Abstract
Free full text

Migration of Cytotoxic T Lymphocytes in 3D Collagen Matrices
Abstract
CD8+ cytotoxic T lymphocytes (CTL) and natural killer cells are the main cytotoxic killer cells of the human body to eliminate pathogen-infected or tumorigenic cells (also known as target cells). To find their targets, they have to navigate and migrate through complex biological microenvironments, a key component of which is the extracellular matrix (ECM). The mechanisms underlying killer cell’s navigation are not well understood. To mimic an ECM, we use a matrix formed by different collagen concentrations and analyze migration trajectories of primary human CTLs. Different migration patterns are observed and can be grouped into three motility types: slow, fast, and mixed. The dynamics are well described by a two-state persistent random walk model, which allows cells to switch between slow motion with low persistence and fast motion with high persistence. We hypothesize that the slow motility mode describes CTLs creating channels through the collagen matrix by deforming and tearing apart collagen fibers and that the fast motility mode describes CTLs moving within these channels. Experimental evidence supporting this scenario is presented by visualizing migrating T cells following each other on exactly the same track and showing cells moving quickly in channel-like cavities within the surrounding collagen matrix. Consequently, the efficiency of the stochastic search process of CTLs in the ECM should strongly be influenced by a dynamically changing channel network produced by the killer cells themselves.
Significance
Cytotoxic T lymphocytes (CTLs) are key players of the adaptive immune system and eliminate tumor cells or pathogen-infected cells. To fulfill their functions in the body, they migrate in complex biological microenvironments, which is shaped by the extracellular matrix. The mechanisms underlying their navigation and search strategy are not well understood. To better understand CTL migration in the extracellular matrix, we analyze their trajectories in three-dimensional collagen networks. Our main observation is that CTLs tear the collagen fibers forming channels, which facilitate movement of other T cells in the collagen network. We describe the underlying slow, fast, and mixed motilities and observed persistences by a two-state random walk model, which reproduces the behavior of CTLs without additional free parameters.
Introduction
Cytotoxic T lymphocytes (CTLs) are fully activated CD8+ T cells, which are key players of the adaptive immune system to eliminate tumorigenic or pathogen-infected cells (1). CTLs need to find the cognate antigens presented by target cells, for example pathogen-infected or tumorigenic cells, to eliminate those aberrant cells in the immunosurveillance process. These target cells are often low in number in the early stages of disease development (2, 3, 4). Thus, the ability of CTLs to efficiently navigate and search is crucial for an efficient immune response. Migration behavior of immune cells in the body and the search strategies they might follow is currently of great interest in physics and biology (5, 6, 7). Migration of naive T cells in lymph nodes reportedly follows a Brownian or even subdiffusive dynamics (8, 9, 10), but switchings between fast and slow motility modes have also been observed (11). Outside the lymph node, activated T cells are destined to find their targets in peripheral tissues, most of which are characterized by a dense extracellular matrix (ECM) (12). Here, a faster migration, e.g., via longer phases of superdiffusive dynamics or less switchings to the slow diffusive mode, is advantageous to scan a larger tissue efficiently. For instance, it was reported that the dynamics of CD8+ T cells in infected brain tissue resembles a Levy walk (13).
The ECM—the major component of peripheral tissues—mainly consists of collagens and has essential regulatory roles in nearly all cellular functions. Some collagens have inhibitory effects on the function of different immune cells (14,15). In various types of cancer, the collagen network becomes dense, stiff, and linearized in the vicinity of tumors, facilitating the transport of cancerous cells and making the ECM an important player in cancer metastasis, intravasion, and prognosis (16, 17, 18, 19, 20, 21). Additionally, the proliferation of CTLs is impaired in a high-density collagen matrix (22). Recently, different immune cells have been investigated in immunotherapy studies as potential drug delivery vehicles into tumors (23,24). Understanding the migration and interactions of immune cells in collagen networks is crucial to unravel the underlying details of the immune response and design effective treatment strategies.
Collagen-based assays have been used to investigate the migration of lymphocytes in ECM and study the possible underlying mechanisms of immune interactions with ECM (14,25, 26, 27, 28, 29). In a recent study, collagen hydrogels were employed to compare migration patterns of human CD8+ T cells in aligned and nonaligned collagen fibers microenvironments, resembling tumor cells and normal tissues, respectively (30).
In this study, we use bovine collagen to construct a three-dimensional (3D) environment in vitro as a model for the ECM. The trajectories of primary human CTLs in collagen matrices with different concentrations are analyzed for two blood donors. We find three different types of motion in both donors; the migration of CTLs can be categorized into slow, fast, and mixed subgroups and show that a persistent random walk model with two different motility states, a slow one and a fast one, and transitions between describes the experimental data accurately. Finally, we provide a biophysical interpretation of the two motility modes of T cells in collagen matrix related to channel formation and movement within channels.
Materials and Methods
Ethical considerations
Research carried out for this study with human material (leukocyte reduction system chambers from human blood donors) is authorized by the local ethics committee (declaration from 16.4.2015 (84/15; Prof. Dr. Rettig-Stürmer)).
Human primary CTL isolation, stimulation, and nucleofection of CTLs
Peripheral blood mononuclear cells were obtained from healthy donors as previously described (31). Human primary CTLs were negatively isolated from peripheral blood mononuclear cells using Dynabeads Untouched Human CD8 T Cells Kit (Thermo Fisher Scientific, Waltham, MA) or CD8+ T Cell Isolation Kit, human (Miltenyi Biotec, Bergisch Gladbach, Germany), stimulated with Dynabeads Human T-Activator CD3/CD28 (Thermo Fisher Scientific) in AIMV medium (Thermo Fisher Scientific) with 10% fetal calf serum (FCS) and 33 U/mL of recombinant human IL-2 (Thermo Fisher Scientific). 48 h after stimulation, beads were removed, and 5 × 106 CTLs were electroporated with 2 μg plasmid (H2B-GFP to label nucleus (32) and LifeAct-mRuby or pMax-mCherry to label cell bodies) using the Human T Cell Nucleofector Kit (Lonza, Basel, Switzerland). Medium was changed 6 h after nucleofection, and transfected CTLs were maintained in AIMV medium (Thermo Fisher Scientific) with 10% FCS and 33 U/mL of recombinant human IL-2 (Thermo Fisher Scientific). Cells were used 24–36 h after nucleofection (33).
3D live cell imaging
3D live cell imaging was visualized with Cell Observer or light-sheet microscopy as described previously (34). Briefly, human primary CTLs were resuspended first in phosphate-buffered saline (Thermo Fisher Scientific); afterwards, neutralized collagen stock solution (bovine collagen type I, 8 mg/mL; Advanced BioMatrix, Carlsbad, CA) was added to a final concentration of 2, 4, or 5 mg/mL collagen with a cell density of 10 × 106 cells/mL. This cell/collagen mixture was loaded in a capillary for light-sheet microscopy or in a chamber with a coverslip on top for Cell Observer. The capillary was closed and incubated for 60 min in an incubator. Afterwards, the polymerized collagen rod was pushed out hanging in the medium at 37°C with 5% CO2 for equilibration for another 60 min. To visualize collagen structure, analyzed collagen matrix was stained with 50 μg/mL Atto 488 N-hydroxysuccinimide (NHS) ester (Thermo Fisher Scientific) in AIMV medium at room temperature after collagen polymerization. Afterwards, the matrix was washed in AIMV medium. After collagen polymerization, cells in the matrix with or without collagen staining were incubated in AIMV medium with 10% FCS at 37°C with 5% CO2 for 1 h. Afterwards, the migration of cells was visualized by light-sheet microscopy (20× objective) at 37°C with a z-step size of 1 μm and a time interval of 30 s. The migration trajectories were tracked and analyzed using Imaris 8.1.2 (containing Imaris, ImarisTrack, ImarisMeasurementPro, and ImarisVantage; Bitplane, software available at http://bitplane.com) (34).
Data analysis
The experimental trajectories consist of a set of T cell positions recorded after equal time intervals. Every two successive recorded positions are used to calculate the instantaneous velocity, and every three of them to extract the corresponding turning angle ϕ. The values of ϕ around zero represent a tendency to continue along the previous direction of motion, i.e., a persistent motion. In contrast, ϕ-values close to π indicate reversing the direction of motion. Accordingly, we define the instantaneous persistence as
We apply a minimal duration threshold of six frames (i.e., 3 min) to filter out short trajectories, which leads to smooth velocity and turning angle distributions. We checked that moderate changes of the minimal duration of trajectories has a negligible influence on the relative population of different categories of T cell migration pattern. Moreover, a second threshold is applied on the duration of trajectories when analyzing the switching statistics between the substates of the mixed migration type. To minimize the effects of the tracking time window, we only analyze trajectories with the duration of 50 min (i.e., 100 frames). The longest possible tracking time is 60 min because the duration of our live cell imaging is 1 h. We checked that the switching statistics are not significantly affected by a slight change (~20%) of the minimal trajectory duration.
T cells enter the camera field at different times. We shift the starting time of all trajectories so that all tracks start at the same time (t = 0). Throughout the manuscript, we use the notation t for the time from the beginning of each track and Δt for the time interval between two successive recorded positions.
Results
To investigate migration patterns of CTLs in a 3D environment, we embedded primary human CTLs into collagen matrices and visualized their movements using light-sheet microscopy (Fig. 1). Different concentrations of collagen mimic the ECM of normal tissue (2 mg/mL), soft solid tumor (4 mg/mL), and hard solid tumor (5 mg/mL), respectively (35, 36, 37). The resulting parameters are summarized in Table 1. The cross correlation between velocity and persistence,
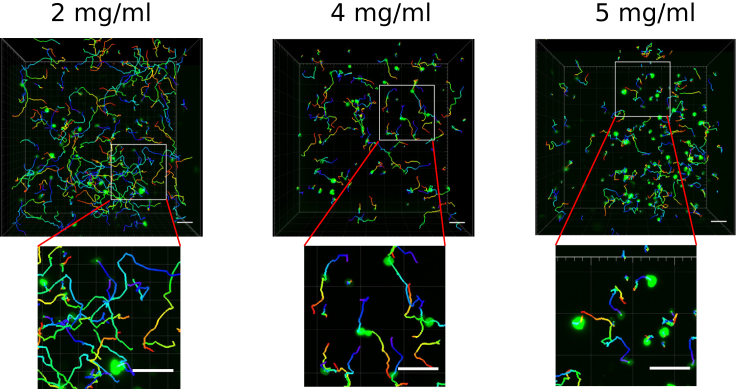
Trajectories of CTLs in 3D collagen matrices. CTL migration was visualized by light-sheet microscopy (20× objective) at 37°C for 30 min with an interval of 30 s in 3D collagen matrices with different concentrations. The nuclei of human primary CTLs were labeled with overexpressed Histone 2B-GFP (green). CTL migration trajectories were tracked automatically using Imaris 8.1.2. Scale bars, 40 μm. In the lower panels, magnified images of the area marked by the solid squares are shown. To see this figure in color, go online.
Table 1
Key Statistical Parameters of T Cells in Collagen Matrices with Different Densities
Donor 1 | Donor 2 | |||||
---|---|---|---|---|---|---|
Density | 2 | 4 | 5 | 2 | 4 | 5 |
ν ± ste | 0.10 ± 0.002 | 0.06 ± 0.002 | 0.05 ± 0.003 | 0.07 ± 0.004 | 0.04 ± 0.002 | 0.03 ± 0.001 |
0.30 ± 0.018 | 0.36 ± 0.024 | 0.35 ± 0.018 | 0.30 ± 0.044 | 0.36 ± 0.026 | 0.44 ± 0.03 | |
0.64 | 0.35 | 0.35 | 0.36 | 0.29 | 0.31 |
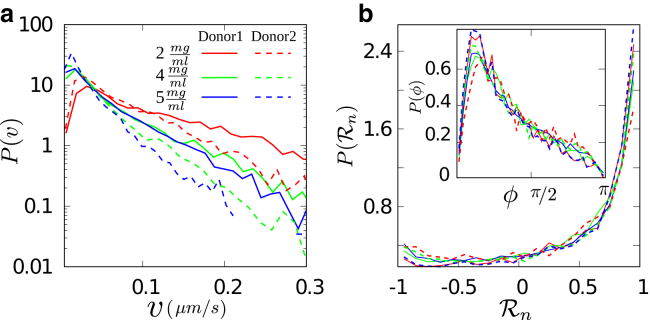
Distributions of (a) velocity and (b) persistence of T cells in collagen networks with different concentrations. Turning angle distributions are shown in the inset. To see this figure in color, go online.
T cell dynamics
To better understand the dynamics of T cells in matrices with different collagen concentrations, we analyze the velocity autocorrelation Cν,ν as well as the mean-square displacement (MSD) separately for each experimental condition in Fig. 3. The gray dashed line in Fig. 3 b corresponds to Brownian diffusion. The smaller slope of the MSD curves under all conditions shows that the cell motion is slower than normal diffusion and eventually crosses over to diffusive dynamics at long times. Both the decay of Cν,ν and the crossover of the MSD to asymptotic diffusion show that the cell orientation is randomized after a while.
Three motility groups can be distinguished in CTL dynamics
Single track analysis of CTL trajectories reveals that there are three different types of CTL trajectories: 1) slow T cells that perform a subdiffusive motion with velocities that always remain below a threshold value, 2) a faster group with velocities always above a threshold value, and 3) the third group with velocities that switch between fast and slow modes. In our analysis, we noticed that CTLs from both donors have all motility types, although the corresponding fractions are not exactly the same in both donors (shown in Table 2). This difference could owe to variations between donors because the CTLs were primary CD8+ T cells. The velocity evolution of typical tracks and a few trajectories for each cell motility type are shown in Fig. 4.
Table 2
Percentage of Different Motility Types of CTLs and the Threshold Velocities νc1 and νc2 to Categorize T Cell Migration Patterns for Each Experiment with a Different Donor or Collagen Density
Donor 1 | Donor 2 | |||||
---|---|---|---|---|---|---|
Density | 2 | 4 | 5 | 2 | 4 | 5 |
Slow (%) | 33 | 43 | 48 | 15 | 27 | 26 |
Fast (%) | 35 | 14 | 19 | 30 | 25 | 24 |
Mixed (%) | 26 | 37 | 25 | 48 | 40 | 43 |
νc1 (μm/s) | 0.075 | 0.055 | 0.04 | 0.05 | 0.02 | 0.02 |
νc2 (μm/s) | 0.11 | 0.09 | 0.10 | 0.08 | 0.04 | 0.04 |
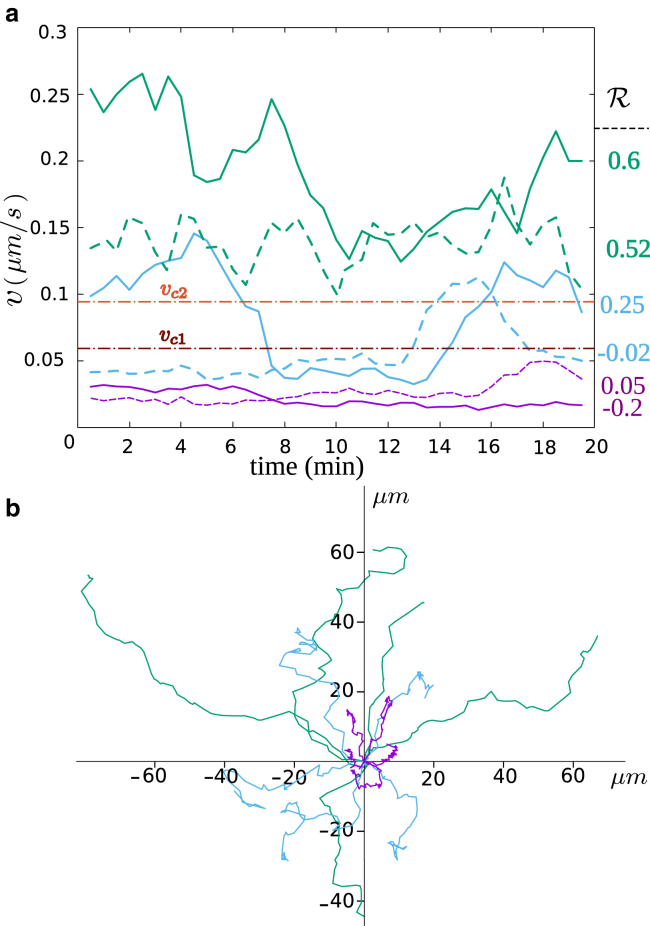
(a) Typical velocities of CTL trajectories. Green, purple, and blue colors correspond to a few examplary trajectories of fast, slow, and mixed types of motility, respectively. The corresponding persistence value
We define the three classes of trajectory types—fast, slow, and mixed—by introducing two threshold velocities νc1 and νc2 (νc2 > νc1 > 0). A trajectory is classified into the “fast” type if the velocity is at all time t larger than νc2, i.e., ν(t) > νc2 for all t. It is of the “slow” type if its velocity is always smaller than νc1, i.e., ν(t) < νc1 for all t and of the “mixed” type if ν(t) > νc2 for some t and ν(t′) < νc1 for some other t′. For each experimental data set, distinguished by donor and collagen density, we adapt (νc1, νc2) such that the number of trajectories that does not belong into one of the defined classes (e.g., those with ν(t) > νc1 for all t but not ν(t) > νc2 for all t) becomes minimal. We checked that in all experiments, the trajectories that do not belong to any of the three identified migration categories remain below 6–8% of all trajectories when adopting an optimal set of (νc1, νc2). The relative populations of the cells in three migration types moderately change upon varying the threshold velocities νc1 and νc2 around their optimal choices. Throughout the article, we refer to the fast and slow migration types with F and S subscripts and represent the slow and fast substates of the mixed type of migration with subscripts I and II, respectively.
To further confirm that the three populations are not donor dependent, we pooled data from two donors and analyzed the average velocities and persistence of all populations. Fig. 5, a and b summarizes the average velocities and persistences of different types in different collagen concentrations for both donors. One observes a moderate increase of the average persistence, and a moderate decrease of the average velocity is observed with increasing collagen density. The scatter plots of instantaneous persistence versus velocity, which are shown in Fig. 5 c in different collagen densities, indicate once again that the faster T cells are more persistent than the slow ones. The MSD of different types of motion are clearly distinguishable (see e.g., Fig. 5 d). The similarities in the overall time evolution of the MSD curves indicate that the underlying structures guiding the cells are similar. The differences in the level of MSD curves reflect the differences in the average velocity of CTLs in various migration types. In the next section, we compare the MSD T cell trajectories with the prediction of a two-state random velocity model.

(a)
The two-state motility type
In the following, we study the mixed-velocity trajectories of T cells in more details.
MSD
The MSD of the mixed type coincides with the total MSD in nearly all cases (see the solid line in Fig. 5 d). This shows that the mean velocity of all T cells is nearly the same as the mean velocity of the mixed type when the resident times in the substates of the mixed type are taken into account.
Exponential distribution of sojourn time in each state
The distribution of the times that the T cells remain in one state before they switch, the so-called sojourn times, follow an exponential decay as shown in Fig. 6. In this example, the sojourn time distribution of T cells in the different states of the mixed T cell migration type of two donors is plotted. The exponential decay of these distributions indicates that the transition probabilities are time independent.
Probability distribution of persistence in different migration types
Fig. 7 shows the probability distribution of the instantaneous persistence of the three different cell types. Whereas the distributions for fast and mixed types show a persistent motion for all collagen concentrations, slow T cells perform antipersistent motion in 2 mg/mL collagen and become persistent in denser ones. A possible explanation is that the average pore size increases with decreasing collagen density (38), which implies that for low collagen densities, T cells can more easily find some pores around them that are large enough to get into them; this might allow slow T cells to change their direction when they face a constriction while creating a channel. This is, however, less probable in denser collagens as most pores are smaller than T cells and need equal effort to pass.

(a) Probability distributions of persistence p < 0.001, t-test). The error bars represent the standard error. (c) Shown is similar to (a) for fast and mixed cell types. To see this figure in color, go online.
CTLs enter channels in the collagen matrix
Next, we explored in detail how CTLs migrate in collagen matrices. We fluorescently stained collagen and visualized the movements of CTLs using light-sheet microscopy. We found that during migration, CTLs could enter channels in the collagen matrix. Inside the channel, they had a high speed that was significantly slowed down when leaving the channel (Fig. 8, a and c; Video S1). Slow CTLs appeared to be trapped in some channels and moved slowly (Fig. 8, b and c; Video S2). In addition, we observed that in some cases, after one CTL migrated through the matrix, a second CTL followed the same path, indicating that CTLs use channels created by other cells (see Fig. 8 d; Video S3). When the trajectories of two CTLs overlap, the following cell probably moves within the channel created by the leading cell, resulting in a substantial velocity increase of the following cells as shown in Fig. 8 e.

Visualization of CTL migration in 3D collagen matrices. Primary Human CTLs were transiently transfected with mCherry (red). Collagen (5 mg/mL) was stained with Atto 488 NHS ester (green or gray as indicated). CTL migration was visualized at 37°C using light-sheet microscopy. Exemplary cells for mixed and slow migration are shown in (a and b), respectively (Videos S1 and S2). One layer of z-stack is presented. (c) Quantification of migration velocity at all time points were examined. CTL migration trajectories were tracked and analyzed by Imaris. Scale bars, 10 μm. (d) Shown is an exemplary migrating CTL following another cell in the same trace. CTLs were visualized with Cell Observer. Nuclei were labeled with Hoechst 33342 and tracked with Imaris. The trajectory of the preceding and the succeeding cell is in red and purple, respectively. Scale bars, 7 μm (Video S3). (e) The velocity of the preceding cell is similar with the succeeding cell before the overlapping part of the traces. When the traces overlap, the velocity of the succeeding cell increases. Each dot presents one time point for the same cell. (f) The correlation of cell body volume and velocity during cell migration is shown. Each dot represents one cell. All results were from at least three independent experiments. The error bars represent the standard error. To see this figure in color, go online.
CTLs (bead-stimulated primary human CD8+ T cells) were transiently transfected with mCherry (red). Collagen (5 mg/ml) was stained with Atto 488 NHS (left: in green, right: in grey). CTL migration was visualized using lightsheet microscopy with a 20X objective at 37oC with an interval of 30 sec. A z-stack was acquired with a step size of 1 μm. One layer is shown here. Time stamp is HH:MM:SS. Scale bar is 10 μm.
CTLs (bead-stimulated primary human CD8+ T cells) were transiently transfected with mCherry (red). Collagen (5 mg/ml) was stained with Atto 488 NHS (left: in green, right: in grey). CTL migration was visualized using lightsheet microscopy with a 20X objective at 37oC with an interval of 30 sec. A z-stack was acquired with a step size of 1 μm. One layer is shown here. Time stamp is HH:MM:SS. Scale bar is 10 μm.
CTLs (bead-stimulated primary human CD8+ T cells) were stained with Hoechst 33342 (blue) and then embedded in collagen matrix (2 mg/ml). Cell migration was visualized using Cell Observer (20X objective) at 37oC with an interval of 30 sec. The migration trajectories were tracked with Imaris8.1.2. The trajectory of the preceding and the succeeding cell are in red and purple, respectively. Time stamp is HH:MM:SS. Scale bar is 7 μm.
CTLs form channels in the matrix during migration
To explore in detail how CTLs migrate in collagen matrices, we investigated whether CTLs can actively create channels while migrating through the matrix. As mentioned earlier, CTLs transiently transfected with red fluorescent protein mCherry were embedded in the fluorescently labeled collagen matrix. As shown in Video S4, using light-sheet microscopy, we observed that during migration, CTLs formed protrusions (blob-like structures) at the leading edge and that these protrusions preferably extended to deformable parts of the matrix and push the matrix aside. After CTLs went through the area, the matrix sprang back to some extent but did not relax to the original form. Therefore, we conclude that through migration, CTLs broaden more easily deformable parts of the matrix to create channels, which plausibly facilitates the migration of the other CTLs entering the same area.
CTLs (bead-stimulated primary human CD8+ T cells) were transiently transfected with mCherry (red). Collagen (5 mg/ml) was stained with Atto 488 NHS (left: in green, right: in grey). CTL migration was visualized using lightsheet microscopy with a 20X objective with an interval of 30 sec. A z-stack was acquired with a step size of 1 μm. Maximum intensity projection is shown in the top panel. Middle panel isone layer of the z-stack, where a channel, which was broadened by the CTL (highlighted by the arrow on the right-hand side), is shown. The lowest panel is one layer of the z-stack, where the pre-existing channel that was broadened by the CTL is shown and its contour at time 0 is shown with the dotted line. Time stamp is MM:SS. Scale bar is 10 μm.
CTL size is not involved in migration speed
To examine whether cell size is involved in migration speed, we analyzed the correlation between cell body volume and velocity for the same cells over time and found no correlation between cell size and migration speed (Fig. 8 f), indicating that the cell size per se plays an unlikely role in determination of fast, mixed, or slow migration types.
Two-state persistent random walk model
In the following, we show that the experimentally measured T cell trajectories are well described by a stochastic process that involves a persistent random walk with two different motility states (39). Similar stochastic two-state models have been widely used to describe altering phases of motion in other systems (40,52, 53, 54).
We adopt a discrete time approach because it is best adapted to our experimental data, which consists of the positions of the CTLs at equidistant times. First, we focus on the trajectories of the mixed type, which involves the slow and the fast motility mode and transitions between them. Later, we show that the trajectories of the fast type and those of the slow type are described by the same stochastic process as the mixed type but without transitions from the fast to the slow mode or from low to fast, respectively. This observation complies with the physical interpretation that the slow motility mode is caused by CTLs creating new channels in the collagen matrix and the fast motility mode by CTLs using already existing channels. A persistent random walk in discrete time is a stochastic process for the position of a particle that moves during a time interval Δt with a certain velocity v in a certain direction ϕ. At the end of the time interval, a transition takes place to a new velocity and a new direction. These transitions are characterized by a velocity distribution F(ν) and a turning angle distribution R(ϕ). A persistent random walk with two motility states involves two velocity distributions FI(ν) and FII(ν) and two turning angle distribution RI(ϕ) and RII(ϕ) for the slow (I) and fast (II) motility mode and transitions between the two motility states characterized by transition probabilities κI→II and κII→I for switching from state I to II and vice versa. These probabilities are estimated by the inverse of the sojourn time in the two states of mixed trajectories, e.g.,
with x′ = x − νΔtcosθ and y′ = y − νΔtsinθ. By solving these sets of master equations, one can evaluate arbitrary moments of the position of the walker, such as the MSD. The analytical details to calculate the MSD are presented in the Appendix. It should be emphasized that the derived formula for the MSD, i.e., the second moment of the position, depends only on the first two moments of the velocity distributions FI(ν) and FII(ν) and the first moment of the turning angle distribution.
We extracted the model parameters from the experimental data analysis; thus, there remains no free parameter to be tuned. In the case of the mixed type, moments of velocity (
Table 3
Parameters Extracted from the Experimental Data of Mixed Type of Motility and Used in the Two-State Model
Donor 1 | Donor 2 | |||||
---|---|---|---|---|---|---|
Density | 2 | 4 | 5 | 2 | 4 | 5 |
0.066 | 0.047 | 0.048 | 0.047 | 0.023 | 0.020 | |
0.138 | 0.120 | 0.111 | 0.099 | 0.061 | 0.055 | |
0.0189 | 0.0038 | 0.0037 | 0.0149 | 0.0009 | 0.0006 | |
0.0510 | 0.0198 | 0.0165 | 0.038 | 0.0055 | 0.0040 | |
RI | 0.15 | 0.35 | 0.38 | 0.19 | 0.26 | 0.35 |
RII | 0.62 | 0.59 | 0.59 | 0.33 | 0.56 | 0.69 |
Δt (min) | 0.5 | 0.5 | 0.5 | 0.5 | 0.5 | 0.5 |
κI→II | 0.20 | 0.08 | 0.09 | 0.11 | 0.10 | 0.07 |
κII→I | 0.06 | 0.14 | 0.17 | 0.14 | 0.11 | 0.13 |
2.5 | 6.2 | 5.5 | 4.5 | 5 | 7.1 | |
8.3 | 3.5 | 2.9 | 3.6 | 4.6 | 3.8 |
Table 4
Parameters Extracted from the Experimental Data of Fast (Up) and Slow (Down) Types of Motility
Donor 1 | Donor 2 | |||||
---|---|---|---|---|---|---|
Density | 2 | 4 | 5 | 2 | 4 | 5 |
0.17 | 0.12 | 0.09 | 0.11 | 0.07 | 0.07 | |
0.033 | 0.019 | 0.013 | 0.018 | 0.008 | 0.006 | |
RF | 0.54 | 0.41 | 0.45 | 0.53 | 0.40 | 0.56 |
Δt (min) | 0.5 | 0.5 | 0.5 | 0.5 | 0.5 | 0.5 |
0.032 | 0.019 | 0.017 | 0.027 | 0.011 | 0.010 | |
0.0014 | 0.0006 | 0.0005 | 0.0010 | 0.0002 | 0.0001 | |
RS | −0.12 | 0.18 | 0.19 | 0.05 | 0.21 | 0.37 |
Δt (min) | 0.5 | 0.5 | 0.5 | 0.5 | 0.5 | 0.5 |
The time evolution of the MSD obtained from the model is in good agreement with the data (exemplary match is shown in Fig. 9). Although the model describes the dynamics of T cells very well, the MSD does not contain further distinctive information to differentiate between various migration types of T cells.
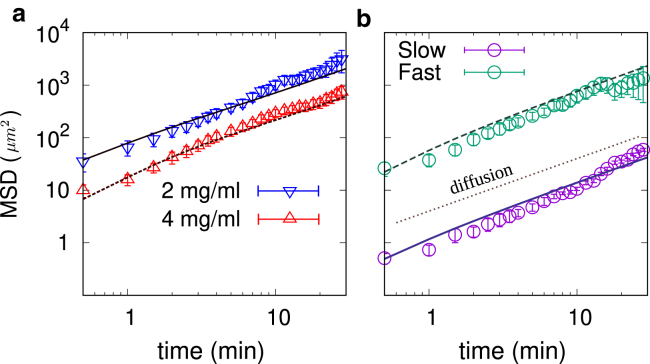
(a) The MSD of mixed motility type in various collagen concentrations obtained from the experiments (symbols) and analytical approach (Eq. 2 with parameters extracted from experimental data summarized in Table 3) (solid lines) for donor 2. (b) Shown is the MSD of slow and fast motility types in collagen concentration 4 mg/mL. The solid lines represent the theoretical estimate of Eq. 5 with parameters extracted from the experiments (Table 4). The error bars represent the standard error. To see this figure in color, go online.
Discussion
We analyzed the trajectories of CTLs within 3D collagen matrices with different concentrations. We found three motility types in all experiments: slow, fast, and mixed. Similar motility types have been reported for natural killer cells in hydrogel collagen with a concentration of 3 mg/mL in the presence of target cells (29). The similarity of the characteristics of CTLs and natural killer cell trajectories points toward a common mechanism for migration of both cell types through collagen networks.
A plausible scenario to explain our findings is that the cells that arrive first in the collagen network perform a persistent random walk unless they move into denser areas of the network, where they become slower but eventually find a way to move again, which leads to two-state motility. When the cells move through the collagen network, they leave a channel by displacing or stretching collagen fibers. These channels facilitate the movement of other T cells, such that cells entering already existing channels move faster and tend to remain in the existing channel network. They do not switch to slow movement and thus establish the fast type. The slow cells mainly remain in one part of the network and only “wiggle” around and seem to be nearly immobile.
In principle, more and more channels can be built by migrating T cells with time, therefore leading to an increase in overall migration speed over time. For our experiments, however, the visualization period was about an hour, and we can estimate the number of new channels that are produced by the T cells. The cell density is 107 cells/mL, which gives an average cell to cell distance of 50 μm. The average velocity of the slow cells—which are those that drill new channels and are nearly one third of all T cells—is 0.02 μm/s. If the motion would be totally persistent
Compelling evidence shows that when immune cells, including T cells, go through a constricted space, the nucleus, as the stiffest organelle in the cells, is the rate-limiting factor (44,45). Therefore, when the width of the channels in ECM is smaller than the diameter of nucleus, it would become a speed limiting factor for CTL migration in 3D matrix. If the channels are too narrow for the nucleus to pass, the T cells would appear to halt at the position until the matrix breaks loose or until they manage to squeeze themselves out. According to the experimentally determined pore size distribution in collagen matrices with various concentrations (38), one can estimate that the average pore diameter is around 7.5 μm for collagen density 2 mg/mL. The diameter distribution is broad enough to have some pores with a diameter around 9 μm, where T cells with a diameter of 10 μm can squeeze through without deforming the surrounding collagen fibers. On the other hand, in collagen concentrations 4 and 5 mg/mL, the average pore diameter is less than 5 μm with a very narrow distribution, and T cells hardly find pores large enough to squeeze through. This is likely the reason of the different
The morphology of the “channel” visible in Fig. 8 is incompatible with a randomly generated filament network (see background). The latter has of course randomly distributed regions with higher and lower filament density, but elongated cylindrical tunnels as visible in Fig. 8 with a diameter of approximately equal to the diameter of a T cell and completely void of filaments cannot occur by chance with a significant probability. The cylindrical tunnels could be produced by T cells, as observed in Video S4, as they pass through and, if some collagen fibers do not completely spring back to their original position, leaving a broadened channel behind. Another possibility is that these channels have been produced by either T cells degrading the local matrix by secretion of matrix metalloproteases (MMP) or by T cells tearing matrix apart by the exertion of mechanical forces during the 2 h before the observation, and tracking was started, leaving behind elongated, cylindrical tunnels of approximately the same diameter as T cells. Concerning the former option, it is reported that treatment of MMP inhibitors in human CD4+ T cell blasts does not affect T cell speed (46). In CD4+ T cells, MMP2 and MMP9 are expressed (47), which do not degrade collagen type I (48), which was used in our experiments. The collagen type I-degrading MMPs (MMP1, MMP8, MMP12, and MMP14) are not expressed in bead-stimulated primary human CD8+ T cells (unpublished data). Because of lack of MMPs, channel formation most probably proceeds via collagen filament deformation or destruction rather than degradation.
In summary, the aim of this study was to analyze the migration dynamics of CTLs in collagen matrices with different densities to understand potential differences in T cell migration patterns and to elucidate the role of collagen density. The investigation of the effects of the migration patterns that we reveal for the search efficiency of CTLs remains for future studies. Based on our observations, we expect that the search efficiency of T cells decreases in dense collagen matrices because even though the migration pattern per se does not differ, the velocity of CTLs decreases in dense ECM. Interestingly, T cells “dig” into the collagen network and create channels that are later used by other T cells. This is beneficial for faster movement but not necessarily for a more efficient search; channels are only there, where other CTLs have been before. When searching for scattered immobile targets, it is generally not a good strategy to follow the trail of other searchers; as long as those did not find the target, CTLs should avoid the channels because they do not lead toward the target. This is different when CTLs follow a chemotactic signal toward an area of infection; then, channels help subsequent cells to reach this area faster by faster movement. Also, when searching a mobile target, it could be beneficial to follow channels because a mobile target could have moved toward a channel after it was created. Obviously, the effect of channels on the search efficiency of CTLs in collagen matrices is complex but an interesting issue that should be addressed in future studies.
Author Contributions
Z.S., B.Q., M.H., and H.R. designed the research. R.Z. performed the experiments, tracked the trajectories, extracted the corresponding 3D coordinates, and did the analyses in Fig. 8, c, e, and f. Z.S. analyzed the experimental data and employed the analytical model. All authors contributed to the interpretation of the results. Z.S., B.Q., and H.R. wrote the manuscript, which was edited by all authors.
Acknowledgments
We acknowledge financial support from German Research Foundation through Collaborative Research Center SFB 1027 (Projects A2 and A3); M.H. received financial support from Federal Ministry of Education and Research grant 031L0133, and R.Z. received financial support from HOMFOR2018 grant.
Footnotes
Supporting Material can be found online at https://doi.org/10.1016/j.bpj.2020.10.020.
Appendix
We confine ourselves to a two-dimensional model to derive an analytical formula for the MSD of one Cartesian coordinate
with
and
In Eq. 2,
The master equation for the probability distribution
where
For large times, the term proportional to t dominates the r.h.s. (since
For ballistic motion, i.e.,
References
Articles from Biophysical Journal are provided here courtesy of The Biophysical Society
Full text links
Read article at publisher's site: https://doi.org/10.1016/j.bpj.2020.10.020
Read article for free, from open access legal sources, via Unpaywall:
http://www.cell.com/article/S0006349520308250/pdf
Citations & impact
Impact metrics
Article citations
Spatial interactions modulate tumor growth and immune infiltration.
NPJ Syst Biol Appl, 10(1):106, 30 Sep 2024
Cited by: 0 articles | PMID: 39349537 | PMCID: PMC11442770
Mechanisms of metastatic colorectal cancer.
Nat Rev Gastroenterol Hepatol, 21(9):609-625, 28 May 2024
Cited by: 3 articles | PMID: 38806657
Review
Understanding the matrix: collagen modifications in tumors and their implications for immunotherapy.
J Transl Med, 22(1):382, 24 Apr 2024
Cited by: 5 articles | PMID: 38659022 | PMCID: PMC11040975
Review Free full text in Europe PMC
A pancreatic cancer organoid-in-matrix platform shows distinct sensitivities to T cell killing.
Sci Rep, 14(1):9377, 23 Apr 2024
Cited by: 0 articles | PMID: 38654067 | PMCID: PMC11039640
Mechanical forces amplify TCR mechanotransduction in T cell activation and function.
Appl Phys Rev, 11(1):011304, 01 Mar 2024
Cited by: 1 article | PMID: 38434676
Review
Go to all (27) article citations
Data
Data behind the article
This data has been text mined from the article, or deposited into data resources.
BioStudies: supplemental material and supporting data
Similar Articles
To arrive at the top five similar articles we use a word-weighted algorithm to compare words from the Title and Abstract of each citation.
Targeting the Microtubule-Network Rescues CTL Killing Efficiency in Dense 3D Matrices.
Front Immunol, 12:729820, 17 Aug 2021
Cited by: 7 articles | PMID: 34484240 | PMCID: PMC8416057
A calcium optimum for cytotoxic T lymphocyte and natural killer cell cytotoxicity.
J Physiol, 596(14):2681-2698, 12 Mar 2018
Cited by: 44 articles | PMID: 29368348
Cytotoxic T cells swarm by homotypic chemokine signalling.
Elife, 9:e56554, 13 Oct 2020
Cited by: 38 articles | PMID: 33046212 | PMCID: PMC7669268
Professional killers: The role of extracellular vesicles in the reciprocal interactions between natural killer, CD8+ cytotoxic T-cells and tumour cells.
J Extracell Vesicles, 10(6):e12075, 01 Apr 2021
Cited by: 29 articles | PMID: 33815694 | PMCID: PMC8015281
Review Free full text in Europe PMC
Funding
Funders who supported this work.
Bundesministerium für Bildung und Forschung (1)
Grant ID: 031L0133
Deutsche Forschungsgemeinschaft (1)
Grant ID: SFB 1027