Abstract
Free full text

RNA-GPS Predicts SARS-CoV-2 RNA Residency to Host Mitochondria and Nucleolus
Abstract
SARS-CoV-2 genomic and subgenomic RNA (sgRNA) transcripts hijack the host cell's machinery. Subcellular localization of its viral RNA could, thus, play important roles in viral replication and host antiviral immune response. We perform computational modeling of SARS-CoV-2 viral RNA subcellular residency across eight subcellular neighborhoods. We compare hundreds of SARS-CoV-2 genomes with the human transcriptome and other coronaviruses. We predict the SARS-CoV-2 RNA genome and sgRNAs to be enriched toward the host mitochondrial matrix and nucleolus, and that the 5′ and 3′ viral untranslated regions contain the strongest, most distinct localization signals. We interpret the mitochondrial residency signal as an indicator of intracellular RNA trafficking with respect to double-membrane vesicles, a critical stage in the coronavirus life cycle. Our computational analysis serves as a hypothesis generation tool to suggest models for SARS-CoV-2 biology and inform experimental efforts to combat the virus. A record of this paper’s Transparent Peer Review process is included in the Supplemental Information.
Introduction
COVID-19 (coronavirus disease 2019) has become a global pandemic, fueled by the rapid spread of the coronavirus SARS-CoV-2 (severe acute respiratory syndrome coronavirus 2), a positive-strand RNA virus (Wu et al., 2020a; Sanche et al., 2020). The scientific community is actively trying to understand SARS-CoV-2’s biological mechanisms and effects. Here, we computationally analyze the subcellular localization patterns of SARS-CoV-2 RNA transcripts. Our results suggest potential avenues for experimental validation and follow-up, while providing a template for in silico analyses of viral RNA.
RNA subcellular localization is critical to a myriad of cellular processes (Ryder and Lerit, 2018; Chin and Lécuyer, 2017; Buxbaum et al., 2015). Researchers have also discovered that RNA localization plays a significant role in the life cycle of viruses, with functions ranging from regulating sites of virion assembly (Becker and Sherer, 2017) to disrupting host mitochondrial function (Somasundaran et al., 1994). However, the subcellular localization of SARS-CoV-2 (and other conronavirus) RNA is largely unexplored. Gaining a better understanding of the behavior and localization of SARS-CoV-2’s RNA genome and transcripts can lead to a better understanding of its function and pathogenicity, potentially revealing targetable mechanisms.
To computationally study this aspect of SARS-CoV-2 biology, we built upon our recent work developing RNA-GPS, a state-of-the-art computational model for predicting high-resolution RNA localization in human cells (Wu et al., 2020b). RNA-GPS was trained on transcriptome-wide localization patterns of human RNAs across eight subcellular landmarks (Fazal et al., 2019). RNA-GPS’s strong performance, coupled with viruses’ dependence on hijacking and repurposing existing cell machinery for reproduction, suggests that RNA-GPS could provide insights into SARS-CoV-2’s localization behavior and can focus future experimental efforts.
We use RNA-GPS to interrogate the dominant subcellular residency patterns of SARS-CoV-2’s genome, which spans approximately 30 kilobases of single-stranded positive-sense RNA (Kim et al., 2020) (Figure 1 A). RNA-GPS predicts that SARS-CoV-2 and the transcripts it forms have enriched residency at the nucleolus and the mitochondria. We note that our analysis may suggest potential localization mechanisms for SARS-CoV-2, rather than direct physical localization, particularly with regard to our mitochondrial prediction. Comparison of SARS-CoV-2’s predicted residency with that of other human coronaviruses, including strains causing the common cold, Middle East respiratory syndrome (MERS), and the SARS outbreak of 2003, shows that SARS-CoV-2 exhibits a stronger mitochondrial and nuclear residency signal than a large majority of its coronavirus relatives. We additionally find that this residency signal appears to be driven by the 5′ and 3′ ends of the viral genome. We conclude by connecting our predictions to known RNA and viral biology and proposing possible explanatory mechanisms for previously observed phenomena. Our findings entreat experimental validation and serve as a framework for applying machine learning for principled hypothesis generation in viral biology.
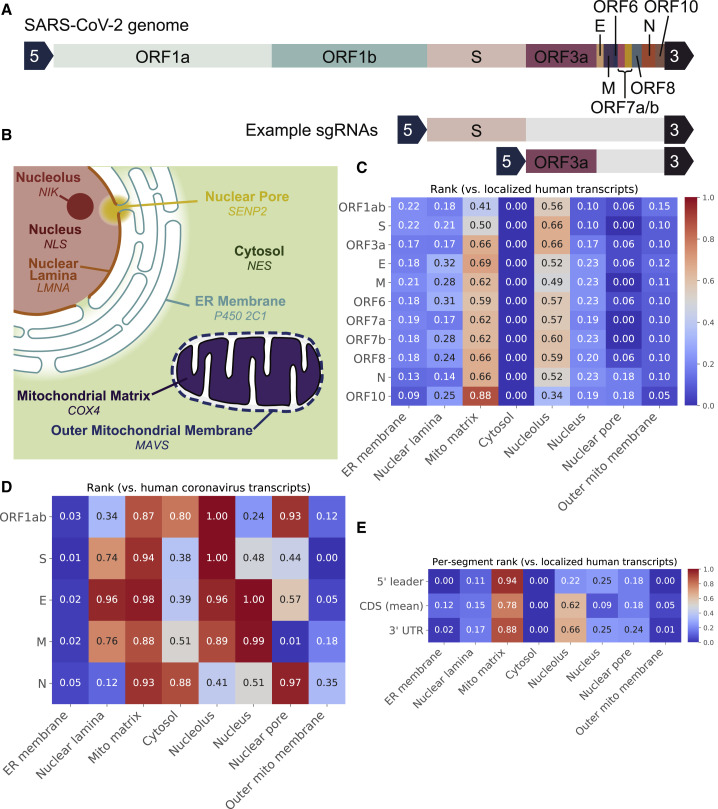
Depictions of the SARS-CoV-2 Genome, the Eight Compartments that RNA-GPS Predicts Viral Transcript Residency to, and the Predicted Residencies for SARS-CoV-2 sgRNAs and its 5′/CDS/3′ Sequence Segments
(A and B) The SARS-CoV-2 genome produces a series of sub-genomic RNAs (sgRNAs), each encoding one or more genes or proteins (A). These sgRNAs share a common leader 5′ sequence and a common trailing 3′ UTR sequence (arrow blocks). For each sgRNA, RNA-GPS predicts residency to each compartment in (B). Italicized text indicates the APEX2 fusion protein used to measure transcripts corresponding to each localization (see Table S1).
(C and D) (C) Heatmap of rank scores, indicating how strongly each sgRNA (rows) is predicted to exhibit subcellular residency at each compartment (columns), compared with endogenous human transcripts measured to localize to that compartment. Colors indicate rank scores; color scale is shared across all heatmaps. Most sgRNAs share similar residency patterns, exhibiting statistically significant enrichment toward the mitochondrial matrix and nucleolus (see Table S3). We also computed these rank scores against a baseline of other coronavirus residency signals (D). SARS-CoV-2 exhibits a stronger mitochondrial matrix residency signal than most other coronaviruses, along with greater overall nuclear residency, particularly at the nucleolus. For context, coronaviruses are generally predicted to have residency at the nucleolus, mitochondrial matrix, and ER membrane (see Figure S2). These predictions are also consistent across different models (see Figure S3) and negative-strand SARS-CoV-2 sgRNA precursors (see Figure S4).
(E) Shows the predicted residency rank scores for shared 5′ and 3′ segments and an averaged residency rank score for the variable coding segments. Even on their own, the short ~90–250 base pair 5′ and 3′ segments carry mitochondrial and nucleolar residency signals.
Results
We leverage our recent work developing RNA-GPS, a computational model predicting high-resolution RNA subcellular localization in human cells (Wu et al., 2020b). Our model was built using APEX-seq data, which fuses the APEX2 (engineered ascorbate peroxidase, version 2) protein to various protein localization sequences (Figure 1B; Table S1) to guide APEX2 to each subcellular region for subsequent proximity biotinylation of nearby RNAs (Fazal et al., 2019). The resultant transcripts captured and measured at the nucleolus, for example, are transcripts proximal to APEX2 in the nucleolus, as well as those proximal to APEX2 throughout its entire lifecycle, including its transport to the nucleolus. Such “en route” transcripts constitute a small proportion of total transcripts, except in the notable case of the mitochondrial matrix COX4 marker (Richter-Dennerlein et al., 2016), which picks up a sizable proportion of nuclear-encoded transcripts as it is imported to the mitochondria (Figure S1A). Though this is surprising, these nuclear-encoded, mitochondrial-enriched transcripts are reproducibly distinct from noise (Figures S1B and S1C) and actually enrich for cytoskeletal and intracellular transport processes (Figure S1D). For the sake of brevity, we will refer to these measurements using their final destinations, as confirmed by imaging: the cytosol, endoplasmic reticulum (ER), mitochondrial matrix, outer mitochondrial membrane, nucleus, nucleolus, nuclear lamina, and nuclear pore. RNA-GPS predicts localization to each of these eight neighborhoods (Figure 1B).
Although RNA-GPS is trained on human, not viral, RNA transcripts, its ability to generalize across cell types not used in training (Wu et al., 2020b), combined with the fact that viruses commandeer human cellular machinery, suggests that it offers a reasonable hypothesis of viral transcript-localization behavior given currently available data. Nonetheless, there is inherent uncertainty associated with generalizing our model across species, and we use the term dominant subcellular residency to indicate this predictive uncertainty where appropriate.
We consider viral transcript subcellular residency predictions to each compartment averaged across all released and annotated SARS-CoV-2 genomes available as of April 6, 2020 (n = 213) on GenBank (NCBI Resource Coordinators, 2018). SARS-CoV-2 is believed to enter the cell as a positive-strand genomic RNA, subsequently forming 11 positive-strand subgenomic RNA (sgRNA) transcripts encoding different open reading frames and sharing the same 5′ leader sequence and 3′ untranslated region (UTR) (Figure 1A) (Kim et al., 2020). Within each viral genome, we predict the residency of each sgRNA produced from the primary SARS-CoV-2 genome.
To better understand how strong these predicted residency probabilities are in a meaningful biological context, we frame them relative to predictions for other relevant baseline transcript sequences. We consider two such baselines: (1) the distribution of model predictions on transcripts exhibiting significant localization within the human HEK293T cell line (n = 366 transcripts), as measured by APEX-seq (Fazal et al., 2019), and (2) the distribution of model predictions on transcripts derived from human coronaviruses, excluding SARS-CoV-2 (n = 191 genomes, spanning diseases from the common cold to MERS, Table S2). The human baseline quantifies the strength of RNA residency signals in SARS-CoV-2 relative to naturally occurring human transcripts with well-characterized localization behaviors. The coronavirus baseline focuses on differences in the transcript residency behavior of SARS-CoV-2 relative to similar viral specimens—differences that may help researchers focus on the peculiarities of this virus. For both baselines, we calculate the proportion of the baseline distribution that the SARS-CoV-2 subcellular residency prediction exceeds, which we refer to as a rank score. For example, a residency rank score of 0.6 for the nucleolus relative to human transcripts suggests that the particular viral RNA is more likely to have been picked up by the nucleolus APEX-seq marker compared with 60% of human RNAs that are empirically measured to do so.
SARS-CoV-2 RNA Subcellular Residency Patterns
We find that, compared to transcripts with known localizations in human cells, SARS-CoV-2 has a notable residency signal toward the nucleolus, as well as the mitochondrial matrix (Figure 1C). These residency signals are consistent across different sgRNAs encoded by the virus (shown in each row of Figure 1C) and represent statistically significant predicted residency (Table S3). The nucleolus is known to play a prominent role in the viral life cycle, even for viruses that primarily replicate in the cytoplasm as SARS-CoV-2 presumably does (Salvetti and Greco, 2014). While some RNA viruses like human immunodeficiency virus (HIV) have been reported to localize RNA to the mitochondria (Somasundaran et al., 1994), there has not been direct evidence that SARS-CoV-2 does this. As previously discussed, since much of the APEX-seq mitochondrial data used to train RNA-GPS actually consists of nuclear-encoded transcripts likely picked up as the APEX-COX4 fusion protein is transported to the mitochondria, we hypothesize that our predicted mitochondrial residency is alluding to similarity in localization pathways, rather than localization destination.
In addition to framing our localization results in the context of endogenous human transcripts, we also compare predicted residency of SARS-CoV-2 sgRNAs with that of other human coronaviruses (Figure 1D). Here, we observe similar overall trends in our residency predictions. Consistent with the comparison with human transcripts, we find that the SARS-CoV-2 mitochondrial matrix residency signal is stronger than that of many other coronaviruses. Additionally, we see an overall pattern suggesting that SARS-CoV-2 may have a greater affinity for nuclear neighborhoods (nuclear pore, nucleus, nucleolus, and nuclear lamina) compared to other coronaviruses.
We also compared the dominant subcellular residency patterns of the coronavirus family (excluding SARS-CoV-2) with human transcripts using RNA-GPS. We found that the most prominent residency signals for general human coronaviruses pointed toward the nucleolus, mitochondrial matrix, and ER membrane (Figure S2). Overall, our computational analysis suggests that SARS-CoV-2’s predicted sgRNA transcript residency enriching for the mitochondrial matrix and nucleolus may be amplifications of behaviors that were already present in coronaviruses.
While direct experimental data measuring coronavirus sgRNA transcript localization is not currently available, we sought to validate our predictions on other human viruses with known subcellular localizations. After conducting a systematic literature search, we found one such example: the human cytomegalovirus (CMV) β2.7 mRNA transcript, which localizes to the inner mitochondrial membrane (Williamson et al., 2012) and is approximately 2.5 kilobases long. RNA-GPS predicts this transcript to reside at the mitochondrial matrix with a rank score of 0.81; no other compartments have a rank score exceeding 0.5 (Figure 2 A). Thus, the algorithm’s residency prediction is in close agreement with experimental evidence for β2.7 mRNA localization. While large-scale comparisons are not currently feasible due to lack of datasets measuring viral transcript localization, this example provides some reassurance that RNA-GPS’ predicted viral residencies are reasonable.
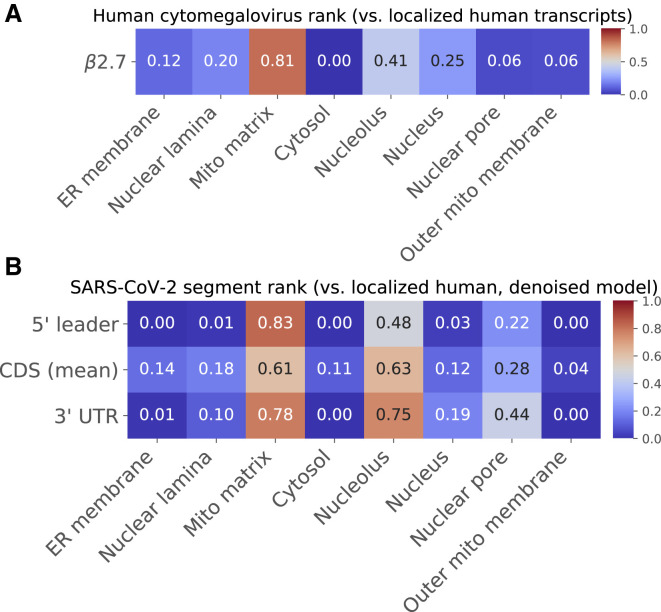
Validation of SARS-CoV-2 Residency Predictions
(A) RNA-GPS predictions for the human cytomegalovirus β2.7 transcript, which has been shown to localize to the inner mitochondrial membrane. RNA-GPS correctly predicts its residency to the closest compartment it has been trained on—the mitochondrial matrix. This provides support that RNA-GPS can make reasonable predictions on viral RNA.
(B) To evaluate the effect of the potentially noisy mitochondrial examples in our APEX-seq training set on predicted SARS-CoV-2 residencies, we trained a “denoised” variant of RNA-GPS on a subsetted dataset that excludes these examples. This denoised model predicts the same residency pattern for the three components of the SARS-CoV-2 sgRNAs (compare with Figure 1E). For additional analysis of the mitochondrial dataset and predictions, see Figure S1.
To further validate the robustness of these results, we also trained a different predictive algorithm (a recurrent neural network; see STAR Methods for additional details) on the APEX-seq data and performed a similar set of experiments, comparing SARS-CoV-2 dominant subcellular residency predictions with human and coronavirus baselines (Figures S3A and S3B). This alternative model also predicts strong mitochondrial matrix and nucleolus residency for SARS-CoV-2. Since this algorithm uses a very different modeling strategy from RNA-GPS and nonetheless converges to similar findings, this suggests that the mitochondrial matrix and nucleolus residency predictions are not artifacts of a particular computational modeling strategy, but rather arise from a consistent signal in the data, thus increasing our confidence in our findings.
In addition to evaluating robustness of our results to modeling strategies, we also evaluated robustness with respect to the APEX-seq data used to train the models. As we previously mentioned, many APEX-seq transcripts used to train RNA-GPS’s mitochondrial predictions are actually nuclear encoded. These transcripts exhibited relatively low (albeit significant) enrichment compared with transcripts natively encoded in the mitochondrial genome. To ensure that our results have not been driven by potentially noisy data in this regime, we excluded nuclear-encoded, “noncanonical” mitochondrial matrix transcripts with relatively low APEX-seq enrichment signal (lowest 20% of log-fold-change enrichment scores) and retrained RNA-GPS on this adjusted dataset. This “denoised” model recapitulates the same SARS-CoV-2 residency toward the mitochondrial matrix and nucleolus (Figure 2B), suggesting that our predictions are robust to potential noise in the training data. In summary, our predicted residencies are robust across different modeling strategies and across variation in the data used to train these models.
SARS-CoV-2 Negative-Strand RNA Also Shows Residency to Mitochondria and Nucleolus
During their replication life cycle, coronaviruses like SARS-CoV-2 copy their positive-strand RNA to create a negative-strand RNA that serves as the template for viral “transcription” and production of sgRNAs (Wu and Brian, 2010). We applied RNA-GPS to the negative-strand SARS-CoV-2 sgRNA precursors and discovered that they also exhibit residency to the mitochondrial matrix and nucleolus (Figure S4). This result suggests that the sequence features driving these residency patterns are independently present in both positive- and- negative-strand RNAs, further boosting the localization capability of SARS-CoV-2 during different stages of its viral cycle.
SARS-CoV-2 5′ and 3′ UTRs Contain Strong Residency Signals
In addition to predicting residency, our computational model can also help us understand which regions of the transcript may be more responsible for driving these predictions. At a high level, this can be done by evaluating which features were most important for RNA-GPS’s predictions. We specifically investigated the potential contribution of the three main regions of the SARS-CoV-2 sgRNAs: the shared 5′ leader sequence, the shared 3′ UTR, and the variable “coding” sequence in the between (i.e. bases not in the 5′ or 3′ pentagon caps in Figure 1A). We predicted residency for each of these regions by itself (averaging across all variants of the coding region) (Figure 1E). The 5′ leader sequence shows the strongest residency signal for the mitochondrial matrix and relatively low signal for the nucleolus. In contrast, the 3′ UTR has the strongest residency for the nucleolus and also has a strong signal for the mitochondrial matrix. The coding sequence (CDS) also shows specific signals for these two compartments. As the 5′ and 3′ sequences are shared by the different SARS-CoV-2 sgRNAs, this is likely a strong factor behind the consistent residency patterns that we predict across the different sgRNAs. We also performed further computational ablation studies of RNA-binding protein (RBP) motifs in SARS-CoV-2 (see STAR Methods). However, computational deletions of all instances of each individual RBP motif, repeated across all enriched RBPs, did not significantly alter the RNA-GPS residency predictions. This result suggests that the SARS-CoV-2 residency signal could be abundant in the viral genome and may involve complex interactions not captured by relatively short, single RBP-binding motifs.
Discussion
In this work, we apply computational models of human RNA transcript localization to better understand the subcellular localization behavior of the SARS-CoV-2 genome and its constituent sgRNAs. This approach builds upon the idea that the virus uses existing host cell machinery to reproduce and, consequently, that sequence-based localization signals are likely shared between human and coronavirus transcripts. The strengths of this approach include (1) the potential to understand viral RNA localization without the risk of live viral cultures; (2) the ability to examine hundreds of viral isolates and related coronaviruses and thousands of RBP motif ablations; (3) the ability to examine viral genes, UTRs, and negative strands individually, which may otherwise require the ability to precisely synchronize and arrest the viral life cycle. We find that SARS-CoV-2 appears to harbor strong transcript residency signals toward the mitochondrial matrix and nuclear compartments, often comparable to human RNAs and more so than other coronaviruses. This intriguing hypothesis suggests future experimental exploration and validation.
As we mentioned previously, we believe that our predicted mitochondrial residency signal is more indicative of a localization pathway than a destination; in the context of coronavirus biology, this may specifically be related to double-membrane vesicles (DMVs). Coronaviruses are known to produce DMVs to serve functions like concealing the virus from cellular defenses (Hagemeijer et al., 2012; Knoops et al., 2008). While these DMVs are generally believed to be formed via viruses manipulating the ER membrane (Blanchard and Roingeard, 2015), the mechanism for importing and packaging proteins and RNA into these miniature organelles is not as clearly understood. One possible mechanism for importing viral RNA involves the virus exploiting the RNA localization mechanisms that the cell already possesses for endogenous double-membrane organelles, namely, the mitochondria. Indeed, introducing just two amino acid point mutations in the murine coronavirus causes both a significant drop in the number of DMV structures observed, as well as a sharp increase in viral protein localization at the mitochondria (Clementz et al., 2008). This alludes to a high degree of resemblance between DMV and mitochondrial localization mechanisms—leading to our hypothesis that our mitochondrial matrix residency predictions are capturing this similarity between the DMV and mitochondria. Furthermore, DMVs have been shown to contain double-stranded RNA (Hagemeijer et al., 2012); our strand-agnostic residency predictions are concordant with this evidence and might even encourage formation of such complexes. Under this model, SARS-CoV-2’s strong mitochondrial residency signal relative to other coronaviruses might even contribute to its similarly high infectivity by increasing its efficacy in forming these DMV structures.
Another possible interpretation of these predicted residencies is that previously studied viral protein localizations are influenced by transcript-level localizations, a mechanism that is highly prevalent for proteins in normal human cells (Blower, 2013). Protein-protein interaction studies performed on SARS-CoV-2 have found that its NSP5 (within ORF1a), NSP13 (within ORF1b), ORF6, and ORF10 proteins interact with host proteins that predominantly localize to nuclear compartments (Gordon et al., 2020). The same study found that the ORF9b protein, produced by the “N” sgRNA, interacts with TOMM70, a mitochondrial import receptor that plays a critical role in modulating interferon response—a key antiviral cellular defense pathway (Liu et al., 2010). In both cases, localized viral transcripts could help drive viral protein localization, enabling more focused protein-protein interactions.
A limitation of our work lies in its application of models trained on human RNA transcript localization data to viral transcripts. It is possible that SARS-CoV-2 infection could alter the host subcellular structures and RNA transport machinery so drastically that our learned localization patterns from human cells no longer hold. If RNA-GPS’s predictions turn out to be wrong for this reason, this might suggest that coronavirus infection devastates host cell RNA trafficking and localization—a previously unrecognized feature of COVID-19 pathobiology. After all, the vast majority of RBPs in the host cell, which are key drivers of transcript localization, recognize and process RNAs irrespective of whether they are endogenous or foreign, and the inability to “properly” localize viral RNAs should mirror a similar breakdown for host cell transcripts. As we are unable to use existing experimental evidence to thoroughly evaluate and cross-reference the predictions discussed here, future experiments in this vein are clearly necessary. Given the historical scarcity of studies focusing on viral transcript localization, such experiments would likely reveal interesting, crucial insights into viral pathobiology, whether they confirm our specific mitochondrial and nucleolus predictions or not. It is worth pointing out, though, that this is but one of many complex, interconnected viral mechanisms at play.
In summary, we build upon recent computational models of RNA subcellular localization to study, in silico, the localization properties of SARS-CoV-2 transcripts. Our results suggest that predicted transcript residency signals, specifically toward the nucleolus and mitochondrial matrix, may be important, unique characteristics of SARS-CoV-2 that warrant additional study. We connect these observations to known viral biology regarding DMV structures in viral replication, as well as SARS-CoV-2 protein localization patterns. In doing so, we propose potential cellular mechanisms that underpin viral biology—mechanisms that warrant experiments validating their accuracy and perhaps even their potential as therapeutic targets. More broadly, we hope that our study helps define a framework for applying machine learning models to enable focused hypothesis generation, enabling similar studies that leverage data science to rapidly respond to emerging epidemiological challenges.
Key Changes Prompted by Reviewer Comments
In the interest of transparency, the following changes were made in this paper during review. We used “RNA subcellular residency” rather than “RNA localization” to describe RNA-GPS prediction results, as this is more reflective of the underlying APEX-seq training data and its inherent differences compared with viral transcripts. We clarified the origin, specificity, and interpretation of nuclear-encoded transcripts enriched by the COX4-APEX2 mitochondrial matrix landmark, thus enhancing our interpretation of this predicted localization. We added a positive control showing that a CMV mRNA with known mitochondrial localization is correctly predicted by RNA-GPS. We thank the reviewers and editor for insightful comments that have improved this work. For context, the complete Transparent Peer Review Record is included within the Supplemental Information.
STAR
Methods
Key Resources Table
REAGENT or RESOURCE | SOURCE | IDENTIFIER |
---|---|---|
Deposited Data | ||
Coronavirus (incl. SARS-CoV-2) genome sequences | NCBI GenBank | Various (see query strings in covid19/baseline.py and covid19/covid19.py source code files in GitHub repository) |
Human cytomegalovirus genome sequence | NCBI GenBank | NC_006273.2 |
APEX-seq RNA localization data | Fazal et al., 2019 | GEO: GSE116008 |
RNA binding protein motif database | Ray et al., 2013 | MEME Motif Databases |
seqFISH data | Fazal et al., 2019 | Derived from Figure 2, PMID 31230715 |
Software and Algorithms | ||
RNA-GPS model and SARS-CoV-2 analysis code | This manuscript and Wu et al., 2020b | https://github.com/wukevin/rnagps |
Resource Availability
Lead Contact
Further information and requests for resources should be directed to and will be fulfilled by the Lead Contact, Howard Y. Chang ([email protected]).
Materials Availability
This computational study did not generate or use new reagents.
Data and Code Availability
The data supporting the findings of this study are all available within publicly available repositories as listed in the Key Resources Table. All code required to query and download viral sequences, as well as to reproduce results and figures can be found within the GitHub repository listed in the Key Resources Table. All software dependencies for RNA-GPS and the SARS-CoV-2 analysis described herein are freely available. Within the GitHub repository, most code pertaining to SARS-CoV-2 analysis can be found under the “covid19” folder; other folders contain supporting data and source code.
Method Details
Obtaining Viral Genomes
SARS-CoV-2 viral genomes were programmatically queried from the NCBI GenBank online database using the BioPython library’s Entrez module (Cock et al., 2009). The exact query sequence used can be found within the “covid19/covid19.py” file in the GitHub repository. Returned results were then filtered to retain only assemblies that included annotated, named sgRNA “genes.” We consider the sgRNAs corresponding to ORF1ab, S, ORF3a, E, M, ORF6, ORF7a, ORF7b, ORF8, N, and ORF10, as these have the most consistent annotations. In cases where the shared 5’ leader sequence or the 3’ tail were not explicitly annotated, their regions were inferred to be the 5’ and 3’ trailing bases outside of any coding regions, respectively. As there are many SARS-CoV-2 genome assemblies that fit these criteria, subcellular residency predictions are averaged across all genomes.
Viral genomes constituting the coronavirus baseline follow an identical process, save for using a different NCBI GenBank query sequence that specifically fetches matches to the six coronaviruses known to infect humans (excluding SARS-CoV-2): 229E, NL63, OC43, HKU1, MERS-CoV (beta coronavirus that causes Middle East Respiratory Syndrome, or MERS), and SARS-CoV (the beta coronavirus that causes severe acute respiratory syndrome, or SARS) (Su et al., 2016). The exact query sequence used can be found in the “covid19/baseline.py” source file in the GitHub repository. A detailed breakdown of the exact number of genomes derived from each strain is in Table S2.
The human cytomegalovirus was chosen for additional evaluation based on a systematic literature review of viral RNA localization studies. This is the only example we found that associates a specific viral transcript with a consistent experimentally validated localization. The viral sequence for validation of our model predictions was obtained from the NCBI GenBank reference sequence NC_006273.2. Due to lack of standardized 5′ and 3′ UTR region annotations for this transcript (despite these being referenced in the literature), we manually determined these regions after reviewing literature and the overall genome annotation.
Sequence Featurization and Predictive Models
RNA-GPS uses k-mer featurization with k = 3, 4, 5, applied independently to the 5′ untranslated region (UTR), coding sequence (CDS), and 3′ UTR parts of the transcript (Wu et al., 2020b). This creates a feature space of (43 + 44 + 45) x 3 = 1344 x 3 = 4032 dimensions. These features are then consumed by a random forest model (implemented using the scikit-learn Python library) to generate localization/residency predictions. Extending this definition to the coronavirus sgRNA sequences, we consider the shared 5′ leader sequence the fixed 5′ UTR input to our model, shared 3′ UTR sequence the fixed 3′ UTR input to our model, and the variable sgRNA sequence the “CDS” input. For sake of consistency with sgRNA transcript mechanisms, this “CDS” sequence includes the current reading frame, along with any 3′ downstream bases until the shared 3′ UTR region begins. Each sgRNA is individually assigned predicted residencies. RNA-GPS’s per-segment featurization also enables the per-segment residency analysis. For this, we selectively provide the model with only features that correspond to a single segment (i.e. the 5′ UTR, CDS, or 3′ UTR), with zero values for other features.
For the deep recurrent model, we implemented and trained a recurrent neural network that consumes raw bases as input, maps these to a 32-dimensional embedding layer, passes these through two 64-dimensional gated recurrent units (GRU), and finally a fully-connected layer with sigmoid activation producing 8 localization/residency predictions. This flavor of GRU network is popular in sequence modeling and uses “gating” mechanisms to improve learning of longer-range sequence dependencies (Chung et al., 2014). The model was implemented in PyTorch and was trained to minimize a binary cross-entropy loss using the Adam optimizer (Kingma and Ba, 2014) with a batch size of 1, with early stopping based on validation set area under the receiver operating characteristic (AUROC).
Training Data for Predictive Models
Both RNA-GPS and the GRU model are trained and tuned on the same APEX-seq data, measuring localization within HEK293T cells (Fazal et al., 2019). Localization within this dataset is expressed as an enrichment score compared to the rest of the cell. We consider transcripts that exhibit significant enrichment (log fold change (logFC) > 0 and adjusted p-value ≤ 0.05) for at least one of the eight measured compartments (n = 3660). Many transcripts contain more than one significant localization. Furthermore, due to the nature of the APEX-seq technology, transcripts measured at a specific compartment may also contain transcripts that were picked up as the APEX2 labeling protein itself was being transported to that compartment. This effect is usually minimal, except for mitochondrial transcripts (see Figure S1). We use data splits of 80% train (n = 2928), 10% validation (n = 366), and 10% train (n = 366). As is conventional, the validation set was used for hyperparameter tuning and model architecture tuning.
When removing potentially spurious mitochondrial examples, we start with the above dataset and remove all transcripts that measured to localize to the mitochondrial matrix but have log fold change enrichment in the bottom 20th percentile of localized mitochondrial matrix transcripts. This removes the bottom 20% of mitochondrial sequences with the lowest enrichment relative to the rest of the cell (n = 61) – this denoised dataset contains 240 mitochondrial matrix transcripts instead of 301, and a total of 3599 transcripts compared to 3660 previously.
RNA Binding Protein Motif Ablation
We use a database of 102 RNA binding protein binding motifs (Ray et al., 2013). To identify matches, we use the same methodology as was used in the RNA-GPS manuscript (Wu et al., 2020b). We start with the position weight matrix (PWM) that describes the motif, adjust its probabilities to account for the background nucleotide composition of each transcript sequence, define a cutoff score slightly lower than the maximum achievable log-likelihood for that PWM, and identify any subsequences that exceed that cutoff.
When ablating these PWMs, we use the same methodology for identifying hits, and subsequently replace all hits with “N” bases, re-featurizing the ablated sequence as necessary before feeding into the model, thus generating the ablated localization predictions.
Quantification and Statistical Analysis
Baseline Construction and Rank Score
Baseline distributions are constructed by running a set of baseline transcript sequences through a model predicting transcript localization/residency. For each individual model, there is a per-localization baseline derived from human APEX-seq measurements, and one derived from human coronaviruses excluding SARS-CoV-2. For each localization neighborhood within the human baseline, we consider only transcripts that exhibit significant localization to that neighborhood, as defined by having a logFC > 0 and adjusted p-value ≤ 0.05 when running differential expression analysis against the remainder of the cell. Additionally, we only use transcripts not used for model training/tuning (i.e. the test data split), as this most closely approximates what the model would predict when presented with novel sequences.
For the coronavirus baseline, we do not have systematically measured localization data, so we cannot constrain this baseline using known localizations behaviors. Instead, each SARS-CoV-2 sgRNA is compared only to homologous sgRNAs from other coronaviruses. For example, the spike protein’s residency prediction is only compared against residency predictions of other coronavirus spike proteins. This limits our comparison to the set of genes with easily traceable homology across human coronaviruses, namely ORF1ab, spike (S), envelope (E), membrane (M), and nucleocapsid (N) (Woo et al., 2010).
For both these baselines, we define a rank score as the proportion of baseline values that a SARS-CoV-2 sgRNA residency prediction exceeds. A hypothetical value of 0.5 would correspond to a median, 0.25 would correspond to the first quartile, etc.; rank score is thus bound between 0 and 1 (inclusive). Note that this rank is calculated for each individual compartment separately, as the baselines themselves are compartment specific. As previously discussed, subcellular residency predictions are averaged across all valid SARS-CoV-2 genomes prior to calculating rank scores. Furthermore, since the human baseline is constrained by measured localizations, whereas the coronavirus baseline is constrained by sequence homology, rank scores for these two baselines are not directly comparable.
Significance Test for Localization
In addition to computing the rank scores described above, we also evaluate whether these rank scores correspond to significant enrichment. To do this, we compare the underlying predicted residency probabilities (not the rank scores) against a “null” distribution of localization probabilities for human transcripts exhibiting no significant localization. We do this using a one-sided Wilcoxon rank-sum test (scipy Python package (Virtanen et al., 2020), with the hypothesis that residency probabilities exceed that of the null distribution. Our data satisfies the Wilcoxon rank-sum test’s assumptions of independence, and our residency/localization prediction probabilities are naturally ordinal. To address the fact that we do multiple comparisons, we use the Holm method (statsmodels Python package (Seabold and Perktold, 2010)) to correct the resultant p-values.
Analysis of Sequential FISH Images
The sequential FISH experiments were described in (Fazal et al., 2019), and resulted in data for 29 transcripts retained for further analysis. The analysis was also described in (Fazal et al., 2019), and briefly consists of compiling imaging data from 20 fields of view, each with > 20 cells, and with the data processed using MATLAB. For the quantification of each field of view, a mask was generated for each gene of interest using a uniform threshold cutoff of 0.5–0.998, after removing non-cell pixels. The co-localization score with mitochondria was calculated by interesting the mask of a particular gene with the mask of the mitochondrial-resident transcript MT-ND3, and then dividing the summed intensity of the intersected mask by the summed intensity of the gene masks of interests. The quantification results for all 20 fields of view were then averaged to obtain the final number.
Gene ontology Enrichment Analysis
To perform gene ontology enrichment analysis, we used the PANTHER tool (Mi et al., 2019) provided by the Gene Ontology Consortium (Ashburner et al., 2000, The Gene Ontology, 2019). Genes were compared in an overrepresentation test against a reference list of all genes in the Homo sapiens database using Fisher’s Exact test, with false discovery rate correction. The annotation used was “Reactome version 65”
Plotting
Plots were generated using a combination of seaborn and matplotlib Python packages (Hunter, 2007).
Acknowledgments
We thank the members of the Chang and Zou laboratories for helpful discussions. We thank Shuo Han, Alistair Boettiger, and Alice Ting for generating and analyzing FISH images presented herein. H.Y.C. is supported by RM1-HG007735 and R01- HG004361. H.Y.C. is an investigator of the Howard Hughes Medical Institute. J.Z. is supported by NSF CCF 1763191, NIH R21 MD012867-01, NIH P30AG059307, NIH U01MH098953, and grants from the Silicon Valley Foundation and the Chan-Zuckerberg Initiative. F.M.F. is supported by an NIH K99/R00 award from NHGRI (HG010910).
Author Contributions
H.Y.C. and J.Z. conceived the idea for this project and supervised its execution. K.E.W. gathered, preprocessed, and analyzed data for this project with input from all authors. F.M.F. and K.R.P. contributed to the analysis of mitochondrial APEX-seq and FISH data with input from all authors. All authors contributed to interpreting localization results in the context of coronavirus biology. K.E.W. wrote the manuscript with input from all authors.
Declaration of Interests
K.R.P. is a consultant for Maze Therapeutics. H.Y.C. is affiliated with Accent Therapeutics, Boundless Bio, 10X Genomics, Arsenal Bio, and Spring Discovery. J.Z. is affiliated with InterVenn Biosciences.
Footnotes
Supplemental Information can be found online at https://doi.org/10.1016/j.cels.2020.06.008.
Supplemental Information
References
- Ashburner M., Ball C.A., Blake J.A., Botstein D., Butler H., Cherry J.M., Davis A.P., Dolinski K., Dwight S.S., Eppig J.T. Gene ontology: tool for the unification of biology. The Gene Ontology Consortium. Nat. Genet. 2000;25:25–29. [Europe PMC free article] [Abstract] [Google Scholar]
- Becker J.T., Sherer N.M. Subcellular Localization of HIV-1 HIV-1 gag-pol mRNAs regulates sites of virion assembly. J. Virol. 2017;91:e02315–e02316. [Europe PMC free article] [Abstract] [Google Scholar]
- Blanchard E., Roingeard P. Virus-induced double-membrane vesicles. Cell. Microbiol. 2015;17:45–50. [Europe PMC free article] [Abstract] [Google Scholar]
- Blower M.D. Molecular insights into intracellular RNA localization. In: Jeon K.W., editor. International Review of Cell and Molecular Biology. Academic Press; 2013. pp. 1–39. [Europe PMC free article] [Abstract] [Google Scholar]
- Buxbaum A.R., Haimovich G., Singer R.H. In the right place at the right time: visualizing and understanding mRNA localization. Nat. Rev. Mol. Cell Biol. 2015;16:95–109. [Europe PMC free article] [Abstract] [Google Scholar]
- Chin A., Lécuyer E. RNA localization: making its way to the center stage. Biochim Biophys Acta Gen Subj. 2017;1861:2956–2970. [Abstract] [Google Scholar]
- Chung J., Gulcehre C., Cho K., Bengio Y. Empirical evaluation of gated recurrent neural networks on sequence modeling. arXiv. 2014 arXiv:1412.3555v1 [Google Scholar]
- Clementz M.A., Kanjanahaluethai A., O'Brien T.E., Baker S.C. Mutation in murine coronavirus replication protein nsp4 alters assembly of double membrane vesicles. Virology. 2008;375:118–129. [Europe PMC free article] [Abstract] [Google Scholar]
- Cock P.J.A., Antao T., Chang J.T., Chapman B.A., Cox C.J., Dalke A., Friedberg I., Hamelryck T., Kauff F., Wilczynski B., de Hoon M.J.L. Biopython: freely available Python tools for computational molecular biology and bioinformatics. Bioinformatics. 2009;25:1422–1423. [Europe PMC free article] [Abstract] [Google Scholar]
- Fazal F.M., Han S., Parker K.R., Kaewsapsak P., Xu J., Boettiger A.N., Chang H.Y., Ting A.Y. Atlas of subcellular RNA localization revealed by APEX-seq. Cell. 2019;178:473–490.e26. [Europe PMC free article] [Abstract] [Google Scholar]
- Gordon D.E., Jang G.M., Bouhaddou M., Xu J., Obernier K., O’Meara M.J., Guo J.Z., Swaney D.L., Tummino T.A., Huettenhain R. A SARS-CoV-2-Human protein-protein interaction map reveals drug targets and potential drug-repurposing. bioRxiv. 2020 10.1101/2020.03.22.002386. [Europe PMC free article] [Abstract] [CrossRef] [Google Scholar]
- Hagemeijer M.C., Vonk A.M., Monastyrska I., Rottier P.J., de Haan C.A. Visualizing coronavirus RNA synthesis in time by using click chemistry. J. Virol. 2012;86:5808–5816. [Europe PMC free article] [Abstract] [Google Scholar]
- Hunter J.D. Matplotlib: a 2D graphics environment. Comput. Sci. Eng. 2007;9:90–95. [Google Scholar]
- Kim D., Lee J.Y., Yang J.S., Kim J.W., Kim V.N., Chang H. The architecture of SARS-CoV-2 transcriptome. Cell. 2020;181:914–921.e10. [Europe PMC free article] [Abstract] [Google Scholar]
- Kingma, D., and Ba, J. (2014). Adam: a method for stochastic optimization. International Conference on Learning Representations.
- Knoops K., Kikkert M., Worm S.H.E.v.d., Zevenhoven-Dobbe J.C., van der Meer Y., Koster A.J., Mommaas A.M., Snijder E.J. SARS-coronavirus replication is supported by a reticulovesicular network of modified endoplasmic reticulum. PLoS Biol. 2008;6:e226. [Europe PMC free article] [Abstract] [Google Scholar]
- Liu X.Y., Wei B., Shi H.X., Shan Y.F., Wang C. Tom70 mediates activation of interferon regulatory factor 3 on mitochondria. Cell Res. 2010;20:994–1011. [Abstract] [Google Scholar]
- Mi H., Muruganujan A., Ebert D., Huang X., Thomas P.D. PANTHER version 14: more genomes, a new PANTHER GO-slim and improvements in enrichment analysis tools. Nucleic Acids Res. 2019;47:D419–D426. [Europe PMC free article] [Abstract] [Google Scholar]
- NCBI Resource Coordinators Database resources of the National Center for Biotechnology Information. Nucleic Acids Res. 2018;46:D8–D13. [Europe PMC free article] [Abstract] [Google Scholar]
- Ray D., Kazan H., Cook K.B., Weirauch M.T., Najafabadi H.S., Li X., Gueroussov S., Albu M., Zheng H., Yang A. A compendium of RNA-binding motifs for decoding gene regulation. Nature. 2013;499:172–177. [Europe PMC free article] [Abstract] [Google Scholar]
- Richter-Dennerlein R., Oeljeklaus S., Lorenzi I., Ronsör C., Bareth B., Schendzielorz A.B., Wang C., Warscheid B., Rehling P., Dennerlein S. Mitochondrial protein synthesis adapts to influx of nuclear-encoded protein. Cell. 2016;167:471–483.e10. [Europe PMC free article] [Abstract] [Google Scholar]
- Ryder P.V., Lerit D.A. RNA localization regulates diverse and dynamic cellular processes. Traffic. 2018;19:496–502. [Europe PMC free article] [Abstract] [Google Scholar]
- Salvetti A., Greco A. Viruses and the nucleolus: the fatal attraction. Biochim. Biophys. Acta. 2014;1842:840–847. [Europe PMC free article] [Abstract] [Google Scholar]
- Sanche S., Lin Y.T., Xu C., Romero-Severson E., Hengartner N., Ke R. The novel Coronavirus, 2019-nCOV, is highly contagious and more infectious than initially estimated. medRxiv. 2020 medrxiv.org/content/10.1101/2020.02.07.20021154v1 [Google Scholar]
- Seabold, S., and Perktold, J. (2010). Statsmodels: econometric and statistical modeling with Python. Proceedings of the 9th Python in Science Conference 2010, pp. 92–96.
- Somasundaran M., Zapp M.L., Beattie L.K., Pang L., Byron K.S., Bassell G.J., Sullivan J.L., Singer R.H. Localization of HIV RNA in mitochondria of infected cells: potential role in cytopathogenicity. J. Cell Biol. 1994;126:1353–1360. [Europe PMC free article] [Abstract] [Google Scholar]
- Su S., Wong G., Shi W., Liu J., Lai A.C.K., Zhou J., Liu W., Bi Y., Gao G.F. Epidemiology, genetic recombination, and pathogenesis of coronaviruses. Trends Microbiol. 2016;24:490–502. [Europe PMC free article] [Abstract] [Google Scholar]
- The Gene Ontology, Consortium The gene ontology resource: 20 years and still GOing strong. Nucleic Acids Res. 2019;47:D330–D338. [Europe PMC free article] [Abstract] [Google Scholar]
- Virtanen P., Gommers R., Oliphant T.E., Haberland M., Reddy T., Cournapeau D., Burovski E., Peterson P., Weckesser W., Bright J. SciPy 1.0: fundamental algorithms for scientific computing in Python. Nat. Methods. 2020;17:261–272. [Europe PMC free article] [Abstract] [Google Scholar]
- Williamson C.D., DeBiasi R.L., Colberg-Poley A.M. Viral product trafficking to mitochondria, mechanisms and roles in pathogenesis. Infect. Disord. Drug Targets. 2012;12:18–37. [Europe PMC free article] [Abstract] [Google Scholar]
- Woo P.C., Huang Y., Lau S.K., Yuen K.Y. Coronavirus genomics and bioinformatics analysis. Viruses. 2010;2:1804–1820. [Europe PMC free article] [Abstract] [Google Scholar]
- Wu F., Zhao S., Yu B., Chen Y.M., Wang W., Song Z.G., Hu Y., Tao Z.W., Tian J.H., Pei Y.Y. A new coronavirus associated with human respiratory disease in. China Nat. 2020;579:265–269. [Europe PMC free article] [Abstract] [Google Scholar]
- Wu H.Y., Brian D.A. Subgenomic messenger RNA amplification in coronaviruses. Proc. Natl. Acad. Sci. USA. 2010;107:12257–12262. [Europe PMC free article] [Abstract] [Google Scholar]
- Wu K.E., Parker K.R., Fazal F.M., Chang H.Y., Zou J. RNA-GPS predicts high-resolution RNA subcellular localization and highlights the role of splicing. RNA. 2020;26:851–865. [Europe PMC free article] [Abstract] [Google Scholar]
Full text links
Read article at publisher's site: https://doi.org/10.1016/j.cels.2020.06.008
Read article for free, from open access legal sources, via Unpaywall:
http://www.cell.com/article/S2405471220302374/pdf
Citations & impact
Impact metrics
Citations of article over time
Article citations
Post-Acute Sequelae and Mitochondrial Aberration in SARS-CoV-2 Infection.
Int J Mol Sci, 25(16):9050, 21 Aug 2024
Cited by: 0 articles | PMID: 39201736 | PMCID: PMC11354507
Review Free full text in Europe PMC
Trafficking of mitochondrial double-stranded RNA from mitochondria to the cytosol.
Life Sci Alliance, 7(9):e202302396, 02 Jul 2024
Cited by: 2 articles | PMID: 38955468 | PMCID: PMC11220484
Mitochondria in COVID-19: from cellular and molecular perspective.
Front Physiol, 15:1406635, 21 Jun 2024
Cited by: 0 articles | PMID: 38974521
Review
Mechanism, structural and functional insights into nidovirus-induced double-membrane vesicles.
Front Immunol, 15:1340332, 11 Jun 2024
Cited by: 0 articles | PMID: 38919631 | PMCID: PMC11196420
Review Free full text in Europe PMC
Focusing on mitochondria in the brain: from biology to therapeutics.
Transl Neurodegener, 13(1):23, 17 Apr 2024
Cited by: 3 articles | PMID: 38632601 | PMCID: PMC11022390
Review Free full text in Europe PMC
Go to all (107) article citations
Data
Data behind the article
This data has been text mined from the article, or deposited into data resources.
BioStudies: supplemental material and supporting data
GEO - Gene Expression Omnibus
- (1 citation) GEO - GSE116008
RefSeq - NCBI Reference Sequence Database
- (2 citations) RefSeq - NC_006273.2
Similar Articles
To arrive at the top five similar articles we use a word-weighted algorithm to compare words from the Title and Abstract of each citation.
Decoding SARS-CoV-2 hijacking of host mitochondria in COVID-19 pathogenesis.
Am J Physiol Cell Physiol, 319(2):C258-C267, 08 Jun 2020
Cited by: 214 articles | PMID: 32510973 | PMCID: PMC7381712
SARS-CoV-2 Is Restricted by Zinc Finger Antiviral Protein despite Preadaptation to the Low-CpG Environment in Humans.
mBio, 11(5):e01930-20, 16 Oct 2020
Cited by: 93 articles | PMID: 33067384 | PMCID: PMC7569149
A Structural View of SARS-CoV-2 RNA Replication Machinery: RNA Synthesis, Proofreading and Final Capping.
Cells, 9(5):E1267, 20 May 2020
Cited by: 286 articles | PMID: 32443810 | PMCID: PMC7291026
Review Free full text in Europe PMC
Funding
Funders who supported this work.
Chan Zuckerberg Initiative
Howard Hughes Medical Institute
NHGRI NIH HHS (6)
Grant ID: K99 HG010910
Grant ID: R00 HG010910
Grant ID: RM1 HG007735
Grant ID: RM1 HG010023
Grant ID: T32 HG000044
Grant ID: R01 HG004361
NIA NIH HHS (1)
Grant ID: P30 AG059307
NIMH NIH HHS (1)
Grant ID: U01 MH098953
NIMHD NIH HHS (1)
Grant ID: R21 MD012867