Abstract
Free full text

RNA splicing is a primary link between genetic variation and disease
Abstract
Noncoding variants play a central role in the genetics of complex traits, but we still lack a full understanding of the molecular pathways through which they act. We quantified the contribution of cis-acting genetic effects at all major stages of gene regulation from chromatin to proteins, in Yoruba lymphoblastoid cell lines (LCLs). About ~65% of expression quantitative trait loci (eQTLs) have primary effects on chromatin, whereas the remaining eQTLs are enriched in transcribed regions. Using a novel method, we also detected 2893 splicing QTLs, most of which have little or no effect on gene-level expression. These splicing QTLs are major contributors to complex traits, roughly on a par with variants that affect gene expression levels. Our study provides a comprehensive view of the mechanisms linking genetic variation to variation in human gene regulation.
Expression quantitative trait loci (eQTLs) are highly enriched among the risk loci for complex diseases (1, 2), suggesting that risk variants often act by affecting aspects of gene regulation. Previous attempts to elucidate the mechanisms underlying eQTLs revealed that a large fraction of eQTLs are due to single-nucleotide polymorphisms (SNPs) that affect transcription factor binding or other aspects of chromatin function at enhancers or promoters (3–9). Moreover, SNPs that lie within active chromatin regions in relevant cell types are highly enriched among the signals obtained through genome-wide association studies (GWASs) (10, 11). In a few cases, specific links were identified between genetic variation, variation in chromatin, gene expression differences, and disease risk (12). Genetic variation might also affect gene regulation and function through pre-mRNA splicing by affecting either the expression levels or amino acid sequences of the resulting proteins (13). There are reports of splicing variants contributing to complex traits (14); however, a recent comprehensive study of splicing found no enrichment of predicted splicing variants among signals identified in GWASs (15).
We aimed to compile a detailed accounting of the effects of genetic variants on gene regulation from chromatin to proteins. To do this, we analyzed molecular data for eight regulatory traits measured in lymphoblastoid cell lines (LCLs) derived from Yoruba individuals (Fig. 1A), for which genome sequence data are available (16). Altogether, data are available for all eight molecular phenotypes in 32 individuals and at least six phenotypes in 68 individuals (table S8).
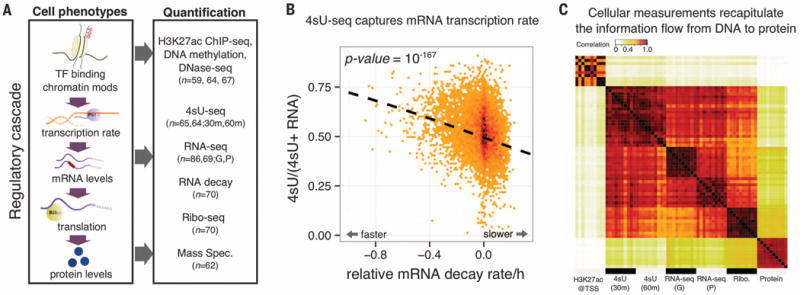
(A) QTLs mapped for eight cellular phenotypes in LCLs. For 4sU, 30 m and 60 m refer to different measurement time points. For RNA-seq, G and P refer to data from two studies (6, 17). TF, transcription factor. (B) Steady-state RNA levels reflect a balance between transcription and decay. Normalized mRNA decay rates (x axis) are plotted against the ratio of new mRNA to steady-state mRNA (y axis). Each data point is a gene. (C) A correlation matrix of seven data sets reflects the expected order of steps in gene regulation. Each entry in the matrix shows the correlation across genes between measurements of a pair of samples and/or data types. The plot shows 10 different random samples for each data type.
Seven of the eight main molecular measurements analyzed in this study were characterized previously (4–6, 17–19). We additionally measured transcription rates in 65 individuals, using 4sU-labeled RNA sequencing (4sU-seq). The 4sU assay uses a pulse of modified uridine to label the accumulation of new transcripts during a fixed time interval, in order to measure the rate of RNA synthesis (16). To confirm that the 4sU-seq data do indeed measure transcription rates, we examined them in the context of mRNA decay measurements for the same LCLs (Fig. 1B). Steady-state mRNA levels should reflect a balance between transcription and decay. We found that the ratio of new transcript levels (based on 4sU data) to steady-state RNA levels is negatively correlated with RNA decay estimates (P = 10−167, χ2 goodness of fit).
We also computed correlations between read counts across five molecular measurements (Fig. 1C). As expected, 4sU and RNA-seq data are highly correlated [Spearman correlation (rho) > 0.90]. Histone H3 lysine 27 acetylation (H3K27ac) read counts at transcription start sites (TSSs) are more strongly correlated with 4sU-seq than with RNA-seq data (P = 4 × 10−7, permutation). This is consistent with the expectation that the effect of promoter function on transcription rate is more direct than on steady-state mRNA levels. Overall, the correlation structure reflects the fact that promoter activity, transcription rates, mRNA expression levels, translation levels, and protein expression levels are regulated in a sequential ordered cascade.
We next performed mapping of QTLs across the eight molecular phenotypes, using a uniform QTL mapping pipeline [Fig. 2 and table S1 (16)]. We found that effect sizes are correlated across all phenotypes, with a minimum correlation of 0.23 between H3K27ac marks and protein levels (Fig. 2A). Effect sizes for the RNA-related phenotypes are all highly correlated (minimum r2 = 0.87). Effect sizes at the protein level are smaller on average than for translation (P = 0.002, Mann-Whitney U test), which is consistent with a previous report of potential protein expression buffering (fig. S4) (19).
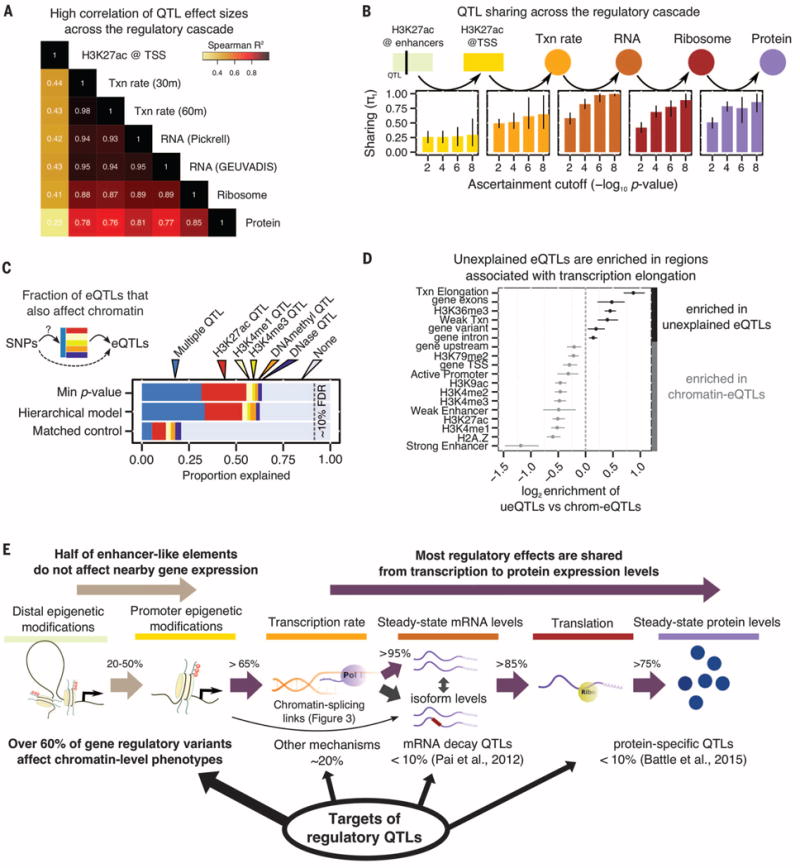
(A) Correlation of effect sizes across different measurements from eQTLs identified in the GEUVADIS YRI sample (6). Txn rate, transcription rate. (B) QTL sharing across the regulatory cascade. Each panel shows the estimated fraction of QTLs identified at one stage that are preserved at the next stage of regulation. The four bars in each panel correspond to the P-value threshold for ascertaining QTLs in each assay, using the linear regression t statistics. Bars represent 80% confidence intervals on π1, the fraction of true positives (16). The enhancer→TSS panel considers the effect of H3K27ac QTLs on the nearest TSS. (C) The fraction of expression QTLs that also affect chromatin-level phenotypes, as estimated by two models, and for matched control SNPs. About 35% of gene eQTLs do not appear to affect chromatin traits. QTLs for H3K4me1 and H3K4me3 are from (8). (D) Functional context of eQTL SNPs that are not associated with chromatin changes (“unexplained”) versus those eQTLs that are also chromatin QTLs. 5′ untranslated regions were excluded from the “gene exons” annotation. Five annotations with bootstrap P > 0.05 are not shown. (E) Summary of the effects of regulatory QTLs and of their sharing through the regulatory cascade.
The high correlations of QTL effect sizes across regulatory stages suggest high proportions of shared QTLs. To quantify this, we considered the set of significant QTLs at each phenotype and estimated the sharing of QTLs at downstream stages of regulation (16) (Fig. 2C and fig. S2). Starting with enhancers, we observed that ~25% of H3K27ac QTLs [histone acetylation QTLs (haQTLs)] affect the expression levels of their nearest genes and ~50% affect the expression of any gene within 500 kb (fig. S2). In contrast, the majority of promoter haQTLs also affect expression (>65%) (Fig. 2B).
When we used the same approach starting at transcription, we found that over 85% of QTLs were shared from one regulatory stage to the next, from 4sU to protein (Fig. 2B and fig. S2E). Using a Bayesian model to quantify QTL sharing among traits, we estimated that 73% of QTLs that affect transcription rates also affect protein expression (16) (fig. S3). These observations are consistent with a general percolation of genetic effects from transcription through the regulatory cascade; however, it remains possible that some QTLs might affect multiple aspects of posttranscriptional regulation independently.
We next examined how often eQTLs can be explained by inter-individual variation in chromatin properties (Fig. 2C), by estimating the fraction of eQTLs that are also QTLs for a chromatin-level trait, such as DNaseI-sensitive sites, DNA methylation, H3K27ac, and H3K4me1 and H3K4me3 QTLs as determined in (8). We confirmed that the majority of eQTLs are also nearby chromatin QTLs (65 versus 20% for matched control SNPs), which is consistent with previous reports (4).
Thus ~35% of eQTLs are not associated with known chromatin QTLs. To investigate whether these represent a distinct functional class, we asked whether the unexplained eQTLs are biased toward particular genomic regions or functional annotations (Fig. 2D). Indeed, compared to eQTLs that also affect chromatin, the chromatin-independent eQTLs were enriched within gene bodies (exons: P = 5.4 × 10−8; and introns: P = 0.006; Mann-Whitney U test). Further, there is particular enrichment in regions associated with transcriptional elongation marks (P = 3.0 × 10−21; Mann-Whitney U test). Hence, many of the unexplained eQTLs may affect transcriptional or posttranscriptional processes, alongside the more widely studied effects on chromatin function. Together, these analyses help us provide an overview of the genetic effects on diverse aspects of gene regulation (Fig. 2E).
We next turned to the effects of genetic variation on pre-mRNA splicing (Fig. 3). Most studies of splicing QTLs (sQTLs) have measured either expression levels of individual exons or of transcript isoforms (6, 14, 17,). Because both of these are difficult to estimate accurately from short-read data, we developed a new method to detect splicing variation, LeafCutter (20), which focuses on reads that span splice junctions (16). Using this approach, we identified 2893 sQTLs in 2313 genes at 10% false discovery rate (FDR) (16).
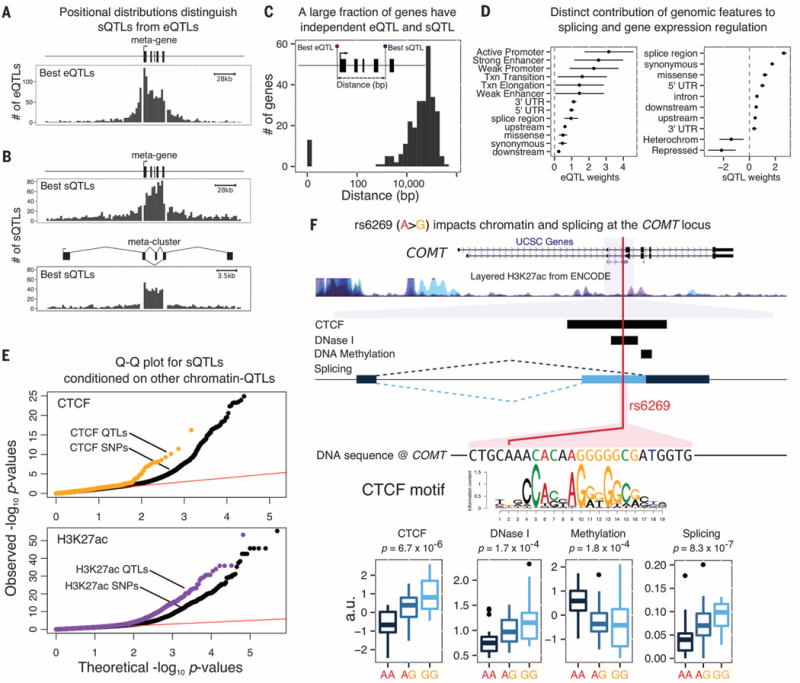
Properties of sQTLs. Most sQTLs act independently from eQTLs: Positional distributions of (A) eQTLs and (B) sQTLs at 5% FDR are consistent with our mechanistic understanding of gene transcription and splicing. (C) The distance between the best eQTL and best sQTL for genes with both types of QTL is typically large, suggesting distinct causal variants. (D) A hierarchical model reveals distinct genomic features that are most relevant for eQTLs and sQTLs, respectively. (E) QTLs for CTCF binding, and H3K27ac levels are more likely to be sQTLs than matched SNPs within CTCFand H3K27ac ChIP-seq peaks, respectively. (F) Example of an sQTL (rs6269) that is also a QTL for CTCF, DNaseI sensitivity, and DNA methylation. The allele that is associated with increased CTCF occupancy is also associated with increased use of an alternative upstream splice site for an exon of the catechol-O-methyltransferase gene, COMT, which is consistent with the model that PolII pausing at CTCF binding sites can promote upstream exon inclusion (21). COMT, which regulates dopamine, has possible roles in neuropsychiatric conditions (25). In Europeans, the sQTL is in nearly complete linkage disequilibrium with a missense variant, rs4680, which has been the main focus of attention to date.
We verified that sQTLs and eQTLs tend to be independent. Unlike eQTLs, which are enriched near TSSs, the sQTLs are enriched within gene bodies and in particular within the introns they regulate (Fig. 3, A and B). Moreover, most of the sQTLs are not associated with gene expression levels (74% have P values >10−2, t test; fig. S9).
We examined 275 genes associated with both an sQTL and an eQTL at 10% FDR. The lead eQTL and sQTL SNP is the same for only 14 of these genes. In most cases, the lead SNPs are more than 10 kb apart, suggesting that the majority of these effects are independent (Fig. 3C and fig. S12). Although most sQTLs do not affect overall expression, the majority (89%) affect the predicted coding sequences, thereby potentially affecting protein function (fig. S10).
We used a hierarchical model to identify which genomic annotations are most relevant to splicing versus gene expression (16). As expected, genetic variants located in active promoters, strong enhancers, and weak promoters were most likely to affect gene expression. In contrast, splicing was most strongly affected by variants near splice sites and by synonymous and missense variants (Fig. 3D).
Although active chromatin does not seem to be the primary driver of sQTLs, DNA-binding proteins such as the CTCF transcription factor may affect transcription speed and thereby affect splicing, which occurs cotranscriptionally (21). sQTL SNPs show a modest enrichment for association with chromatin QTLs (17.5%) compared to matched control SNPs (13.3%). Overall, we identified 171 sQTLs associated with two or more chromatin-level phenotypes (table S7; example in Fig. 3F). Specifically considering QTLs for CTCF (16) and for H3K27ac, we found that chromatin QTLs are more likely to affect splicing than matched control SNPs (P = 2 × 10−5 and P = 1 × 10−34, respectively, likelihood-ratio test; Fig. 3E). Variations in CTCF binding and H3K27ac levels have been shown to correlate with differences in splicing (21, 22). Our findings, however, provide direct evidence that genetic variation can affect splicing by altering chromatin-level traits.
Our results indicate that splicing is a primary target of common genetic variation, which, in most cases, has direct effects on protein sequences. Although studies have implicated variants in active chromatin regions and eQTLs as contributing significantly to complex traits (2, 8, 10, 23), the importance of splicing remains unclear. We therefore wondered what role common sQTLs might play in complex diseases.
Owing to the extensive sharing of QTLs across cell types (2, 24), we reasoned that QTLs identified in LCLs should be informative about the relative contribution of different regulatory mechanisms to complex traits, in particular to immune-related diseases (8). We thus compiled genome-wide summary statistics for rheumatoid arthritis, multiple sclerosis, Alzheimer’s disease, schizophrenia, height, and body mass index (16). Using two tests with different underlying statistical models, we searched for functional annotations that are associated with GWAS signals (16, 23).
As expected, eQTLs and haQTLs are predicted to contribute to rheumatoid arthritis, multiple sclerosis, and height according to one or both methods (Fig. 4). Consistent with the notion that disease SNPs in histone modification peaks are mediated through the SNPs’ effect on chromatin, haQTLs are more enriched in risk loci than are variants that lie within H3K27ac peaks overall (Fig. 4C and fig. S14).
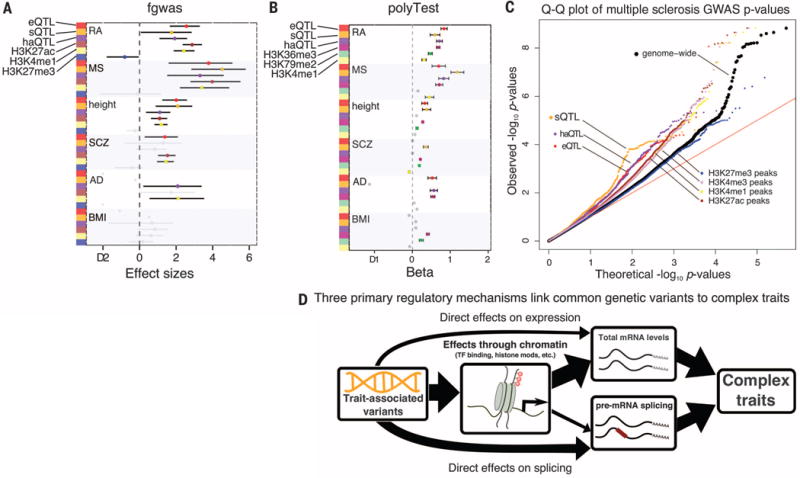
(A) Annotations identified with significant enrichment for GWAS traits by fgwas (23). (B) Annotations identified with significant enrichment for GWAS traits by polyTest (16). (C) Quantile-quantile (Q-Q) plot for multiple sclerosis GWAS suggests that splicing plays an important role in the etiology of multiple sclerosis. (D) Model of the regulatory mechanisms through which common variants affect complex traits.
sQTLs appear to have effects of similar or even larger magnitude than eQTLs. For instance, there is an enrichment of sQTLs with low P values in the multiple sclerosis GWASs, even when compared to eQTLs (Fig. 4C and fig. S15). These enrichments are robust to the eQTL and sQTL detection cutoffs, suggesting that they are not simply due to the power of detection (fig. S16). We also found similar patterns when we compared the effect of sQTLs on multiple sclerosis to the effects of eQTLs identified in three purified immune cell types (fig. S17).
In conclusion, three main pathways mediate the impact of genetic variation on gene regulation with phenotypic and pathogenic consequences. Of these, our work uncovers an unexpectedly important role of RNA splicing in modulating phenotypic traits (Fig. 4D). These findings indicate that RNA splicing should be a focal point in future work on connecting genetic variation to complex disease.
Acknowledgments
We thank S. Prabhakar for early access to the H3K27ac data; J. Blischak for technical assistance with the 4sU experiments; the anonymous reviewers for helpful comments; and A. Battle, A. Pai, N. Banovich, A. Fu, X. Lan, A. Harpak, and other members of the Pritchard/Gilad Labs for helpful discussions. This work was supported by NIH grants R01MH084703, RO1MH101825, U01HG007036, and U54CA149145; by a Center for Computational, Evolutionary and Human Genomics Fellowship; and by the Howard Hughes Medical Institute. The 4sU-seq data have been deposited in the Gene Expression Omnibus (www.ncbi.nlm.nih.gov/geo/) under accession no. GSE75220; other accession numbers can be found in table S8.
Footnotes
SUPPLEMENTARY MATERIALS
www.sciencemag.org/content/352/6285/600/suppl/DC1
Materials and Methods
References (26–53)
REFERENCES AND NOTES
Full text links
Read article at publisher's site: https://doi.org/10.1126/science.aad9417
Read article for free, from open access legal sources, via Unpaywall:
https://science.sciencemag.org/content/sci/352/6285/600.full.pdf
Citations & impact
Impact metrics
Citations of article over time
Alternative metrics
Article citations
A compendium of genetic variations associated with promoter usage across 49 human tissues.
Nat Commun, 15(1):8758, 09 Oct 2024
Cited by: 1 article | PMID: 39384785 | PMCID: PMC11464533
Full-length transcriptome assembly of black amur bream (Megalobrama terminalis) as a reference resource.
Mol Biol Rep, 51(1):1101, 29 Oct 2024
Cited by: 0 articles | PMID: 39470845
The Role of RNA Splicing in Liver Function and Disease: A Focus on Metabolic Dysfunction-Associated Steatotic Liver Disease.
Genes (Basel), 15(9):1181, 08 Sep 2024
Cited by: 0 articles | PMID: 39336772 | PMCID: PMC11431473
Review Free full text in Europe PMC
From computational models of the splicing code to regulatory mechanisms and therapeutic implications.
Nat Rev Genet, 02 Oct 2024
Cited by: 0 articles | PMID: 39358547
Review
Deciphering the impact of genomic variation on function.
Nature, 633(8028):47-57, 04 Sep 2024
Cited by: 3 articles | PMID: 39232149
Review
Go to all (331) article citations
Other citations
Data
Data behind the article
This data has been text mined from the article, or deposited into data resources.
BioStudies: supplemental material and supporting data
SNPs
- (1 citation) dbSNP - rs4680
Similar Articles
To arrive at the top five similar articles we use a word-weighted algorithm to compare words from the Title and Abstract of each citation.
DNase I sensitivity QTLs are a major determinant of human expression variation.
Nature, 482(7385):390-394, 05 Feb 2012
Cited by: 429 articles | PMID: 22307276 | PMCID: PMC3501342
Genetic control of RNA splicing and its distinct role in complex trait variation.
Nat Genet, 54(9):1355-1363, 18 Aug 2022
Cited by: 49 articles | PMID: 35982161 | PMCID: PMC9470536
Genetic analyses support the contribution of mRNA N6-methyladenosine (m6A) modification to human disease heritability.
Nat Genet, 52(9):939-949, 29 Jun 2020
Cited by: 94 articles | PMID: 32601472 | PMCID: PMC7483307
Expression Quantitative Trait Loci Information Improves Predictive Modeling of Disease Relevance of Non-Coding Genetic Variation.
PLoS One, 10(10):e0140758, 16 Oct 2015
Cited by: 15 articles | PMID: 26474488 | PMCID: PMC4608673
Review Free full text in Europe PMC
Funding
Funders who supported this work.
Center for Computational, Evolutionary and Human Genomics Fellowship
Howard Hughes Medical Institute
NCI NIH HHS (2)
Grant ID: U54 CA149145
Grant ID: U54CA149145
NHGRI NIH HHS (2)
Grant ID: U01HG007036
Grant ID: U01 HG007036
NIH (4)
Grant ID: RO1MH101825
Grant ID: U01HG007036
Grant ID: U54CA149145
Grant ID: R01MH084703
NIMH NIH HHS (4)
Grant ID: R01 MH101825
Grant ID: R01MH084703
Grant ID: R01 MH084703
Grant ID: R01MH101825