reproduction of computation procedures
All data retrieval and processing steps are documented in a series of Jupyter notebooks at www.github.com/theandygross/HIV_Methylation.
Selection criteria and subject recruitment
HIV+ subject samples were obtained from CHARTER as a Resource (www.charterresource.ucsd.edu). The CHARTER study was comprised of HIV-infected participants at varying stages of disease and with differing histories of antiretroviral treatment, with a focus on neuromedical and neurobehavioral assessments (Heaton et al., 2010). We requested information on subjects for which DNA had been obtained. Demographic and clinical data were filtered for non-Hispanic white males (to match the control group) who were free of Hepatitis C virus, not diabetic, on cART, and adherent to therapy. Two groups were selected for study, those more recently infected by HIV (but after the acute infection stage, 0.8 – 5.0 years of infection) and those chronically infected (>12.0 years). As a control, 44 non-Hispanic white males without HIV were recruited from the San Diego area.
For validation samples 35 HIV+ subjects along with 25 healthy controls were recruited prospectively for the purpose of this study to match the characteristics of the primary cohort. Cells were purified using immunomagnetic separation and DNA was extracted from purified cell populations. While most subjects used had both neutrophils and CD4+ T-cells profiled, differing DNA yield for some subjects prohibited profiling of both cell types for some patients.
Clinical and demographic data are presented in Table S1 and Table S5.
Sample collection and methylation analysis
DNA was purified from whole blood samples using PaxGene collection tubes (Qiagene) and FlexiGene DNA extraction kits (Qiagen). Methylation analysis was performed using Infinium HumanMethylation450 BeadChip Kits (Illumina). 500ng of DNA was bisulfite converted using EZ DNA Methylation Kits (Zymo Research) and subsequently processed for HumanMethylation450 BeadChips following manufacturer’s instructions. Following hybridization, BeadChips were scanned using the Illumina HiScan System.
Data pre-processing
All methylation data for HIV+ and HIV− subjects were deposited in the Gene Expression Omnibus (GEO) under accession number GSE67705. For the Hannum et al. (Hannum et al., 2012) and EPIC (Riboli et al., 2002) studies, raw data were obtained from GEO accessions GSE40279 and GSE51032. All data were processed through the Minfi R processing pipeline (Aryee et al., 2014). Cell counts were estimated by the estimate Cell Counts function in Minfi using flow sorted cell populations made available by Houseman et al. (Houseman et al., 2012). To limit variability in methylation levels due to differing cell type composition in the whole blood samples, methylation levels were adjusted for each CpG marker as follows:
Average methylation levels for each cell type were obtained from the Houseman et al. (Houseman et al., 2012) flow sorted blood dataset.
A theoretical methylation level was assessed for each patient by assuming their blood to be a mixture of these pure cell populations at the estimated cell type proportions.
The difference of each patient’s methylation level from the average was assessed.
This difference was subtracted from the original raw dataset.
We followed the protocol established to be optimal by Marabita et al. (Marabita et al., 2013) first quantile normalizing the data and then performing beta-mixture quantile (BMIQ) normalization (Teschendorff et al., 2013). To limit batch effects, all arrays across the three studies were normalized together. For use in the Horvath methylation age model, raw data were normalized to a gold standard reference distribution following the protocol provided in the manuscript (Horvath, 2013). The sole deviation from the Horvath protocol was an additional cell composition adjustment performed in a similar manner as described above, after BMIQ normalization. While the cell composition adjustment was not part of either the Horvath or Hannum et al. processing pipeline, recent work (Jaffe and Irizarry, 2014) has shown cell type composition to be a key confounding factor in methylation analysis.
Benchmarking the aging models
Aging models were assessed using the Hannum et al. and EPIC (Riboli et al., 2002) datasets. While the Hannum et al. dataset was used to train both epigenetic aging models, the EPIC data were made available after the time of construction of both models and thus provide an independent assessment of performance. We limited analysis to patients between the ages of 25 and 68 years of age for better comparison to the HIV cohort. Among the HIV and EPIC cohorts, we saw slightly better performance of the Hannum model (which was trained using only whole blood data) than the Horvath model (trained in a variety of tissues), but when a simple average of these two models was taken (the ‘consensus model’), we found better performance than either separately (Table S2).
Epigenetic model concordance filter
One key drawback of current models of molecular age is the lack of a confidence measure in model prediction for any particular individual. To address, this we utilized the concordance between the two models as an additional filter of data quality. Despite general agreement between the models, in a number of subjects biological age predictions varied by more than 20%. This analysis resulted in the filtering of three HIV+ cases and two HIV− controls in our primary cohort (Table S1).
Linear scaling of epigenetic age
For both models and across all datasets a linear scaling factor existed when comparing chronological verses biological age. In order to properly compare the performance of the models and to best calibrate them to our dataset, we performed a linear adjustment to all model fits for the control data to a unit slope with a zero intercept. Note that this affected the model error when compared in an absolute sense, but did not affect the correlation between biological and chronological age. In HIV+ patients, we adjusted this particular cohort to the regression fit of the matched controls.
Screening for differentially methylated markers in response to HIV infection
For the results described in Figure 4 and and5,5, we ran a multivariate linear model to test for differentially methylated markers in response to HIV infection. This model used predicted cell type composition and age as covariates. Significance was assessed via a likelihood-ratio test for the improvement of a model fit with HIV as the variable of interest.
Disorder of Methylation in Response to HIV and Aging
We observed increasing disorder of the methylome by both aging and HIV infection. To assess the possibility that the increasing age advancement might be explained by increasing disorder, we conducted a principal component analysis on 7967 age-associated markers that trended away from < 50% of sites methylated with age(Figure S5A). This analysis produced a similar result to that shown in Figure 1C, in which the first principal component of the cohort was still associated with both with age and HIV infection. Furthermore, we observed that only 231 of 436 (53%) markers used in the two aging models tended towards methylation values of 50% of sites methylated (i.e. disorder, binomial P = 0.2) and found that while there is a general entropy increase in HIV+ patients across the entire methylome (Figure S5B), there is no such effect in the markers used in the biological age models (Figure S5C).
Identification of differentially methylated regions
We identified 25,491 markers that were associated with HIV, trended away from disorder, and were not associated with age. For the discovery and visualization of differentially methylated regions (Figure 5A), we calculated a rolling statistic on the density of ‘hits’ in 200 marker windows. From this analysis it was clear that a genomic region encompassing the HLA and histone gene clusters was enriched for markers in our query set, and post-hoc analysis confirmed a strong enrichment in the genomic interval traditionally assigned to the HLA region (~29MB – 33MB on chromosome 6, odds ratio = 1.3, P < 10−10). For further refinement and visualization of this signal we conducted a similar scan statistic on a section of chromosome 6 in Figure 5D. In this targeted analysis we relaxed our criteria and looked for regions of consistent increases or decreases in methylation in HIV+ verses HIV− subjects. This analysis showed a number of ‘peaks’ of hypomethylation both in the histone gene region as well as near the HLA genes.
Accounting for the probe density of the HLA region
One potential confounding factor of this analysis is the high density of markers in the HLA region due to the design of the Illumina chip. Taking the non-uninform density of the chip into account, the scan statistic searched for regions across a fixed number of markers as opposed to a fixed-width genomic interval. Despite this, it is possible that the tight clustering of markers in this region gave us more power to detect short differentially methylated regions within this genetic locus. The presence of two peaks in the histone cluster region directly upstream the HLA locus gives strong support to this being a specific effect. The density of probes in the histone region was typical compared to the rest of the genome, and the coincidence of these two signals being close to each other solely by chance is minimal.
Supplementary Material
1
2
3
4
Acknowledgments
This work was supported by the National Institute of Mental Health (P30 MH062261), the National Cancer Institute (P30 CA023100) and the California Institute for Regenerative Medicine. The CHARTER study is supported by the National Institutes of Health (HHSN271201000030C). We acknowledge the work of the UNMC Flow Cytometry Research Facility in data acquisition for this publication. We wish to thank Roman Sasik and Aaron Chang for advice on methylome analysis, and Cherie Ng for constructive discussions and comments on the manuscript.
Footnotes
AUTHOR CONTRIBUTIONS
HF and TI conceived of the study. HF, BMM and KZ organized the HIV+ and control patient cohorts. DC, KF, KL, KLJ, and MK performed the sample preparation and methylation profiling experiments. AMG, PAJ, HS, and TI conducted study and analysis design. AMG performed all statistical and bioinformatics analyses. AMG, PAJ, JFK, HF and TI wrote the manuscript.Publisher's Disclaimer: This is a PDF file of an unedited manuscript that has been accepted for publication. As a service to our customers we are providing this early version of the manuscript. The manuscript will undergo copyediting, typesetting, and review of the resulting proof before it is published in its final citable form. Please note that during the production process errors may be discovered which could affect the content, and all legal disclaimers that apply to the journal pertain.
References
- Althoff KN, McGinnis KA, Wyatt CM, Freiberg MS, Gilbert C, Oursler KK, Rimland D, Rodriguez-Barradas MC, Dubrow R, Park LS, et al. Comparison of Risk and Age at Diagnosis of Myocardial Infarction, End-Stage Renal Disease, and Non-AIDS-Defining Cancer in HIV-Infected Versus Uninfected Adults. Clin Infect Dis 2014 [Europe PMC free article] [Abstract] [Google Scholar]
- Ambrosi A, Glad IK, Pellin D, Cattoglio C, Mavilio F, Di Serio C, Frigessi A. Estimated comparative integration hotspots identify different behaviors of retroviral gene transfer vectors. PLoS Comput Biol. 2011;7:e1002292. [Europe PMC free article] [Abstract] [Google Scholar]
- Appay V, Rowland-Jones SL. Premature ageing of the immune system: the cause of AIDS? Trends Immunol. 2002;23:580–585. [Abstract] [Google Scholar]
- Apps R, Qi Y, Carlson JM, Chen H, Gao X, Thomas R, Yuki Y, Del Prete GQ, Goulder P, Brumme ZL, et al. Influence of HLA-C expression level on HIV control. Science. 2013;340:87–91. [Europe PMC free article] [Abstract] [Google Scholar]
- Aryee MJ, Jaffe AE, Corrada-Bravo H, Ladd-Acosta C, Feinberg AP, Hansen KD, Irizarry RA. Minfi: a flexible and comprehensive Bioconductor package for the analysis of Infinium DNA methylation microarrays. Bioinformatics. 2014;30:1363–1369. [Europe PMC free article] [Abstract] [Google Scholar]
- Beerman I, Bock C, Garrison BS, Smith ZD, Gu H, Meissner A, Rossi DJ. Proliferation-Dependent Alterations of the DNA Methylation Landscape Underlie Hematopoietic Stem Cell Aging. Stem Cell. 2013;12:413–425. [Abstract] [Google Scholar]
- Boks MP, van Mierlo HC, Rutten BPF, Radstake TRDJ, De Witte L, Geuze E, Horvath S, Schalkwyk LC, Vinkers CH, Broen JCA, et al. Longitudinal changes of telomere length and epigenetic age related to traumatic stress and post-traumatic stress disorder. Psychoneuroendocrinology. 2015;51:506–512. [Abstract] [Google Scholar]
- Bonaparte MI, Barker E. Killing of human immunodeficiency virus-infected primary T-cell blasts by autologous natural killer cells is dependent on the ability of the virus to alter the expression of major histocompatibility complex class I molecules. Blood. 2004;104:2087–2094. [Abstract] [Google Scholar]
- Cao W, Jamieson BD, Hultin LE, Hultin PM, Effros RB, Detels R. Premature Aging of T cells Is Associated With Faster HIV-1 Disease Progression. JAIDS Journal of Acquired Immune Deficiency Syndromes. 2009;50:137–147. [Europe PMC free article] [Abstract] [Google Scholar]
- Cawthon RM, Smith KR, O’Brien E, Sivatchenko A, Kerber RA. Association between telomere length in blood and mortality in people aged 60 years or older. The Lancet. 2003;361:393–395. [Abstract] [Google Scholar]
- Christensen BC, Houseman EA, Marsit CJ, Zheng S, Wrensch MR, Wiemels JL, Nelson HH, Karagas MR, Padbury JF, Bueno R, et al. Aging and environmental exposures alter tissue-specific DNA methylation dependent upon CpG island context. PLoS Genet. 2009;5:e1000602. [Europe PMC free article] [Abstract] [Google Scholar]
- Cohen GB, Gandhi RT, Davis DM, Mandelboim O, Chen BK, Strominger JL, Baltimore D. The selective downregulation of class I major histocompatibility complex proteins by HIV-1 protects HIV-infected cells from NK cells. Immunity. 1999;10:661–671. [Abstract] [Google Scholar]
- Day K, Waite LL, Thalacker-Mercer A, West A, Bamman MM, Brooks JD, Myers RM, Absher D. Differential DNA methylation with age displays both common and dynamic features across human tissues that are influenced by CpG landscape. Genome Biol. 2013;14:R102. [Europe PMC free article] [Abstract] [Google Scholar]
- Deaton AM, Bird A. CpG islands and the regulation of transcription. Genes Dev. 2011;25:1010–1022. [Europe PMC free article] [Abstract] [Google Scholar]
- Deeks SG. HIV infection, inflammation, immunosenescence, and aging. Annu Rev Med. 2011;62:141–155. [Europe PMC free article] [Abstract] [Google Scholar]
- Deeks SG, Tracy R, Douek DC. Systemic effects of inflammation on health during chronic HIV infection. Immunity. 2013;39:633–645. [Europe PMC free article] [Abstract] [Google Scholar]
- Dubrow R, Silverberg MJ, Park LS, Crothers K, Justice AC. HIV infection, aging, and immune function: implications for cancer risk and prevention. Curr Opin Oncol. 2012;24:506–516. [Europe PMC free article] [Abstract] [Google Scholar]
- Farrar DE, Glauber RR. Multicollinearity in Regression Analysis: The Problem Revisited. The Review of Economics and Statistics. 1967;49:92–107. [Google Scholar]
- Fellay J, Shianna KV, Ge D, Colombo S, Ledergerber B, Weale M, Zhang K, Gumbs C, Castagna A, Cossarizza A, et al. A whole-genome association study of major determinants for host control of HIV-1. Science. 2007;317:944–947. [Europe PMC free article] [Abstract] [Google Scholar]
- Freiberg MS, Chang CCH, Kuller LH, Skanderson M, Lowy E, Kraemer KL, Butt AA, Bidwell Goetz M, Leaf D, Oursler KA, et al. HIV infection and the risk of acute myocardial infarction. JAMA Intern Med. 2013;173:614–622. [Europe PMC free article] [Abstract] [Google Scholar]
- Goulder PJR, Walker BD. HIV and HLA class I: an evolving relationship. Immunity. 2012;37:426–440. [Europe PMC free article] [Abstract] [Google Scholar]
- Guaraldi G, Orlando G, Zona S, Menozzi M, Carli F, Garlassi E, Berti A, Rossi E, Roverato A, Palella F. Premature age-related comorbidities among HIV-infected persons compared with the general population. Clin Infect Dis. 2011;53:1120–1126. [Abstract] [Google Scholar]
- Hannum G, Guinney J, Zhao L, Zhang L, Hughes G, Sadda S, Klotzle B, Bibikova M, Fan J-B, Gao Y, et al. Genome-wide Methylation Profiles Reveal Quantitative Views of Human Aging Rates. Mol Cell. 2012:1–9. [Europe PMC free article] [Abstract] [Google Scholar]
- Heaton RK, Clifford DB, Franklin DR, Woods SP, Ake C, Vaida F, Ellis RJ, Letendre SL, Marcotte TD, Atkinson JH, et al. HIV-associated neurocognitive disorders persist in the era of potent antiretroviral therapy: CHARTER Study. Neurology. 2010;75:2087–2096. [Europe PMC free article] [Abstract] [Google Scholar]
- Heyn H, Li N, Ferreira HJ, Moran S, Pisano DG, Gomez A, Diez J, Sanchez-Mut JV, Setien F, Carmona FJ, et al. Distinct DNA methylomes of newborns and centenarians. Proc Natl Acad Sci USA. 2012;109:10522–10527. [Europe PMC free article] [Abstract] [Google Scholar]
- Horvath S. DNA methylation age of human tissues and cell types. Genome Biology. 2013;14:R115. [Europe PMC free article] [Abstract] [Google Scholar]
- Horvath S, Garagnani P, Bacalini MG, Pirazzini C, Salvioli S, Gentilini D, Di Blasio AM, Giuliani C, Tung S, Vinters HV, et al. Accelerated epigenetic aging in Down syndrome. Aging Cell 2015 [Europe PMC free article] [Abstract] [Google Scholar]
- Horvath S, Levine AJ. HIV-1 Infection Accelerates Age According to the Epigenetic Clock. Journal of Infectious Diseases. 2015;212:1563–1573. [Europe PMC free article] [Abstract] [Google Scholar]
- Houseman E, Accomando WP, Koestler DC, Christensen BC, Marsit CJ, Nelson HH, Wiencke JK, Kelsey KT. DNA methylation arrays as surrogate measures of cell mixture distribution. BMC Bioinformatics. 2012;13:86. [Europe PMC free article] [Abstract] [Google Scholar]
- International HIV Controllers Study. Pereyra F, Jia X, McLaren PJ, Telenti A, de Bakker PIW, Walker BD, Ripke S, Brumme CJ, Pulit SL, et al. The major genetic determinants of HIV-1 control affect HLA class I peptide presentation. Science. 2010;330:1551–1557. [Europe PMC free article] [Abstract] [Google Scholar]
- Jaffe AE, Irizarry RA. Accounting for cellular heterogeneity is critical in epigenome-wide association studies. Genome Biol. 2014;15:R31. [Europe PMC free article] [Abstract] [Google Scholar]
- Joshi D, O’Grady J, Dieterich D, Gazzard B, Agarwal K. Increasing burden of liver disease in patients with HIV infection. Lancet. 2011;377:1198–1209. [Abstract] [Google Scholar]
- Kennedy BK, Berger SL, Brunet A, Campisi J, Cuervo AM, Epel ES, Franceschi C, Lithgow GJ, Morimoto RI, Pessin JE, et al. Geroscience: linking aging to chronic disease. Cell. 2014;159:709–713. [Europe PMC free article] [Abstract] [Google Scholar]
- Kovari H, Sabin CA, Ledergerber B, Ryom L, Worm SW, Smith C, Phillips A, Reiss P, Fontas E, Petoumenos K, et al. Antiretroviral drug-related liver mortality among HIV-positive persons in the absence of hepatitis B or C virus coinfection: the data collection on adverse events of anti-HIV drugs study. Clin Infect Dis. 2013;56:870–879. [Europe PMC free article] [Abstract] [Google Scholar]
- Kulkarni S, Savan R, Qi Y, Gao X, Yuki Y, Bass SE, Martin MP, Hunt P, Deeks SG, Telenti A, et al. Differential microRNA regulation of HLA-C expression and its association with HIV control. Nature. 2011;472:495–498. [Europe PMC free article] [Abstract] [Google Scholar]
- Leeansyah E, Cameron PU, Solomon A, Tennakoon S, Velayudham P, Gouillou M, Spelman T, Hearps A, Fairley C, Smit DV, et al. Inhibition of Telomerase Activity by Human Immunodeficiency Virus (HIV) Nucleos(t)ide Reverse Transcriptase Inhibitors: A Potential Factor Contributing to HIV-Associated Accelerated Aging. Journal of Infectious Diseases. 2013;207:1157–1165. [Abstract] [Google Scholar]
- Leung V, Gillis J, Raboud J, Cooper C, Hogg RS, Loutfy MR, Machouf N, Montaner JSG, Rourke SB, Tsoukas C, et al. Predictors of CD4:CD8 ratio normalization and its effect on health outcomes in the era of combination antiretroviral therapy. PLoS ONE. 2013;8:e77665. [Europe PMC free article] [Abstract] [Google Scholar]
- Lindsey J, McGill NI, Lindsey LA, Green DK, Cooke HJ. In vivo loss of telomeric repeats with age in humans. Mutation Research/DNAging. 1991;256:45–48. [Abstract] [Google Scholar]
- Luz PM, Grinsztejn B, Velasque L, Pacheco AG, Veloso VG, Moore RD, Struchiner CJ. Long-term CD4+ cell count in response to combination antiretroviral therapy. PLoS ONE. 2014;9:e93039. [Europe PMC free article] [Abstract] [Google Scholar]
- Maartens G, Celum C, Lewin SR. HIV infection: epidemiology, pathogenesis, treatment, and prevention. Lancet. 2014;384:258–271. [Abstract] [Google Scholar]
- Marabita F, Almgren M, Lindholm ME, Ruhrmann S, Fagerström-Billai F, Jagodic M, Sundberg CJ, Ekström TJ, Teschendorff AE, Tegner J, et al. An evaluation of analysis pipelines for DNA methylation profiling using the Illumina HumanMethylation450 BeadChip platform. Epigenetics. 2013;8:333–346. [Europe PMC free article] [Abstract] [Google Scholar]
- Marioni RE, Shah S, McRae AF, Chen BH, Colicino E, Harris SE, Gibson J, Henders AK, Redmond P, Cox SR, et al. DNA methylation age of blood predicts all-cause mortality in later life. Genome Biol. 2015;16:25. [Europe PMC free article] [Abstract] [Google Scholar]
- Nightingale S, Winston A, Letendre S, Michael BD, McArthur JC, Khoo S, Solomon T. Controversies in HIV-associated neurocognitive disorders. Lancet Neurol. 2014;13:1139–1151. [Europe PMC free article] [Abstract] [Google Scholar]
- Numata S, Ye T, Hyde TM, Guitart-Navarro X, Tao R, Wininger M, Colantuoni C, Weinberger DR, Kleinman JE, Lipska BK. DNA methylation signatures in development and aging of the human prefrontal cortex. Am J Hum Genet. 2012;90:260–272. [Europe PMC free article] [Abstract] [Google Scholar]
- Pathai S, Lawn SD, Gilbert CE, McGuinness D, McGlynn L, Weiss HA, Port J, Christ T, Barclay K, Wood R, et al. Accelerated biological ageing in HIV-infected individuals in South Africa. Aids. 2013;27:2375–2384. [Europe PMC free article] [Abstract] [Google Scholar]
- Riboli E, Hunt KJ, Slimani N, Ferrari P, Norat T, Fahey M, Charrondière UR, Hémon B, Casagrande C, Vignat J, et al. European Prospective Investigation into Cancer and Nutrition (EPIC): study populations and data collection. Public Health Nutr. 2002;5:1113–1124. [Abstract] [Google Scholar]
- Rosenblatt HM, Stanley KE, Song LY, Johnson GM, Wiznia AA, Nachman SA, Krogstad PA Pediatric AIDS Clinical Trials Group 377 Study Team. Immunological response to highly active antiretroviral therapy in children with clinically stable HIV-1 infection. J Infect Dis. 2005;192:445–455. [Abstract] [Google Scholar]
- Rickabaugh TM, Kilpatrick RD, Hultin LE, Hultin PM, Hausner MA, Sugar CA, Althoff KN, Margolick JB, Rinaldo CR, Detels R, et al. The Dual Impact of HIV-1 Infection and Aging on Naïve CD4+ T-Cells: Additive and Distinct Patterns of Impairment. PLoS ONE. 2011;6:e16459. [Europe PMC free article] [Abstract] [Google Scholar]
- Rickabaugh TM, Baxter RM, Sehl M, Sinsheimer JS, Hultin PM, Hultin LE, Quach A, Martínez-Maza O, Horvath S, Vilain E, et al. Acceleration of Age-Associated Methylation Patterns in HIV-1-Infected Adults. PLOS ONE. 2015;10:e0119201. [Europe PMC free article] [Abstract] [Google Scholar]
- Serrano-Villar S, Sainz T, Lee SA, Hunt PW, Sinclair E, Shacklett BL, Ferre AL, Hayes TL, Somsouk M, Hsue PY, et al. HIV-infected individuals with low CD4/CD8 ratio despite effective antiretroviral therapy exhibit altered T cell subsets, heightened CD8+ T cell activation, and increased risk of non-AIDS morbidity and mortality. PLoS Pathog. 2014;10:e1004078. [Europe PMC free article] [Abstract] [Google Scholar]
- Siebold AP, Banerjee R, Tie F, Kiss DL, Moskowitz J, Harte PJ. Polycomb Repressive Complex 2 and Trithorax modulate Drosophila longevity and stress resistance. Proc Natl Acad Sci USA. 2010;107:169–174. [Europe PMC free article] [Abstract] [Google Scholar]
- Smith RL, de Boer R, Brul S, Budovskaya Y, van Spek H. Premature and accelerated aging: HIV or HAART? Front Genet. 2012;3:328. [Europe PMC free article] [Abstract] [Google Scholar]
- Solomon A, Tennakoon S, Leeansyah E, Arribas J, Hill A, Van Delft Y, Moecklinghoff C, Lewin SR. No difference in the rate of change in telomere length or telomerase activity in HIV-infected patients after three years of darunavir/ritonavir with and without nucleoside analogues in the MONET trial. PLoS ONE. 2014;9:e109718. [Europe PMC free article] [Abstract] [Google Scholar]
- Strategies for Management of Antiretroviral Therapy (SMART) Study Group. El-Sadr WM, Lundgren JD, Neaton JD, Gordin F, Abrams D, Arduino RC, Babiker A, Burman W, Clumeck N, et al. CD4+ count-guided interruption of antiretroviral treatment. N Engl J Med. 2006;355:2283–2296. [Abstract] [Google Scholar]
- Teschendorff AE, Menon U, Gentry-Maharaj A, Ramus SJ, Weisenberger DJ, Shen H, Campan M, Noushmehr H, Bell CG, Maxwell AP, et al. Age-dependent DNA methylation of genes that are suppressed in stem cells is a hallmark of cancer. Genome Research. 2010;20:440–446. [Europe PMC free article] [Abstract] [Google Scholar]
- Teschendorff AE, Marabita F, Lechner M, Bartlett T, Tegner J, Gomez-Cabrero D, Beck S. A beta-mixture quantile normalization method for correcting probe design bias in Illumina Infinium 450 k DNA methylation data. Bioinformatics. 2013;29:189–196. [Europe PMC free article] [Abstract] [Google Scholar]
- Thomas R, Apps R, Qi Y, Gao X, Male V, O’hUigin C, O’Connor G, Ge D, Fellay J, Martin JN, et al. HLA-C cell surface expression and control of HIV/AIDS correlate with a variant upstream of HLA-C. Nat Genet. 2009;41:1290–1294. [Europe PMC free article] [Abstract] [Google Scholar]
- Torres RA, Lewis W. Aging and HIV/AIDS: pathogenetic role of therapeutic side effects. Lab Invest. 2014;94:120–128. [Europe PMC free article] [Abstract] [Google Scholar]
- Trono D, Van Lint C, Rouzioux C, Verdin E, Barré-Sinoussi F, Chun TW, Chomont N. HIV persistence and the prospect of long-term drug-free remissions for HIV-infected individuals. Science. 2010;329:174–180. [Abstract] [Google Scholar]
- Weidner CI, Lin Q, Koch CM, Eisele L, Beier F, Ziegler P, Bauerschlag DO, Jöckel KH, Erbel R, Mühleisen TW, et al. Aging of blood can be tracked by DNA methylation changes at just three CpG sites. Genome Biol. 2014;15:R24. [Europe PMC free article] [Abstract] [Google Scholar]
- West J, Beck S, Wang X, Teschendorff AE. An integrative network algorithm identifies age-associated differential methylation interactome hotspots targeting stem-cell differentiation pathways. Sci Rep. 2013;3:1630. [Europe PMC free article] [Abstract] [Google Scholar]
- Zanet DL, Thorne A, Singer J, Maan EJ, Sattha B, Le Campion A, Soudeyns H, Pick N, Murray M, Money DM, et al. Association Between Short Leukocyte Telomere Length and HIV Infection in a Cohort Study: No Evidence of a Relationship With Antiretroviral Therapy. Clinical Infectious Diseases. 2014;58:1322–1332. [Abstract] [Google Scholar]
Full text links
Read article at publisher's site: https://doi.org/10.1016/j.molcel.2016.03.019
Read article for free, from open access legal sources, via Unpaywall:
http://www.cell.com/article/S1097276516300028/pdf
Citations & impact
Impact metrics
Citations of article over time
Alternative metrics
Article citations
Microglia and macrophages alterations in the CNS during acute SIV infection: A single-cell analysis in rhesus macaques.
PLoS Pathog, 20(9):e1012168, 16 Sep 2024
Cited by: 2 articles | PMID: 39283947 | PMCID: PMC11426456
Deciphering the role of FOXP4 in long COVID: exploring genetic associations, evolutionary conservation, and drug identification through bioinformatics analysis.
Funct Integr Genomics, 24(5):167, 19 Sep 2024
Cited by: 0 articles | PMID: 39298002
A systematic review of phenotypic and epigenetic clocks used for aging and mortality quantification in humans.
Aging (Albany NY), 16(17):12414-12427, 30 Aug 2024
Cited by: 0 articles | PMID: 39215995 | PMCID: PMC11424583
Review Free full text in Europe PMC
Retro-age: A unique epigenetic biomarker of aging captured by DNA methylation states of retroelements.
Aging Cell, 23(10):e14288, 02 Aug 2024
Cited by: 1 article | PMID: 39092674 | PMCID: PMC11464121
Chronic and Latent Viral Infections and Leukocyte Telomere Length across the Lifespan of Female and Male Individuals Living with or without HIV.
Viruses, 16(5):755, 10 May 2024
Cited by: 0 articles | PMID: 38793637 | PMCID: PMC11125719
Go to all (178) article citations
Other citations
Data
Data behind the article
This data has been text mined from the article, or deposited into data resources.
BioStudies: supplemental material and supporting data
GEO - Gene Expression Omnibus (3)
- (1 citation) GEO - GSE51032
- (1 citation) GEO - GSE40279
- (1 citation) GEO - GSE67705
Similar Articles
To arrive at the top five similar articles we use a word-weighted algorithm to compare words from the Title and Abstract of each citation.
DNA Methylation as a Biomarker of Aging in Epidemiologic Studies.
Methods Mol Biol, 1856:219-231, 01 Jan 2018
Cited by: 14 articles | PMID: 30178254
Review
An Epigenetic Clock Measures Accelerated Aging in Treated HIV Infection.
Mol Cell, 62(2):153-155, 01 Apr 2016
Cited by: 20 articles | PMID: 27105110 | PMCID: PMC5315270
Expression profiling of chromatin-modifying enzymes and global DNA methylation in CD4+ T cells from patients with chronic HIV infection at different HIV control and progression states.
Clin Epigenetics, 10:20, 13 Feb 2018
Cited by: 17 articles | PMID: 29449904 | PMCID: PMC5812196
The methylome of the celiac intestinal epithelium harbours genotype-independent alterations in the HLA region.
Sci Rep, 9(1):1298, 04 Feb 2019
Cited by: 12 articles | PMID: 30718669 | PMCID: PMC6362130
Funding
Funders who supported this work.
NCI NIH HHS (2)
Grant ID: U24 CA184427
Grant ID: P30 CA023100
NIDA NIH HHS (1)
Grant ID: R01 DA030962
NIGMS NIH HHS (2)
Grant ID: T32 GM008666
Grant ID: P30 GM103509
NIMH NIH HHS (3)
Grant ID: HHSN271201000030C
Grant ID: P30 MH062261
Grant ID: U24 MH100925