Abstract
Free full text

Substitution mutational signatures in whole-genome-sequenced cancers in the UK population*
Associated Data
Abstract
Whole-genome sequencing (WGS) permits comprehensive cancer genome analyses, revealing mutational signatures, imprints of DNA damage and repair processes that have arisen in each patient’s cancer. We performed mutational signature analyses on 12,222 WGS tumor-normal matched pairs, from patients recruited via the UK National Health Service. We contrasted our results to two independent cancer WGS datasets, the International Cancer Genome Consortium (ICGC) and Hartwig Foundation, involving 18,640 WGS cancers in total. Our analyses add 40 single and 18 double substitution signatures to the current mutational signature tally. Critically, we show for each organ, that cancers have a limited number of ‘common’ signatures and a long tail of ‘rare’ signatures. We provide a practical solution for utilizing this concept of common versus rare signatures in future analyses.
Introduction
The global cancer burden was estimated at 19.3 million new cases and 10.0 million deaths in 2020 (1). Worldwide, cancer is the first or second leading cause of mortality before the age of 70 (1). The genome of a cancer is a highly distorted entity that has acquired thousands of genetic aberrations since conception (2). If examined comprehensively, cancer genomes can thus reveal insights regarding carcinogenesis (2).
Today, modern sequencing technologies have augmented the scale and rapidity of genome re-sequencing (3), permitting whole-genome sequencing (WGS) approaches that provide an all-inclusive perspective on cancer genomes (4). Beyond the handful of causative ‘driver’ mutations, WGS allows exploration of the full landscape of ‘passenger’ mutations that describe the processes that have arisen during tumorigenesis, resulting in patterns termed ‘mutational signatures’ (5–7). While drivers become important targets for therapeutic intervention, mutational signatures provide clues regarding historical environmental exposures and highlight potentially targetable pathway defects (4, 6, 8, 9).
Substantial efforts by The Cancer Genome Atlas (TCGA) (10), the International Cancer Genome Consortium (ICGC) (9, 11), and the Hartwig Medical Foundation (HMF) (12) have helped advance cancer genomics considerably in recent years. However, an endeavor to generate whole cancer genomes from national public health cancer services would be a welcome demonstration of how cancer genomic data can be derived from patients in real-time and ultimately benefit patients and the scientific community.
Here, we examined a new cohort of 15,838 WGS cancers from patients recruited from all 13 National Health Service (NHS) Genomic Medicine Centres across England as part of the Genomics England (GEL) 100,000 Genomes Project (100kGP) (7, 13) [GEL v8 data release]. We report the analysis of mutational signatures and highlight a conceptual advance that come from being able to examine this substantial WGS collection. We add 40 single base substitution (SBS) mutational signatures and 18 double base substitution (DBS) mutational signatures to the current tally. We compare these additional signatures to known etiologies and end by suggesting principles of how to meaningfully utilize mutational signatures in future analyses.
Results
The GEL cohort
All 15,838 tumor-normal sample pairs were taken through 100 kGP bioinformatic somatic-variant analysis pipelines. We restricted our analysis to high-quality data derived from flash frozen material, involving 12,222 GEL tumor samples from 11,585 individuals (several participants had synchronous or metachronous tumors). For this evaluation, the final dataset included 298,694,545 substitutions, 2,675,617 double substitutions, 154,675,475 indels, and 1,958,105 rearrangements (Fig. 1, A and B, tables S1 and S2) of 19 tumor types (skin, lung, stomach, colorectal, bladder, liver, uterus, ovary, biliary, kidney, pancreas, breast, prostate, bone/soft-tissue, central nervous system (CNS), lymphoid, oropharyngeal, neuroendocrine tumors (NET), and myeloid).
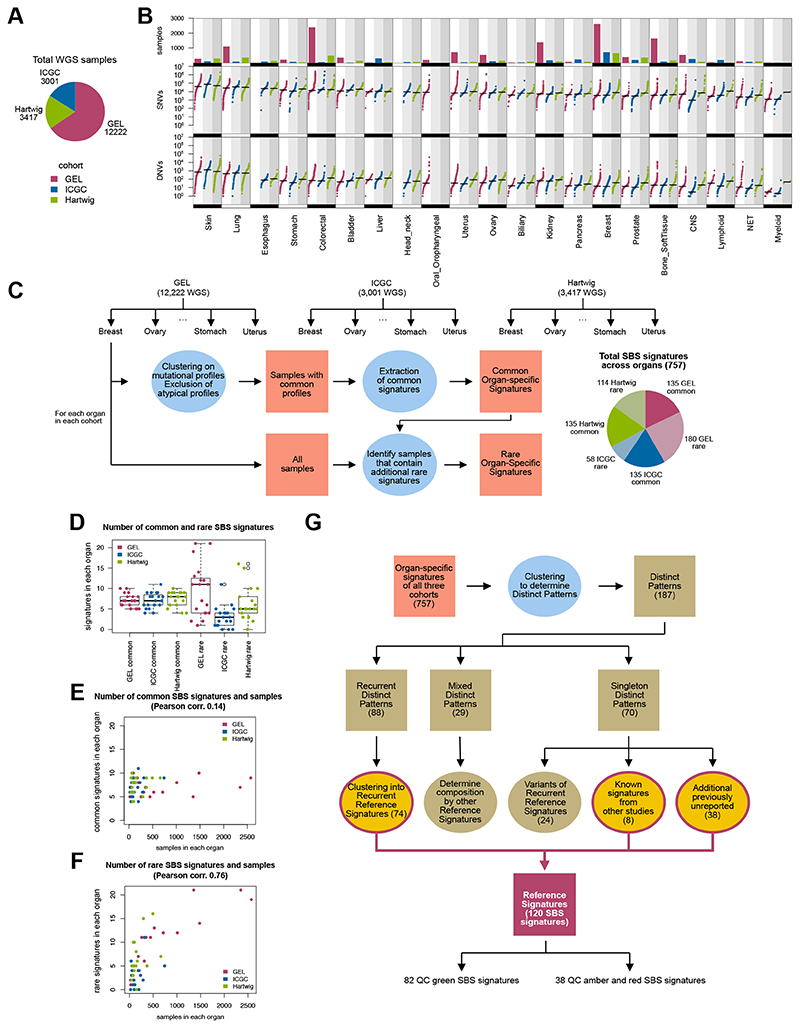
(A) WGS cases included in analyses. (B) Number of samples and mutational burden of somatic single nucleotide variants (SNVs) and double nucleotide variants (DNVs) across 21 tumor-types that have been WGS’d by GEL, ICGC and Hartwig. Not all tumor-types are represented in all three cohorts (for example, esophagus, head andneck, oropharyngeal). CNS = central nervous system; NET = neuroendocrine tumors. (C) Schematic representation of the workflow of mutational signature analysis. Three cohorts (GEL, ICGC and Hartwig) were evaluated independently. For each organ in each cohort, mutational catalogs were clustered, where samples with atypical catalogs were excluded from the extraction process. Samples with similar catalogs were subjected to signature extraction to obtain a set of common organ-specific signatures. These common signatures were fitted into all samples, highlighting samples that had a high error profile that were subsequently used to identify rare signatures. Pie chart shows the total number of SBS signatures identified for each independent extraction of each organ in all three cohorts. (D) Number of common and rare SBS signatures in each cohort. (E) Common SBS signatures as a function of number of samples analyzed. (F) Rare SBS signatures as a function of number of samples analyzed. (G) Procedure to determine the Reference Signatures from all the cohort-organ signatures identified. Numbers refer to the SBS signatures analysis. For details, see Materials and Methods.
Common and rare mutational signatures
The national GEL sequencing endeavor delivers thousands of samples for certain tumor-types (1,009 lung, 1,355 kidney, 2,572 breast, and 1,480 bone/soft tissue cancers), an order of magnitude (or two) greater than previous WGS efforts for some organs. This permits robust detection of signatures that are rare – those occurring in 1% of the tumors or fewer. Furthermore, already-sequenced WGS cohorts such as ~3,000 primary cancers from ICGC and ~3,400 metastatic from HMF, provide a powerful means of validating findings.
We performed mutational signature extractions confined to specific tumor-types using an updated signature extraction method (Fig. 1C, fig. S1, tables S3 to S6, Materials and Methods). Briefly, for each tumor type, we clustered mutational catalogs (counts of SBS in 96-element form or DBS in 78-element form), selecting only samples with recurrent, commonly occurring profiles to perform signature extraction (fig. S1, A to C). Cases with unusual profiles and likely to have rare signatures were excluded in the first extraction. Thus, this yielded a set of highly accurate ‘common signatures’ that are prevalent for that tumor type. Next, by fitting these common signatures into all samples, cases that are likely to have additional patterns not fully explained by common signatures alone would report a high ‘error’ (or discrepancy between true sample catalog and reconstructed catalog) (fig. S1D). Potential additional signatures were then extracted from these samples to obtain a set of ‘rare signatures’ (fig. S1, E to H, Materials and Methods). Accordingly, we obtained a set of common signatures and a set of rare signatures for each tumor-type. In all, for SBS, we identified 135 common signatures and 180 rare signatures in 19 tumor types within the GEL cancer cohort.
To validate these common and rare signatures, we performed signature extractions in independent cohorts of 3,001 ICGC primary WGS cancers (19 tumor-types) and 3,417 metastatic Hartwig WGS samples (18 tumor-types). We identified 135 common signatures in ICGC, 58 rare. In Hartwig, we found 135 common signatures and 114 rare (tables S7 to S10). We performed an agnostic three-way signature comparison in 16 tissue types that were present in all three cohorts (fig. S2). We found that signatures from the same organ in different cohorts were more similar to each other than to those in other tissue type, providing reassuring evidence that mutational signatures in each organ are highly reproducible, have tissue-specificities, and were detectable regardless of sequencing platform or mutation-calling algorithms (fig. S2).
Notably, the number of common signatures in each organ is usually limited (between five and ten for SBS) and is independent of the number of samples analyzed per organ (Fig. 1, D and E, fig. S3, A and B, tables S11 and S12). By contrast, the number of rare signatures varies and is highly correlated with the number of samples analyzed (Fig. 1F and fig. S3C). This illuminates why ubiquitous, organ-specific signatures are detectable even with limited numbers of whole genomes, whereas sporadic, rare signatures are more likely to be detected with increased sample size.
Reference mutational signatures
Biologically, the same mutational processes could underpin signatures extracted from different tumor-types. Thus, we considered all common and rare GEL, ICGC, and Hartwig tissue-specific signatures together, involving 18,640 WGS cancer samples (Fig. 1G, Materials and Methods) and performed a clustering analysis to derive a set of ‘Reference Signatures’. First, we identified clusters of highly similar patterns that we termed ‘distinct patterns’ (tables S13 to S16). Each distinct pattern could be either: i) a true signature, thus observable in independent extractions of diverse organs and cohorts (recurrent pattern); ii) a mix of other signatures (mixed pattern); iii) a pattern seen in only one extraction (singleton pattern) (Fig. 1G, tables S17 and S18). Next, we determined a minimal set of ‘Reference Signatures’, which were classified as quality control (QC) green, amber or red, where green implied high-confidence signatures observed in multiple independent extractions and amber/red signatures were observed only once or were possible mathematical artefacts (Fig. 1G, tables S19 to S22). In all, we identified 82 SBS and 27 DBS high-quality signatures (figs. S4 to S6). Henceforth, we will only discuss these high-quality QC green signatures, although all signatures are available for reference in supplementary material (tables S19 and S20).
Reference Signatures were compared and matched with COSMIC mutational signatures (14), confirming 42 previously described COSMIC SBS signatures and 9 COSMIC DBS signatures (Fig. 2, A and B, fig. S3, D to G, fig. S4, fig. S6A and table S19). We found 40 previously unreported high-confidence SBS signatures and 18 previously unreported DBS signatures in this analysis (fig. S5 and fig. S6B). Respecting prior nomenclature (14), these SBS signatures have been numbered from 95 onwards, and DBS signatures from 12 onwards (table S19). Note that COSMIC and/or Reference Signatures are a simplified means of discussing signatures that are mutually present across tissues. However, they are purely mathematical constructs - an averaged result across different organs - thus organ-specific signatures are more likely to be accurate biological representations of the mutational processes that occur within a tissue. We also provide the numbers of mutations associated with each reference signature per sample (tables S23 and S24), and matrices to map each reference signature to organ-specific signatures (tables S25 and S26), for SBS and DBS respectively.
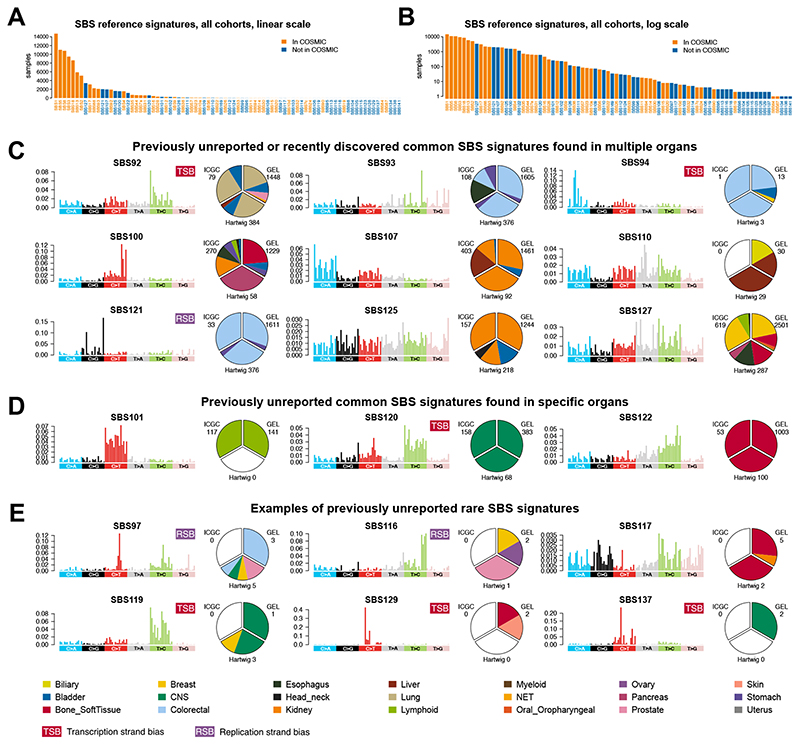
(A) Frequency of SBS signatures in the present study. Orange bars highlight 42 signatures reported in this study and present in COSMIC v3.2. Blue bars highlight 40 previously unreported signatures found in the present analysis. (B) Same information as A with log scale on y-axis. (C) Four previously unreported (SBS107, SBS100, SBS110 and SBS121) and five recently reported (SBS92, SBS93, SBS94,SBS125, and SBS127) common SBS signatures found in many organ systems. (D) Previously unreported common SBS signatures found in single organs only. (E) A few examples of previously unreported rare signatures. TSB, transcriptional strand bias; RSB, replication strand bias. SBS signatures can be browsed here: https://signal.mutationalsignatures.com/explore/study/6?mutationType=1.
Previously unreported mutational signatures
Single base substitution (SBS) signatures
We note four previously unreported and five recently reported signatures (15,16,17), that are common, recurring in many samples of multiple tumor-types in all three cohorts (GEL, ICGC, and Hartwig), detectable because of the scale of this analysis (Fig. 2C). Among the previously unreported signatures, SBS107 is dominated by C>A variants and reported consistently in kidney and bladder cancers, suggestive of an organ-specific process. SBS100 bears similarities to the APOBEC signature SBS2; however, it presents a taller TCC>TTC peak and additional context-independent C>T mutations. SBS110 has the tallest T>A peak at CTG>CAG, with contributions from T>C at ATA and ATG. The preponderance in the liver/biliary tract would suggest a compound that is likely cleared through the hepatobiliary system. SBS121 is characterized by C>G variants mostly at ACT and TCT contexts, shows replication strand bias and is mostly found in colorectal and stomach cancer. We also confirm the recently reported SBS92 (15), SBS93, SBS94 (16), SBS125, and SBS127 (RefSig N12 and N1 respectively (17)).
Three signatures occurred frequently in specific tumor-types (Fig. 2D): SBS120 dominated by T>C mutations at ATN and a distinctive peak of C>T at GCG, seen in 75% of CNS cancers; SBS122 characterized by T>C mutations in general but primarily TTN, in 67% of sarcomas; and SBS101 defined by C>T variants, in 68% of lymphoid cancers.
Thirty-one additional rare previously unreported signatures of high-confidence were present in ~1% or fewer samples (Fig. 2E). We discuss several in detail in relevant sections below, and for brevity, tabulate the majority in table S19. Associated information such as transcriptional and replication strand asymmetries are included there. All mutational signatures data can also be browsed at our website, Signal: https://signal.mutationalsignatures.com/explore/study/6.
Double (DBS) and triple (TBS) base substitution signatures
We adopted similar principles to identify 39 DBSs, including 27 high-confidence ones (Materials and Methods, table S20 and fig. S6). We performed three additional evaluations. First, we curated dinucleotides for each DBS signature in the GEL dataset to check that they were in cis. Second, for a DBS signature that was correlated with an SBS signature, an in-silico analysis assessing whether the DBS pattern could be expected given the SBS pattern was performed (Materials and Methods). Third, we investigated up to 10 nt of mutational context of relevant dinucleotides for each DBS signature. These assessments were critical in refuting several DBS signatures as being simply due to chance, described below.
Of eleven previously described COSMIC DBS signatures (14), we identified nine and were unable to extract DBS6 or DBS9 (Fig. 3A, fig. S3, F and G, and fig. S6A). Of our 27 high-confidence DBS signatures, 17 had bona fide dinucleotides in cis. We confirmed previously reported signatures and their associated etiologies: DBS1 (UV light), DBS2 (smoking), DBS5 (platinum therapy), and DBS11, associated with APOBEC, here verified as APOBEC-induced given the 10 nt sequence context analysis showing a TpCC preponderance (Fig. 3B). DBS7 was previously reported as associated with MMR defects (14), while we find associations with SBS17 instead (fig. S7A). DBS8, mostly in colorectal cancer, showed dinucleotide variants often preceded by a Cytosine and followed by an Adenine (fig. S7, B and C).
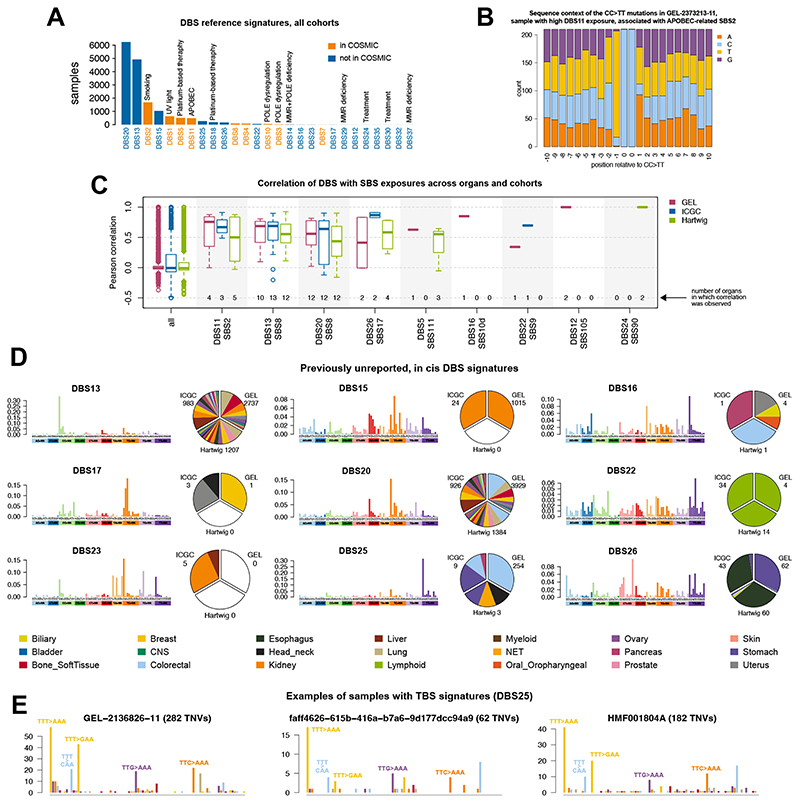
(A) Frequency of DBS signatures in the present study. (B) Flanking sequence context surrounding mutated dinucleotides of DBS11, which is correlated with APOBEC-related SBS2, to demonstrate a preference for TpCCpN context similar to the TpCpN sequence predilection of APOBECs. (C) Correlation of DBS with SBS exposures across cohorts. Numbers in each column report the number of organs implicated in the correlative analyses. A correlation is computed independently for each organ and the correlations are displayed as a boxplot. Boxplots denote median (horizontal line) and 25th to 75th percentiles (boxes). The lower and upper whiskers extend 1.5×IQR (IQR: inter-quartile range). (D) Examples of previously unreported DBS signatures. (E) Samples with TBS1. The total number of samples and total number of TNVs are too low to perform a formalmutational signature analysis. All DBS signatures identified in the present study can be browsed here: https://signal.mutationalsignatures.com/explore/study/6?mutationType=2.
We confirm DBS5 and DBS18 are associated with prior platinum exposure (18). Mutational context analysis indicates that these are distinct signatures: DBS5 has the tallest peak of CT>AA mutations without preference in flanking sequences, while DBS18 has the tallest peak of CT>AC mutations, where the dinucleotide is always preceded by a Cytosine (fig. S7D). Both signatures have a TG>GT peak most frequently followed by a Guanine (fig. S7, E and F).
DBS13 and DBS20 were low-burden signatures that appear to correlate with each other and SBS8 (Fig. 3, C and D). DBS16 was associated with SBS10d (Fig. 3C), a hypermutator signature recently reported as due to polymerase δ (POLD) dysfunction (19). DBS22 is not associated with very prominent peaks (highest probabilities only 7%). However, it appears to be correlated with SBS9 and is only seen in lymphoid cancers (Fig. 3, C and D). DBS26 is similar to DBS7 and correlates with SBS17 in esophageal and stomach cancers (Fig. 3, C and D). DBS30 was observed in one lymphoid cancer sample and may be related to treatment (fig. S6B).
DBS25 is characterized by an excess of TT>AA that, on inspection, reveals a triple base substitution signature (TBS). Exploring all triple base possibilities, we obtain an 864-channel profile that systematically reports an excess of TTT>AAA followed by TTT>GAA, TTT>CAA, and minor contributions of TTG>AAA, and TTC>AAA. We propose that this is called TBS1 (Fig. 3E and table S27). However, the number of mutations and implicated samples are too low to perform a formal mutational signature analysis.
Our curation steps uncovered several DBS signatures, including previously reported ones, that comprise adjacent substitutions that are not in cis and are simply the mathematical outcome of an associated SBS hypermutator (fig. S8). For example, DBS3 and DBS10 were similar and correlated with polymerase ε (POLE)-attributed SBS10a (fig. S8, A to C). In silico analysis showed that a DBS pattern that recapitulates DBS3/DBS10 could be reproduced from hypermutated samples of SBS10a (fig. S8D). The alleged double substitutions were not, in fact, in cis. Similarly, DBS12 (associated with SBS105), DBS14 (associated with SBS14), DBS29 (associated with SBS20), and DBS37 (associated with SBS26) could all be generated mathematically from their associated SBS signatures (fig. S8, E to H), indicating that these were not true dinucleotides, but simply single nucleotide variants occurring next to each other by chance. One exception, DBS24 – associated with SBS90, attributed to duocarmycin exposure – has a pattern that can be mostly recapitulated by simulation of SBS90, apart from the CT>AA component (fig. S8I). Three signatures were not in the GEL cohort and could not be curated (DBS23, DBS32, DBS35) due to lack of access to sequencing data.
Contrasting previously unreported signatures with previously reported endogenous processes
Deamination and amplified deamination
Pervasive patterns of deamination are widely observed in malignant and non-malignant tissues. SBS1 characterized by C>T mutations at CpG is due to deamination of methyl-cytosine, while SBS2 and SBS13 are due to APOBEC-related deamination. Both are likely physiological: SBS1 occurs by natural hydrolytic processes, while SBS2 and SBS13 arise through transient single-stranded DNA availability (20).
Two rare signatures also characterized by C>T transitions at CpG are SBS96 and SBS95, differing by their ability to demonstrate marked hypermutator phenotypes and relative C>T peak heights (Fig. 4, A and B). SBS96, present in 18 of 12,222 GEL samples (0.15%, table S23, reported as due to inherited and/or acquired mutations in MBD4 (21), has C>T at ACG as its tallest peak. We identified germline truncating MBD4 mutations with loss of heterozygosity (LOH) of the alternative allele to explain 12 of 18 samples (6/10 patients) with SBS96 (table S28 and Fig. 4C). MBD4 germline variants were also seen in 35 other GEL patients, yet SBS96 was not observed in their tumors because the wild-type parental allele was intact in all assessable cases. We note that SBS96 was observed in extremely rare cancers such as myxofibrosarcomas and uveal melanoma. SBS95 is distinguishable from SBS96 by having its tallest peak at CCG and by exhibiting transcriptional strand bias. SBS95 occurred in a lymphoid and a stomach cancer in GEL and one head and neck cancer in the ICGC cohort (table S23). None had MBD4 mutations. The cause for SBS95 remains unclear.

(A) Five signatures characterized by substitutions at NCG nucleotides are contrasted to each other. SBS profiles are shown, along with prevalence of signatures across three cohorts (GEL, ICGC and Hartwig), transcriptional strand bias (TSB) and replication strand bias (RSB). (B) Mutationburdens associated with each signature in all tumor-types. SBS1 is common and seen in all cohorts in many tumor-types. SBS95, SBS96, SBS87, and SBS105 are rare and are associated with a higher mutation burden when compared to SBS1. Y-axis shows mutation count on log-scale. Summaries comparing signatures in etiological groupings can be found here: https://signal.mutationalsignatures.com/explore/study/6. (C) SBS96 signature and prevalence among samples in the three cohorts. The Venn diagram illustrates the number of patients in GEL with SBS96 and biallelic loss ofMBD4. (D) SBS108 signature and prevalence among samples in the three cohorts. The Venn diagram illustrates the number of patients in GEL with SBS108 and biallelic OGG1 G308E. (E) SBS30 signature and prevalence among samples in the three cohorts. The Venn diagram illustrates the number of patients in GEL with SBS30 and biallelic loss of NTHL1. Only samples with SBS30 were inspected for NTHL1 mutations.(F) SBSs associated with MMR and POLE/POLD gene defects. (G) Proportion of samples across GEL organs with MMR or POLE/POLD defects related signatures or with high HRDetect score. (H) Proportion of samples with MMR biallelic loss and/or POLE/POLD dysregulation in each group of samples presenting an SBS mutational signature in (F). (I) Proportion of HRDetect-high samples with biallelic loss in genes linked to homologous recombination repair, number of samples in parenthesis.
Two signatures were characterized by C>N at CpG (Fig. 4A). SBS87 (22), with its tallest peak at CCG, was observed in one breast cancer. A related signature with C>N at all CpGs, SBS105, was reported in one breast and one bladder cancer in GEL. Although we have not found a cause for SBS105, it is associated with DBS12, a mathematical outcome of a high rate of SBS105 (fig. S8E), and does not exhibit transcriptional strand bias. Mechanistically, SBS105 would require deamination at CpGs followed by generic misincorporation during DNA replication and/or repair, not limited to the A-rule (23), to generate this pattern.
Despite all occurring at CpGs, these signatures have distinguishing characteristics. Discriminating MBD4-related SBS96 is particularly important given reports that such tumors have sensitivities to checkpoint therapies (24).
DNA repair deficiency phenomena
A multitude of DNA repair genes and proteins serve as guardians of the genome (25). If compromised, they can result in mutational patterns in human cells.
Compromised components of base excision repair (BER)
SBS18 was previously described in neuroblastomas and adrenocortical cancers (5, 26). Subsequently, a hypermutated version of a signature similar to SBS18 was described in tumors from patients with biallelic mutations in MUTYH, a gene encoding a BER protein (MUTYH glycosylase) that corrects oxidative damage (27). Recently, it was demonstrated that OGG1 (8-oxo-guanine glycosylase) loss produces a phenocopy of SBS18 and that signatures defined by tall peaks at C>A at GCA, ACA, GCT, and TCT are due to an excess of 8-oxo-dG (25). Signature SBS108 resembles SBS18 and could be due to 8-oxo-dG (25) though has differences, including the tallest C>A peak at GCA instead of TCT (Fig. 4D). Intriguingly, three GEL patients having tumors with SBS108 all carried a germline polymorphism in OGG1 (rs113561019 p.G308E) that has been reported as a risk allele in microsatellite-stable hereditary nonpolyposis colorectal cancer (MSS-HNPCC) (28). We assessed the background frequency of this allele and found it present in 98 individuals (0.85%, table S28). Fifteen patients had tumors estimated as homozygous for the rs113561019 allele, including the three with SBS108 and 12 additional samples. It is possible that the presence of other strong signatures encumbered SBS108 detection in these cases.
Seven samples from six patients carried SBS30 associated with variants in NTHL1, another BER glycosylase (Fig. 4E, tables S29 and S30). Two cases had germline nonsense NTHL1 mutations with associated loss of the wild-type parental allele. Three cases had somatic rearrangements deleting large sections of the gene. One of the three, GEL-2126555-11, an ovarian cancer, had a mixed phenotype of SBS30 and features of BRCA2 loss and carried a germline BRCA2 frameshift mutation which creates deletion signatures. This case also had two somatic deletions affecting NTHL1.
Mismatch repair and polymerase abnormalities
Replication of the nuclear genome occurs with high fidelity because of post-replicative mismatch repair (MMR) activity and base selectivity and proofreading capacity of DNA polymerases, particularly POLE and POLD. Unsurprisingly, MMR pathway defects and selected mutations in polymerases cause high rates of mutagenesis.
We confirm four MMR deficiency (MMRd) signatures reported previously, including SBS6, SBS15, SBS26, and SBS44 (Fig. 4F,G). As noted previously ((5, 9, 14), we find a particular enrichment of mutations in MMR genes (MLH1, MSH2, and MSH6) in SBS6, SBS15, and SBS44, many of which exhibit loss of the alternative parental allele as well (Figure 4H and tables S29 and S30). In SBS26, previously shown to be identical to signatures of human knockouts of PMS2 (25), we indeed identified 14 PMS2 inactivating mutations (ten germline and four somatic, 7/14 biallelic) in 23 samples from 22 patients (Fig. 4H and tables S29 and S30). Some caution should be exercised in interpreting somatic mutations in cancers with high burdens of substitutions or indels as these could be chance events. Regardless, it is worthy to note that a genetic driver cannot be identified for approximately one in every two cancers with MMRd signatures. Methylation data are not available for assessment.
In addition, we confirm SBS10a is associated with POLE dysregulation. 100% of 65 GEL samples with SBS10a had POLE mutations consistent with proofreading dysfunction (Fig. 4H and tables S29 and S30). We also confirm that two of five GEL samples with SBS10d carried POLD1 exonuclease domain mutation, p.(Asp316Asn) reported previously (29). Here, we report an identical p.(Asp903Tyr) mutation in DNA polymerase domain B in the remaining three samples.
Two signatures were previously attributed to a mixed phenotype of MMRd and polymerase mutants, SBS14 (MMRd and POLE dysfunction) and SBS20 (MMRd and POLD dysfunction) (29). Of 14 samples with SBS14, 13 had potential POLE drivers (four established and nine putative, tables S29 and S30). Eleven out of fourteen samples also had truncating mutations in MMR genes (MSH6, MSH2, MLH1, or PMS2: three germline and 15 somatic mutations), but only six appeared to be inactivated on both parental alleles. Similarly, of eight samples with SBS20, four had missense drivers in POLD1 (one germline and four somatic). Seven of the eight also had inactivating mutations in MSH6 or MSH2, germline (n=4) and/or somatic (n=7), six of which showed biallelic inactivation. Again, all these tumors had high mutation burdens; thus, some mutations could be chance events due to high MMRd mutation rates. Moreover, elevated mutation rates of MMRd signatures cause a high likelihood of substitutions occurring adjacent to each other, falsely creating DBS patterns DBS14, DBS29, and DBS37 (fig. S8, F to H).
Lastly, we identify a signature with a defined C>T peak at GCG, SBS97, most closely resembling SBS15; however, it can be distinguished from SBS15 by strong T>C at GTC and T>G at GTT trinucleotides (Fig. 4F). Seen in three colorectal cancers in GEL and five in Hartwig, SBS97 is rare, has a strong hypermutator phenotype (29-65 subs/Mb), and a strikingly high indel rate exceeding substitutions (67-99 indels/Mb). All three GEL cases also have considerable structural variation (0.02-0.05 SV/Mb), revealing that chromosomal instability and microsatellite instability are not mutually exclusive in colorectal cancer. No causative drivers have been confirmed so far.
In all, MMRd and polymerase-dysregulated signatures are prominent in colorectal (413/2,348, ~18%) and uterine cancers (258/713, 36%) in the GEL cohort (Fig. 4D). Sporadic incidences of MMRd occurred in the stomach (11), prostate (3), pancreas (1), ovary (18), NET (2), lung (8), kidney (9), oropharyngeal (1), CNS (3), breast (14), sarcoma (16) and bladder cancers (3) (total 89/9,161, <1% total), with clinical implications.
Compromised components of double-strand break repair (DSBR)
SBS3 was previously shown to distinguish BRCA1/BRCA2-null from sporadic breast cancers (6). SBS8 is increased in BRCA1/BRCA2-null cancers (9). We applied a previously developed algorithm, HRDetect (17, 30), designed to detect tumors with BRCA1/BRCA2-compromised DSBR, to the GEL cohort (Fig. 4G, fig. S9 and table S31). The prevalence of HRDetect high scores (5th-95th percentile confidence interval above 0.5) was variable within each tumor type. More than 30% of all ovarian cancers had high HRDetect scores, ~11% of breast cancers (predominantly estrogen receptor-positive cancers), ~7% of pancreatic cancers, ~4% of all uterine cancers, 1.6% of lung cancers, ~1% of stomach cancer, and less than 1% of prostate, bone and colorectal cancers also had high scores. The causes of high HRDetect scores were identified in 231/493 individuals (47%, biallelic loss confirmed in 40%, Fig. 4I and tables S29 and S30) and included germline and somatic mutations in BRCA1, BRCA2, PALB2, RAD51C, and RAD51D as described previously (6, 9, 31, 32). Promoter hypermethylation data were not available.
Environmental sources of mutational signatures
UV-like C>N signatures at CCN and TCN
We reinforce SBS7a (defined by C>T at CCN and TCN) in skin tumors with associated DBS1 characterized by CC>TT dinucleotides (33) (Fig. 5, A and B). However, we highlight three signatures that occurred at similar trinucleotides CCN/TCN and that could be miscalled as UV-related but may be due to alternative etiologies.

(A) SBS signatures occurring at CCN and TCN, with similarities to UV-related SBS7a. (B) Correlations of the signatures in (A) with DBS signatures. (C) SBS signatures presenting T>A, with similarities to AAI-associated SBS22. (D) SBS signatures indicating prior platinum exposure. (E) Correlations of the signatures in (D) with DBS signatures. (F) SBS signatures presenting C>A with similarities to tobacco-associated SBS4. (G) Correlation of the signatures in (F) with DBS signatures. Numbers in each column report the number of organs implicated in the correlative analyses. A correlation is computed independently for each organ and the correlations are displayed as a boxplot. Boxplots denote median (horizontal line) and 25th to 75th percentiles (boxes). The lower and upper whiskers extend 1.5×IQR.
SBS129, observed once in a nodular malignant melanoma (GEL-2501934-11) and once in a leiomyosarcoma (GEL-2300438-11), is characterized by C>T transitions at CCN, particularly CCA and CCT, but not TCN trinucleotides (Fig. 5A). It is distinguishable from SBS7a by its rarity and lack of CC>TT dinucleotides. However, SBS129 presents a transcriptional strand asymmetry with excess C>T mutations on the non-transcribed strand, the same as SBS7a. Apart from somatic TP53 mutations, no other potential genetic associations have been identified.
SBS38 is identical in its trinucleotide preponderance to SBS129, except it results in C>A transversions instead (Fig. 5A). Although reported before (14), it is rare, and its etiology is unknown. Here, we identify it in 30 cancers (29 skin, one lung, table S23) in GEL and note that it can either be a dominating phenotype or occur in combination with SBS7a, SBS17, and SBS18. Notably, among the samples affected by SBS38, we found all three anorectal mucosal cancers in the GEL cohort, an aggressive, unusual mucosal melanocytic cancer. This uncommon signature occurring in a very rare tumor-type hints at a germline genetic predisposition. Yet, we have not been able to identify a causative gene. Minor transcriptional strand bias is noted with more mutations on the transcribed strand for C>A mutations.
Lastly, SBS137 was observed twice in GEL brain cancers (table S23) and would superficially seem highly similar to UV (Fig. 5A). Critically, affected tumors do not have a CC>TT DBS signature (Fig. 5B) and demonstrate transcriptional strand bias in the opposite direction to UV (table S32), with an excess of C>T mutations on the transcribed strand (likely representing an excess of G>A on the non-transcribed strand). Its tallest peak is at CCC, dissimilar to the SBS7a peak at TCC. By contrast, in a metastatic CNS lesion derived from a cutaneous primary (GEL-2906789-11), the classic appearance of SBS7a and DBS1 is observed. This suggests that SBS137 is a distinct signature with currently uncertain cause.
Aristolochic-acid exposure and similar patterns
SBS22 is due to aristolochic acid (AAI) (33) (Fig. 5C). All three renal cancers in GEL with SBS22 were from patients reporting ethnic-minority ancestry. None reported past exposure to AAI.
We noted that SBS113 is similar to SBS22, has tall peaks in T>A with additional contributions from T>C at GTN, and is seen in one CNS (GEL-2585923-11), one colorectal (GEL-2282347-11), and one lung cancer (GEL-2158956-11). There is no history of exposure to AAI in these patients, although all three patients had complex therapeutic histories, including extensive exposure to psychotropic drugs and anti-epileptics.
In previous work, alternative compounds from unrelated chemical families, specifically dibenzo[a,l]pyrene (DBP) and its diol-epoxide (DBPDE) from the polycyclic aromatic hydrocarbons (PAH) family in tobacco smoke, that caused bulky adducts on adenines similar to AAI, were capable of generating signatures nearly identical to AAI (33). Thus, given similarities to SBS22, SBS113 may represent mutational processes with alternative etiologies that also cause adducted adenines.
Platinum exposure
SBS31 is associated with prior platinum exposure (34) (Fig. 5D). This signature – characterized by C>T peaks at CCC and CCT, C>A peaks at ACC, CCT, GCC, and a modest T>A peak at CTN – has been demonstrated experimentally in a human cell line model previously (33).
SBS35 is similar to SBS31, though it has smaller contributions at all trinucleotides and looks noisier (14). SBS104 may be related to SBS31 as it shows C>A peaks at CCC and CCT and was found in two Hartwig metastatic samples that had exposure to platinum. Two additional signatures, SBS111 and SBS112, have the components seen in SBS31, albeit with additional features particularly in C>A and noisier C>T components. Clinical histories of the patients carrying these signatures reveal that all had past diagnoses of primary malignant neoplasms of the ovary, stomach, esophageal cancer, breast and non-Hodgkin’s lymphoma, and presented with secondaries or new primary malignancies. All patients had complex chemotherapy including platinum exposure. Perhaps these signatures are complex outcomes of multiple treatments and immune-modulation on the genome of the tumor samples isolated for sequencing. Two DBS platinum signatures (DBS5 and DBS18) are also associated with these SBS signatures (Fig. 5E).
Tobacco-related signatures and others with similar C>A components
SBS4, associated with tobacco smoke exposure (33) (Fig. 5F), is seen mainly in lung cancers (at high levels ~ 90 subs/Mb). SBS4 is noted very rarely in other tumor-types (table S23), including one breast cancer (GEL-2791664-11), one colorectal lesion noted to be ‘metastatic’ (GEL-2842602-11), one ‘diffuse astrocytoma’ (GEL-2645293-11), and two CNS lesions of unknown primary (GEL-2860373-11, GEL-2500813-11). SBS4 presence is supported by DBS2 (Fig. 5G) and transcriptional strand bias in all these cases and probably indicates metastatic lesions of lung primary in these instances.
Two signatures that have similarities to SBS4 are SBS94 and SBS109 (Fig. 5F). SBS94 is characterized by C>A mutations with the tallest peak at CCC followed by CCA. In colon (9 cases) and breast (1 case), it does not have a hypermutator phenotype nor an associated DBS, but transcriptional and replication strand bias are noted for C>A variants (table S19). In bladder cancers (3 cases), there is a marked DBS pattern, despite low mutational burden (0.15-8 subs/Mb). The cause for this curious difference in tissue behavior is unclear.
SBS109 is a C>A pattern with tall peaks at NCA and NCT, though tallest primarily at ACA and TCT. Only seven bladder cancers demonstrate this phenotype and it does not have any associated DBS or TSB. The mutation burden is also low at only 0.3-3 subs/Mb. SBS107 is seen at low levels in bladder and kidney cancers (0.04-6 subs/Mb) across many samples of these tumor-types. It is a common signature in kidney/bladder cancers (1,461/1,704) and is akin to SBS109 but with additional contributions at NCC.
There are multiple signatures that have been attributed to environmental exposures which we will not discuss, including SBS11 (associated with alkylation on a mismatch repair deficient background), SBS90 (associated with duocarmycin), and SBS88 (reported as due to colibactin produced by pks+ E. Coli infection) (35, 36).
Utilizing mutational signatures going forward
The ever-increasing number of mutational signatures poses the challenge of using mutational signature analysis in practice, whether in a new study of aggregated samples or for individual patients. To address this, we acknowledge that most non-expert users will aim to understand which mutational signatures are present in a new set of patient samples that are often tissue-specific. This signature ‘fitting’ process requires users to utilize a set of circumscribed signatures to ask which pre-defined signatures are present in their samples. To explore how to better perform fitting, we first consider mutational signatures per tumor-type, using CNS tumors from the GEL cohort (Fig. 6) as an example. Additional per tumor signature summaries can be found in fig. S10 to S51 and at our website, Signal: https://signal.mutationalsignatures.com/explore/study/6.
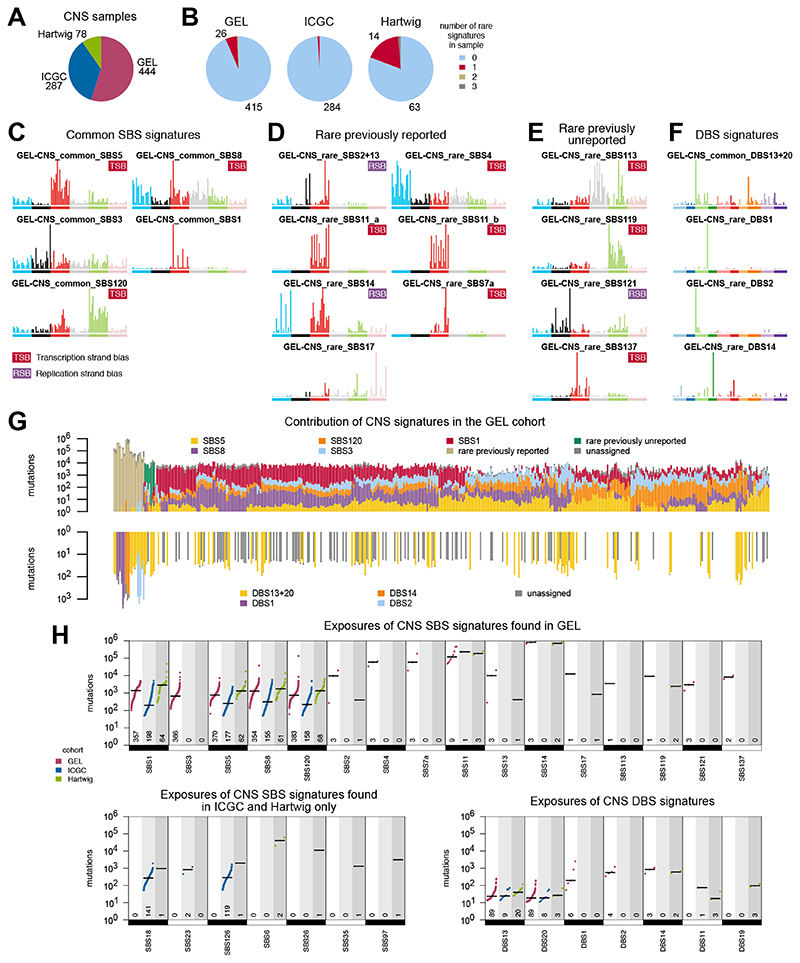
(A) Number of CNS tumors from cohorts of GEL, ICGC and Hartwig. (B) Most CNS tumors have common signatures only (light blue in pie chart) and a fraction have one rare signature (maroon). Numbers for pie chart wedges of less than 5% not shown. (C) Common SBS signatures in CNS GEL tumors. (D) Previously reported rare SBS signatures in CNS GEL tumors. (E) Prreviously unreported rare SBS signatures in CNS GEL tumors. (F) DBS signatures in CNS GEL tumors. (G) Distribution of mutational signatures in all CNS GEL tumors. For each sample, the total number of mutations is shown in log scale, while signature exposure proportions are colored linearly. Samples are clustered according to the exposure proportions using hierarchical clustering with average linkage. (H) Mutational frequencies ofcommon and rare signatures of CNS GEL cancers. Numbers at the bottom indicate the numbers of samples with each signature.
Per tumor-type summaries
A total of 809 WGS CNS tumors have been evaluated. Six percent of CNS tumors in GEL have rare signatures (Fig. 6, A and B). Common signatures in the GEL CNS cohort that have been previously reported include age-associated SBS1 and SBS5, HR-deficiency-related SBS3 and SBS8, and a previously unreported common signature, SBS120, is present in many CNS tumors at a low to moderate mutation rate (Fig. 6C). Common CNS signatures exhibit clear and reproducible tissue-specificity (fig. S52). Rare signatures observed in the GEL CNS cohort that have been previously reported include the APOBEC signatures SBS2/SBS13, SBS17 of unknown etiology, SBS11 due to temozolomide on an MMR-deficient genetic background, and MMRd signatures (SBS14) (Fig. 6D). We noted rare occurrences of tobacco-related SBS4 and UV-induced SBS7a in metastatic lesions.
We also identified several previously unreported rare signatures in CNS tumors (Fig. 6E), including SBS113 mentioned earlier, with similarities to AAI-related SBS22. SBS121, defined by C>G at ACT and TCT, is common in colorectal and stomach cancers but seen in three CNS tumors only, and its etiology is unknown. SBS119 is present in a single CNS tumor as a hypermutator phenotype (28 SBS/Mb) in GEL and in two CNS tumors in Hartwig. Lastly, SBS137 is distinct from UV, has no DBS despite a high mutational burden, and is CNS-specific and rare.
DBS1 and DBS2 are associated with UV and tobacco smoke exposure, respectively, and are seen in the samples with SBS7a and SBS4. Two previously unreported DBS signatures are observed (Fig. 6F): DBS13/DBS20 are relatively common, while DBS14 is due to the high mutational burden of MMRd SBS14 (fig. S8F).
Reassuringly, common signatures are seen in all three cohorts (GEL, ICGC, and Hartwig) robustly (Fig. 6, G and H), while the presence of rare signatures is a function of the size of the examined cohort. In all, this example highlights the landscape of common and rare signatures in this tumor-type (Fig. 6G) and provides pointers regarding how to pragmatically use mutational signatures for signature fitting of new samples.
Fitting signatures: FitMS
Cancer samples have a median of five common signatures, and when rare signatures are present, there is usually only one existent per sample (fig. S53, A and B). Learning from these results, we developed a signature-fitting algorithm, Fit Multi-Step (FitMS) (fig. S53C), which first estimates the presence of tissue-relevant common signatures, and then attempts to identify additional rare signatures in a second step, assuming that only one rare signature or two may be present.
To evaluate the performance of FitMS, we performed a simulation study where each simulated sample comprised five organ-specific common signatures, and some samples carried one rare signature (Materials and Methods). We contrasted three strategies: first, fitting all common and rare signatures together in a single step (fit all); second, a two-step method fitting common signatures using a constraint of positive residuals that are matched to rare signatures in its second step (constrainedFit); and third, a two-step method fitting common signatures, followed by the addition of rare signatures one at a time to achieve a reduction in the residual between true and modeled catalogs (errorReduction). The two-step errorReduction FitMS strategy demonstrated superior performance (fig. S53, D to F), improving the fit of common and rare signatures better than the ‘constrainedFit’ or ‘fit all’ approaches. Moreover, using organspecific common signatures rather than corresponding reference signatures improved the accuracy of signature assignment (fig. S53, G to I).
Therefore, for practical purposes, to assess which signatures are present in any new sample or set of samples, we recommend this two-step process (Fig. 7): first fitting common organ-specific signatures followed by a search for rare signatures, which can be achieved using FitMS.
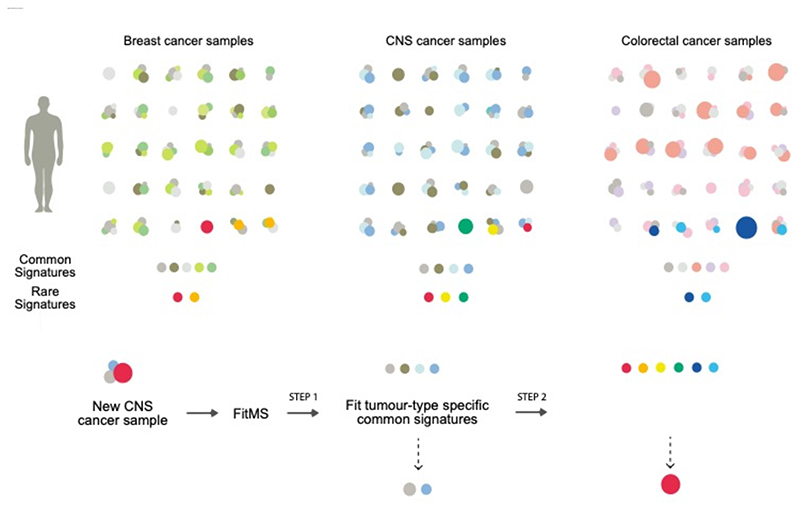
Schematic depiction of common (gray and lighter colors) versus rare signatures in three example tumor-types (breast, central nervous system (CNS) and colorectal cancers). Each patient could have different amounts of some (or all) of the common signatures. Occasionally, a patient may carry a rare signature as well (bright colors). Some common signatures are ubiquitous and present in nearly all tumor-types while some common signatures may be restricted to some tumor-types. Rare signatures may be unique (for example, yellow dot) or could also occur in other tumor-types (for example, red dots). We propose a practical package, FitMS that utilizes the insights obtained through this work. Given a new sample, for example, a new brain cancer WGS mutation catalog, FitMS will fit common CNS signatures before attempting to discover additional rare signatures seen in CNS and other tumors.
Discussion
We report a comprehensive SBS and DBS signatures analysis of a large cohort of 18,640 WGS tumors. Notably, majority of these samples were from patients recruited via the UK NHS (12,222) from across England, and the availability of open access WGS cancer data from ICGC and Hartwig Foundation were crucial for validation of findings. In all, 40 SBS and 18 DBS signatures that had not been previously reported, were revealed due to the increase in WGS cohort size. We were also able to confirm 42 previously reported SBS signatures and 9 previous DBS signatures. We introduce the notion of common and rare signatures for each tumor-type and observe that although the cohort of WGS cancers has increased substantially, most of the common signatures have been identified and many of the previously unreported signatures are low-frequency, rare processes. The landscape of signatures is thus likely to be saturating.
The power to accurately discern mutational signatures is orders of magnitude greater using a pure WGS dataset when compared to other sequencing strategies. The genomic footprint for whole exomes (WES) is 100-fold lower and 2,000-4,000-fold lower in targeted sequencing (TS) experiments. Analyzing solely WGS cancers, rather than pooling data from diverse sequencing strategies, also avoids issues related to differing AT/GC representation in WES/TS data, which influence signature extractions.
Methodologically, several points are worthy to note. First, grouping samples by organs and focusing on common mutational profiles has produced signatures that are highly reproducible across cohorts. Removing atypical samples in the first extraction step is especially important for large cohorts, where very rare signatures may be present and could interfere with the accurate identification of common signatures. Second, the use of three large independent cohorts is crucial to validate signatures found in single organs, such as SBS120, and that could otherwise be mistaken for other signatures or considered artefactual. Third, while some signatures may have very similar 96-element SBS profiles to other well-known signatures, additional information, such as co-occurrence with DBS signatures or transcriptional/replication strand bias, can suggest a different etiology and help validate them as distinct signatures. Thus, deeper investigation can often show distinctions indicating diverse etiologies, a caveat that must be considered when using mutational signatures in future analyses.
From a biological perspective, it is essential to discriminate signatures that provide diagnostic insights or are therapeutically informsoative from other signatures, particularly when there are feature similarities between them. Notable examples deliberated earlier include distinguishing MBD4-compromised SBS96 from other signatures with CpG propensity or correctly differentiating signatures that occur predominantly at CCN and TCN from UV-related SBS7a.
Additionally, we highlight endogenous signatures indicative of pathway defects that are detectable using WGS signatures but for which a genetic driver cannot be identified. It is worthy of note that a causal genetic event could not be detected for one in two cases with MMRd and one in two cases with HR-deficiency, indicating that signature analysis has increased sensitivity to identify these defects than examining mutations in selected genes, using targeted sequencing strategies. Furthermore, an agnostic WGS approach to tumor characterisation will help reveal abnormalities that we currently neither seek nor detect using customary diagnostic pathways. For example, we found MMRd associated signatures in many tumour types with a frequency lower than 1% including stomach, prostate, pancreas, ovary, NET, lung, kidney, oropharyngeal, CNS, breast, sarcoma and bladder cancers. Given reported therapeutic relationships between MMRd phenotypes and immune checkpoint inhibitors (37–39), from a personalized pan-cancer therapeutics perspective, many of these patients could be eligible for treatment options that would otherwise not be available to them.
We note that many of the previously unreported signatures have no known etiology currently. This is not surprising because of the complexity of drawing causal relationships, particularly for endogenous signatures, which can be the outcome of multiple co-occurring events. For example, a gene defect in MBD4 could convert the ubiquitous C>T at CpG into a hypermutator phenotype (SBS96), or a pathophysiological state such as replication stress could amplify APOBEC-related SBS13. Some endogenous signatures may only manifest as part of an adaptive response to stressful stimuli. For example, SBS17, defined by T>G and T>C mutations, was reported in mouse cells that have been through immortalization, in normal human cells treated with 5-FU (40), and in a wide variety of cancers. Thus, many of the signatures of unknown etiology could be due to not just a single gene defect but multi-gene or complex pathway abnormalities (41) and/or may become overt following an adaptive response to cellular stress. Further work will be required to fully comprehend the causes of many cancer mutational signatures.
As our knowledge base increases, the complexity of assigning genetic causality to signatures is evident in examples such as the OGG1 polymorphic risk allele, where some patients exhibit SBS108 clearly, and others do not. Looking forward, alternative strategies may be needed to detect the contribution of moderate and lower penetrance germline risk alleles to somatic signatures in large cohorts.
Notably, the present analysis introduces the concept of common versus rare signatures within each tumor-type. It highlights how an increased number of samples may help discern common signatures that occur at low levels for specific tumor-types. Greater sample numbers may also help unveil signatures that occur at a low frequency in the population. Crucially, the availability of independent, open-access datasets such as from the ICGC and HMF has been instrumental in corroborating these common and rare signatures identified within the GEL dataset. While it is far simpler to discuss signatures as unifying reference patterns across all organs, it is important to note that these are mathematical reference patterns, an average of many extractions, and not necessarily an accurate biological representation of the process in any given tissue. For users seeking to learn what signatures may be present in a new set of samples, it may be more advisable to use organ-specific signatures to perform an analysis rather than mathematically-averaged signatures.
Thus, here we suggest a strategy of using mutational signatures, which considers the biological insights and complexities described in this work. FitMS invites the user to use common organspecific signatures in the first instance, followed by hunting down the presence of rare signatures subsequently (Fig. 7).
Indeed, as many national cancer genomic endeavors take off worldwide over the next decade, we look forward to utilizing WGS data maximally to advance individualized cancer care.
Methods summary
Datasets
We considered three large pan-cancer whole genome cohorts: the Genomics England Limited (GEL) version 8 cohort of the 100,000 Genomes Project (7) comprising 15,838 WGS paired samples, the ICGC cohort (9, 11) comprising 3,001 WGS paired samples, and the Hartwig cohort (12) of 3,417 WGS tumor samples. After considering comparability of tumor-types across cohorts and quality control (QC) of GEL data, we focused our analysis on 12,222 high quality WGS GEL cases (tables S1 to S6).
Mutational Signature Extraction
For each tumor sample, we counted the number of somatic mutations and constructed SBS (96 channel) and DBS (78 channel) mutational catalogs (tables S7 and S8). Mutational signatures were analyzed independently for each tumor type in each of the three cohorts (Fig. 1C). First, we clustered mutational catalogs and excluded samples with unusual profiles (hierarchical clustering using 1 – cosine similarity as distance) (fig. S1, A to C), aiming at reducing the number of rare, complicating signatures and obtaining fewer, more accurate signatures. Second, we used non-negative matrix factorization (NMF) with Kullback-Leibler divergence (KLD) optimization, repeated bootstrapping (at least 300 bootstraps), and removed poor local minima (17). We identified a set of ‘common’ mutational signatures that were organ- and cohort-specific. Third, we fitted the common signatures into all samples of a given cohort and tissue type, and identified samples with high reconstruction error to identify unexplained processes or ‘rare’ mutational signatures (details in supplementary materials) (fig. S1, D to H) (tables S9 to S12).
To define signature exposures in each sample, we used a signature ‘fit’ procedure. Briefly, the number of mutations attributed to each signature in each sample were estimated using organ-specific signatures detected in their originating cohort utilizing KLD optimization (NNLM R package) and bootstrapping (200 bootstraps) (17). Point estimates of exposures were the median of the exposures obtained from bootstrapping. Exposures below 5% of the total SBS burden or below 25% of DBS burden per sample were set to zero because of the risk of over-fitting.
Reference signatures
To permit comparability across cohorts and organs, we defined ‘reference signatures’ (Fig. 1G). In brief, we clustered all common and rare mutational signatures (757 SBS or 301 DBS signatures) (tables S13 and S14) and obtained clusters of highly similar signatures (187 SBS and 60 DBS clusters). Cluster averages were termed ‘distinct patterns’ (tables S15 and S16). We assigned each distinct pattern to one of three groups: i) a reliably recurrent distinct pattern observed in multiple independent extractions; ii) a mix of two or more distinct patterns; iii) a singleton pattern found in one organ in one cohort (tables S17 and S18). Recurrent distinct patterns were additionally clustered to remove patterns that may simply be a variant of another pattern. Mixed distinct patterns that could be estimated as a combination of two distinct patterns using non-negative least squares were dismissed. Singleton distinct patterns were also curated and dismissed if they could simply be variants of other reference signatures. If they had been reported in other studies, they were retained as reference signatures. A total of 120 SBS and 39 DBS reference signatures were identified.
A QC status was assigned to each of the reference signatures: green, amber or red. QC green signatures were those extracted independently multiple times and/or reported in orthogonal studies. QC amber status was given to signatures with limited supporting evidence, such as signatures identified in only one extraction and not reported previously. QC red status was assigned to signatures that were mathematical or alignment artefacts. After QC, 82/120 SBS and 27/39 DBS reference signatures remained QC green (tables S19 and S20, SBS/DBS final reference signatures (tables S21 and S22), exposures (tables S23 and S24)). Conversion matrices that map reference signatures to organ-specific signatures of each cohort are in tables S25 and S26).
Additional analytics relating to correlations with germline and somatic driver events can be found in supplementary materials and tables S27 to S30.
Replication and transcription strand bias were calculated as in previous work (42). Briefly, we counted classes of single nucleotide variants (C>A, C>G, C>T, T>A, T>C, T>G) taking into account whether they appeared on the lagging or leading strand (according to MCF-7 reference repliseq data), or on the transcribed or non-transcribed strand (according to gene orientation) (42). A paired two-tailed Student’s t-test was used to determine the significant deviation from the ‘natural’ bias given by the regions base content. The log2 ratio was used to determine the size of the asymmetry between the two strands (table S32).
HRDetect scores were computed as previously described (17, 30). HRDetect input features are exposures of SBS3 and SBS8, proportions of short deletions at microhomology, HRD-LOH index, and exposures of rearrangement signatures 3 and 5. Rearrangement signature exposures were estimated by using KLD optimization, bootstrapping, and previously published rearrangement signatures (17). HRDetect scores were computed both as point estimates and also as a distribution obtained from 1000 bootstrapped scores, as previously described (17) (table S31).
FitMS and simulation study
Signature Fit Multi-Step (FitMS) is an algorithm designed to estimate signature exposures taking advantage of the concept of common and rare signatures. FitMS has two steps. In the first step, only common signature exposures are estimated. In the second step, the presence of potential rare signatures is estimated, achievable through two possible strategies: constrainedFit or errorReduction. The constrainedFit strategy uses constrained non-negative least squares (limSolve R package) to estimate the residual between the observed and reconstructed catalogs, using only common signatures. If this residual resembled a rare signature (cosine similarity of at least 0.8) then we assumed that rare signature was present in the sample. In the errorReduction strategy, the error (KLD) between the original catalog and the fit obtained using only common signatures was compared with the error obtained using one additional rare signature, for all rare signatures considered. A rare signature is considered present if the reduction in error is at least 15%. Regardless of strategy, we recomputed sample exposures using both common signatures and any additional rare signatures.
To evaluate the performance of FitMS (fig. S53), we simulated 100 genomes, each containing 5 common signatures chosen randomly from the 9 GEL-Breast common SBS signatures. In addition, one rare signature was added to 25 out of 100 samples, each rare signature chosen randomly from 54 possible rare, curated SBS reference signatures observed in at least two independent extractions. We compared the two FitMS strategies against a “fit all” strategy, where all 9+54 signatures were used in one single signature fitting process. Each signature fit strategy produced a first estimate of the exposures, which tended to overfit signatures into samples, resulting in false positive assignments of signatures to samples with very few associated mutations. To remove false positives, we removed signature exposures that represented a very small proportion of mutations, testing thresholds from 0 to 10% of total sample mutations (fig. S53, D to I).
For users of FitMS, the set of common and rare signatures that could be fitted into any sample is thus organ-dependent and lists of signatures per organ can be found in table S33.
Full materials and methods are available in the supplementary materials (43).
Supplementary Material
Supplementary Figures
Supplementary Text
Acknowledgments
This work was enabled by access to data and findings generated by the 100,000 Genomes Project, under the auspices of the Pan-Cancer GeCIP (project RR239). The 100,000 Genomes Project is managed by Genomics England Limited (a wholly owned company of the Department of Health and Social Care) funded by the National Institute for Health Research and NHS England. The Wellcome Trust, Cancer Research UK and the Medical Research Council have also funded research infrastructure. The 100,000 Genomes Project uses data provided by patients and collected by the National Health Service as part of their care and support. This publication and the underlying research are facilitated by data that were generated by the Hartwig Medical Foundation (HMF) and the Center for Personalized Cancer Treatment (CPCT) in the Netherlands, and the International Cancer Genome Consortium.
Funding
Cancer Research UK (CRUK) Advanced Clinician Scientist Award grant C60100/A23916
Dr Josef Steiner Cancer Research Award 2019, Medical Research Council (MRC) Grant-in-Aid to the MRC Cancer unit
CRUK Pioneer Award, CRUK Early Detection Project Award C60100/A27815
CRUK Grand Challenge Award grant C60100/A25274
NIHR Research Professorship NIHR301627
This work is also supported by the National Institute of Health Research (NIHR) Cambridge Biomedical Research Centre grant BRC-125-20014. The views expressed are those of the author(s) and not necessarily those of the NIHR or the Department of Health and Social Care.
Footnotes
Author contributions:
Conceptualization: SNZ, ADMethodology: AD, TDA, HRD, AMM
Resources, new genomics and clinical data: MAB, GERC
Software: AD, XZ, TDA, AMM, JMLD, SS, JC, DPG
Data curation: GERC, AD, SNZ, HRD, AMM, YM, TDA
Investigation: SNZ, AD, XZ, TDA, HRD, AMM, GCCK, JMLD, LH, LC, GR, VYWW, ASN, AB, SEM, JY, DPG, YM, CB
Visualization: AD
Funding acquisition: SNZ
Project administration: SNZ
Supervision: SNZ, HRD
Writing – original draft: SNZ, AD
Writing – review & editing: SNZ, AD, GCCK, HRD, XZ, SS
.Competing interests:
AD, XZ, HRD and SNZ hold patents or have submitted applications on clinical algorithms of mutational signatures (MMRDetect (number pending), HRDetect: PCT/EP2017/060294, Clinical use of signatures: PCT/EP2017/060289, Rearrangement sigantures methods: PCT/EP2017/060279, Clinical predictor: PCT/EP2017/060298, Hotspots for chromosomal rearrangements: PCT/EP2017/060298) and during this project, served advisory roles for AstraZeneca, Artios Pharma and the Scottish Genomes Project.
*This manuscript has been accepted for publication in Science. This version has not undergone final editing. Please refer to the complete version of record at http://www.sciencemag.org/. The manuscript may not be reproduced or used in any manner that does not fall within the fair use provisions of the Copyright Act without the prior, written permission of AAAS.
Contributor Information
Genomics England Research Consortium :
Data and materials availability
Primary data from the 100,000 Genomes Project, which are held in a secure Research Environment, are available to registered users. Please see https://www.genomicsengland.co.uk/about-gecip/for-gecip-members/data-and-data-access for further information or contact Matt Brown, Chief Scientific Officer at Genomics England ([email protected]). The ICGC cohort contains 2471 cancer whole genomes from PCAWG (EGAS00001001692) and 530 additional breast cancers (450 from EGAS00001001178 and 80 from EGAD00001002740). The Hartwig cohort can be accessed via at www.hartwigmedicalfoundation.nl/en. Data access requests and institutional agreements are required for all cohorts. The results of the analysis can be browsed at https://signal.mutationalsignatures.com/explore/study/6, or downloaded as a compressed archive from Zenodo (44). The code used for this analysis is available as Code S1 (R scripts) and Code S2 (new version of R package signature.tools.lib that includes FitMS) on Zenodo (45).
References and Notes
Full text links
Read article at publisher's site: https://doi.org/10.1126/science.abl9283
Read article for free, from open access legal sources, via Unpaywall:
https://www.ncbi.nlm.nih.gov/pmc/articles/PMC7613262
Citations & impact
Impact metrics
Citations of article over time
Alternative metrics

Discover the attention surrounding your research
https://www.altmetric.com/details/127075438
Smart citations by scite.ai
Explore citation contexts and check if this article has been
supported or disputed.
https://scite.ai/reports/10.1126/science.abl9283
Article citations
Base-excision repair pathway shapes 5-methylcytosine deamination signatures in pan-cancer genomes.
Nat Commun, 15(1):9864, 14 Nov 2024
Cited by: 0 articles | PMID: 39543136 | PMCID: PMC11564873
TP53 mutation status and consensus molecular subtypes of colorectal cancer in patients from Rwanda.
BMC Cancer, 24(1):1266, 11 Oct 2024
Cited by: 0 articles | PMID: 39394554 | PMCID: PMC11468329
Prevalence of germline variants in Brazilian pancreatic carcinoma patients.
Sci Rep, 14(1):21083, 10 Sep 2024
Cited by: 0 articles | PMID: 39256447 | PMCID: PMC11387492
Mutational Features and Tumor Microenvironment Alterations in High-Grade Appendiceal Cancers Treated With Iterative Hyperthermic Intraperitoneal Chemotherapy.
JCO Precis Oncol, 8:e2400149, 01 Sep 2024
Cited by: 0 articles | PMID: 39259912
Large-scale analysis of whole genome sequencing data from formalin-fixed paraffin-embedded cancer specimens demonstrates preservation of clinical utility.
Nat Commun, 15(1):7731, 04 Sep 2024
Cited by: 0 articles | PMID: 39231944 | PMCID: PMC11374794
Go to all (85) article citations
Data
Data behind the article
This data has been text mined from the article, or deposited into data resources.
BioStudies: supplemental material and supporting data
Data Citations
- (1 citation) DOI - 10.5281/zenodo.5571551
SNPs
- (1 citation) dbSNP - rs113561019
Similar Articles
To arrive at the top five similar articles we use a word-weighted algorithm to compare words from the Title and Abstract of each citation.
The repertoire of mutational signatures in human cancer.
Nature, 578(7793):94-101, 05 Feb 2020
Cited by: 1602 articles | PMID: 32025018 | PMCID: PMC7054213
A Compendium of Mutational Signatures of Environmental Agents.
Cell, 177(4):821-836.e16, 11 Apr 2019
Cited by: 301 articles | PMID: 30982602 | PMCID: PMC6506336
A practical framework and online tool for mutational signature analyses show inter-tissue variation and driver dependencies.
Nat Cancer, 1(2):249-263, 17 Feb 2020
Cited by: 129 articles | PMID: 32118208 | PMCID: PMC7048622
Portrait of a cancer: mutational signature analyses for cancer diagnostics.
BMC Cancer, 19(1):457, 15 May 2019
Cited by: 51 articles | PMID: 31092228 | PMCID: PMC6521503
Review Free full text in Europe PMC
Funding
Funders who supported this work.
Cancer Research UK (7)
Grant ID: 24043
Advancing the understanding and applications of mutational signatures
Professor Serena Nik-Zainal, University of Cambridge
Grant ID: 23916
Accelerating translation of mutational signatures to the clinic
Professor Serena Nik-Zainal, University of Cambridge
Grant ID: A23433
Advancing the understanding and applications of mutational signatures
Professor Serena Nik-Zainal, University of Cambridge
Grant ID: A23916
Prevent Ductal Carcinoma in Situ Invasive Overtreatment Now - PRECISION
Professor Serena Nik-Zainal, University of Cambridge
Grant ID: A25274
Accelerating translation of mutational signatures to the clinic
Professor Serena Nik-Zainal, University of Cambridge
Grant ID: 23433
eDyNAmiC (extrachromosomal DNA in Cancer) – Understanding the biology of ecDNA generation and action, and developing new ways to target these mechanisms in cancer
Professor Paul Mischel, Stanford University
Grant ID: CGCATF-2021/100012
National Institute for Health Research (NIHR) (2)
Harnessing the power of cancer whole genome sequencing for clinical utility
Professor Serena Nik-Zainal, University of Cambridge
Grant ID: NIHR301607
Preventing Healthcare Associated Infection and Antimicrobial Resistance in Africa
Professor Nicholas Feasey, Liverpool School of Tropical Medicine
Grant ID: NIHR301627