Abstract
Background
Treatment with immune checkpoint blockade (ICB) with agents such as anti-programmed cell death protein 1 (PD-1), anti-programmed death-ligand 1 (PD-L1), and/or anti-cytotoxic T-lymphocyte-associated protein 4 (CTLA-4) can result in impressive response rates and durable disease remission but only in a subset of patients with cancer. Expression of PD-L1 has demonstrated utility in selecting patients for response to ICB and has proven to be an important biomarker for patient selection. Tumor mutation burden (TMB) is emerging as a potential biomarker. However, refinement of interpretation and contextualization is required.Materials and methods
In this review, we outline the evolution of TMB as a biomarker in oncology, delineate how TMB can be applied in the clinic, discuss current limitations as a diagnostic test, and highlight mechanistic insights unveiled by the study of TMB. We review available data to date studying TMB as a biomarker for response to ICB by tumor type, focusing on studies proposing a threshold for TMB as a predictive biomarker for ICB activity.Results
High TMB consistently selects for benefit with ICB therapy. In lung, bladder and head and neck cancers, the current predictive TMB thresholds proposed approximate 200 non-synonymous somatic mutations by whole exome sequencing (WES). PD-L1 expression influences response to ICB in high TMB tumors with single agent PD-(L)1 antibodies; however, response may not be dependent on PD-L1 expression in the setting of anti-CTLA4 or anti-PD-1/CTLA-4 combination therapy. Disease-specific TMB thresholds for effective prediction of response in various other malignancies are not well established.Conclusions
TMB, in concert with PD-L1 expression, has been demonstrated to be a useful biomarker for ICB selection across some cancer types; however, further prospective validation studies are required. TMB determination by selected targeted panels has been correlated with WES. Calibration and harmonization will be required for optimal utility and alignment across all platforms currently used internationally. Key challenges will need to be addressed before broader use in different tumor types.Free full text

Development of tumor mutation burden as an immunotherapy biomarker: utility for the oncology clinic
Abstract
Background
Treatment with immune checkpoint blockade (ICB) with agents such as anti-programmed cell death protein 1 (PD-1), anti-programmed death-ligand 1 (PD-L1), and/or anti-cytotoxic T-lymphocyte-associated protein 4 (CTLA-4) can result in impressive response rates and durable disease remission but only in a subset of patients with cancer. Expression of PD-L1 has demonstrated utility in selecting patients for response to ICB and has proven to be an important biomarker for patient selection. Tumor mutation burden (TMB) is emerging as a potential biomarker. However, refinement of interpretation and contextualization is required.
Materials and methods
In this review, we outline the evolution of TMB as a biomarker in oncology, delineate how TMB can be applied in the clinic, discuss current limitations as a diagnostic test, and highlight mechanistic insights unveiled by the study of TMB. We review available data to date studying TMB as a biomarker for response to ICB by tumor type, focusing on studies proposing a threshold for TMB as a predictive biomarker for ICB activity.
Results
High TMB consistently selects for benefit with ICB therapy. In lung, bladder and head and neck cancers, the current predictive TMB thresholds proposed approximate 200 non-synonymous somatic mutations by whole exome sequencing (WES). PD-L1 expression influences response to ICB in high TMB tumors with single agent PD-(L)1 antibodies; however, response may not be dependent on PD-L1 expression in the setting of anti-CTLA4 or anti-PD-1/CTLA-4 combination therapy. Disease-specific TMB thresholds for effective prediction of response in various other malignancies are not well established.
Conclusions
TMB, in concert with PD-L1 expression, has been demonstrated to be a useful biomarker for ICB selection across some cancer types; however, further prospective validation studies are required. TMB determination by selected targeted panels has been correlated with WES. Calibration and harmonization will be required for optimal utility and alignment across all platforms currently used internationally. Key challenges will need to be addressed before broader use in different tumor types.
Introduction
Currently, immune checkpoint blockade (ICB) therapy has increased the overall survival (OS) rates of patients with advanced melanoma, non-small-cell lung cancer (NSCLC), urothelial cancer (UC), renal cell carcinoma (RCC), and other cancer types [1–8]. Tumors often upregulate immune checkpoints to avoid being detected and killed by the host immune system. Activation of checkpoint cascades such as those controlled by programmed cell death protein (PD-1) or CTLA-4 result in inactivation of tumor-specific T cells and immune evasion [9–12]. Treatment with anti-PD-1, anti-programmed death-ligand 1 (anti-PD-L1), or anti-CTLA-4 reinvigorates T cells and allows the adaptive immune system to target tumor cells [13, 14]. Detection of tumor and/or immune cell PD-L1 by immunohistochemical measurement has been extensively studied as a predictor of response to anti-PD(L)-1 treatment and has been convincingly demonstrated to be a valid biomarker in some settings. PD-L1 expression by immunohistochemistry (IHC) is an Food and Drug Administration (FDA)-approved companion diagnostic test for pembrolizumab in NSCLC, gastric/gastroesophageal junction adenocarcinoma, cervical cancer and UC [15–20], and has shown some predictive ability across several other cancer types including head and neck and small-cell lung carcinoma [21–23].
PD-L1 quantitation for immunotherapy response prediction is imperfect and there is a need for improved biomarkers of response. The presence of tumor-infiltrating lymphocytes (TILs) might confer a prognostic and a predictive impact [24, 25]. The T-cell-inflamed gene expression profile (GEP) [26], immune gene expression signatures [27, 28], as well as description of the microbiome [29–31] also represent emerging predictive biomarkers.
Cancer is a genetic disease. Neoplastic transformation results from the accumulation of somatic mutations in the DNA of affected cells. These genetic alterations include driver mutations, mutations that directly affect tumor growth such as those in TP53, epidermal growth factor receptor (EGFR) or RAS, and passenger mutations, which are alterations that do not directly impact the growth of the cancer cell [32–34]. Genetic changes in tumors can include non-synonymous mutations largely comprised of missense mutations (point mutations that change the amino acid codon), synonymous mutations (silent mutations that do not alter amino acid coding), insertions or deletions (indels, which can cause frameshifts), and copy number gains and losses. There is dramatic variation in the frequency of each type of these genetic alterations between individual tumors and between different tumor types [35–38]. Tumor mutation burden (TMB) can be used to predict ICB efficacy and has since become a useful biomarker across many cancer types for identification of patients that will benefit from immunotherapy [39–42].
TMB and its relationship to neoantigens
A minority of somatic mutations in tumor DNA can give rise to neoantigens, mutation-derived antigens that are recognized and targeted by the immune system, especially after treatment with agents that activate T cells [39, 43–46]. These mutations can be transcribed and translated, and neoantigen-containing peptides can be processed by the antigen-processing machinery and loaded on to major histocompatibility complex (MHC) molecules for presentation on the cell surface. Importantly, however, not all mutations will generate neoantigens. In fact, only a minority of mutations generate peptides that are properly processed and loaded on to MHC complexes, and even fewer are able to be recognized by T cells [47, 48]. Therefore, not all neopeptides presented on the cell surface are immunogenic [48–50]. Importantly, however, the more somatic mutations a tumor has, the more neoantigens it is also likely to form, and TMB can represent a useful estimation of tumor neoantigenic load.
It is important to note that the presence of immunogenic neoantigens is not the only factor that influences the ability of T cells to recognize and kill tumor cells. Inactivating mutations in the antigen presentation pathway can occur, which can influence the ability of cells to present peptides to the immune system. Some immunologically relevant genes that can become mutated in cancers include JAK1, JAK2, B2M, STK11, and others [51, 52]. The presence of these alterations can modulate the overall effect of TMB or neoantigen load on ICB response.
Variation of TMB across tumor types
As TMB evolves as a relevant tool for the identification of patients likely to respond to ICB, a large effort has been made to characterize the type and the extent of TMB variation across tumor types and histologies. Over the last few years, a large number of studies have been able to map and characterize TMB variations across disease pathologies [37, 38, 53], documenting the highest levels of TMB in melanoma followed by NSCLCs and other squamous carcinomas, while leukemias and pediatric tumors show the lowest levels of TMB. Cancers such those of the breast, kidney, and ovary display intermediate levels of mutational load. In addition to the variation in the levels of TMB across tumor types, a significant TMB range is also observed within the same cancer type. Within NSCLCs, there is a high degree of variation in TMB across patients attributable to smoking status compared with gastric and breast cancers. Mutational signatures characterized by Stratton et al. have shed light on the origins of tumor somatic mutations [53]. For example, UV light and tobacco carcinogens are the dominant mutational processes in melanoma and NSCLC, respectively. Later in tumor evolution, mutations present in some cells but not others (so-called subclonal mutations) occur. In many tumor types, these mutations have been attributed to the APOBEC cytidine deaminase family and can also occur following cytotoxic chemotherapy in resistant emergent subclones [54–57]. The clonal nature of neoantigens has been shown to be relevant for T cell priming and responses to immune checkpoint inhibitors. Considering that intratumoral heterogeneity (ITH) can vary across tumor types [58] and can potentially impact antitumor immunity, it is important to consider ITH when analyzing TMB.
Variables defining TMB
Considering that high TMB correlates with a greater probability of displaying tumor neoantigens on HLA molecules on the surface of tumor cells [59, 60], it is rational to hypothesize that the tumors with the highest TMB are more likely to respond to ICB agents as this greater mutation load would increase the likelihood of recognition by neoantigen-reactive T cells. Consistent with this hypothesis, several studies have demonstrated an association between high TMB and response to anti-CTLA-4 in melanoma [39, 61] and anti-PD-1 in NSCLC [40]. Subsequent trials retrospectively analyzing TMB association with ICB treatment have been conducted in NSCLC, small-cell lung cancer (SCLC), and melanoma and have shown a correlation between TMB and ICB benefit [41, 61–65]. Figure 1 shows the evolution of TMB as an immunotherapy biomarker over the last several years. TMB-based assays are currently being considered by the FDA for approval as companion diagnostics for ICB agents.
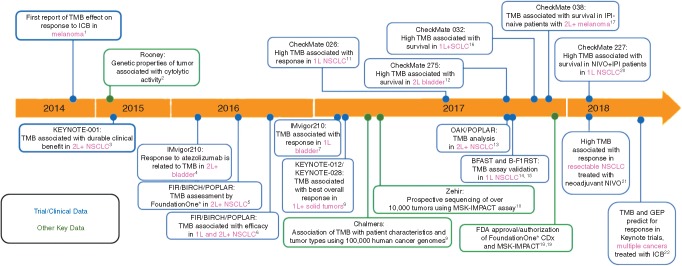
The evolution of tumor mutation burden as an immunotherapy biomarker. Major studies that are important in the development of TMB as a biomarker are shown. Color coding indicates type of study. The studies are ordered as a function of time, with the year indicated in the timeline. ICB, immune checkpoint blockade; 1L, first line; 2L, second line; +, and others; I-O, immune-oncology agent; IPI, ipilimumab; NIVO, nivolumab; NSCLC, non-small cell lung cancer; SCLC, small cell lung cancer; TMB, tumor mutational burden. 1. Snyder A et al. N Engl J Med 2014; 371(23): 2189–2199. 2. Rooney MS et al. Cell 2015; 160(1–2): 48–61. 3. Rizvi NA et al. Science 2015; 348(6230): 124–128. 4. Rosenberg JE et al. Lancet 2016; 387(10031): 1909–1920. 5. Kowanetz M et al. Poster presentation at ESMO 2016. Abstract 77P. 6. Kowanetz M et al. Oral presentation at WCLC 2016. Abstract 6149. 7. Balar AV et al. Lancet 2017; 389(10064): 67–76. 8. Seiwert TW et al. J Clin Oncol 2018; 36(suppl 5S; abstract 25). 9. Chalmers ZR et al. Genome Med 2017; 9(1): 34. 10. Zehir A et al. Nat Med 2017; 23(6): 703–713. 11. Carbone DP et al. N Engl J Med 2017; 376(25): 2415–2426. 12 Galsky MD et al. Poster presentation at ESMO 2017. Abstract 848PD. 13. Gandara DR et al. Oral presentation at ESMO 2017. Abstract 1295O. 14. Fabrizio DA et al. Poster presentation at ESMO 2017. Abstract 102P. 15. Mok T et al. Poster presentation at ESMO 2017. Abstract 1383TiP. 16. Antonia SJ et al. Oral presentation at WCLC 2017. Abstract 11063. 17. Riaz N et al. Cell 2017; 171(4): 934–949. 18. Foundation Medicine. http://investors.foundationmedicine.com/releasedetail.cfm?ReleaseID=1050380 (11 December 2017, date last accessed). 19. US Food and Drug Administration. https://www.fda.gov/NewsEvents/Newsroom/PressAnnouncements/ucm585347.htm (1 December 2017, date last accessed). 20. Hellmann MD et al. N Engl J Med 2018, 10.1056/NEJMoa1801946. 21. Forde PM et al. N Engl J Med 2018, 10.1056/NEJMoa1716078. 22. Cristescu et al. Science 2018; 362(6411).
For the initial studies, TMB was determined by whole exome sequencing (WES) carried out on tumor DNA and matching normal DNA. Normal germline variations in DNA sequence between individuals must be identified and removed from consideration in order to tabulate only the somatic alterations, a process that has been well-established [66, 67]. TMB is usually reported as the total number of coding and somatic mutations, but in some cases, can also include insertions and deletions (indels). Exonic TMB is theoretically best measured by WES because this technique samples the entire exome. However, TMB by WES is not yet routinely used as a clinical tool for predicting response to ICB and is used for research only at this time largely due to its greater cost and complexity.
Clinical WES is offered in Clinical Laboratory Improvement Amendments (CLIA)-approved settings and active development to bring these tests into the clinic is ongoing. Recent efforts have begun to validate targeted NGS panels against WES data as these panels are already being used routinely in clinic for oncogenic mutation detection [37, 68]. With the Foundation Medicine (FM) NGS approach (F1CDx), TMB was defined as the number of base substitutions (including synonymous mutations) in the coding region of targeted genes. Germline DNA was not sequenced but filtering for both oncogenic driver events and germline status was carried out using public and private variant databases. The total mutations/megabase (mut/Mb) calculation included both synonymous and non-synonymous mutations requiring a bridging formula for conversion to number of missense mutations as determined by WES. The MSKCC NGS approach (MSK-IMPACT) tabulated non-synonymous mutations using sequencing data from both tumor and germline DNA (for variant calling). The most recent version of this panel sequences 468 genes covering 1.22 Mb. It has been shown that large targeted panels are sufficiently accurate for TMB estimation [37, 69] and panels tested to date (F1CDx and MSK-IMPACT), have demonstrated their predictive ability for ICB response [4, 37, 70–73] (Table 1). Both F1CDx and MSK-IMPACT have been approved by the US FDA.
Table 1.
Key parameters for some TMB assays
Parameter | WES | FM NGS (F1CDx) | MSKCC NGS (MSK-IMPACT) |
---|---|---|---|
No. of genes | ~22 000 gene coding regions | 324 cancer-related genes | 468 cancer-related genes |
Types of mutations captured | Coding missense mutations in tumor genome | Coding, missense, and indel mutations per Mb of tumor genome | Coding missense mutations per Mb of tumor genome |
Germline mutations | Subtracted using patient-matched normal samples | Estimated via bioinformatics algorithms and subtracted | Subtracted using patient-matched blood samples |
Capture region (tumor DNA) | ~30 Mb | 0.8 Mb | 1.22 Mb |
TMB definition | No. of somatic, missense mutations in the sequenced tumor genome | No. of somatic, coding mutations (synonymous and non-synonymous), short indels per Mb of tumor genome | No. of somatic, missense mutations per Mb of tumor genome |
WES, whole exome sequencing; FM, Foundation Medicine; NGS, next generation sequencing; Mb, megabase.
Several key variables need to be considered across platforms: depth of sequencing, length of sequencing reads, choice of aligners, variant callers, and filters used. They will all influence TMB measurement (overview provided in Table 1). Preanalytical factors—including sample collection and processing, input material quality and quantity, fixation methodology, and library preparation—affect the quantity and quality of DNA and thus shape TMB estimation values. For example, fixatives and fixation time are preanalytical factors that influence the degree of formaldehyde fixed-paraffin embedded (FFPE)-induced deamination artifacts, which impact analysis of TMB counts. Also, low tumor purity, which can result from sampling errors or a dense tumor microenvironment, may lead to reduced TMB assay sensitivity.
Sequence coverage and read depth differ between WES and targeted gene panel assays, with WES covering the entire exome coding region and targeted gene panels variably covering pre-specified territories of the exome or genome. Hence, the size and location of the interrogated region differs between targeted gene panel assays and requires careful consideration for accurate TMB assessment (Figure 2). For example, confidence intervals for TMB estimation increase with the use of gene panels that cover only a small region of the genome/exome compared with those that assess a larger area, suggesting that the use of small gene panels can considerably over- or underestimate TMB [69, 74]. While paired germline sequencing would reduce overall false positive mutation calls, a dual analysis is not always carried out. In the absence of such a comparison, large germline databases are needed to reduce false positive mutation calling and identify germline variants. Depth of sequencing also differs between WES and targeted gene panel assays; sequencing depth is much higher for targeted gene panels than for WES. Both coverage and sequencing depth can determine assay sensitivity and specificity, and therefore, influence TMB estimation output.
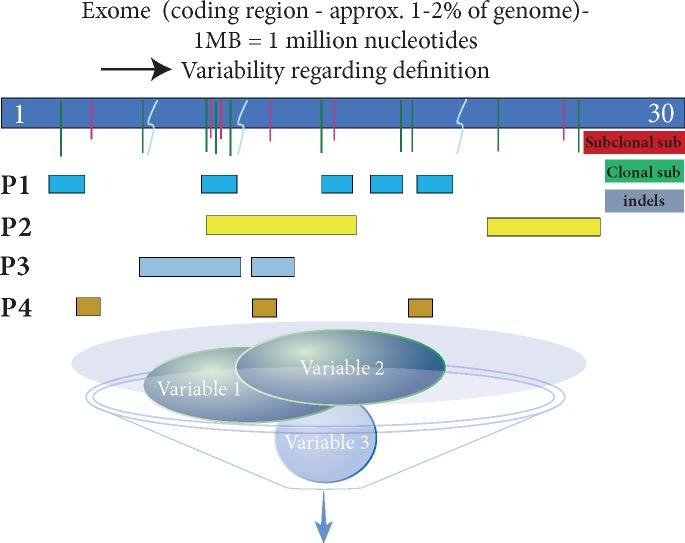
Target regions and sizes of four different hypothetical gene panels (P1–P4). Depending on the size and territory of the exome that is captured by P1–P4, respectively, TMB counts will differ. Other parameters, e.g. filtering of germline variants and cut points for allelic frequencies (blue circles), discussed in this review will influence TMB measurement further.
Bioinformatic algorithms strongly influence TMB estimation and reporting. As they differ widely across gene panels platforms, it is important that these specific procedures are transparent and open to the scientific and medical community. For example, the mutation types considered for TMB assessment can vary from one assay to another. These may include or exclude short insertions and deletions (indels) and/or synonymous and nonsynonymous base substitutions/single nucleotide variants. For most retrospective analyses that employed WES, TMB was calculated from missense mutations only, leaving out indels and other mutations, whereas some targeted approaches can include these variant types. Moreover, cut points and filtering algorithms for putative germline variants, variant allele frequency, and FFPE-induced deamination artifacts may vary between assays and can strongly impact TMB values. For example, variant allele frequencies cut-offs can vary from 0.5% to 10%, with lower thresholds increasing the risk of including false positives arising from sequencing artifacts such as C to T transitions introduced by formalin fixation.
TMB definition and correlation with response to ICB
Before exploration of TMB as a biomarker, the expression of PD-L1 in the tumor microenvironment was also being actively investigated as a biomarker and demonstrated some success in identifying patients most likely to benefit. PD-L1 quantitation and has been approved as a companion diagnostic for pembrolizumab in NSCLC [75]. PD-L1 expression as a biomarker has demonstrated an inconsistent record of enriching for response to ICB, which is related to the dynamic and heterogeneous expression of PD-L1 in the tumor microenvironment, assay interpretation, and a lack of standardization across PD-L1 platforms [76], warranting the exploration of new biomarkers for patient selection. TMB will also raise similar practical points, as mentioned previously for PD-L1, possibly with greater complexity and some concerns for accessibility. Additionally, data suggest that TMB will not replace other biomarkers such as IHC-based PD-L1 assessment, but possibly complement them. Importantly, PD-L1 and TMB have consistently been shown to represent independent, not correlated, predictive variables [42, 68, 77–79].
The Checkmate 026 trial in first-line NSCLC comparing nivolumab with standard of care (SOC) demonstrated no improvement in the primary end point of progression-free survival (PFS) in patients with PD-L1 expression ≥5% [42, 73]. Based on the emerging phase II TMB data, DNA sequencing of tumors from Checkmate 026 was carried out post hoc and yielded compelling results. Patients with high TMB defined as those with tumors with at least 243 missense mutations (the upper TMB tertile) were found to have significantly improved PFS with nivolumab treatment over SOC chemotherapy [hazard ratio (HR) 0.62]. A caveat to this result is that all patients that underwent this TMB analysis also had PD-L1 expression of ≥1%. Importantly, patients with mutations in the lower 2/3 (less than 243 mutations) had a worse PFS with nivolumab treatment compared with SOC (HR 1.82), demonstrating the importance of low TMB as a negative predictor of benefit in the context of effective SOC options. Similarly, in UC, a phase III trial in platinum-treated patients, IMvigor211, failed to meet its primary end point of OS improvement with atezolizumab compared with SOC chemotherapy. Even with pre-specified PD-L1 selection, no improvement in OS was demonstrated. Again, based on compelling phase II TMB data in UC, a post hoc analysis of TMB employing a threshold of 9.65 mut/Mb was conducted and showed a non-significant but numerically improved OS (HR 0.68). Strikingly, the subgroup of patients with both a high TMB and increased PD-L1 (IC2/3) had an HR of 0.50 with atezolizumab treatment [21].
Although TMB and PD-L1 do not co-associate in multiple trials [42, 80, 81], greater benefit with single agent anti-PD-1 and anti-PD-L1 is consistently observed with high TMB and PD-L1 expression, suggesting these independent biomarkers can be used in concert [82, 83]. Given the high response rates when combining PD-L1 with CTLA-4 blockade in initial solid tumor studies, there was speculation that patients with lower TMB tumors could benefit by the addition of anti-CTLA-4 to anti-PD-1 but this was not the case. In NSCLC, using a similar TMB threshold equivalent to ~200 missense mutations (10 mut/Mb), the benefit with combination ICB was independent of PD-L1 expression in the ≥10 mut/Mb patient population [62]. A similar benefit that was dependent on TMB but independent of PD-L1 expression was observed in SCLC with nivolumab and ipilimumab in combination [62, 63]. These data are further supported in a phase III NSCLC trial (Checkmate 227) comparing first-line nivolumab + ipilimumab with SOC chemotherapy [84]. This study showed a significantly improved PFS in high TMB versus SOC in both PD-L1 positive (HR 0.62) and negative (HR 0.48) patients. The above observations suggesting that TMB, and not necessarily PD-L1 status, associates with response to combination ICB (ipilimumab plus nivolumab) and is consistent with a scenario where tumors with high TMB are potentially immunogenic but T-cell infiltration and/or activation is controlled in a CTLA-4-dependent manner. This control can be either cell intrinsic by upregulation of CTLA-4 on effector T cells (Teff), or by trans-regulation via CTLA-4hi regulatory T cells (Treg). Lack of infiltration or T-cell activation would result in reduced IFN-γ within tumors, correlating with low or negative PD-L1 status at diagnosis. Upon CTLA-4-blockade or anti-CTLA-4-driven Treg depletion [85], re-activated effector T cells would upregulate PD-1 and promote PD-L1 upregulation, hence explaining the synergy with PD-1-blockade. Altogether, the previous supposition that PD-L1 positive, inflamed tumors respond to ICB but PD-L1 negative, non-inflamed tumors do not require adjudication based on the emerging data with TMB and combination immunotherapy (Figure 3).
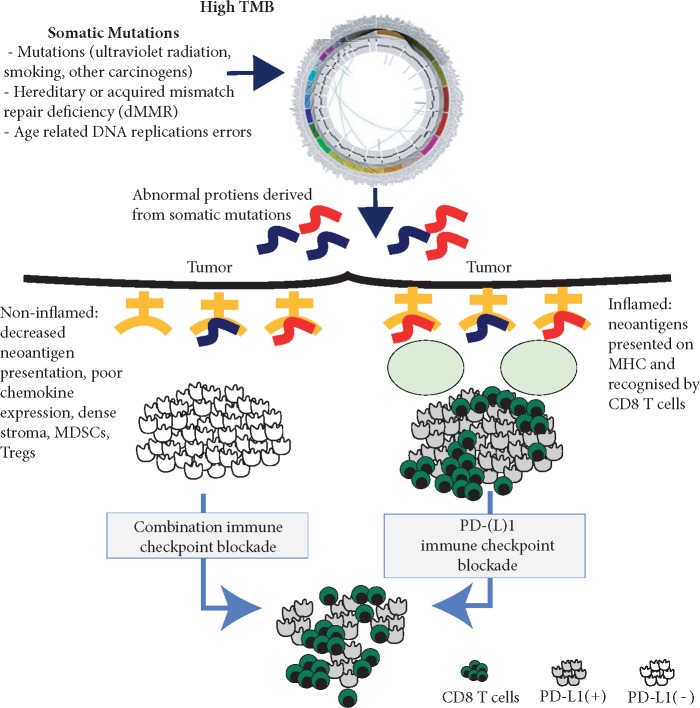
Mutations, neoantigens, and immune checkpoint blockade. Somatic mutations can generate neopeptides that are presented by MHC molecules. Both inflamed and non-inflamed tumors, as well as PD-L1 positive or negative tumors, can respond to immune checkpoint blockade therapy. TMB, tumor mutation burden; MMR, mismatch repair.
Interestingly, TMB has also shown predictive value in immunotherapy modalities other than immune checkpoint blockde therapy. Lauss et al. observed that tumor mutation and neoantigen load predicted improved PFS and OS for melanoma patients who were treated with adoptive T cell transfer therapy. This finding suggests that greater numbers of potential neoantigens in tumors may promote better clinical response to expanded and reinfused TILs [86].
Is TMB ready to enter the clinic?
The first FDA approval based on the concept of mutation burden was anti-PD1 therapy for patients with microsatellite instability-high (MSI-H) cancers. MSI is one of a number of defects in DNA repair that results in the accumulation of very high levels of TMB. In the initial reports of anti-PD1 therapy in which multiple different types of tumors were treated, only one of the 33 patients with colorectal cancer had an objective response to treatment [87, 88]. The discovery that this single responder had MSI-H colorectal cancer led to successful prospective clinical trials of pembrolizumab in adult and pediatric patients with MSI-H or DNA mismatch repair-deficient (dMMR) solid tumors, and the rapid approval of pembrolizumab in this biomarker-defined group of patients [89, 90]. Importantly, this was the first tissue-agnostic drug approval and the first approved companion biomarker assay for any cancer therapy by the FDA [91,92], and the approval foretells a future in which tumor genomic analyses can be used to personalize cancer immunotherapy. Although the majority of patients with MSI-H solid tumors also have a high TMB, only 16% of patients with high TMB tumors are MSI-H [37]. Whereas NSCLC might become one of the first indications for TMB application as a biomarker (as a variable itself rather than via a surrogate-like MMR deficiency), MSI-H is extremely rare in this entity.
Can the experience with MSI-H tumors be applied to MSI stable tumors? The median number of mutations in MSI-H tumors is often in the thousands [89, 91], whereas in microsatellite stable NSCLC, e.g. it is ~200 mutations. Furthermore, the aggregate data from multiple studies in SCLC, NSCLC, and UC approximate the TMB threshold required to enrich for benefit with ICB (at least in high TMB tumors) to reside at ~200 missense mutations, which is equivalent to 10 mut/Mb by FoundationOne testing or ~7 mut/Mb by MSK-IMPACT testing [63, 70, 71, 73]. Employing higher thresholds of selection to 16.2 mut/Mb with atezolizumab [80] and 15 mut/Mb with ipilimumab and nivolumab [71] in NSCLC did not improve efficacy. Given the consistent findings for these tumor types, one could envision the use of TMB selection in the clinic for patient decision-making. Checkmate 227 in first-line NSCLC was the first study to prospectively include PFS in high TMB (≥10 mut/Mb) patients as a co-primary end point. This trial demonstrated in TMB high patients randomized to ipilimumab and nivolumab versus chemotherapy a significant improvement in PFS (7.1 versus 3.2months) and response rate (45.3% versus 24.6%) [84]. A summary of the clinical data defining a TMB threshold with ICB treatment is presented in Table 2. Several ongoing clinical trials are using TMB as a key stratification factor or a landmark end point (Table 3). They will help decipher the role of TMB in the treatment decision process across cancer types and, by using distinct TMB thresholds and definitions, support a more refined definition of TMB utility.
Table 2.
Key trials defining a TMB threshold for ICB benefit
Cancer | Trial and treatment | Method | Threshold defined | RR | PFS | OS | Ref. |
---|---|---|---|---|---|---|---|
Melanoma | Anti-CTLA-4 | WES | 100 mutations | OS advantage | [39] | ||
Melanoma | CM 038 | WES | 100 mutations | OS advantage in ipilimumab naive | [64] | ||
Phase II nivolumab | |||||||
NSCLC | KN 001 phase I/II | WES | 200 mutations | 59% versus 12% | NR versus 3.4 months | [40] | |
Pembrolizumab | |||||||
NSCLC | BIRCH, FIR phase II | FM NGS | 9.9 mut/Mb | 25% versus 14% | HR 0.64 | HR 0.87 | [70] |
Atezolizumab | |||||||
NSCLC | POPLAR randomized phase II atezolizumab versus docetaxel | FM NGS | 9.9 mut/Mb | 20% versus 4% | 7.3 versus 2.8 months | 16.2 versus 8.3 months | [70] |
NSCLC | MSKCC: various immunotherapies | MSKCC NGS | 7.4 mut/Mb | 38.6% versus 25% | [68] | ||
NSCLC | CM 012 | WES | 158 mutations | 51% versus 13% | 17.1 versus 3.7 months | [62] | |
Nivolumab/ipilimumab | |||||||
NSCLC | CM 568 | FM NGS | 10 mut/Mb | 44% versus 12% | 7.1 versus 2.6 months | [71] | |
Nivolumab/ipilimumab | |||||||
SCLC | CM 032 phase II nivolumab | WES | 248 mutations | 46.2% versus 21.3% | 7.8 versus 1.4 months | 22 versus 5.4 months | [63] |
versus nivolumab/ipilimumab | |||||||
NSCLC | CM 026 randomized phase III nivolumab versus chemotherapy | WES | >243 mutations | 47% versus 23% | HR 0.62 | HR 1.10 | [42] |
NSCLC | CM 227 randomized phase III nivolumab/ipilimumab versus chemotherapy | FM NGS | >10 mut/Mb | 45.3% versus 24.6% | 7.1 versus 3.2 months | NA | [77] |
UC | CM 275 phase II | WES | ≥170 versus <85 mutations | 31.9% versus 10.9% | 3 versus 2 months | 11.63 versus 5.72 months | [78] |
Nivolumab | |||||||
UC | IMvigor210 phase II | FM NGS | 16 mut/Mb | OS advantage | [72] | ||
Atezolizumab | |||||||
UC | IMVigor211 phase III | FM NGS | >9.65 mut/Mb | HR 0.68 | [73] | ||
Atezolizumab versus chemotherapy | |||||||
Solid tumor | Various immunotherapies | FM NGS | 20 mut/Mb | 58% versus 20% | 12.8 versus 3.3 months | NR versus 16.3 months | [79] |
Solid tumor | KN 028 and KN 012 | WES | 102 mutations | 30% versus 7% | 109 versus 59 days | [81] | |
Pembrolizumab | |||||||
HNSCC | KN 012 and KN 055 pembrolizumab | WES | 175 mutations | HR 0.64 | HR 0.98 | [83] |
CM, checkmate; KN, keynote; NSCLC, non-small-cell lung cancer; SCLC, small-cell lung cancer; UC, urothelial cancer; HNSCC, head and neck squamous cell carcinoma; WES, whole exome sequencing; NGS, next generation sequencing; HR, hazard ratio; NA, not applicable; mut, mutation; FM, Foundation Medicine.
Table 3.
Ongoing clinical trials registered in ClinicalTrials.gov investigating immune checkpoint blockade in the context of TMB assessment
Trial name (NCT number) | Phase | Tumor type | Therapy | |
---|---|---|---|---|
1 | MK-3475-016 (NCT01876511) | II | MSI-positive or | Pembrolizumab |
MSI-negative CRC or other cancers | ||||
2 | PRO 02 | II | Advanced solid tumors | Multiple targeted therapies, including atezolizumab |
(NCT02091141) | ||||
3 | IMpower110 | III | NSCLC | Atezolizumab versus chemotherapy |
(NCT02409342) | ||||
4 | OpACIN (NCT02437279) | I | Melanoma | Adjuvant ipilimumab+nivolumab |
5 | CA209-260 | II | Melanoma or UC | Nivolumab±ipilimumab |
(NCT02553642) | ||||
6 | TAPUR (NCT02693535) | II | Advanced solid tumors | Multiple targeted therapies; including pembrolizumab and nivolumab+ipilimumab |
7 | AAAQ5450 | II | NSCLC | Pembrolizumab±chemotherapy |
(NCT02710396) | ||||
8 | NCI-2016-00666 | II | Desmoplastic melanoma | Pembrolizumab |
(NCT02775851) | ||||
9 | CheckMate 714 | II | SCCHN | Ipilimumab+nivolumab |
(NCT02823574) | ||||
10 | MultiVir Ad-p53-001 (NCT02842125) | I/II | Advanced solid tumors | Adenoviral p53+pembrolizumab/nivolumab or chemotherapy |
11 | B-F1RST (NCT02848651) | II | NSCLC | Atezolizumab |
12 | NCI-2016-01589 | II | NSCLC (EGFR-mutated) | Multiple, including nivolumab and pembrolizumab |
(NCT02949843) | ||||
13 | OpACIN-neo | II | Melanoma | Neoadjuvant ipilimumab+nivolumab |
(NCT02977052) | ||||
14 | NCI-2016-01698 (NCT02965716) | II | Melanoma | Pembrolizumab+talimogene laherparepvec (virus therapy) |
15 | PEER (NCT02990845) | I/II | Breast | Pembrolizumab+exemestane (aromatase inhibitor)+leuprolide (anti-GnRH) |
16 | ULTIMATE | II | Breast | Tremelimumab+durvalumab+exemestane (aromatase inhibitor) |
(NCT02997995) | ||||
17 | CL-PTL-126 | II | Gynecological cancers | Atezolizumab+vigil (immuno-stimulatory autologous cellular therapy) |
(NCT03073525) | ||||
18 | CA209-777 (NCT03091491) | II | NSCLC (EGFR mutant positive) | Nivolumab±ipilimumab |
19 | ISABR | I/II | NSCLC | Durvalumab+radiation |
(NCT03148327) | ||||
20 | CMIW815X2102J (NCT03172936) | 1 | Advanced solid tumors and lymphomas | PDR001 (anti-PD-1) + MIW815/ADU-S100 (IFN genes stimulator) |
21 | B-FAST | II/III | NSCLC | Atezolizumab versus chemotherapy |
(NCT03178552) | ||||
22 | KELLY (NCT03222856) | II | Breast (HR+/HER2− subtype) | Pembrolizumab+chemotherapy |
23 | RESPONDER | II | UC | Pembrolizumab |
(NCT03263039) | ||||
24 | IFG-NIB-01 (NCT03289819) | II | Breast (triple negative subtype) | Pembrolizumab+chemotherapy |
25 | NET-002 (NCT03278379) | II | Neuroendocrine | Avelumab |
26 | B9991023 | II | NSCLC, UC | Avelumab+chemotherapy |
(NCT03317496) | ||||
27 | CA209-929 | II | Breast, ovarian, gastric | Ipilimumab+nivolumab |
(NCT03342417) | ||||
28 | Javelin Parp Medley (NCT03330405) | Ib/II | Advanced solid tumors | Avelumab+talazoparib (anti-PARP) |
29 | R2810-ONC-1763 | II | NSCLC | Cemiplimab (anti-PD-1)±ipilimumab |
(NCT03430063) | ||||
30 | NIVES (NCT03469713) | II | RCC | Nivolumab+radiotherapy |
31 | Javelin Medley VEGF (NCT03472560) | II | NSCLC, UC | Avelumab+axitinib (TKI) |
32 | PERSEUS1 (NCT03506997) | II | Prostate | Pembrolizumab |
33 | ARETHUSA | II | CRC | Pembrolizumab, temozolomide |
(NCT03519412) | ||||
34 | KEYNOTE-495 | II | NSCLC | Pembrolizumab+lenvatinib (anti-VEGF) or MK-4280 (anti-LAG-3) |
(NCT03516981) | ||||
35 | MOVIE (NCT03518606) | I/II | Advanced solid tumors | Durvalumab+tremelimumab+chemotherapy |
36 | CIBI308A102 | I/II | Advanced solid tumors | Sintilimab (anti-PD-1) |
(NCT03568539) | ||||
37 | Ad-p53-002 (NCT03544723) | II | SCCHN | Ad-p53+nivolumab |
Some NGS assay providers have already nominated TMB thresholds for certain applications. For example, the 10 mut/Mb threshold (via F1CDx NGS assay) captures a significant fraction of cancer patients and may be particularly useful to identify cancer types with lower proportion of high TMB tumors, much as MSI testing can currently accomplish (Figure 4). However, applications to additional other tumor types will also require their own threshold validation. This must be examined before application to different malignancies. Furthermore, there is a need for harmonization of TMB reporting across different NGS assays currently in use and new NGS targeted assay platforms will also require their own validation.
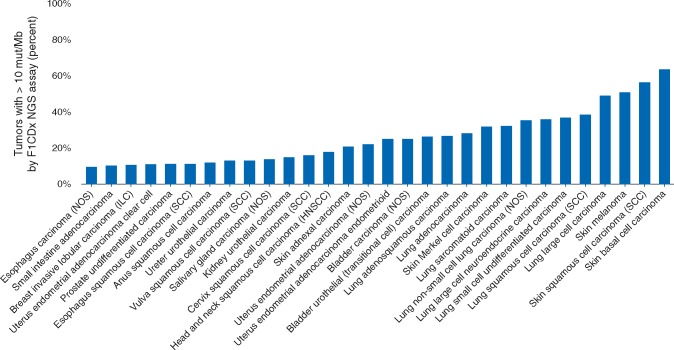
Impact of TMB pan-cancer: percent of solid tumors with TMB ≥10 mut/Mb. Analysis of top 30 solid tumor types selected from 104,814 total cases sorted by percent of cases with TMB ≥10 mut/Mb according to the Foundation Medicine database. TMB is defined as the number of somatic synonymous and non-synonymous base substitutions and indels divided by the region over which it was counted. Only cancer types with at least 100 total cases are reported. The average across all solid tumor types was 13.3%.
TMB limitations and perspectives
TMB is not without limitations. It is a relatively new type of biomarker, and defining standards for determination and reporting of TMB are not well established. Proteins generated from gene fusions and post-translational modifications of non-mutated proteins are not accounted for in current iterations of TMB, but nonetheless may contribute to neoantigenic load. More critically, current iterations of the TMB assign an equal weight to each tumor mutation, but it is increasingly clear that not all mutations are created equal [53, 56]. Some mutations result in the formation of higher ‘quality’ antigens, which are more readily identified as ‘non-self’ by the immune system and are more likely to induce a robust antitumor immune response. Antigens resulting from viral open reading frames in a cancer’s genome are an example of a high-quality antigen. This may be the reason the subset of Merkel-cell carcinoma that is associated with the Merkel-cell polyomavirus has a moderate TMB [93] but amongst the highest response rates of any tumor type with anti-PD1 therapy [94].
Another example of a tumor type with intermediate levels of TMB but a high response rate to ICB is RCC [3, 95, 96]. Recent work by Turajlic et al. shows that in addition to single nucleotide variants, frameshift mutations generated by insertion and deletions that result in the generation of an entirely new peptide amino acid chain before a stop codon being reached, also contribute to the generation of potent tumor neoantigens and the overall TMB of cancers [97]. Interestingly, they demonstrated that RCCs have the highest frequency and number of indel mutations across cancer types. In MSI tumors, genetic instability manifests as short indels resulting from lack of repair of slippages during replication. This, in MMR deficient tumors, indels may also need to be considered in defining total TMB.
Another challenge is to understand how to use TMB while taking into account specific mutations that have been shown to influence response to ICB treatment. For example, mutations in genes such as JAK1, JAK2, β2M, SKT11, SERPINB3, and SERPINB4 have been shown to affect ICB response [51, 98]. Some mutations such as those in JAK1 and JAK2 are rare and do not validate in all patient cohorts [64, 99]. Similarly, some immune evasion mechanisms such as transforming growth factor β (TGF-β) signaling [100] or indoleamine 2,3-dioxygenase (IDO) activity may influence ICB response [101]. The importance of these alterations will have to be tested in prospective trials. For the variables that are currently validated as most useful, a model taking into consideration TMB, individual mutations or pathways that affect ICB outcomes, and PD-L1 levels—perhaps in the form of a nonogram—could be developed to further improve predictive models. Similar models are in use for predicting the likelihood of disease control in patients with prostate cancer and for quantifying benefit from chemotherapy for breast cancer patients [102–104]. It should be noted that the use of expression signatures have had a checkered past in the cancer biomarker field. Despite thousands of expression signatures nominated for use as biomarkers, very few have found reliable use in the clinic, especially when the expression signatures do not correlate with reproducible genetic alterations. Therefore, use of expression signatures in the immuno-oncology setting needs to be carefully vetted. Indeed, the history of cancer biomarker development suggests that genetic alterations and not simply altered expression of a given target or pathway of interest, which can often be reversible, are more robust predictors of response to a therapy targeting that pathway. Despite expression of IDO1 in tumors, genetic evidence that IDO is a cancer driver is lacking. It is perhaps not surprising, then, that a recent large phase III trial testing an IDO inhibitor in combination with anti-PD-1 did not shown benefit [105], leading to the widespread discontinuation of IDO inhibitor development. However, some expression signatures appear to be promising for detection of successful anticancer immunity. Interestingly, Cristescu et al. show that TMB and a T-cell inflamed gene expression signature can both provide predictive value for clinical response in patients treated on four Keynote trials [26]. Furthermore, the utility of TMB and other biomarkers noted above in patients treated with ICB plus chemotherapy is unclear and will need to be studied. If TMB is predictive in these settings, it is likely that new thresholds may need to be established.
Regardless, building future algorithms for identifying patients that will benefit from ICB will likely require assessment of tumor and immune cells qualitatively and quantitatively. TMB, specific mutations in oncogenes, as well as PD-L1 expression will describe the tumor component while immune cell PD-L1 expression, HLA genotype, TCR repertoire, and possibly immune signatures (as determined, e.g. by gene expression analysis) might be taken into account for the immune component of response.
Discussion
Conclusions
The relationship between TMB and response to immune checkpoint inhibitors is paving the way towards a precision immuno-genetics approach to cancer treatment. From the initial clinical observations associating tumors with genetic damage from environmental factors, we have begun a journey of discovery that will greatly broaden the scope and practice of precision oncology. TMB and other genetic determinants of response to immunotherapy have already provided exciting new avenues to make cancer treatment more precise. Nevertheless, challenges remain. Our knowledge of how genetics shapes immune response in unclear and this gap in knowledge must be bridged in order to build even better predictive models. How TMB can be used in combination with PD-L1 quantitation or measures of tumor inflammation needs to be improved. Moreover, the impact of how HLA genotype and other germline variations influences the effect of TMB and response to ICB needs to be explored further [106]. Lastly, as discussed above, we highlight the need for cross-assay standardization of NGS methods and solidification of interpretation of TMB levels in order to ensure reliable treatment decisions in the clinic based on tumor genetics.
Acknowledgements
We thank Naiyer Rizvi and the Chan laboratory for excellent discussions. We thank Dr Vincent Miller at Foundation Medicine for helpful discussions.
Funding
TAC is supported in part by NIH/NCI Cancer Center Support Grant P30 CA008748, NIH R35 CA232097, and the Mellnikoff Precision Immunotherapy Kidney Cancer Fund. MY acknowledges research grants from Exelixis, Merck, and Bristol-Myers Squibb. AS reports research funding from Chugai and BMS. SP has received grants/research support from the following: (sub)investigator in trials sponsored by Amgen, AstraZeneca, Boehringer-Ingelheim, Bristol-Myers Squibb, Clovis, F. Hoffmann-La Roche, Illumina, Merck Sharp and Dohme, Merck Serono, Novartis, and Pfizer. No additional grant funding is applicable.
Disclosure
EJ has licensed GVAX/Listeria vaccines to AduroBiotech with potential to receive royalties. EJ is on the Genocea, Adaptive Biotech, DragonFly and CSTONE SABs. EJ acknowledges grants from Aduro Biotech, BMS, Amgen and Hertig. TAC is a co-founder of Gritstone Oncology and holds equity. TAC holds equity in An2H. TAC acknowledges grant funding from Bristol-Myers Squibb, AstraZeneca, Illumina, Pfizer, An2H, and Eisai. TAC has served as a paid advisor for Bristol-Myers Squibb, Illumina, Eisai, and An2H. MSK has licensed the use of TMB for the identification of patients that benefit from immune checkpoint therapy to PGDx. MSK, RMS, DC, LGTM, and TAC receive royalties as part of this licensing agreement. MY is a paid advisor for Eisai and received research support from Genentech/Roche. AS acknowledges advisory board honoraria from Bayer, BMS, AstraZeneca, Novartis, ThermoFisher, Illumina. AS reports speaker’s honoraria from BMS, Bayer, MSD, Roche, Illumina, AstraZeneca, Novartis, ThermoFisher, Takeda. SP acknowledges receipt of honoraria or consultation fees from: Abbvie, Amgen, AstraZeneca, Bayer, Biocartis, Boehringer-Ingelheim, Bristol-Myers Squibb, Clovis, Daiichi Sankyo, Debiopharm, Eli Lilly, F. Hoffmann-La Roche, Foundation Medicine, Illumina, Janssen, Merck Sharp and Dohme, Merck Serono, Merrimack, Novartis, Pharma Mar, Pfizer, Regeneron, Sanofi, Seattle Genetics and Takeda. SP has given lectures in the following company’s organized public events: AstraZeneca, Boehringer-Ingelheim, Bristol-Myers Squibb, Eli Lilly, F. Hoffmann-La Roche, Merck Sharp and Dohme, Novartis, Pfizer, Takeda. SP has been (sub)investigator in trials (institutional financial support for clinical trials) sponsored by Amgen, AstraZeneca, Boehringer-Ingelheim, Bristol-Myers Squibb, Clovis, F. Hoffmann-La Roche, Illumina, Merck Sharp and Dohme, Merck Serono, Novartis, and Pfizer. SAQ is a scientific founder and paid scientific advisor of Achilles Therapeutics. SAQ is also a scientific advisor to F. Hoffmann-La Roche, BBT, Bicycle therapeutics, Morphosys and Ioncturas. CS discloses receipt of grants/research support from Pfizer, AstraZeneca, BMS, Ventana; receipt pf honoraria or consultancy fees by Boehringer Ingelheim, Eli Lily, Servier, Novartis, Roche-Genentech, GlaxoSmithKline, Pfizer, BMS, Celgene, AstraZeneca, Illumina, Sarah Cannon Research Institute; stock options/shareholders of Apogen Biotechnologies, Epic Bioscience, GRAIL and has stock options and is co-founder of Achilles therapeutics and patents for method for treating cancer: PCT/EP2016/059401, immune checkpoint intervention in cancer: PCT/EP2016/071471; method: PCT/GB2018/051893; method: PCT/GB2018/051892; method: PCT/GB2018/052004.
References
Articles from Annals of Oncology are provided here courtesy of Oxford University Press
Full text links
Read article at publisher's site: https://doi.org/10.1093/annonc/mdy495
Read article for free, from open access legal sources, via Unpaywall:
http://www.annalsofoncology.org/article/S0923753419309974/pdf
Citations & impact
Impact metrics
Citations of article over time
Alternative metrics
Smart citations by scite.ai
Explore citation contexts and check if this article has been
supported or disputed.
https://scite.ai/reports/10.1093/annonc/mdy495
Article citations
Genetic Alterations in Chromatin Regulatory Genes in Upper Tract Urothelial Carcinoma and Urothelial Bladder Cancer.
Cancer Med, 13(21):e70398, 01 Nov 2024
Cited by: 0 articles | PMID: 39513266 | PMCID: PMC11544325
Construction of a tumor mutational burden-derived LncRNA prognostic computational framework associated with therapy sensitivity in skin cutaneous melanoma.
J Transl Med, 22(1):966, 24 Oct 2024
Cited by: 0 articles | PMID: 39449143 | PMCID: PMC11515383
Comprehensive pan-cancer analysis of FUTs family as prognostic and immunity markers based on multi-omics data.
Discov Oncol, 15(1):567, 16 Oct 2024
Cited by: 0 articles | PMID: 39414693 | PMCID: PMC11485001
Pan-cancer analysis and experimental validation of FPR3 as a prognostic and immune infiltration-related biomarker for glioma.
Front Genet, 15:1466617, 09 Oct 2024
Cited by: 0 articles | PMID: 39445161 | PMCID: PMC11496095
Unraveling the potential biomarkers of immune checkpoint inhibitors in advanced ovarian cancer: a comprehensive review.
Invest New Drugs, 21 Oct 2024
Cited by: 0 articles | PMID: 39432145
Review
Go to all (1,400) article citations
Data
Data behind the article
This data has been text mined from the article, or deposited into data resources.
BioStudies: supplemental material and supporting data
Clinical Trials (Showing 37 of 37)
- (1 citation) ClinicalTrials.gov - NCT03278379
- (1 citation) ClinicalTrials.gov - NCT02965716
- (1 citation) ClinicalTrials.gov - NCT03469713
- (1 citation) ClinicalTrials.gov - NCT02848651
- (1 citation) ClinicalTrials.gov - NCT02091141
- (1 citation) ClinicalTrials.gov - NCT02823574
- (1 citation) ClinicalTrials.gov - NCT01876511
- (1 citation) ClinicalTrials.gov - NCT03518606
- (1 citation) ClinicalTrials.gov - NCT03472560
- (1 citation) ClinicalTrials.gov - NCT02437279
- (1 citation) ClinicalTrials.gov - NCT03091491
- (1 citation) ClinicalTrials.gov - NCT02409342
- (1 citation) ClinicalTrials.gov - NCT02997995
- (1 citation) ClinicalTrials.gov - NCT02710396
- (1 citation) ClinicalTrials.gov - NCT03073525
- (1 citation) ClinicalTrials.gov - NCT03263039
- (1 citation) ClinicalTrials.gov - NCT03506997
- (1 citation) ClinicalTrials.gov - NCT03317496
- (1 citation) ClinicalTrials.gov - NCT02990845
- (1 citation) ClinicalTrials.gov - NCT03148327
- (1 citation) ClinicalTrials.gov - NCT02693535
- (1 citation) ClinicalTrials.gov - NCT02977052
- (1 citation) ClinicalTrials.gov - NCT02775851
- (1 citation) ClinicalTrials.gov - NCT03342417
- (1 citation) ClinicalTrials.gov - NCT03430063
- (1 citation) ClinicalTrials.gov - NCT02842125
- (1 citation) ClinicalTrials.gov - NCT03178552
- (1 citation) ClinicalTrials.gov - NCT03544723
- (1 citation) ClinicalTrials.gov - NCT03568539
- (1 citation) ClinicalTrials.gov - NCT03516981
- (1 citation) ClinicalTrials.gov - NCT03222856
- (1 citation) ClinicalTrials.gov - NCT03330405
- (1 citation) ClinicalTrials.gov - NCT03519412
- (1 citation) ClinicalTrials.gov - NCT03289819
- (1 citation) ClinicalTrials.gov - NCT02553642
- (1 citation) ClinicalTrials.gov - NCT02949843
- (1 citation) ClinicalTrials.gov - NCT03172936
Show less
Similar Articles
To arrive at the top five similar articles we use a word-weighted algorithm to compare words from the Title and Abstract of each citation.
Association of Survival and Immune-Related Biomarkers With Immunotherapy in Patients With Non-Small Cell Lung Cancer: A Meta-analysis and Individual Patient-Level Analysis.
JAMA Netw Open, 2(7):e196879, 03 Jul 2019
Cited by: 145 articles | PMID: 31290993 | PMCID: PMC6625073
Assessment of Blood Tumor Mutational Burden as a Potential Biomarker for Immunotherapy in Patients With Non-Small Cell Lung Cancer With Use of a Next-Generation Sequencing Cancer Gene Panel.
JAMA Oncol, 5(5):696-702, 01 May 2019
Cited by: 276 articles | PMID: 30816954 | PMCID: PMC6512308
Tumour mutational burden as a biomarker for immunotherapy: Current data and emerging concepts.
Eur J Cancer, 131:40-50, 09 Apr 2020
Cited by: 129 articles | PMID: 32278982 | PMCID: PMC9473693
Review Free full text in Europe PMC
Genomic scoring to determine clinical benefit of immunotherapy by targeted sequencing.
Eur J Cancer, 120:65-74, 04 Sep 2019
Cited by: 30 articles | PMID: 31493723
Funding
Funders who supported this work.
Cancer Research UK (2)
Lung Cancer Centre of Excellence Award
Professor Tariq Enver, University College London
Grant ID: 20466
Deciphering the Immune and Neoantigen Landscape In NSCLC and Their Relevance to Cancer Immunotherapy
Professor Sergio Quezada, University College London
Grant ID: 22246
Exelixis, Merck, and Bristol-Myers Squibb
Mellnikoff Precision Immunotherapy Kidney Cancer Fund
NCI Cancer Center Support (1)
Grant ID: P30 CA008748
NCI NIH HHS (3)
Grant ID: R35 CA232097
Grant ID: R01 CA205426
Grant ID: P30 CA008748
NIH (1)
Grant ID: R35 CA232097
The Francis Crick Institute (1)
Grant ID: 10169