Abstract
Background
Amyotrophic lateral sclerosis (ALS)-related white-matter microstructural abnormalities have received considerable attention; however, gray-matter structural abnormalities have not been fully elucidated. This study aimed to evaluate cortical microstructural abnormalities in ALS and determine their association with disease severity.Methods
This study included 34 patients with ALS and 30 healthy controls. Diffusion-weighted data were used to estimate neurite orientation dispersion and density imaging (NODDI) parameters, including neurite density index (NDI) and orientation dispersion index (ODI). We performed gray matter-based spatial statistics (GBSS) in a voxel-wise manner to determine the cortical microstructure difference. We used the revised ALS Functional Rating Scale (ALSFRS-R) to assess disease severity and conducted a correlation analysis between NODDI parameters and ALSFRS-R.Results
In patients with ALS, the NDI reduction involved several cortical regions [primarily the precentral gyrus, postcentral gyrus, temporal cortex, prefrontal cortex, occipital cortex, and posterior parietal cortex; family-wise error (FWE)-corrected P<0.05]. ODI decreased in relatively few cortical regions (including the precentral gyrus, postcentral gyrus, prefrontal cortex, and inferior parietal lobule; FWE-corrected P<0.05). The NDI value in the left precentral and postcentral gyrus was positively correlated with the ALS disease severity (FWE-corrected P<0.05).Conclusions
The decreases in NDI and ODI involved both motor-related and extra-motor regions and indicated the presence of gray-matter microstructural impairment in ALS. NODDI parameters are potential imaging biomarkers for evaluating disease severity in vivo. Our results showed that GBSS is a feasible method for identifying abnormalities in the cortical microstructure of patients with ALS.Free full text

Cortical microstructural abnormalities in amyotrophic lateral sclerosis: a gray matter-based spatial statistics study
Abstract
Background
Amyotrophic lateral sclerosis (ALS)-related white-matter microstructural abnormalities have received considerable attention; however, gray-matter structural abnormalities have not been fully elucidated. This study aimed to evaluate cortical microstructural abnormalities in ALS and determine their association with disease severity.
Methods
This study included 34 patients with ALS and 30 healthy controls. Diffusion-weighted data were used to estimate neurite orientation dispersion and density imaging (NODDI) parameters, including neurite density index (NDI) and orientation dispersion index (ODI). We performed gray matter-based spatial statistics (GBSS) in a voxel-wise manner to determine the cortical microstructure difference. We used the revised ALS Functional Rating Scale (ALSFRS-R) to assess disease severity and conducted a correlation analysis between NODDI parameters and ALSFRS-R.
Results
In patients with ALS, the NDI reduction involved several cortical regions [primarily the precentral gyrus, postcentral gyrus, temporal cortex, prefrontal cortex, occipital cortex, and posterior parietal cortex; family-wise error (FWE)-corrected P<0.05]. ODI decreased in relatively few cortical regions (including the precentral gyrus, postcentral gyrus, prefrontal cortex, and inferior parietal lobule; FWE-corrected P<0.05). The NDI value in the left precentral and postcentral gyrus was positively correlated with the ALS disease severity (FWE-corrected P<0.05).
Conclusions
The decreases in NDI and ODI involved both motor-related and extra-motor regions and indicated the presence of gray-matter microstructural impairment in ALS. NODDI parameters are potential imaging biomarkers for evaluating disease severity in vivo. Our results showed that GBSS is a feasible method for identifying abnormalities in the cortical microstructure of patients with ALS.
Introduction
Amyotrophic lateral sclerosis (ALS) is a fatal neurodegenerative disease, which is characterized by a progressive loss of the upper and lower motor neurons. It causes a deterioration in motor abilities, including muscle atrophy and weakness, respiratory insufficiency, and dysphagia (1). Death related to ALS often occurs three to five years after the onset of symptoms, with respiratory failure being the chief cause (2). For early intervention and improved prognosis, it is widely believed that a timely diagnosis of ALS is essential (3). Therefore, it is crucial to clarify brain structural changes in individuals who are suspected to have ALS, as these changes may serve as neuroimaging biomarkers for the diagnosis and monitoring of disease progression.
A postmortem study reported widespread neuronal loss and axonal degeneration in patients with ALS (4). Magnetic resonance imaging (MRI) studies have identified different patterns of cortical morphology in vivo in patients with ALS, such as reduced gyrification (5), density (6), and cortical thickness (7). The brain regions implicated in ALS pathologies include motor-related areas (i.e., precentral gyrus) and extra-motor areas (e.g., cingulate gyrus, temporal lobe, thalamus, and caudate nucleus (8). Notably, the cortical abnormalities [such as macrostructural atrophy (9) and microstructural impairment (10)] are associated with disease severity and illness duration.
A promising tool for imaging of the brain microstructure is diffusion MRI (dMRI). Because it is sensitive to water molecule motion, dMRI can be used to probe the microstructure of the tissue (11). As the most popular dMRI method, diffusion tensor imaging (DTI) has consistently detected impaired white-matter (WM) microstructure in patients with ALS, mainly along the corticospinal tract (CST) and the body of the corpus callosum (12). The majority of existing DTI studies have focused on ALS-related WM microstructural abnormalities; however, the gray-matter (GM) abnormalities have not been extensively investigated (13). In fact, DTI is not a favored approach for characterizing the GM microstructure (which consists primarily of neuron cells and dendrites) due to its inability to quantify non-Gaussian diffusion arising from biological barriers (e.g., axonal sheaths, cellular membranes, and organelles) (14). Furthermore, the application of DTI for GM evaluation is limited by the partial volume effect of the WM and the cerebrospinal fluid (CSF) adjacent to the cortex. Neurite orientation dispersion and density imaging (NODDI), an advanced multicompartment diffusion model, can be used to characterize changes in the cortical microstructure, not only mitigating these DTI limitations but also ensuring higher tissue specificity (14). NODDI can estimate the biologically relevant parameters according to a “tissue model” (15). Such parameters include the orientation dispersion index (ODI), which quantifies the angular variation of neurites, and the neurite density index (NDI), which quantifies the density of neurites (14). Additionally, NODDI can account for partial volume effects by separating out the CSF component of the diffusion signal (16).
NODDI has been proven to provide better results than conventional DTI in ALS-related studies (17). For example, earlier NODDI studies on ALS demonstrated that NDI is more sensitive than DTI metrics in identifying WM microstructure abnormalities (reflected by reduced NDI) that involve the corpus callosum, frontotemporal-related tracts, and bilateral CST (18,19). In addition to WM abnormalities, ALS-related GM alterations (as shown by decreased NDI and ODI) in the right precentral gyrus were correlated with disease duration in another NODDI study that used voxel-based analysis (VBA) (18). Notably, VBA has been demonstrated to be largely affected by partial volume effects due to the spatial smoothing that excludes individual brain structure differences (20). Therefore, VBA does not quite provide a perfect fitting of the individual brain images to a common template. To address these limitations, GM-based spatial statistics (GBSS) has been proposed, which is a statistical technique that adapts the tract-based spatial statistics framework for voxel-wise analysis of NODDI metrics in GM (21). GBSS leverages NODDI to enable the GM-specific statistical analysis of cortical microstructure in an unbiased, voxel-wise manner. Unlike VBA, GBSS can reduce partial volume contamination and improve the sensitivity of NODDI measures for GM microstructural evaluation (21).
GBSS has been widely applied in the investigation of cortical GM microstructure changes related to neuropsychological disorders, such as Alzheimer disease (22), Parkinson disease (23), and schizophrenia (24). We thus hypothesized that ALS-related GM microstructural abnormalities could also be quantified by GBSS. To this end, we combined GBSS and NODDI to evaluate the cortical microstructural abnormalities in patients with ALS and to determine their association with disease severity.
Methods
Subjects
This study was conducted in accordance with the Declaration of Helsinki (as revised in 2013) and was approved by the Research Ethics Committee of Fujian Medical University Union Hospital (No. 2022WSJK022). Informed consent was obtained from all the participants. This study included 64 participants, including 30 healthy controls (HCs) and 34 patients with ALS. In the outpatient and inpatient departments of neurology, patients with ALS were recruited, while HCs were recruited from the local community through our study advertisements. All participants were examined and screened by an experienced neurologist. The diagnosis of ALS was established according to the El Escorial criteria (25), and the severity of disease was assessed using the revised ALS Functional Rating Scale (ALSFRS-R) (26). We calculated the rate of disease progression as follows: (48 − ALSFRS-R)/disease duration. Table 1 summarizes the participants’ clinical characteristics and demographics. Participants were excluded for the following reasons: (I) other neuropsychiatric disorders, such as Alzheimer disease, Parkinson disease, epilepsy, or depression; (II) intake of psychotropic medications; (III) presence of other serious disorders, such as respiratory failure, angiocardiopathy, and cancer; and (IV) contraindications to MRI examination.
Table 1
Clinical and demographic index | Healthy controls (n=30) | Patients with ALS (n=34) | P value |
---|---|---|---|
Age (years) | 53.1±7.6 | 54.0±11.9 | 0.733a |
Gender (males/females) | 23/7 | 20/14 | 0.129b |
Education (years) | 7.4±2.9 | 6.2±4.2 | 0.075c |
ASLFRS-R score | – | 36.8±7.1 | – |
Disease duration (months) | – | 11.9±8.3 | – |
Progression rate (points/month) | – | 1.3±1.2 | – |
Data are presented as the mean ± standard deviation. a, b, and c were determined by the independent samples t-test, Chi-square test, and Mann-Whitney test, respectively. ALS, amyotrophic lateral sclerosis; ALSFRS-R, revised Amyotrophic Lateral Sclerosis Functional Rating Scale.
MRI data acquisition
We used a Prisma 3-T MRI scanner (Siemens Healthineers, Erlangen, Germany) for data acquisition. We used a multishell spin-echo echo-planar imaging sequence for the diffusion-weighted image collection, and each nonzero b value (b values =1,000, 2,000, and 3,000 s/mm2) had 64 gradient directions. We also obtained ten non-diffusion-weighted (b value =0 s/mm2) images. The additional acquisition parameters were as follows: repetition time (TR) =2,500 msec, echo time (TE) =81 msec, number of averages =1, slice thickness =2 mm, field of view (FOV) =260×260 mm, matrix =130×130, flip angle =90°, number of slices =72, the voxel spatial resolution =2.0×2.0 mm2, and multiband factor =4.
Data preprocessing
We conducted preprocessing on diffusion data using the Functional Magnetic Resonance Imaging of the Brain (FMRIB) Software Library (FSL) (27), including eddy current and head motion correction. Subsequently, the corrected data with a b value of 1,000 s/mm2 were applied to fit the diffusion tensor model (28) with an open-source package in Python (DIPY). We employed the weighted linear least squares method (29) for the calculation of fractional anisotropy (FA). Additionally, the corrected data with all b values were fitted to the three-compartment NODDI tissue model (14) with the Accelerated Microstructure Imaging via Convex Optimization (AMICO) (30), from which we derived quantitative maps of the intracellular volume fraction (FICVF) of neurites, ODI, and isotropic volume fraction (ISOVF). In this context, we considered FICVF to be the NDI and ISOVF to be the CSF fraction.
GBSS analysis
We performed GBSS to analyze the microstructural changes of GM using scripts available online (https://github.com/arash-n/GBSS) (24). As illustrated in Figure 1, FA maps were segmented into two-tissue classes to obtain a WM fraction map via Atropos (31). We then calculated the GM fraction by subtracting the WM fraction and CSF fraction from 1. Pseudo-T1-weighted images were then generated by combining the GM fraction and WM fraction based on their corresponding weights. A study-wise template was constructed using participants’ pseudo-T1-weighted images through Advanced Normalization Tools (ANTs; http://stnava.github.io/ANTs) (32). Subsequently, we warped the GM fraction and NODDI metrics in the native space to the template using warp parameters. The mean GM fraction map in the study-wise space was used to generate the GM skeleton via the tbss_skeleton tool in FSL (33). Finally, we projected the NODDI metrics onto the skeleton with the assistance of the distancemap program in FSL (33). Notably, the GM skeleton encompassed voxels meeting the criterion of GM fraction >0.65 in >75% of the participants, while the remaining voxels were filled with the surrounding voxels (24).
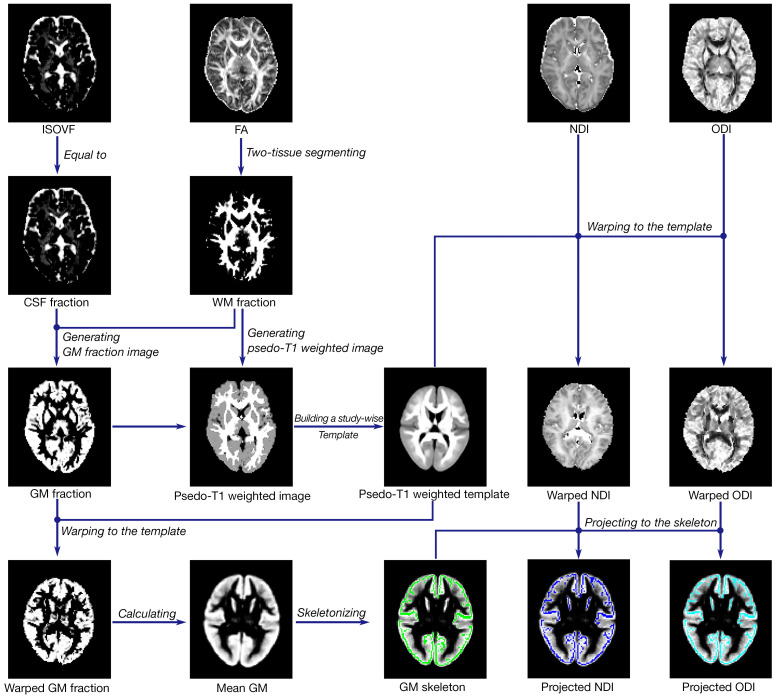
Data processing pipeline. First, metrics were reconstructed using DTI and NODDI models, including FA, ISOVF, ODI, and NDI. Second, GM fraction images were obtained using WM fraction and CSF fraction. Third, native pseudo-T1 images were generated by adding the weighted WM fraction and GM fraction. Fourth, a study-wise template was created using ANTs, and metrics were warped to the template. Finally, NDI and ODI were projected onto the skeleton represented in green after the GM skeleton was generated using the mean GM fraction map. ISOVF, isotropic volume fraction; CSF, cerebrospinal fluid; GM, gray matter; FA, fractional anisotropy; WM, white matter; NDI, neurite density index; ODI, orientation dispersion index; DTI, diffusion tensor imaging; NODDI, neurite orientation dispersion and density imaging; ANTs, Advanced Normalization Tools.
Statistical analysis
We investigated the cortical microstructural distinctions between the HCs and individuals with ALS based on NDI and ODI metrics. Additionally, the associations between NODDI metrics and clinical information, including ALSFRS-R score, disease duration at the scan date, and progression rate were evaluated. These evaluations were limited to areas with significant intergroup differences. A nonparametric permutation test was conducted for these analyses using the Randomise tool in FSL with 5,000 permutations, with adjustments being made for age, sex, and years of education. Threshold free cluster enhancement (TFCE) (34) was applied to identify significant regions, with no arbitrary cluster-forming threshold being defined. Family-wise error (FWE) correction was performed to address multiple comparisons, with a significant threshold set at PFWE<0.05. To pinpoint significant regions, we aligned the Brainnetome Atlas (35) and probabilistic cerebellar atlas (36) to the study-wise space using ANTs.
Results
In patients with ALS, a significantly decreased NDI was found in several regions of the GM, primarily the left precentral gyrus, left postcentral gyrus, bilateral temporal cortex (including the bilateral superior temporal gyrus, middle temporal gyrus, inferior temporal gyrus, fusiform gyrus, hippocampus, and parahippocampal gyrus), left prefrontal cortex (including the left middle frontal gyrus, inferior frontal gyrus, and anterior cingulate gyrus), bilateral occipital cortex (including the right medioventral occipital cortex and bilateral lateral occipital cortex), bilateral posterior parietal cortex (including the bilateral inferior parietal lobule, precuneus, and posterior cingulate gyrus), and bilateral cerebellum (FWE-corrected P<0.05; see Figure 2, Table 2).
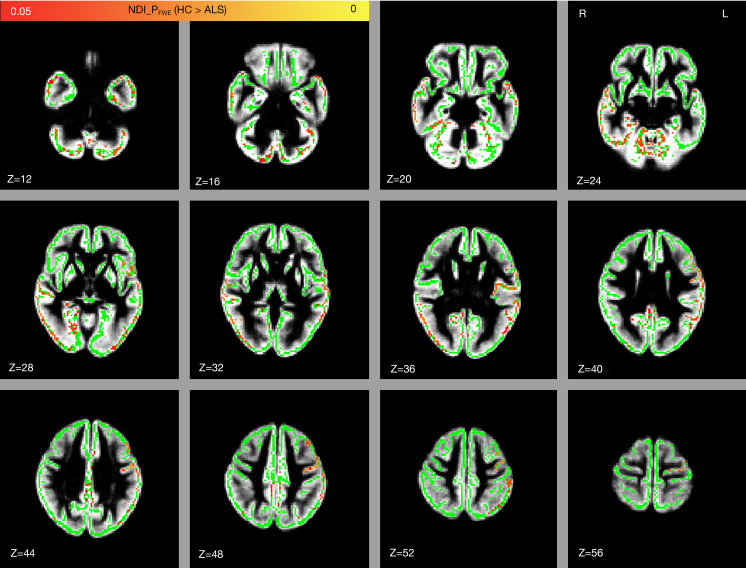
Comparison of NDI between the healthy controls and patients with ALS. Significant regions in red and yellow are reported for P values <0.05 with FWE correction. The gray-matter skeleton is shown in green, and the mean gray matter is shown in gray. NDI, neurite density index; FWE, family-wise error; HC, healthy control; ALS, amyotrophic lateral sclerosis; R, right; L, left; Z, z-axis of coordinates.
Table 2
Cluster | Number of voxels | Coordinates | Ppeak value | Region | ||
---|---|---|---|---|---|---|
X | Y | Z | ||||
1 | 1,242 | 71 | 47 | 21 | 0.026 | Fusiform gyrus L (16 voxels) |
Fusiform gyrus R (66 voxels) | ||||||
Parahippocampal gyrus L (11 voxels) | ||||||
Parahippocampal gyrus R (29 voxels) | ||||||
Cingulate gyrus R (3 voxels) | ||||||
Medioventral occipital cortex L (14 voxels) | ||||||
Medioventral occipital cortex R (21 voxels) | ||||||
Hippocampus L (1 voxel) | ||||||
Hippocampus R (25 voxels) | ||||||
Cerebellum_I-IV L (36 voxels) | ||||||
Cerebellum_I-IV R (29 voxels) | ||||||
Cerebellum_V L (56 voxels) | ||||||
Cerebellum_V R (48 voxels) | ||||||
Cerebellum_VI L (95 voxels) | ||||||
Cerebellum_VI ver (22 voxels) | ||||||
Cerebellum_VI R (91 voxels) | ||||||
Cerebellum_crus I L (78 voxels) | ||||||
Cerebellum_crus I R (55 voxels) | ||||||
Cerebellum_crus II L (98 voxels) | ||||||
Cerebellum_crus II ver (6 voxels) | ||||||
Cerebellum_crus II R (83 voxels) | ||||||
Cerebellum_VIIb L (76 voxels) | ||||||
Cerebellum_VIIb ver (4 voxels) | ||||||
Cerebellum_VIIb R (56 voxels) | ||||||
Cerebellum_VIIIa L (51 voxels) | ||||||
Cerebellum_VIIIa ver (25 voxels) | ||||||
Cerebellum_VIIIa R (67 voxels) | ||||||
Cerebellum_VIIIb L (25 voxels) | ||||||
Cerebellum_VIIIb ver (4 voxels) | ||||||
Cerebellum_VIIIb R (30 voxels) | ||||||
Cerebellum_IX L (14 voxels) | ||||||
Cerebellum_IX ver (2 voxels) | ||||||
Cerebellum_IX R (2 voxels) | ||||||
2 | 1,181 | 87 | 63 | 46 | 0.018 | Middle frontal gyrus L (55 voxels) |
Inferior frontal gyrus L (28 voxels) | ||||||
Precentral gyrus L (265 voxels) | ||||||
Superior temporal gyrus L (172 voxels) | ||||||
Middle temporal gyrus L (120 voxels) | ||||||
Inferior temporal gyrus L (47 voxels) | ||||||
Fusiform gyrus L (60 voxels) | ||||||
Parahippocampal gyrus L (2 voxels) | ||||||
Posterior superior temporal sulcus L (26 voxels) | ||||||
Inferior parietal lobule L (185 voxels) | ||||||
Postcentral gyrus L (105 voxels) | ||||||
Insular gyrus L (29 voxels) | ||||||
3 | 956 | 35 | 57 | 19 | 0.023 | Superior temporal gyrus R (126 voxels) |
Middle temporal gyrus R (273 voxels) | ||||||
Inferior temporal gyrus R (88 voxels) | ||||||
Fusiform gyrus R (57 voxels) | ||||||
Posterior superior temporal sulcus R (41 voxels) | ||||||
Inferior parietal lobule R (38 voxels) | ||||||
Precuneus R (45 voxels) | ||||||
Cingulate gyrus R (5 voxels) | ||||||
Medioventral occipital cortex R (90 voxels) | ||||||
Lateral occipital cortex R (180 voxels) | ||||||
4 | 127 | 66 | 45 | 42 | 0.044 | Precuneus R (21 voxels) |
Precuneus L (37 voxels) | ||||||
Cingulate gyrus L (37 voxels) | ||||||
Cingulate gyrus R (32 voxels) | ||||||
5 | 97 | 50 | 66 | 7 | 0.048 | Superior temporal gyrus R (18 voxels) |
Middle temporal gyrus R (29 voxels) | ||||||
Inferior temporal gyrus R (23 voxels) | ||||||
Fusiform gyrus R (9 voxels) | ||||||
6 | 67 | 80 | 35 | 53 | 0.044 | Inferior parietal lobule L (65 voxels) |
Lateral occipital cortex L (1 voxel) | ||||||
7 | 62 | 80 | 25 | 27 | 0.043 | Inferior temporal gyrus L (3 voxels) |
Fusiform gyrus L (8 voxels) | ||||||
Lateral occipital cortex L (60 voxels) | ||||||
8 | 29 | 88 | 36 | 37 | 0.042 | Middle temporal gyrus L (11 voxels) |
Inferior parietal lobule L (7 voxels) | ||||||
Lateral occipital cortex L (11 voxels) | ||||||
9 | 11 | 68 | 72 | 44 | 0.047 | Cingulate gyrus L (11 voxels) |
ALS, amyotrophic lateral sclerosis; NDI, neurite density index; L, left; R, right; Ver, vermis.
In patients with ALS, a significantly decreased ODI was found in relatively few components of the cortical GM, primarily left precentral gyrus, left postcentral gyrus, left prefrontal cortex (including the left inferior frontal gyrus, middle frontal gyrus, superior frontal gyrus, and orbital gyrus), and left inferior parietal lobule (FWE-corrected P<0.05; see Figure 3, Table 3).
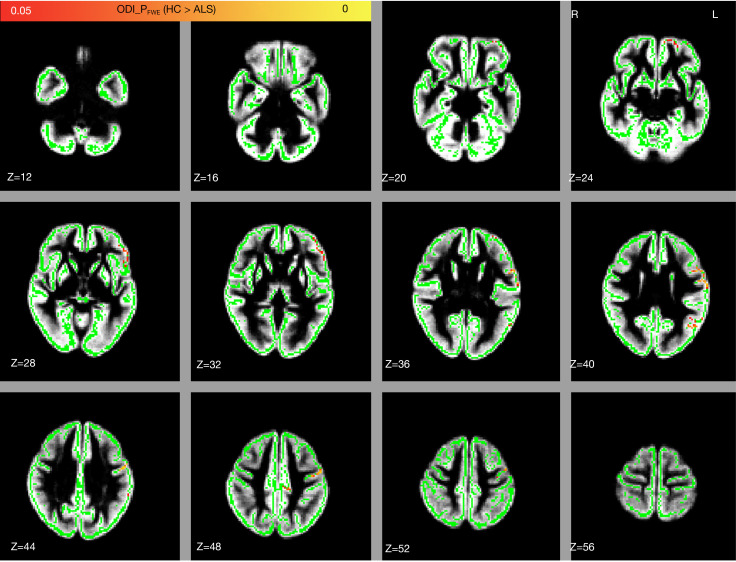
Group difference in the ODI between the healthy controls and patients with ALS. Significant regions in red and yellow are reported for P values <0.05 with FWE correction. Significant regions are displayed on the GM skeleton, which is shown in green. The underlay in gray is the mean gray matter. ODI, orientation dispersion index; FWE, family-wise error; HC, healthy control; ALS, amyotrophic lateral sclerosis; R, right; L, left; Z, z-axis of coordinates; GM, gray-matter.
Table 3
Cluster | Number of voxels | Coordinates | Ppeak value | Region | ||
---|---|---|---|---|---|---|
X | Y | Z | ||||
1 | 150 | 91 | 70 | 38 | 0.004 | Inferior frontal gyrus L (1 voxel) |
Precentral gyrus L (122 voxels) | ||||||
Postcentral gyrus L (26 voxels) | ||||||
2 | 83 | 90 | 82 | 27 | 0.033 | Middle frontal gyrus L (18 voxels) |
Inferior frontal gyrus L (65 voxels) | ||||||
3 | 63 | 84 | 38 | 40 | 0.019 | Superior temporal gyrus L (4 voxels) |
Middle temporal gyrus L (10 voxels) | ||||||
Posterior superior temporal sulcus L (5 voxels) | ||||||
Inferior parietal lobule L (44 voxels) | ||||||
4 | 29 | 84 | 88 | 18 | 0.036 | Middle frontal gyrus L (13 voxels) |
Orbital gyrus L (16 voxels) | ||||||
5 | 27 | 70 | 95 | 26 | 0.032 | Superior frontal gyrus L (11 voxels) |
Middle frontal gyrus L (13 voxels) | ||||||
Orbital gyrus L (3 voxels) |
ALS, amyotrophic lateral sclerosis; ODI, orientation dispersion index; L, left.
The NDI value in the left precentral and postcentral gyrus was positively correlated with the ALSFRS-R score (FWE-corrected P<0.05; see Figure 4, Table 4). We did not observe any correlation, however, between ODI and the ALSFRS-R score or between the diffusion metrics and disease duration or progression rate.
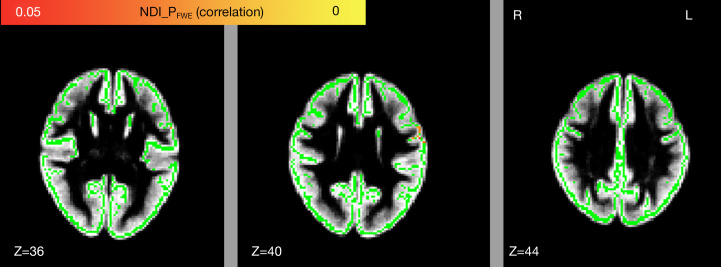
Voxel-wise relationship between NDI and the revised ALSFRS-R. Significant regions in red and yellow are reported for P values <0.05 with FWE correction. Voxels in yellow and red are the significantly positive relationships, voxels in green represent the GM skeleton, and voxels in gray are the mean gray matter. NDI, neurite density index; FWE, family-wise error; R, right; L, left; Z, z-axis of coordinates; ALSFRS-R, the Revised Amyotrophic Lateral Sclerosis Functional Rating Scale; GM, gray matter.
Table 4
Cluster | Number of voxels | Coordinates | Ppeak value | Region | ||
---|---|---|---|---|---|---|
X | Y | Z | ||||
1 | 38 | 92 | 64 | 43 | 0.015 | Precentral gyrus L (26 voxels) |
Postcentral gyrus L (12 voxels) |
ALSFRS-R, revised ALS Functional Rating Scale; NDI, neurite density index; L, left.
Discussion
This study was the first to investigate the microstructural changes in the GM of patients with ALS using the NODDI and GBSS. The primary results of this study were as follows: (I) The NDI reductions involved several cortical GM (primarily the precentral gyrus, postcentral gyrus, temporal cortex, prefrontal cortex, occipital cortex, and posterior parietal cortex) in patients with ALS. (II) Patients with ALS demonstrated ODI reductions in relatively few cortical regions (including the precentral gyrus, postcentral gyrus, prefrontal cortex, and inferior parietal lobule). (III) The NDI value in the left precentral and postcentral gyrus showed a positive correlation with the ALS disease severity. NODDI measurements may provide more detailed information regarding specific GM microstructural changes, which would further shed light on the neuropathological mechanisms of ALS.
As ALS is a neurodegenerative disease, several DTI studies have reported GM microstructural abnormalities in patients with this condition (37,38). NODDI can better characterize the microstructural properties of the GM microstructure as a more advanced diffusion model. ODI can reveal the arrangement of the neurite fibers, which may reflect complexity of dendritic angular variation in GM, and NDI can capture the density of myelinated dendrites in GM (17). Thus, the observed NDI and ODI reductions in GM can reflect dendrite loss and a reduction in spines, respectively (39). In line with our results, previous in vivo studies using MRI reported dendritic degeneration in the motor cortex in patients with ALS (18), which may be related to the C9orf72 mutation (19). In addition, an ex vivo study suggested that the pathological TDP-43 (neuropathological hallmark protein of ALS) can decrease deacetylase activity, resulting in impairment of neurite growth (40).
The most obvious neuroimaging feature in patients with ALS is the impairment of the motor cortex microstructure (41). We consistently observed NDI and ODI reductions in the precentral gyrus (the primary motor cortex) in patients with ALS. In line with our results, the NDI and ODI reductions in the precentral gyrus of patients with ALS were also demonstrated in a previous NODDI study using VBA (18). In addition, we observed NDI and ODI reductions in the postcentral gyrus (primary somatosensory cortex) in patients with ALS. This finding aligns closely with previous studies on ALS, which report morphological atrophy (7) and hypometabolism (42) in the postcentral gyrus. Taken together, these findings further suggest that the impairment in precentral and postcentral gyrus are key underlying causes of motor and sensory disturbances in patients with ALS (43). This conclusion is supported by the observed association between NDI in left precentral and postcentral gyrus and ALS disease severity.
A significant amount of research has shown the temporal regions to have extensive involvement in ALS (8,44,45). Similarly, we observed microstructural impairments in the bilateral temporal cortex of patients with ALS, which was reflected by the decreases of NDI and ODI. Consistent with the results of this study, previous MRI studies have reported morphological atrophy (44) and microstructural changes (45) in the temporal cortex of patients with ALS, with about 40% of these patients exhibiting pathological accumulations of the TDP-43 protein inclusion in the temporal cortex. These inclusions could be related to neurodegeneration in patients with ALS (46). Specifically, we found microstructural impairments in the hippocampus, parahippocampal gyrus, and fusiform gyrus in patients with ALS. Research has shown that the hippocampus and the surrounding parahippocampal, perirhinal, and fusiform gyrus are essential for semantic and memory processing (47). Consistently, memory deficit and language domain impairment have been confirmed in patients with ALS (48). In this study, we also observed GM microstructural impairment in the inferior temporal gyrus and the middle temporal gyrus, which are involved in cognitive processes (49). The superior temporal gyrus, a region with decreased NDI and ODI, is a key structure for language and spatial awareness and exploration (50). Thus, our findings suggest that ALS involved multiple temporal regions, providing further evidence for the heterogeneity in the clinical symptoms of ALS.
We observed the GM microstructural impairment in the prefrontal cortex (revealed by NDI and ODI reduction) in patients with ALS. This is in line with a previous ALS study that reported a decrease in FA in the prefrontal cortex (51), which is known to be involved in cognitive control (52). Functional and structural imaging studies also have supported the presence of prefrontal deficits, which are related to dysfunction of cognitive profile in patients with ALS (53,54). Therefore, we speculate that GM microstructural impairments in the prefrontal cortex may be responsible for the cognitive impairments that have been observed in patients with ALS (1).
Our results also revealed NDI and ODI reductions in the inferior parietal lobule in patients with ALS. Other abnormalities related to ALS have been identified in this region, such as surface area reduction (55) and volume decrease (7). The inferior parietal lobule is involved in motor planning and spatial and nonspatial attention (56). Thus, it could be speculated that microstructural abnormalities in the inferior parietal lobule may be associated with the motor dysfunction and cognitive deficit that have been reported in ALS (1). In addition, we detected the microstructural impairment in the precuneus, which was reflected by a reduction in the NDI. In line with our results, a previous study revealed significant GM volume reduction in the precuneus of patients with ALS (57). Given that the precuneus is known to be the hub for processing and integrating multisensory information from the frontal and temporal inputs (58), the GM microstructural impairment in the precuneus may contribute to the sensory disturbances observed in patients with ALS (43).
The anterior and posterior cingulate gyri, regions with deceased NDI, are important hubs implicated in modulating executive control (59) and retrieval of previously learned data (60), respectively. The altered microstructures in these regions may be responsible for the cognitive dysfunction in patients with ALS (61). In addition, we observed a decreased NDI in the occipital cortex of patients with ALS. In keeping with this finding, ALS-related alterations within the occipital cortex have also been observed, including cortical thinning (62) and metabolic abnormalities (42), which may give rise to dysfunction to the integration of visuomotor information (63).
The NDI decreased in the bilateral cerebellum of patients with ALS. This finding was consistent with previous reports on cerebellar macrostructural atrophy and microstructural impairment in ALS (64,65). The role of the cerebellum in the ALS pathology has already been demonstrated, and its dysfunction can exacerbate patients’ motor and cognitive deficits (64). As the cerebellum is involved in various functions such as motor control and cognition (66), microstructural damage (reflected by decreased NDI) in the cerebellum might be the one of underlying mechanisms for motor and cognitive impairment observed in patients with ALS.
This study has four principal limitations. First, the relatively small sample size might have limited the statistical power and restricted our ability to perform a subgroup analysis. Second, we regrettably did not conduct cognitive measurements on the patients with ALS, which prevented us from examining the association between NODDI measures and cognition level. Third, the well-developed GBSS method can mitigate the impact of complex cortical structures on spatial registration and reduce partial volume effects, but it also is associated with certain drawbacks. Specifically, it requires high spatial registration accuracy, and each change in cortex caused by lesions can affect the extraction of the skeleton, potentially leading to inaccurate skeleton positioning and a subsequent increase in the likelihood of false-positive or false-negative results. Moreover, GBSS employs a skeletonized cortical ribbon to capture diffusion metrics along its trajectories. However, because GM skeletonization is extracted along highly overlapped regions, GBSS also yielded low sensitivity for between-group differences around the cortical sulci (67). Fourth, we failed to consider the cause-and-effect relationship between disease progression and GM microstructural changes due to the study’s cross-sectional nature. In this regard, longitudinal data would better reveal trends in damage to the GM microstructure over time in patients with ALS.
Conclusions
In this study, we revealed that abnormalities in the GM microstructure involving motor-related areas and extramotor regions in patients ALS. The correlation between NDI and disease severity suggested that the NODDI measurements may serve as neuroimaging biomarkers for monitoring the development of the ALS. Additionally, GBSS has demonstrated its feasibility for in the detection of GM microstructural abnormalities in ALS.
Acknowledgments
Funding: This study was supported by grants from the Fujian Provincial Health Technology Project (Nos. 2022QNA022 and 2023CXA009) and the Fujian Medical University Startup Fund for Scientific Research (No. 2022QH1030).
Notes
Ethical Statement: The authors are accountable for all aspects of the work in ensuring that questions related to the accuracy or integrity of any part of the work are appropriately investigated and resolved. This study was conducted in accordance with the Declaration of Helsinki (as revised in 2013) and was approved by the Research Ethics Committee of Fujian Medical University Union Hospital (No. 2022WSJK022). Informed consent was obtained from all participants.
Footnotes
Conflicts of Interest: All authors have completed the ICMJE uniform disclosure form (available at https://qims.amegroups.com/article/view/10.21037/qims-24-236/coif). The authors have no conflicts of interest to declare.
References
Articles from Quantitative Imaging in Medicine and Surgery are provided here courtesy of AME Publications
Citations & impact
This article has not been cited yet.
Impact metrics
Alternative metrics

Discover the attention surrounding your research
https://www.altmetric.com/details/166397215
Data
Data behind the article
This data has been text mined from the article, or deposited into data resources.
BioStudies: supplemental material and supporting data
Similar Articles
To arrive at the top five similar articles we use a word-weighted algorithm to compare words from the Title and Abstract of each citation.
Neurite orientation dispersion and density imaging quantifies microstructural impairment in the thalamus and its connectivity in amyotrophic lateral sclerosis.
CNS Neurosci Ther, 30(2):e14616, 01 Feb 2024
Cited by: 1 article | PMID: 38334027 | PMCID: PMC10853891
White matter microstructural impairments in amyotrophic lateral sclerosis: A mean apparent propagator MRI study.
Neuroimage Clin, 32:102863, 23 Oct 2021
Cited by: 11 articles | PMID: 34700102 | PMCID: PMC8551695
White matter and cortical gray matter microstructural abnormalities in new daily persistent headache: a NODDI study.
J Headache Pain, 25(1):110, 08 Jul 2024
Cited by: 0 articles | PMID: 38977951 | PMCID: PMC11232337
Disentangling in-vivo microstructural changes of white and gray matter in mild cognitive impairment and Alzheimer's disease: a systematic review and meta-analysis.
Brain Imaging Behav, 17(6):764-777, 27 Sep 2023
Cited by: 1 article | PMID: 37752311
Review