Abstract
Free full text

MITI Minimum Information guidelines for highly multiplexed tissue images
Abstract
The imminent release of tissue atlases combining multi-channel microscopy with single cell sequencing and other omics data from normal and diseased specimens creates an urgent need for data and metadata standards that guide data deposition, curation and release. We describe a Minimum Information about highly multiplexed Tissue Imaging (MITI) standard that applies best practices developed for genomics and other microscopy data to highly multiplexed tissue images and traditional histology.
Highly multiplexed tissue imaging using any of a variety of optical and mass-spectrometry based methods (Supplemental Table 1) combines deep molecular insight into the biology of single cells with spatial information traditionally acquired using histological methods, such as hematoxylin and eosin (H&E) staining and immunohistochemistry (IHC)1. As currently practiced, multiplexed tissue imaging of proteins involves 20–60 channels of 2D data, with each channel corresponding to a different antibody or colorimetric stain (Figure 1). Multiple inter-institutional and international projects, such as the Human Tumor Atlas Network (HTAN)2, the Human BioMolecular Atlas Program (HuBMAP)3, and the LifeTime Initiative4 aim to combine such highly multiplexed tissue images with single cell sequencing and other types of omics data to create publicly accessible “atlases” of normal and diseased tissues. Easy public access to primary and derived data is an explicit goal of these atlases and is expected to encompass native-resolution images, segmented single-cell data, anonymized clinical metadata and treatment history (for human specimens), genetic information (particularly for animal models), and specification of the protocols used to acquire and process the data. Given the imminent release of the first atlases, an urgent need exists for data and metadata standards consistent with emerging FAIR (Findable, Accessible, Interoperable, and Reusable) standards5. In this commentary, we establish the MITI (Minimum Information about highly multiplexed Tissue Imaging) standard and associated data level definitions; we also discuss the relationship of MITI to existing standards, practical implementations, and future developments.
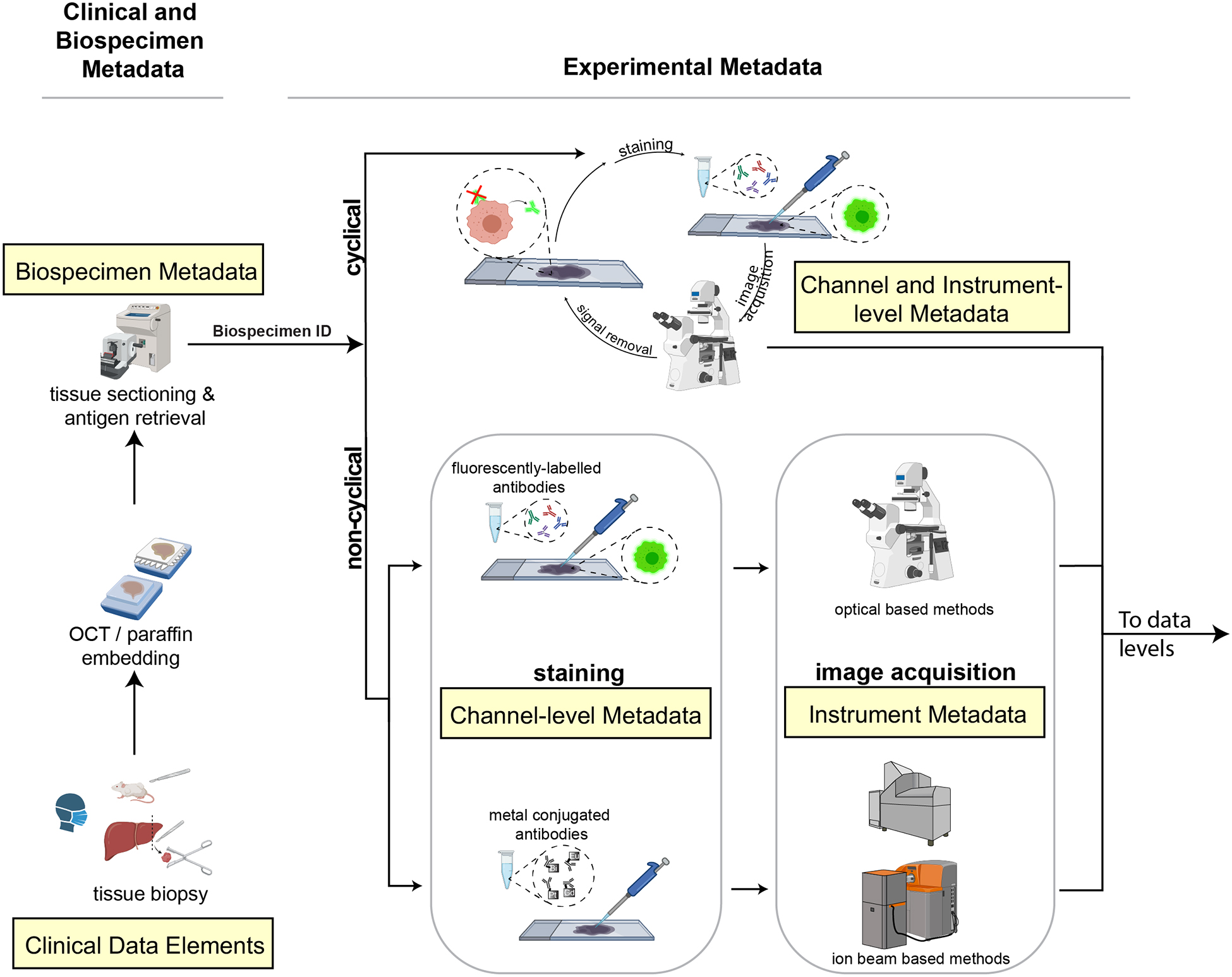
In a typical workflow, samples collected from patient biopsies and resections or from animal models are formaldehyde fixed and paraffin embedded (FFPE) or frozen and then sectioned and mounted onto either a standard glass microscope slide (for CyCIF, mIHC, IMC, MELC or mxIF), fluidic chamber (for CODEX) or specialized carriers (for MIBI). Clinical and biospecimen metadata (extracted from clinical records, for example) is linked to all other levels of metadata via a unique ID (Biospecimen ID). Data is acquired using cyclical or non-cyclical staining and imaging methods and both reagent and experimental metadata collected (consisting of antibody, reagent and instrument metadata). In both cyclic and non-cyclic methods, sections undergo pre-processing, antigen retrieval, and antibody incubation and images are acquired. In cyclical imaging methods, fluorophores or chromogens are inactivated or removed and additional antibodies and/or visualization reagents are applied and data acquisition repeated. Channel and instrument metadata capture these essential details. Created with BioRender.com.
Scope and target audiences
MITI covers biospecimen, reagent, data acquisition and data analysis metadata, as well as data levels for imaging with antibodies, aptamers, peptides, dyes and similar detection reagents (Supplemental Table 1). The standard is also compatible with images based on H&E staining, low-plex immunofluorescence (IF) and IHC. A working group is currently extending MITI to cover subcellular resolution imaging of nucleic acids using methods such as MERFISH6. While conceived with today’s two-dimensional (2D) images in mind (these typically involve 5 – 10 μm thick sections of fixed or frozen specimens), MITI accommodates three-dimensional (3D) datasets acquired using confocal, deconvolution and light sheet microscopes7. MITI has been established as its own organization with its own GitHub repository, governing structure, and procedures for proposing and incorporating revisions. The definition of MITI is available in machine readable YAML format (https://github.com/miti-consortium/MITI) along with other relevant information. MITI has also been implemented in practice (https://github.com/ncihtan/data-models) and used to structure metadata available via the HTAN data portal (https://htan-portal-nextjs.vercel.app). However, MITI is independent of HTAN or any single research consortium.
Highly multiplexed imaging is derived from methods such as IHC and IF that are in widespread use in pre-clinical research using cultured cells and model organisms, and in clinical practice with human tissue specimens. Many standards and best practices have been established for these types of data (Supplemental Table 2), but high-plex imaging presents unique challenges: images are expensive to collect and can be very large (up to 1TB in size), specimens are often difficult to acquire and may have data use restrictions, and accurate clinical and genomic annotation is a necessity. Recent interest in highly multiplexed tissue imaging has been driven by applications in oncology, largely due to the importance of the tumor microenvironment in immuno-editing and responsiveness to immunotherapy, but the approach is broadly applicable to studying normal development, infectious disease, immunology and other topics. HuBMAP3, for example, is using high-plex imaging to study a range of normal human tissues. MITI is also relevant to studies with model organisms and data tables have already been created to store data from genetically engineered mouse models (GEMMs) in a standardized manner.
Multiplexed imaging also promises to impact the pathological diagnosis of diseases, which is rapidly switching to digital approaches8. For over a century, histological analysis of anatomic specimens (from biopsies and surgical resection) has been the primary method of diagnosing diseases such as cancer9, and this remains true today, despite the impact of gene sequencing. Multiplexed tissue imaging promises to augment conventional pathological diagnosis with the detailed molecular information needed to specify use of contemporary precision therapies. This is therefore an opportune time to seek alignment of research and diagnostic approaches by establishing public standards able to take full advantage of the detailed molecular information revealed by emerging imaging methods.
Existing standards and approaches
The Human Genome Project, the Cancer Genome Atlas (TCGA)10 and similar large-scale genomic programs have developed several approaches to data management of immediate relevance to tissue atlases. The first is the concept of “minimum information” metadata, which has been employed in microarrays (the MIAME standard)11, genome sequences (MIGS)12, and biological investigation in general (MIBBI)13. The second is the idea of “data levels” (https://gdc.cancer.gov/resources-tcga-users/tcga-code-tables/data-levels), which specify the extent of data processing (raw, normalized, aggregated or region of interest, corresponding to data levels 1–4) and access control. Access control is required because even anonymized DNA sequencing data pose a re-identification risk14. As a result, the database of Genotypes and Phenotypes (dbGaP), the NCI Genome Data Commons (GDC)15, and the US Federal Register (79 FR 51345) control access to primary sequencing data (so-called level 1 & 2 sequencing data) based on policies set by a data access committee. Higher level genomic data, which are generally more consolidated, involve information aggregated form many patients, and pose little or no re-identification risk can be freely shared16 (Figure 2). When datasets are combined, they acquire the most stringent restriction applied to any constituent element. While we are not aware of any policies addressing the anonymity of histological images, consultation with our Institutional Review Boards (IRBs, ethics committees) has led us to conclude that public release of tissue images does not constitute a risk to patient privacy. MITI data levels are nonetheless consistent with the existing GDC and dbGaP practice that data intended for unrestricted distribution are classified as level 3 and up. In the case of images adhering to the MITI standard, level 3 data have been subjected to quality control and some degree of human annotation, making them more useful in a shared environment than raw images. We anticipate that IRBs and government agencies will in the future provide further guidance on sharing of datasets that combine clinical history, sequence information, and tissue images; MITI will be adapted to accommodate such guidance.
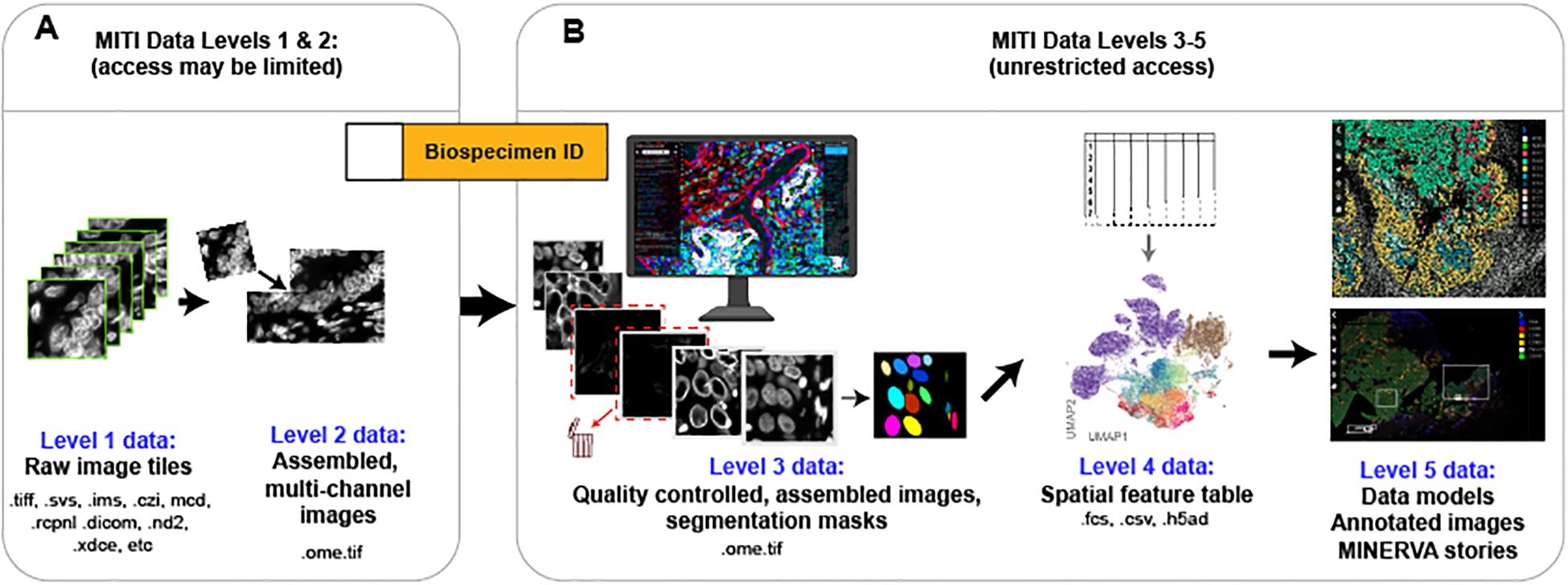
Data levels specify the extent of data processing and, in the case of sequencing data, whether access requires the approval of a data access committee. In common practice, data at levels 3 and up are freely shared. Primary data arising from microscopes and data acquisition instruments corresponds to level 1 data. Because the raw image data acquired from one slide usually consists of separate image fields, possibly from proprietary formats, they are processed to correct for uneven illumination and other instrumentation artifacts and assembled into a single multi-channel image in the OME-TIFF format (level 2 data). OME-TIFF image mosaics undergo quality control (including artefact removal, channel rejection, evaluation of staining quality) to generate full-resolution, assembled and curated level 3 image data; segmentation algorithms generate one or more label masks that also comprise level 3 data. The great majority of users will want to access these level 3 images. Each label mask (e.g., nuclei, cytoplasmic-regions, whole cells, organelles, etc.) is used to compute quantitative features, such as the mean signal intensity, spatial coordinates of individual cells and morphological features, which are stored as level 4 spatial feature tables (where rows represent single cells and columns the extracted cellular features); these data are suitable for analysis using the dimensionality reduction and visualization tools used for other types of single-cell data (e.g. UMAP plots). Spatial models computed from images and spatial feature tables, or by direct application of machine learning to images, as well as images annotated by humans, comprise level 5 data.
The MITI standard also draws extensively on image formats developed for cultured cells and model organisms and on a wide variety of open-source software tools (Supplemental Table 3). Noteworthy among these are the Open Microscopy Environment (OME) TIFF standard17 and the BioFormats18 approach to standardization of microscopy data. MITI field definitions are harmonized with the QUality Assessment and REProducibility for Instruments and Images in Light Microscopy (QUAREP-LiMi)19 effort, the Resource Identification Initiative20, and antibody standardization efforts by the Human Protein Atlas21 and are also compliant with the recently developed Recommended Metadata for Biological Images initiative22. Metadata on model organisms (particularly GEMMs - and patient derived xenografts - PDXs) are aligned with existing standards, many developed for genomic information (see Supplemental Table 2 for a full list of antecedent resources). Well-curated clinical information is essential for the interpretation of data from human specimens but standardizing such information has proven to be a major challenge in the past, for example in TCGA23. Thus, HTAN and other current NCI projects focused on human specimens are emphasizing standardization of clinical metadata, and the MITI standard is designed to closely align with the Genomic Data Commons (GDC) Data Model24 in this regard (Supplemental Tables 5–6).
All imaging methods generate data that comprises a sequence of intensity values on a raster; multi-spectral imaging simply adds new dimensions to the raster. The cameras that collect H&E and IHC images from bright-field microscopes or high-plex images from fluorescence microscopes generate a raster; ablation-based mass-spectrometry imaging (e.g. MIBI and IMC) is also raster based. As currently defined, MITI specifies that raster images should be stored in the OME-TIFF 6 standard, but OME formats are currently being migrated to a set of next generation file formats (collectively OME-NGFF)25 to improve scalability and performance on the cloud. MITI will be updated to align with these new formats as they come into general use. Another area of translational and clinical research in which imaging is commonly encountered is radiology, which is almost entirely digital, and uses data interchange standards governed by DICOM (https://www.dicomstandard.org/). DICOM has recently been extended to accommodate both radiology data and OME-TIFF standards26. The NCI’s ongoing program to create an Imaging Data Commons27 is expected to be based on this dual standard, or on a successor using OME-NGFF. MITI is, or will be, compatible with these foundational data standards.
In highly multiplexed tissue imaging antibodies are either conjugated to fluorophores directly or via oligonucleotides, or are bound to secondary antibodies (Figure 1, Supplemental Table 4). Images are then acquired serially, one to six channels at a time, to assemble data from 20–60 antibodies. In ablation-based methods, antibodies are labelled with metals and vaporized with lasers or ion beams after which they are detected by atomic mass spectrometry (Supplementary Table 4). In all cases, the raw output of data acquisition instruments comprises Level 1 MITI data (Figure 2), analogous to the Level 1 FASTq files in genomics.
Whole slide imaging is required for clinical applications28 and also necessary to ensure adequate power in pre-clinical studies29. However, resolution and field of view have a reciprocal relationship – both with respect to optical physics and the practical process of mapping image fields onto the fixed raster of a camera (or ablating beam). Whole slide images of histological specimens8 must therefore be acquired by dividing a large specimen into contiguous tiles. This usually involves acquisition of ~100 to 1,000 tiles by moving the microscope stage in both X and Y, with each tile being a multi-dimensional, subcellular resolution, TIFF image. Tiles are combined at sub-pixel accuracy into a mosaic image in a process known as stitching. When high-plex images are assembled from multiple rounds of lower-plex imaging, it is also necessary to register channels to each other across imaging cycles and to correct for any unevenness in illumination (so-called flat fielding)30. Stitched and registered mosaics can be as large as 50,000 × 50,000 pixels × 100 channels and require ~500 GB of disk space. They correspond to Level 2 MITI data and represent full-resolution primary images that have undergone automated stitching, registration, illumination correction, background subtraction, intensity normalization and have been stored in a standardized OME format. The level of processing is analogous to BAM files, a common type of Level 2 data in genomics.
Level 3 data represent images that have been processed with some interpretive intent, which may include (i) full-resolution images following quality control or artifact removal, (ii) segmentation masks computed from such images, (iii) machine-generated spatial models, and (iv) images with human or machine-generated annotations. Level 3 MITI data is roughly analogous to Level 3 mRNA expression data in genomics. However, whereas many users of genomic data only require access to processed level 3 and 4 data, which are usually quite compact, quantitative analysis of tissue images adds a requirement for full-resolution primary images so that images and computed features can be examined in parallel31. Level 3 MITI data is intended to be the primary type of image data distributed by tissue atlases and similar projects.
Assembled level 3 images are typically segmented to identify single cells31, which are quantified to produce a “spatial feature table” that describes marker intensities, cell coordinates and other single-cell features. The Level 4 data in spatial feature tables are a natural complement to count tables in single cell sequencing data (e.g. scRNA-seq, scATAC-seq, scDNA-seq) and can be analyzed using many of the same dimensionality reduction methods (e.g. PCA, t-SNE and U-MAP)32 and on-line browsers such as cellxgene (Supplemental Table 3)33. These types of tabular data are all examples of “Feature Observation Matrixes” which are themselves being standardized across domains of biology to improve their utility and inter-compatibility. Level 5 MITI data comprise results computed from spatial feature tables or primary images. Because access to TB-size full-resolution image data is impractically burdensome when reading a manuscript or browsing a large dataset, a specialized type of Level 5 image data has been developed to enable panning and zooming across images using a standard web browser. In the case of Level 5 images viewed with MINERVA software, the aim is to exploit similar functionality and concepts as those in Google Maps or electronic museum guides34. The inclusion of digital docents with images makes it possible to combine pan and zoom with guided narratives that greatly facilitate comprehension of complex datasets and promote new hypothesis generation35.
For any metadata standard to be used, a balance must be struck between ease of data entry, which minimizes non-compliance by data generators, and level of detail, which must be sufficient for data retrieval, analysis, and publication in a reproducible manner. Moreover, specifying a metadata standard is separate from the essential task of developing a practical and reliable means for capturing information needed to ensure adherence to the standard. Two approaches have proven most effective in addressing this requirement. One, exemplified by OMeta36, involves a relational database and web interface that data generators use to input necessary information in a controlled manner. Another approach, exemplified by MAGE-TAB37, involves a standardized format for collecting metadata via a series of structured documents, which are then used to populate web pages and databases38. As a practical test of MITI we have implemented the latter approach in a JSON schema (https://github.com/ncihtan/data-models) that also conforms to the design principles of SCHEMA.org. These principles focus on the creation, maintenance and promotion of schemas for structured data that is supported by major web search engines, thereby enhancing discoverability. In this TAB-like approach the MITI standard is exposed to data collectors as Google Sheets with dropdowns representing controlled vocabularies and highlighting required or optional elements; many fields are automatically validated upon entry. These documents are ingested using SCHEMATIC (Schema Engine for Manifest Ingress and Curation; https://github.com/Sage-Bionetworks/schematic), automatically linked to primary imaging data, and stored as cloud assets. These implementations continue to evolve, and entirely different approaches are possible: nothing in a MITI-type standard constrains how data are collected.
Whereas many research agencies and countries have made a major investment in curating, storing, and distributing genomic data, fewer repositories exist for primary image data. The Image Data Resource39 maintained by the European Bioinformatics Institute (EBI) is an exception, but as the volume of image data grows, other means of data distribution will almost certainly be required. In the U.S., in the absence of a major public investment in data storage, the development of “requester pays”40 access to datasets is a promising development. The primary cost associated with creation and maintenance of a dataset on a commercial cloud service involves data download, not data ingress and storage. In a “requester pays” model, a user seeking access to a dataset pays the cost of data egress directly to the cloud provider making access both secure and anonymous (moreover, the cost of egress into another account on the same commercial cloud is low). Although the “requester pays” approach might appear to create an impediment to research, the actual cost of egress is quite low (currently, about hundred US dollars per TB) compared to any form of data acquisition and a key goal is to avoid a tragedy of the commons in which frequent, duplicate downloads overwhelm the system. A combination of a MITI implementation on a cloud service (as described above) with “requester pays” cloud access will also make it possible for individuals to distribute very large FAIR image datasets at relatively low cost. Such an approach does not obviate the need for public investments, such as those being made but EBI, but does represent a practical way forward to democratize release of standardized data – some of which can then be incorporated into publicly supported resources. Regardless, the MITI standard described here is available for immediate use, without being impacted by how access to the primary data is provisioned.
Public data and metadata standards have been essential for the success of genomics and other fields of biomedicine, but the creation of a new standard is no guarantee of successful adoption. An outpouring of effort 10–20 years ago led to the development of widely adopted and well maintained standards such as MIAME11, MIGS12 and MIBBI13, and these have been consolidated and further documented by the Digital Curation Center (https://www.dcc.ac.uk/), FairSharing.org, and similar projects. However many other minimum information projects have been left unattended41, and it remains unclear whether existing metadata adequately conform to user needs42. The development of MITI and of the initial HTAN implementation enjoys NCI support and is expected to become part of the NCI Cancer Research Data Commons27, helping ensure its viability. However, individuals and organizations are invited to join in the further development of MITI and should make contact via the image.sc forum or submit pull requests (i.e. requests for inclusion in the MITI “code base” at https://github.com/miti-consortium/MITI). Because high high-plex tissue imaging is in its infancy and MITI has attracted the great majority of developers of existing high-plex tissue image acquisition methods, it represents a solid beginning for what will need to be an evolving standard. By having its own repository and governance structure, independent of any particular research program or constituency, MITI also conforms with other requirements of successful open standards43.
Acknowledgements
This work is supported by the HTAN Consortium and the Cancer Systems Biology Consortium (CSBC). A list of all current Consortium members can be found at https://humantumoratlas.org/.
This work was supported by the following grants from the National Cancer Institute under the Human Tumor Atlas Network (HTAN) U2C CA233262 (Harvard Medical School), U2C CA233280 (OHSU), U2C CA233195 (Boston DFCI Broad), U2C CA233291 (Vanderbilt University Medical Center), U2C CA233311 (Stanford University), U2C CA233238 (Boston University Medical Campus), U2C CA233285 (Children’s Hospital of Philadelphia), U2C CA233303 (Washington University St. Louis), U2C CA233280 (Oregon Health and Science University), U2C CA233284 (Memorial Sloan Kettering Cancer Center), U2C CA233254 (Duke University Medical Center) and by other public support including U54 CA225088 (SS, PKS) and U24 CA233243 (Dana-Farber Cancer Institute, Emory University, Institute for Systems Biology, Memorial Sloan Kettering Cancer Center, Sage Bionetworks). DS was funded by an Early Postdoc Mobility fellowship (no. P2ZHP3_181475) from the Swiss National Science Foundation and was a Damon Runyon Fellow supported by the Damon Runyon Cancer Research Foundation (DRQ-03-20); DS is currently supported by the BMBF (01ZZ2004). NG was funded by the NIH Human BioMolecular Atlas Program (HuBMAP) OT2 OD026677 and MDH by NCI/NIH Task Order No. HHSN26110071 under Contract No. HHSN2612015000031.
Competing Interests Statement
PKS is a member of the SAB or BOD of Applied Biomath, RareCyte Inc., and Glencoe Software, which distributes a commercial version of the OMERO data management platform; PKS is also a member of the NanoString SAB and a consultant to Merck and Montai Health. In the last five years the Sorger lab has received research funding from Novartis and Merck. Sorger declares that none of these relationships have influenced the content of this manuscript. SS is a consultant for RareCyte Inc. NG is a co-founder and equity owner of Datavisyn. DS is a consultant for Roche Glycart AG. JRS is Founder and CEO of Glencoe Software, which distributes a commercial version of the OMERO data management platform. SR receives research funding from Bristol-Myers-Squibb, Merck, Affimed, and Kite/Gilead. SR is on the Scientific Advisory Board for Immunitas Therapeutics. DSu is employed by Quantitative Imaging Systems LLC. EAB is an employee of Indica Labs.
Data and Code Availability Statement
The detailed specification of the guidelines outlined in this manuscript are available at https://github.com/miti-consortium/MITI and https://www.miti-consortium.org/
References
Full text links
Read article at publisher's site: https://doi.org/10.1038/s41592-022-01415-4
Read article for free, from open access legal sources, via Unpaywall:
https://www.nature.com/articles/s41592-022-01415-4.pdf
Citations & impact
Impact metrics
Citations of article over time
Alternative metrics

Discover the attention surrounding your research
https://www.altmetric.com/details/124481641
Article citations
Quality control for single-cell analysis of high-plex tissue profiles using CyLinter.
Nat Methods, 30 Oct 2024
Cited by: 0 articles | PMID: 39478175
Consensus tissue domain detection in spatial omics data using multiplex image labeling with regional morphology (MILWRM).
Commun Biol, 7(1):1295, 30 Oct 2024
Cited by: 0 articles | PMID: 39478141 | PMCID: PMC11525554
Making the most of bioimaging data through interdisciplinary interactions.
J Cell Sci, 137(20):jcs262139, 23 Oct 2024
Cited by: 0 articles | PMID: 39440474 | PMCID: PMC11529881
Review Free full text in Europe PMC
Spatial analysis by current multiplexed imaging technologies for the molecular characterisation of cancer tissues.
Br J Cancer, 22 Oct 2024
Cited by: 0 articles | PMID: 39438630
Review
Vitessce: integrative visualization of multimodal and spatially resolved single-cell data.
Nat Methods, 27 Sep 2024
Cited by: 8 articles | PMID: 39333268
Go to all (26) article citations
Similar Articles
To arrive at the top five similar articles we use a word-weighted algorithm to compare words from the Title and Abstract of each citation.
Transition of a Text-Based Insulin Titration Program From a Randomized Controlled Trial Into Real-World Settings: Implementation Study.
J Med Internet Res, 20(3):e93, 19 Mar 2018
Cited by: 12 articles | PMID: 29555621 | PMCID: PMC5881039
Barriers and Facilitators to the Implementation of a Mobile Insulin Titration Intervention for Patients With Uncontrolled Diabetes: A Qualitative Analysis.
JMIR Mhealth Uhealth, 7(7):e13906, 31 Jul 2019
Cited by: 9 articles | PMID: 31368439 | PMCID: PMC6693299
The Drosophila miti-mere gene, a member of the POU family, is required for the specification of the RP2/sibling lineage during neurogenesis.
Development, 120(6):1483-1501, 01 Jun 1994
Cited by: 25 articles | PMID: 8050358
Multiplexed Epitope-Based Tissue Imaging for Discovery and Healthcare Applications.
Cell Syst, 2(4):225-238, 27 Apr 2016
Cited by: 115 articles | PMID: 27135535
Review
Funding
Funders who supported this work.
Bundesministerium für Bildung und Forschung (1)
Grant ID: 01ZZ2004
Damon Runyon Cancer Research Foundation (1)
Grant ID: DRQ-03-20
NCI NIH HHS (24)
Grant ID: K08 CA230213
Grant ID: U24 CA233243
Grant ID: U2C CA233254
Grant ID: U2C CA233311
Grant ID: P30 CA008748
Grant ID: U54 CA225088
Grant ID: HHSN261201500001W
Grant ID: R37 CA266185
Grant ID: U2C CA233291
Grant ID: U2C CA233303
Grant ID: HHSN261201500001G
Grant ID: R01 CA245499
Grant ID: HHSN261201500001C
Grant ID: HHSN261201500003C
Grant ID: U2C CA233238
Grant ID: HHSN261201000031C
Grant ID: R35 CA197570
Grant ID: U2C CA233280
Grant ID: K12 CA184746
Grant ID: U2C CA233195
Grant ID: HHSN261201500003I
Grant ID: U2C CA233262
Grant ID: U2C CA233284
Grant ID: U2C CA233285
NHGRI NIH HHS (1)
Grant ID: T32 HG000044
NIH HHS (1)
Grant ID: OT2 OD026677
Swiss National Science Foundation (1)
Grant ID: P2ZHP3_181475
U.S. Department of Health & Human Services | NIH | National Cancer Institute (10)
Grant ID: U2C CA233303
Grant ID: 1U24CA233243-01
Grant ID: CSBC U54 CA225088
Grant ID: U2C CA233311
Grant ID: U2C CA233195
Grant ID: U2C CA233284
Grant ID: U2C CA233238
Grant ID: U2C CA233262
Grant ID: U2C CA233291
Grant ID: U2C CA233280
U.S. Department of Health & Human Services | National Institutes of Health (1)
Grant ID: OT2 OD026677