Abstract
Free full text

A compendium of RNA-binding motifs for decoding gene regulation
Associated Data
Abstract
RNA-binding proteins are key regulators of gene expression, yet only a small fraction have been functionally characterized. Here we report a systematic analysis of the RNA motifs recognized by RNA-binding proteins, encompassing 205 distinct genes from 24 diverse eukaryotes. The sequence specificities of RNA-binding proteins display deep evolutionary conservation, and the recognition preferences for a large fraction of metazoan RNA-binding proteins can thus be inferred from their RNA-binding domain sequence. The motifs that we identify in vitro correlate well with in vivo RNA-binding data. Moreover, we can associate them with distinct functional roles in diverse types of post-transcriptional regulation, enabling new insights into the functions of RNA-binding proteins both in normal physiology and in human disease. These data provide an unprecedented overview of RNA-binding proteins and their targets, and constitute an invaluable resource for determining post-transcriptional regulatory mechanisms in eukaryotes.
RNA-binding proteins (RBPs) regulate numerous aspects of co- and post-transcriptional gene expression, including RNA splicing, polyadenylation, capping, modification, export, localization, translation and turnover1,2. Sequence-specific associations between RBPs and their RNA targets are typically mediated by one or more RNA-binding domains (RBDs), such as the RNA recognition motif (RRM) and hnRNPK-homology (KH)domains. The human genome, for example, encodes 239 proteins with RRM domains and 38 with KH domains, among a total of 424 known and predicted RBPs3. Canonical RBDs typically bind short, single-stranded (ss)RNA sequences3,4, but some also recognize structured RNAs5.
A minority of the thousands of RBD-containing proteins in eukaryotic genomes have been studied in detail, and the assays used to generate the motifs are heterogeneous. For example, 15% of human, 8% of Drosophila and 3% of Caenorhabditis elegans RBD-containing proteins have known RNA-binding motifs3 (Supplementary Data 1). There are virtually no data on the sequence preferences of RBPs in most organisms, despite the fact that the high numbers of RBPs in some species (such as protist parasites) suggest that gene expression is mostly regulated post-transcriptionally6. The motifs for DNA-binding proteins can be highly similar for closely related proteins, allowing accurate inference of motifs7,8, and in some cases motifs can even be predicted on the basis of specific interactions between DNA-contacting amino acid residues and DNA bases9,10. In contrast, owing to the much higher flexibility of the RNA–protein interface for major types of RBPs, it has been questioned whether such RNA-binding recognition codes exist5. Altogether, the lack of motifs for the vast majority of RBPs across all branches of eukaryotes hinders analysis of post-transcriptional regulation.
To address this issue, we set out to identify binding motifs for a broad range of RBPs, spanning both different structural classes and different species. The resulting motifs represent an unprecedented resource for the analysis of post-transcriptional regulation across eukaryotes; provide insight into the function and evolution of both RBPs and their binding sites; reveal broad linkages among different post-transcriptional regulation processes; and uncover an unexpected role for a splicing factor in the control of transcript abundance that is mis-regulated in autism.
Large-scale analysis of RBPs
RNAcompete is an in vitro method for rapid and systematic analysis of RNA sequence preferences of RBPs11. It involves a single competitive binding reaction in which an RBP is incubated with a vast molar excess of a complex pool of RNAs. The protein is recovered by affinity selection and associated RNAs are interrogated by microarray and computational analyses. Here we used a newly designed RNA pool comprising ~240,000 short (30–41 nucleotides) RNAs that contains all possible 9-base nucleotide sequences (9-mers) repeated at least 16 times. For internal cross-validation, the pool was divided into two halves, each of which contained at least eight copies of all possible 9-mers, 33 copies of each 8-mer, and 155 copies of each 7-mer.
We initially determined the sequence preferences for 207 different RBPs, corresponding to seven different structural classes and representing the products of 193 unique RBP-encoding genes (in several cases, more than one isoform or protein fragment was analysed; Supplementary Data 2). Some proteins were measured more than once, resulting in 231 experiments. The analysed RBPs included 85 from human, 61 from Drosophila and an additional 61 from 18 other eukaryotes selected to be dissimilar to already profiled RBPs. Most RBP fragments analysed (148) contained all annotated RBDs in the protein in addition to 30–50 flanking residues. These fragments succeed more often than full-length proteins or individual RBDs in trial experiments (Supplementary Table 1) and yield data that are consistent with previously known motifs (see below).
Following protein binding microarray procedures12, we processed the data for each RNAcompete experiment to produce both Z and E scores for each individual 7-mer; these summarize the intensity and rank, respectively, of RNAs containing the 7-mer. For each experiment we also generated motifs and consensus sequences. Representative data are shown in Fig. 1a; the scatter plot displays Z scores and motifs for the two halves of the RNA pool for ZC3H10, a human protein with three CCCH zinc fingers that, to our knowledge, has no previously known motif. The vast majority of RBPs appear to bind target sequences in ssRNA, and none absolutely requires a specific RNA secondary structure, although 22 RBPs display a significant preference for (n = 7) or against (n = 15) predicted hairpin loops (see Supplementary Data 3). These findings are consistent with a previous analysis of in vivo binding data13 and with the observation that most RBDs fundamentally recognize ssRNA5. In almost all cases, E scores for 7-mers from the two halves of the RNAcompete pool for a given protein are more similar to each other than to those of other assayed proteins, highlighting the specificity and diversity of RBP sequence preferences (Fig. 1b, Supplementary Fig. 6 and Supplementary Data 4).
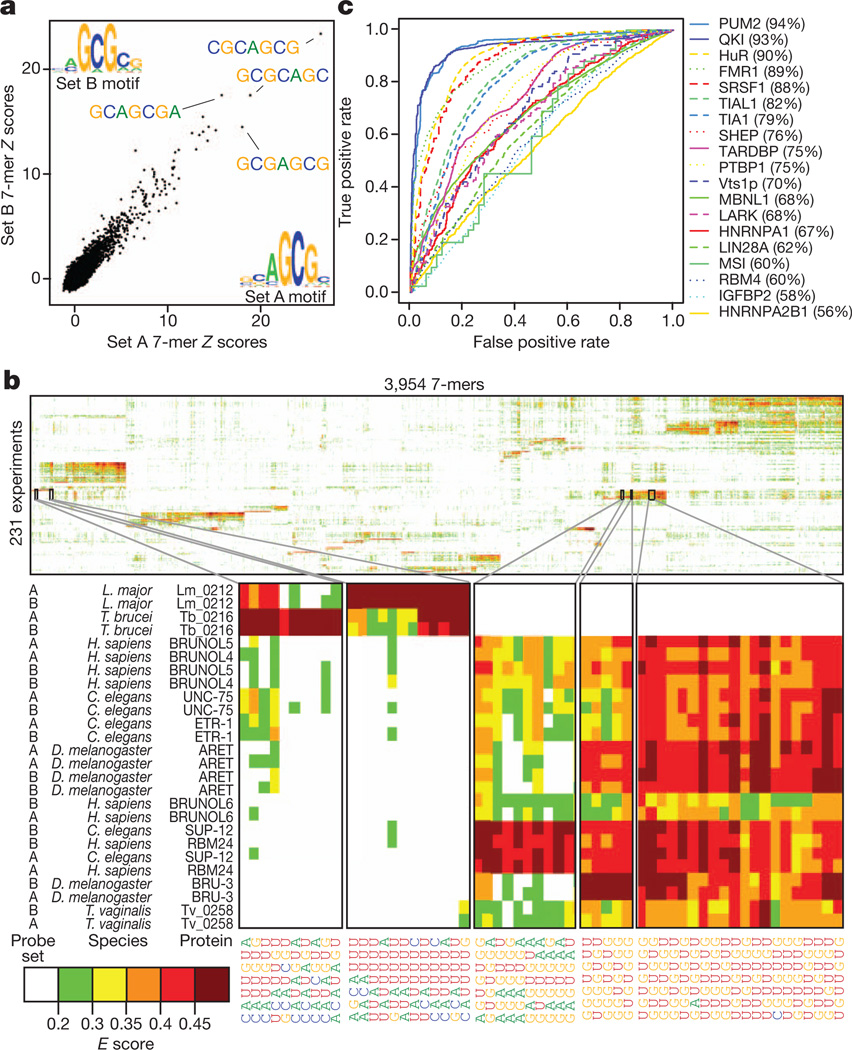
a, 7-mer Z scores and motifs for the two probe sets for ZC3H10. b, Two-dimensional hierarchical clustering analysis (Pearson correlation, average linkage) of E scores for 7-mers with E ≥ 0.4 in at least one experiment, with the two halves of the array kept as separate rows. Long systematic names have been shortened to species abbreviations and RNAcompete assay numbers. c, ROC curves showing discrimination of bound and unbound RNAs by the corresponding protein in vivo. The curve with the highest AUROC is shown if there are multiple in vivo data sets for a protein. FUS and TAF15 were excluded.
Of the 193 unique RBPs, 52 have previously identified consensus RNA-binding sequences. Most of these have obvious similarity to our RNAcompete-derived motifs (Supplementary Data 5; 35 very similar, six partial matches, and 11 discrepancies). Some discrepancies have no clear explanation, but may be due to differences between in vitro and in vivo data, different binding conditions, and/or the proteins analysed (for example, full-length versus RBDs).However, RNAcompete motifs are predictive of RNA sequences bound by the same proteins (or their close homologues) in vivo, as determined from data sets that we compiled from other studies (Fig. 1c; see Supplementary Table 2 for details). In some cases, the RNAcompete motif substantially outperforms the literature motif by AUROC (area under the ROC curve) analysis (Supplementary Fig. 2; values are in Supplementary Data 5): for example, for QKI (quaking), the AUROC for the RNAcompete motif was 93% versus 83% for the literature motif. We found only one instance in which the RNAcompete motif did not have a significant and positive AUROC to at least one corresponding in vivo data set: the RNAcompete motif for FUS produced an AUROC <0.5 when compared to in vivo crosslinking-based data for both FUS and its paralogue TAF15 (ref. 14). One possible explanation is that the consensus that we identified (CGCGC) contains no U residues, and therefore would not crosslink efficiently to protein. Collectively, these analyses demonstrate that the RNAcompete motifs are generally both accurate and functionally relevant.
Conservation of ancient motifs
Among the 207 RBPs we initially analysed, most yielded RNA-binding data distinct from that obtained from all other proteins (Fig. 1b and Supplementary Fig. 6). The major exception is that proteins with closely related RBDs typically yield very similar data. Figure 2 shows motifs for all of the RRM and KH domain proteins in this initial set, clustered by sequence identity among the RBDs. In numerous instances (shaded), groups of ancient families retain closely related sequence preferences. This is clearly seen in RNAcompete-derived motifs for families of proteins with previously characterized members, including the A2BP1/RBFOX1 (hereafter referred to as RBFOX1), BRUNO/ARET, and ELAV/HuR groups (see numbered insets in Fig. 2), as well as for proteins with previously uncharacterized RNA-binding preferences. For example, all RBPs in the SUP12–RBM24–RBM38 cluster (Fig. 2, inset 2) prefer similar (G1U)-rich sequences. These nematode, mouse and human proteins are regulators of muscle development15,16, indicating both biochemical and functional conservation.
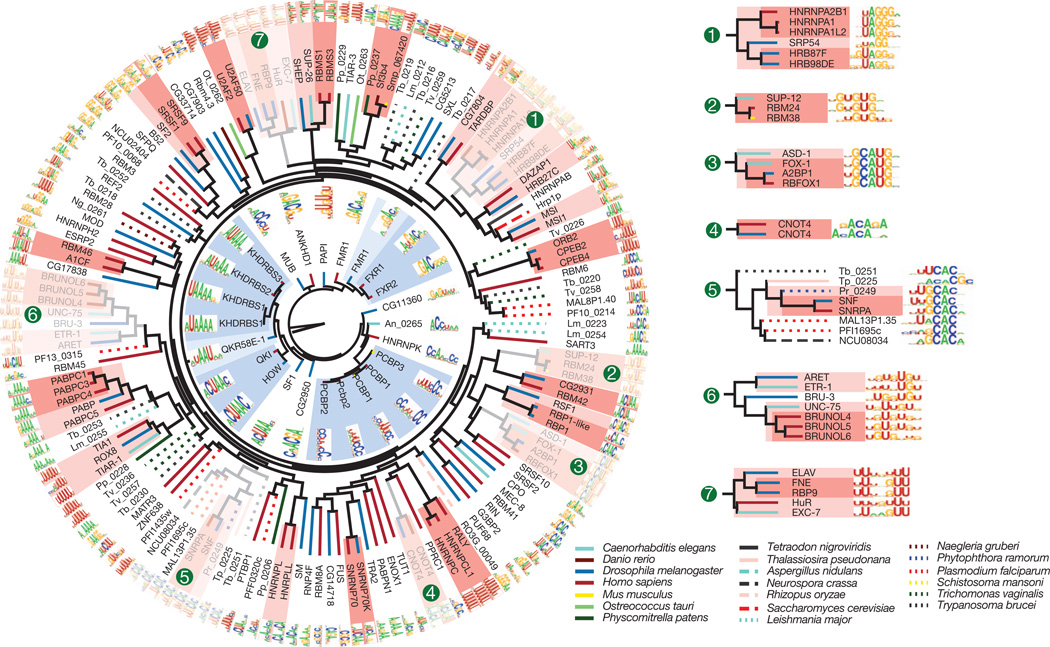
The dendrograms represent complete linkage hierarchical clustering of RBPs by amino acid sequence identity in their RBDs. Line colours indicate species of origin of each protein, and shading indicates clades in which all sequences are more than 70% (dark) or 50% (light) identical.
Subtle differences between more distantly related proteins are found. A notable instance is the group of distant relatives of the metazoan spliceosomal U1 snRNP-binding protein SNRPA/SNF; family members from fungi, protists and algae have all maintained the presumed ancestral CAC core-recognition specificity17, but differ in their preference for flanking nucleotides (Fig. 2, inset 5). The marked change in the central ‘UCAC’ in the unusual consensus in Trypanosoma brucei (HUUCACR) seems to correspond to the unusual T. brucei U1 loop sequence (CAUCAC versus AUUGCAC in most other species).
Quantification of the relationship between RBD sequence identity and RNA-binding motifs by three different metrics shows that, on average, amino acid sequence identity higher than ~70% yields very similar motifs (Fig. 3a). Thus, two proteins for which their RBDs are >70% identical are likely to have a similar, if not identical, RNA sequence specificity. Motifs remain similar at 50% identity. This observation is of tremendous practical value, because it provides a simple heuristic by which the RNA sequence preferences of previously uncharacterized RBPs can be reliably inferred. Anecdotally, it has been reported that specific pairs of closely related RBPs often bind similar sequences (for example, human NOVA1 and NOVA2 and Drosophila Pasilla18); to our knowledge, however, neither the generality nor the precise limitations of this observation have been previously established. Indeed, the heterogeneity of previous data may have complicated comparisons between motifs; for example, very different motifs have been previously described for different HNRNPA family members from human and Drosophila19–22, whereas the RNAcompete motifs for the same proteins are closely related (Fig. 2, inset 1).
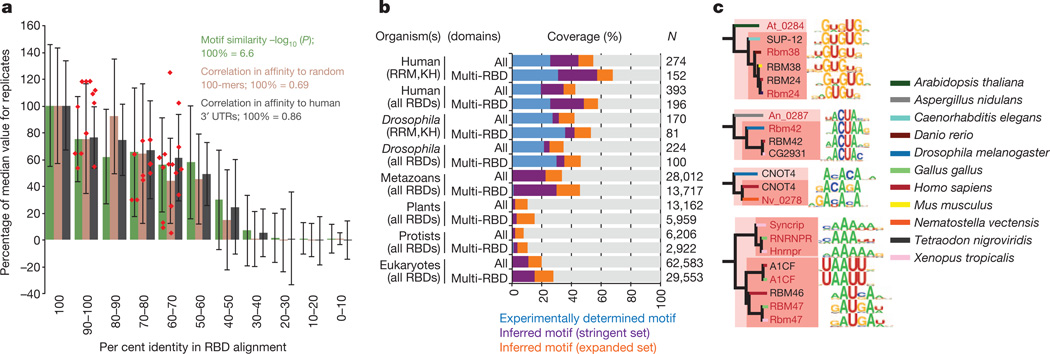
a, Motif similarity versus per cent amino acid sequence identity in all RBDs for pairs of proteins. Motif similarity scored using STAMP47 Pearson-based log10(E value), correlation between PFM affinity scores against 10,000 random-sequence 100-mers, or human 3′ UTRs (for human RBPs). Columns indicate average; error bars indicate standard deviation. Red points: new proteins analysed (see c). b, Stacked bars indicate proportion of each category of RBP encompassed by experimentally determined motifs or inferred motifs using stringent (RNAcompete motifs, ≥70% identity) or expanded criteria (RNAcompete and literature motifs, ≥50% identity) in 288 eukaryotes (Supplementary Data 9). ‘Multi-RBD’ and “All” indicate proteins with >1 or >0 RBDs, respectively. c, Validation of motifs predicted for proteins at 61–96% amino acid identity (red text indicates validation motifs).
If we assume that a closely related RNA motif will be bound by any protein that has >70% sequence identity in its RBDs to those in one of the 207 proteins that we analysed, then the RNAcompete data collectively capture observed or inferred motifs for 57% of all human and 30% of all metazoan RBPs that contain multiple RBDs (which are most likely to bind RNA in a sequence-specific manner) (Fig. 3b and data not shown). Furthermore, if we incorporate previously described motifs compiled from the literature3, and use a threshold of 50% identity between RBDs (a level at which the motifs are typically related, albeit often not identical), then we are able to additionally infer binding preferences for,10%ofRBPs even in plants and protists, despite only 3 and 25 proteins, respectively, having been analysed experimentally (Fig. 3b). We tested the accuracy of these heurisitics by performing RNAcompete analysis of 12 additional proteins from diverse species that are 61–96%identical to proteins with novel motifs that were among the 207 RBPs. These new motifs were highly similar (Fig. 3a, c), even those from distant eukaryotic groups (for example, metazoans versus plants or fungi). Using a cutoff of 70%sequence identity between RBDs, we have systematically mapped motifs across 288 sequenced eukaryotes. This compendium is available in a searchable online database, cisBP-RNA (catalogue of inferred sequence binding preferences for RNA) (http://cisbp-rna.ccbr.utoronto.ca/).
Sequence conservation of motif matches
To investigate the functional relevance of the motifs, we identified strong motif matches within three likely regulatory regions of human pre-mRNAs (5′ untranslated regions (UTRs), 3′ UTRs, and/or alternative exons with flanking introns), and assessed their degree of conservation. Matches to motifs for 49 RBP families (defined on the basis of 70% identity in the RBDs), representing almost two-thirds of the human RBPs (104 of 165) with measured or inferred motifs (using 70% RBD identity), displayed a significant increase (false discovery rate (FDR) <0.01) in conservation relative to immediate flanking sequences, in at least one of the regions that we examined (Fig. 4a). Furthermore, there is an inverse relationship between the degeneracy of columns within an RNAcompete motif and the evolutionary conservation of the matching bases within the predicted binding site in transcripts, indicating that there is conservation of motif matches at these sites23 (Fig. 4b and Supplementary Fig. 5). We conclude that a significant fraction of potential RBP binding sites in regulatory regions are under purifying selection.
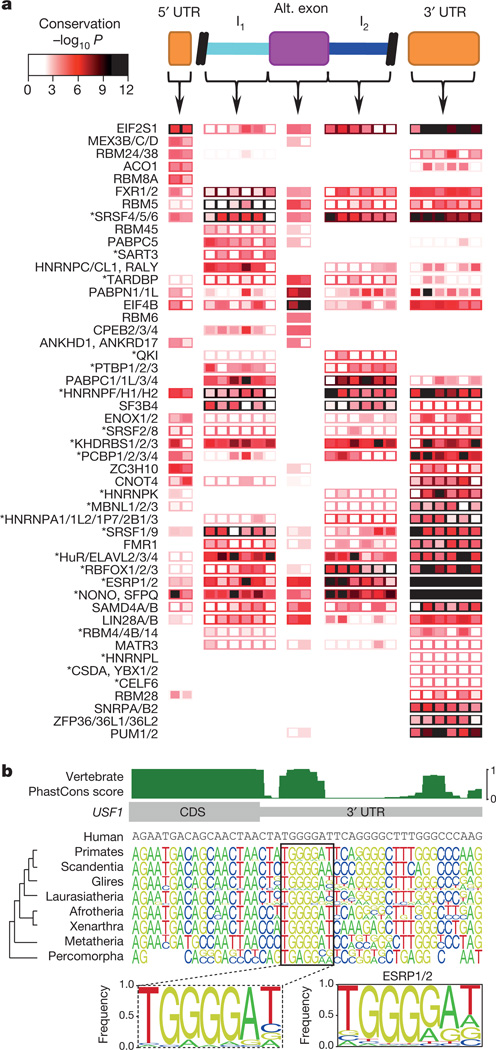
a, Heat map showing conservation in 50-nucleotide bins (columns) in regions indicated at the top of the panel. Rows represent the most significant motif for indicated protein family (see Supplementary Table 4). Box fill: conservation score of the most conserved position in the motif for each bin. Border colour: conservation score when the entire regulatory region is considered as a single bin. Asterisks indicate known splicing factors. b, Alignment of vertebrate sequences over the ESRP1/2 site in the USF1 3′ UTR. Sequence logos are shown for major branches of vertebrate taxonomy. Dashed box: motif derived from the full alignment. The RNAcompete motif for ESRP1/2 is shown to the right.
Often the regulatory region(s) in which a motif is conserved are consistent with the known function of the corresponding binding protein(s). For example, motifs for the alternative splicing factorsRBFOX1, RBFOX2 and RBFOX3 (ref. 4) are conserved in introns downstream of alternative exons, whereas sites for the stability/translation factors PUM1 and PUM2 are most highly conserved in 3′ UTRs24,25 (Fig. 4a). Furthermore, a striking outcome of the conservation analysis is that many proteins with well-defined roles in splicing (those with an asterisk in Fig. 4a) also have conserved motif matches in 3′ UTRs, suggesting more diverse regulatory roles for these factors. Indeed, dual functions for splicing regulators in 3′-end poly-A site selection and mRNA transport have been described26,27, and dual roles for RBPs in the control of splicing and stability are emerging28–30. This analysis suggests that RBP multi-functionality may be more widespread than previously appreciated; motifs for most (38 out of 49) RBP families shown in Fig. 4a display significant conservation in more than one of the three regions examined.
Insights into RBP multi-functionality
The sequence conservation of RBP motif matches in transcripts indicates potential new regulatory associations, particularly those associated with the 3′ UTR (Fig. 4a). To systematically seek possible roles for RBPs in mRNA stability, we identified cases in which there is a relationship between (1) the appearance of one or more strong motifs for an RBP in the 3′ UTR, and (2) (anti-)correlation of the abundance of the transcript and the mRNA expression level of the RBP, over a diverse panel of different cell and tissue types (Fig. 5a, Supplementary Table 3 and Supplementary Data 7). If, for example, levels of transcripts with a binding site for an RBP are significantly anti-correlated with the transcript encoding the RBP, then the RBP is a putative negative regulator of mRNA stability. This analysis identified several known regulators of mRNA stability, including RBM4 and ELAVL1 (refs 31, 32), and correctly predicted the direction of their effect (destabilizing for RBM4 and stabilizing for ELAVL1; Fig. 5a). In other cases (for example, PUM1 and PUM2), the direction of the effect was counter to expectation33, indicating that correlation may reflect possible additional functional roles for these proteins and/or their binding motifs. Nonetheless, the stabilizing/destabilizing roles predicted from this analysis were, on average, closely correlated with genome-wide measurements of RNA stability obtained previously from a thio-U pulse–chase experiment22 (Fig. 5b), supporting a role for these proteins in the regulation of mRNA turnover.
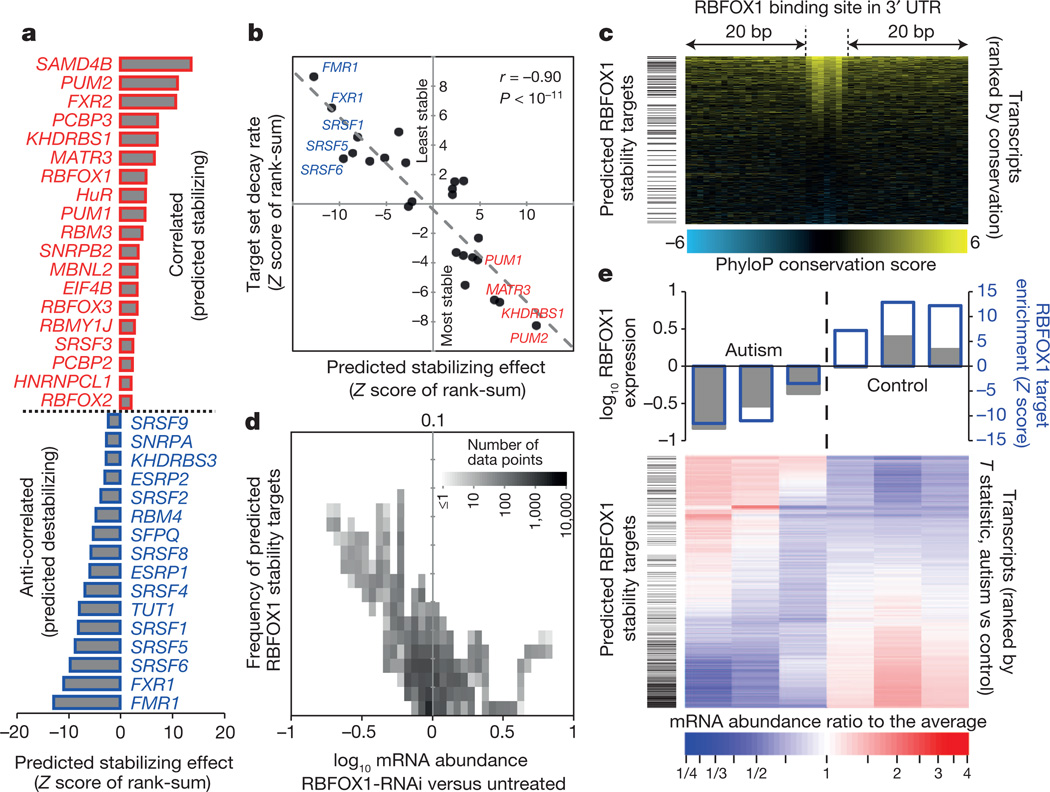
a, Significance (as rank-sum Z score) of bias that RBP motifs in 3′ UTRs of mRNAs confer towards correlated expression with the RBP’s mRNA (FDR <0.1). b, Scatter plot shows Z score (from a) versus rank-sum Z score of the same target set, with mRNAs ranked instead by decay rate in MDA-MB-231 cells, for expressed RBPs. c, Enrichment of predicted RBFOX1 stability targets (by ‘leading-edge’ analysis46) among transcripts with conserved RBFOX1 motifs. d, Density plot showing that RBFOX1 targets are enriched among transcripts most affected by RBFOX1 RNAi36. e, Relationship of mRNA expression levels in autism spectrum disorder brains to RBFOX1 expression and predicted RBFOX1 target status.
We used similar analyses to identify associations between RBP motifs and alternative splicing patterns. For example, consistent with previous results34,35, known splicing regulators, including RBFOX and PTB family members4, were associated with preferential exon inclusion or exclusion in a manner that correlated with the expression and binding location of the RBP (Supplementary Fig. 3 and Supplementary Data 7). Collectively, these analyses indicated previously unanticipated roles in alternative splicing and/or mRNA stability for known RBPs with well-defined sequence preferences as well as for uncharacterized RBPs.
This analysis predicts that RBFOX1 positively regulates mRNA stability (Fig. 5a). These targets tend to have the most conserved RBFOX1 sites in their 3′ UTRs (P < 10−4; one-sided Mann–Whitney U-test of ranks; Fig. 5c). To confirm this prediction, we examined published RNA-seq data following RBFOX1 knockdown by RNA interference (RNAi)36 and found that the predicted RBFOX1 stability targets were collectively reduced in abundance (P < 10−15, Fig. 5d). In these same data, the average reduction in transcript abundance increased with the number of motif matches in the first 300 nucleotides of the 3′ UTR, for all mRNAs (Supplementary Fig. 1a). This prediction is further supported by in vivo experiments in which the mRNA abundance of a reporter construct harbouring a single RBFOX1 site in the 3′ UTR increased, relative to an identical reporter containing a mutant RBFOX1 site, upon induction of RBFOX1 expression (Supplementary Fig. 1b).
Reduced levels of RBFOX1 in the brains of individuals with autism spectrum disorder have been associated with widespread changes in alternative splicing of exons associated with proximal RBFOX1 binding sites37. Notably, the same RNA-seq data used in ref. 37 also support a role for RBFOX1 in stabilizing its predicted mRNA targets (P < 10−30, Fig. 5e). Moreover, genes encoding transcripts with predicted 3′ UTR binding sites for RBFOX1 that show decreases in mRNA levels in autism spectrum disorder are significantly enriched for voltage-gated ion channels, particularly potassium channels (Supplementary Fig. 4), indicating that reduction of the stability of RBFOX1 targets may affect nervous-system-specific processes. This example illustrates how our compendium of RBP recognition motifs can suggest novel roles for specific RBPs in post-transcriptional regulation, and can thus also shed new light on their roles inhuman disease.
Discussion
Learning the patterns of sequence features that dictate global gene regulation remains a major challenge in computational biology2,38,39. The analyses above show that RBP motifs can be readily used to infer human post-transcriptional regulation mechanisms, and can explain evolutionary constraints found within both coding and non-coding regions of transcripts. We anticipate that the same will be true in other species: for example, we have examined data sets measuring translation40, stability41 and localization42 of transcripts in the early Drosophila embryo, obtaining dozens of significant associations between the presence of motif matches and specific regulatory outcomes (Supplementary Data 8). The fact that many RBP motifs have roughly the same information content as motifs of metazoan DNA-binding proteins43, yet face a much smaller search space (for example, a typical human 3′ UTR is <750 nucleotides in length), suggests that RBPs may have a reduced requirement for cooperative interactions to achieve high specificity, relative to transcription factors43.
The functions and evolution of RBPs remain largely unexplored, particularly with regard to their sequence specificity, whereas the number of putative RBPs continues to grow44. Our observations suggest that by profiling a relatively small number of RBPs it should be possible to broadly assess RBP sequence preferences across all eukaryotes. We caution that motif inference based on RBD identity alone is only a first approximation. Nonetheless, inference by simple protein identity is particularly valuable for those RBPs for which it may not be possible to derive recognition codes5. This compendium of motifs provides a valuable resource for furthering our understanding of interactions between RBPs and regulatory sequences, mechanisms of post-transcriptional regulation, and physiological and disease processes.
METHODS SUMMARY
We performed RNAcompete experiments, data processing, motif derivation and comparisons to in vivo data sets as previously described11 with modifications (see Methods). We determined amino acid sequence identity after multiple alignment of concatenated RBD sequences using clustalOmega45. For sequence scans, we performed a one-sided Z test for each motif on its sequence scores, and defined ‘strong motif matches’ as those with scores significantly higher than the mean (FDR <0.1, corrected for all motifs). We used relative PhyloP scores as a measure of conservation. ‘Predicted target set’ refers to genes with strong motif matches that are also the most significantly associated by expression, using leading-edge analysis46. Details are found in the Methods and Supplementary Information.
Supplementary Material
Supplementary Data 1
Supplementary Methods
Supplementary Data 2
Supplementary Data 3
Supplementary Data 4
Supplementary Data 5
Supplementary Data 6
Supplementary Data 7
Supplementary Data 8
Supplementary Data 9
Acknowledgements
We thank H. van Bakel for computational support, A. Ramani and J. Calarco for discussions, Y. Wu, G. Rasanathan, M. Krishnamoorthy, O. Boright, A. Janska, J. Li, S. Talukder, A. Coteand S. Votruba for technical assistance, L. Sutherland for purchasing RBM5 protein and for feedback on the manuscript, S. Jain for software modified to create Fig. 2, and N. Barbosa-Morais for generating cRPKM values from autism RNA-seq data. We thank M. Kiledjian (PCBP1 and PCBP2), J. Stevenin (SRSF2 and SFRS7), S. Richard (QKI), M. Gorospe (TIA1), B. Chabot (SRSF9), A. Berglund (MBNL1), F. Pagani (DAZAP1), A. Bindereif (HNRNPL), M. Freeman (HNRNPK), E. Miska (LIN28A), K. Kohno (YBX1), M. Garcia-Blanco (PTBP1), R. Wharton (PUM-HD), C. Smibert (Vts1p) and M. Blanchette (Hrb27C, Hrb87F and Hrb98DE) for sending published constructs. This work was supported by funding from NIH (1R01HG00570 to T.R.H. and Q.D.M., R01GM084034 to K.W.L.), CIHR (MOP-49451 to T.R.H., MOP-93671 to Q.D.M., MOP-125894 to Q.D.M. and T.R.H., MOP-67011 to B.J.B., and MOP-14409 to H.D.L.), and the Intramural Program of the NIDDK (DK015602-05 to E.P.L.). K.B.C. and S.G. hold NSERC Alexander Graham Bell Canada Graduate Scholarships. M.T.W. was funded by fellowships from CIHR and CIFAR. H.S.N. holds a Charles H. Best Fellowship and was funded partially by awards from CIFAR to T.R.H. and B.J.F. M.I. is the recipient of an HFSP LT Fellowship.
Footnotes
Supplementary Information is available in the online version of the paper.
Author Contributions D.R., H.K., K.B.C., M.T.W. and H.S.N. made unique, essential and extensive contributions to the manuscript, and are ordered by amount of time and effort contributed. D.R. and H.K. developed most of the laboratory and computational components of RNAcompete, respectively. D.R., H.Z., A.Y., H.N., L.H.M., S.A.S., C.A.Y., S.M.K., B.N., D.M., W.L., R.S.L. and M.Q. cloned, expressed and purified the proteins. D.R. ran the RNAcompete assays, including data extraction. H.K. and K.B.C. processed the data, H.K. and K.B.C. generated motifs, and H.K., K.B.C., M.T.W. and H.S.N. performed the motif analyses. H.K. assembled the in vivo protein-RNA data sets. L.H.M. and R.K.D. performed and analysed RIP-seq data. K.B.C. developed the supplementary website and Figs 1 and and22 with assistance from H.K. and M.T.W. M.T.W. and M.A. created the cisBP-RNA database. M.T.W., H.S.N. and T.R.H. created Fig. 3. H.S.N. performed the analyses of human splicing, RNA stability data and human sequence conservation, and created Figs 4 and and5.5. M.I. and S.G. generated and analysed RNA-seq data and S.G. performed reporter-based RNA stability assays. X.L. performed Drosophila data analysis. H.D.L., F.P., A.H.C., R.P.C., B.J.F., R.A.A., K.W.L., L.O.F.P., E.P.L., B.J.B. and A.G.F. helped organize and support the project, and provided feedback on the manuscript. B.J.F., B.J.B. and A.G.F. provided critical advice and commentary on data analysis. Q.D.M. and T.R.H. conceived of the study, supervised the project and wrote the manuscript with contributions from D.R., H.K., K.B.C., B.J.B., A.F. and H.S.N.
Author Information Raw and processed microarray data are available at GEO (http://www.ncbi.nlm.nih.gov/geo/) under accession number GSE41235. The derived motifs and results of analyses are available at http://hugheslab.ccbr.utoronto.ca/supplementary-data/RNAcompete_eukarya/.
The authors declare no competing financial interests.
References
Full text links
Read article at publisher's site: https://doi.org/10.1038/nature12311
Read article for free, from open access legal sources, via Unpaywall:
https://www.ncbi.nlm.nih.gov/pmc/articles/PMC3929597
Citations & impact
Impact metrics
Citations of article over time
Alternative metrics
Smart citations by scite.ai
Explore citation contexts and check if this article has been
supported or disputed.
https://scite.ai/reports/10.1038/nature12311
Article citations
A bioinformatic approach for the prediction and functional classification of Toxoplasma gondii long non-coding RNAs.
Sci Rep, 14(1):27687, 12 Nov 2024
Cited by: 0 articles | PMID: 39533086 | PMCID: PMC11557611
Circular RNA landscape in extracellular vesicles from human biofluids.
Genome Med, 16(1):126, 31 Oct 2024
Cited by: 0 articles | PMID: 39482783 | PMCID: PMC11526565
The MTR4/hnRNPK complex surveils aberrant polyadenylated RNAs with multiple exons.
Nat Commun, 15(1):8684, 17 Oct 2024
Cited by: 0 articles | PMID: 39419981 | PMCID: PMC11487169
ADAR3 modulates neuronal differentiation and regulates mRNA stability and translation.
Nucleic Acids Res, 52(19):12021-12038, 01 Oct 2024
Cited by: 0 articles | PMID: 39217468 | PMCID: PMC11514483
GenerRNA: A generative pre-trained language model for de novo RNA design.
PLoS One, 19(10):e0310814, 01 Oct 2024
Cited by: 0 articles | PMID: 39352899 | PMCID: PMC11444397
Go to all (879) article citations
Other citations
Data
Data behind the article
This data has been text mined from the article, or deposited into data resources.
BioStudies: supplemental material and supporting data
GEO - Gene Expression Omnibus
- (1 citation) GEO - GSE41235
Similar Articles
To arrive at the top five similar articles we use a word-weighted algorithm to compare words from the Title and Abstract of each citation.
Chromatin environment, transcriptional regulation, and splicing distinguish lincRNAs and mRNAs.
Genome Res, 27(1):27-37, 07 Dec 2016
Cited by: 128 articles | PMID: 27927715 | PMCID: PMC5204342
ELAV multimerizes on conserved AU4-6 motifs important for ewg splicing regulation.
Mol Cell Biol, 25(17):7580-7591, 01 Sep 2005
Cited by: 41 articles | PMID: 16107705 | PMCID: PMC1190278
A deep boosting based approach for capturing the sequence binding preferences of RNA-binding proteins from high-throughput CLIP-seq data.
Nucleic Acids Res, 45(14):e129, 01 Aug 2017
Cited by: 8 articles | PMID: 28575488 | PMCID: PMC5737578
Protein Binding to Cis-Motifs in mRNAs Coding Sequence Is Common and Regulates Transcript Stability and the Rate of Translation.
Cells, 10(11):2910, 27 Oct 2021
Cited by: 6 articles | PMID: 34831133 | PMCID: PMC8616275
Review Free full text in Europe PMC
Funding
Funders who supported this work.
CIHR (5)
Grant ID: MOP-125894
Grant ID: MOP-67011
Grant ID: MOP-93671
Grant ID: MOP-49451
Grant ID: MOP-14409
Intramural NIH HHS (1)
Grant ID: Z01 DK015602-01
NCI NIH HHS (2)
Grant ID: P30 CA014520
Grant ID: R01 CA104708
NHGRI NIH HHS (2)
Grant ID: 1R01HG00570
Grant ID: R01 HG005700
NIDDK NIH HHS (1)
Grant ID: DK015602-05
NIGMS NIH HHS (6)
Grant ID: R01 GM051968
Grant ID: R01 GM084034
Grant ID: T32 GM008061
Grant ID: R01 GM058728
Grant ID: R01 GM114386
Grant ID: R01GM084034