Abstract
Free full text

Metastasis-Associated Lung Adenocarcinoma Transcript 1 (MALAT1) lncRNA Conformational Dynamics in Complex with RNA-Binding Protein with Serine-Rich Domain 1 (RNPS1) in the Pan-cancer Splicing and Gene Expression
Abstract
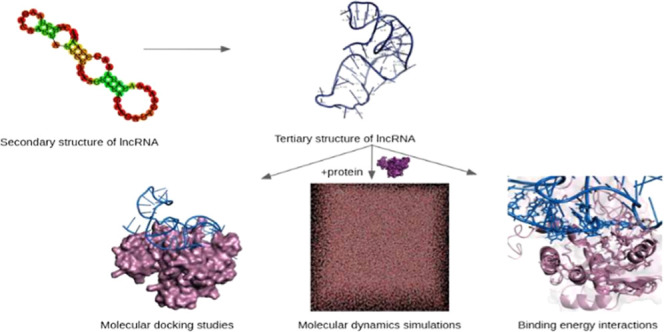
Alternative splicing events increase the transcriptomic and proteomic complexity in cancers. Overexpression of metastasis-associated lung adenocarcinoma transcript 1 (MALAT1), a highly conserved lncRNA, is widely known to promote cancer development, one mechanism for which may be through the regulation of alternative splicing and, thereby, gene expression. Its regulatory interactions with proteins have been a subject of much interest, yet little research has been carried out on the mechanisms adopted. It has been observed that MALAT1 binds to RNA-binding protein with serine-rich domain 1 (RNPS1), being colocalized in the nuclear speckles, and together, these two binding partners may regulate alternative splicing. Upregulated RNPS1 is predicted to play a key role in the pan-cancer development. Experimental tertiary structure of full-length MALAT1 is currently lacking despite the availability of the 3D structure of 3′ expression and nuclear retention element. We hypothesize that the computationally modeled tertiary structures of the specific binding motifs in the M-region, E-region, and full-length structures of MALAT1 may adopt a modular structure and bind to the RNPS1 loop region of RS/P domain involved in exon skipping, interacting in a manner fully consistent with the biochemical experiments. Extensive observations using the powerful molecular dynamics (MD) simulations of MALAT1 regions bound to RNPS1 suggested that all three regions form interactive, yet stable complexes. The ranking of the MM-GBSA- and MM-PBSA-derived binding free energies between these complexes corroborated well in the MD simulations and experiments. Energy decomposition analyses suggested that arginines in the RNPS1 protein are among the major contributors toward the binding free energies as calculated by MM-GBSA present in the Amber package; while among the nucleotides, the major contributors were nucleotides with G and A nucleobases, with more contributory effect in comparison to arginines, across the bound M-region, E-region, and full-length MALAT1. This suggests that specific purines play a greater role in the complex formation, in a loop-specific manner, and the more proactive approach in complexation tilts toward MALAT1. To the best of our knowledge, our studies are the first studies taking a unique approach, utilizing the binding motifs to deduce a tertiary structure of MALAT1, toward our understanding of the lncRNA–protein interactions, stability, and binding on a structural basis. The therapeutic implications of targeting this complex formation to regulate splicing and hence, oncogenesis, is further envisaged.
Introduction
Alternative splicing is a fundamental event in the regulation of gene expression in cancers, apart from constitutive splicing, which is efficiently performed by the spliceosome. Occurring due to exon skipping, which is the most common primary mode in mammals as well as other modes such as mutually exclusive exons, alternative 5′ and 3′ splice junction usage, thereby contributing to the infinite diversity of gene products (mRNAs, lncRNAs, and proteins), alternative splicing is governed by a plethora of trans-acting proteins binding in cis to the generated transcript.1 While the structure and function of the spliceosome has been studied extensively, using molecular dynamics (MD) simulations,2 there is a dearth of an understanding of the exact molecular and regulatory mechanisms involved, and the current picture of a “splicing code”3 is far from being perfect. This, coupled with the fact that several newly emerging molecules such as long noncoding RNAs (lncRNAs) may play a role in the splicing regulation of downstream genes, while themselves undergoing this RNA maturation step, renders the current state of our understanding even more nebulous. Hence, it is crucial to contemplate these lncRNA molecules and attempt to fully understand their interplay with other macromolecules.
Since the early 1990s, the general idea that the mammalian genomes can be transcribed pervasively4 began to take shape. High-throughput cDNA sequencing and chromosome-wide tiling arrays generated a remarkable variety of RNA content, being transcribed in a genome-wide manner. Encyclopedia of DNA Elements (ENCODE) and Functional Annotation of Mammals (FANTOM) consortia together led to the discovery of hundreds of lncRNAs across the kingdoms of life. These were identified in small numbers initially, for example, as X-inactive specific transcript (Xist) lncRNA involved in X-chromosomal inactivation,5,6 as H19 lncRNA, an onco-fetal gene,7 as Airn, antisense to the imprinted Igf2r gene,8 and as Drosophila heat shock-inducible 93D or hsrω gene involved in the regulation of several biological processes.9
While a majority of these lncRNAs discovered are yet to be functionally ascribed, research gleaned from studying a few of these macromolecules have started to reveal common mechanisms of action, especially in the realm of intricate RNA–protein interactions.10 Exemplifying this, Translocated in LipoSarcoma, an RNA-binding protein (RBP), is allosterically modulated by induced ncRNAs to regulate gene expression (through the inhibition of transcription) acting in cis,11 whereas XIST lncRNA drives the recruitment of an important silencing factor SPEN/SHARP, which binds to RNA,12 to spatially amplify its abundance across the inactive X chromosome, and mediates chromosome-wide gene silencing.13 Other lncRNAs have been shown to function as molecular decoys or sponges by functioning as RNA-dependent effectors and/or through the sequestration of the proteins. A classic example of this is muscle-specific lncRNA, Long Intergenic Non-Protein Coding RNA, Muscle Differentiation 1 (LINCMD1) which binds and sequesters miRNAs called miR-133 and miR-135 to regulate the expression of transcription factors such as Myocyte Enhancer Factor 2C (MEF2C) and Mastermind-like 1 (MAML1), which, in turn, regulate the muscle-specific gene expression.14 Moreover, the binding of zinc-finger transcription factor called CCHC-type zinc finger Nucleic Acid Binding Protein (CNBP) with Braveheart (Bvht) lncRNA is implicated in the heart cell lineage differentiation.15 Taking these examples into account, lncRNA–protein complexes have demonstrated regulatory activities at various stages of gene expression, and dissection of the structural basis of such interactions can provide a major understanding on their potential mechanisms of action.
LncRNAs also have the potential to assist proteins in performing the regulation of RNA splicing, stability, and degradation processes in the realm of pan-cancer gene expression and drug sensitivity/resistance.16,17 Furthermore, lncRNAs have also been observed to play a role in RNA splicing. Dysregulated RNA splicing is a characteristic feature present at a pan-cancer level.18 As structure is related to the functioning of macromolecules, structure-wise, secondary and tertiary structure formation has been discovered in several lncRNA molecules. Small sections of lncRNAs, termed modules, present in SRA,19 in COOLAIR(20) and in HOTAIR(21) have been shown to possess secondary structures capable of folding independently, in some cases, in a modular fashion. This reveals that specific regions of lncRNAs are capable of forming distinct functional structures. As an instance, Bvht lncRNA is thought to be a modular lncRNA, with the full 3-D fold possessing distinct binding modules for CNBP binding.15 Metastasis-associated lung adenocarcinoma transcript 1 (MALAT1) has been shown to form triple helical structure at its 3′end that protects it from exonucleases.22 In another study, putative expression and nuclear retention element (ENE)-like structures were found at the 3′ ends of MALAT1 and MENβ ncRNAs with U-rich internal loops.23 Furthermore, this 3′ end was observed to demonstrate structural conservation between human, zebrafish, and lizard, implying that this 3′ end might be critical for MALAT1 functioning.24 Another lncRNA, MEG3, was shown to form tertiary structure interactions with two distal motifs forming pseudoknot interactions between H11 and H27, using atomic force microscopy.25 UV cross-linking experiment and RNA modeling using RNAComposer was used to generate a 3D model of RepA functional domains adopting a compact state, and with tertiary contacts between subdomains D2 and D3.26
Among these lncRNAs, MALAT1 (also known as NEAT2), a highly conserved ~8000 nucleotide spliced noncoding RNA,27 has been widely studied. The mature transcript of this lncRNA is retained in the nucleus and is thought to function as a molecular scaffold for various macromolecular complexes between RNA-binding proteins (RBP) and RNA. This nuclear speckle-localized lncRNA28 can interact directly or indirectly with proteins, through being a target gene of Early Growth Response 1 (EGR1) transcription factor in pan-cancer drug sensitivity/resistance,16 through its aiding in the regulation of gene expression, through interaction with nuclear methyltransferase-like protein 16 (METTL16) contributing to its oncogenic activity29 and through interaction with serine/arginine (SR) proteins involved in the splicing regulation.30 Its ubiquitous expression across major tissues31 and increased stability of the transcribed RNA32 along with a strong promoter activity implies that MALAT1 has potentially crucial functional roles in the mammalian cells. Initially identified as a lncRNA with expression levels being higher in primary lung tumor with high metastatic potential,27MALAT1 has since then been reported in various lymphoid or solid tumors specifically correlating its elevated expression to metastasis and tumor progression. While the overexpression of MALAT1 has been studied extensively in various cancer types, its interaction with proteins has also been a subject of much interest. Yet little research, especially in the area of transient or permanent complex formation, has been carried out in this direction.
RNA-binding protein with serine-rich domain 1 (RNPS1) is a 305 amino acids long protein33 which is considered to be a part of the post-splicing multiprotein complex involved in the surveillance and nuclear export of mRNA. The gene encoding this serine-rich protein was observed to be upregulated and differentially expressed in 15 types of cancer, including cervical cancer, in a comprehensive study carried out by Saleembhasha and Mishra.17 Furthermore, in the same study, interaction of RNPS1 with lncRNAs such as PVT1 and RP11-1149O23.3 was hypothesized to possibly aid in the regulation of its mRNA translation in the cytoplasm and also in the AURKA translation regulation, respectively, providing novel insights into the regulation of pan-cancer gene expression. Another study unveiled the contribution of RNPS1 in cancer development by showcasing RNPS1-mediated alternative splicing favoring the Rac1b/RhoA signaling axis, possibly contributing to metastasis and invasion of cervical cancer cells.34 This demonstrated the involvement of RNPS1 in the regulation of several oncogenic alternative splicing events. Interestingly, Tripathi and colleagues30 showed that the interaction between MALAT1 and serine/arginine (SR) splicing factors was implicated in regulating the levels of phosphorylated SR proteins, specifically in the nuclear speckles. Through this mechanism, MALAT1 modulated the alternative splicing of pre-mRNAs in HeLa cells. Taken together, these observations indicate that the RBP, RNPS1, and the abundantly expressed MALAT1 lncRNA, both are involved in the alternative splicing events in the cancer cells and may potentially interact. Indeed, in the research carried out by Miyagawa et al.,35in vitro, certain fragments of MALAT1, namely, region M, region E, and full-length MALAT1, when bound with RNPS1, directed MALAT-1 localization to nuclear speckles. This suggested that MALAT1 might play important roles in key molecular processes inside the nucleus and the cell.
While various lncRNA–protein interaction studies have provided us with an understanding of the complex behavior of biological processes, subcellular localization, and the diverse functions, the implication of lncRNA–protein interactions in gene regulation and the structural basis of their actions is yet to be fully understood. Studies on RBPome dynamics have been enabled using the orthogonal organic phase separation (OOPS) approach for both coding and noncoding RNAs of more than 60 nucleotide bases in length.36 Conformational changes can be assessed using microsecond temporal resolution of HS-AFM.37 On the other hand, methods such as capture hybridization analysis of RNA targets (CHART)38 and cross-linking and immunoprecipitation (CLIP)39 have faced challenges as the expression levels of lncRNAs in mammalian cells are extremely low and hence, these are difficult to purify. These experimental limitations come to the fore upon noticing that only one MALAT1 region, the 3′ ENE and A-rich tract, is available as a tertiary structure in the Protein Data Bank (PDB) with ID 4PLX.40 Computational methods such as MD simulations have proven to be versatile in uncovering the structural landscape of biomolecular interactions involved in several diseases and deciphering the functional mechanisms. For instance, the structure-based effects of natural inhibitor (−)-epigallocatechin gallate (EGCG), an ATP-competitive inhibitor, on the conformational dynamics of GRP78, wherein its binding alters the conformation of nucleotide binding domain41 and subsequently modulates the conformation of substrate binding domain42 of GRP78, thereby inhibiting GRP78 activity in glioblastoma, have been determined through powerful MD simulations. In another study employing MD simulations in the studies of the spliceosome machinery, the long-range interaction channel between distal proteins of the spliceosome has been explored, supporting the crucial roles of Clf1 and Cwc2 splicing cofactors and Prp8 protein.43 Another study has incorporated MD simulations to showcase the spliceosome as a protein-directed ribozyme through functional dynamics of intron lariat spliceosome complex with Spp42 protein.44 In another instance, the partially folded 3′-ENE conformation and the ENE triplex core (4PLX) of MALAT1 have been subjected to MD simulations, and overall analysis pointed to a globally dynamic and a globally static behavior of these two forms, respectively.45 Spurred by these studies, we reasoned that a global and modular tertiary structure within regions of MALAT1 may exist, in addition to a well-defined secondary structure, and these regions could possibly interact with RBP, aiding in alternative splicing events in cancer. Herein, utilizing the powerful MD simulations and binding free energy (BFE) calculations through MM-GBSA to investigate the landscape of complex formation, dynamics, and binding interactions, we present a detailed structural and mechanistic study of MALAT1-RNPS1 lncRNA–protein complex interactions. Moreover, using the 3D structure of binding motifs, we have taken a unique approach, the first such approach to the best of our knowledge, in the tertiary structure prediction of motifs in MALAT1 regions found to bind experimentally to RNPS1.35 These detailed structural observations show that a vibrant and complex regulatory mechanism of lncRNA actions exists in the gene expression world.
Results
Motif Structures of MALAT1
As has been noted above, chemical probing through low-SHAPE reactivity has revealed 3′ end of MALAT1 to exist with a well-defined structure in a triple helix conformation and forming stable base pairing interactions.22 In fact, several modular folds, motifs, and domains of MALAT1 may exist along its length that are yet to be probed structurally. A study by Miyagawa et al.35 to understand the localization pattern of MALAT1 required construction of a series of plasmids encoding MALAT1 cDNA fragments of ~1 kb length each. These fragments, named from region A to region O, showed differential staining patterns, with regions E and M reported to be essential for MALAT1 localization to nuclear speckles. Furthermore, these regions were also shown to bind to RNPS1 using filter-binding assay in this same study and were observed to possess the nuclear speckle-localization signals, using SRSF2 (SC35) immunostaining.
On this basis of the colocalization of MALAT1 and RNPS1 to nuclear speckles as well as binding with each other, we surmised that these binding interactions may involve transient complex formation and critical interactions for the functioning in the regulation of gene expression. This requires a more in-depth study of these interactions at a structural level.
It is no surprise that the unavailability of the full-length experimental RNA structure, consisting of several thousands of nucleotides, is a barrier. This is compounded by the fact that the present in silico tools are incapable of modeling RNA tertiary structures with more than 500 nucleotide length. This is because of the computational intractability of the many-body problem with the RNA backbone being composed of six torsion angles. Furthermore, there is a dearth of information on the actual binding site/area within these regions of MALAT1. This limitation challenged us to employ a unique approach of working with short motifs gleaned from RBPmap computational tool (http://rbpmap.technion.ac.il/).46 According to the RBPmap developers, users can select motifs from a database of “223 human/mouse and 51 Drosophila melanogaster experimentally defined motifs, extracted from the literature as a position-specific scoring matrix (PSSM)”. This tool maps the binding site of RBP on lncRNA nucleotide sequences accurately by quantifying a significant match score for motifs in lncRNA sequences in terms of Z-score coupled to p-value of <0.05, when the sites are reported as the putative binding sites.46 False-positives are also removed or reduced while scoring significant matches. Toward this end, we considered the topmost binding motifs obtained through RBPMap for E-region (1961-3040 nucleotides length), M-region (6008-7011 nucleotides length), and full-length MALAT1 (1-8379 nucleotides) (Figure Figure11) for our studies and modeled the secondary structure of these motifs through RNAfold47 (Figure Figure22) as follows. First, these MALAT1 region sequences were provided as an input to RBPmap which scanned and searched for motifs that could possibly bind to RBPs. The motif with the highest binding specificity with the highest p-value (p-value < 0.05) was taken into account. A total of 56, 59, and 57 nucleotides were present in each topmost motif in E-region, M-region, and full-length MALAT1 region, respectively. The extracted sequences of these motifs were then taken as inputs for secondary structure modeling via RNAfold. The minimum free-energy structures of MALAT1 motifs, which are predicted using dynamic programming algorithms and loop-based energy models as given by Zuker and Stiegler48 under standard salt concentration of 1 M and 37 °C temperature with RNA parameter set according to the Turner free energy model,49 were considered as the working secondary structures. The secondary structure model of the E-region motif comprised two hairpin loops, three stems, one multibranched loop, and one open loop-like terminal structure; while the M-region motif had one hairpin loop, two stems, one multibranched loop, one bulge, and one open loop-like terminal structure, and the full-length-region motif contained two hairpin loops, three stems, one multibranched loop, and one open loop-like terminal structure. Both canonical Watson–Crick base pairing as well as noncanonical base pairing such as U-G were observed in all of these secondary structures.

Mapping of the E-region, M-region, and full-length regions on the whole sequence of MALAT1 (NCBI accession number NR_002819.4). Regions comprising nucleotides from 1961-3040 and 6008-7011 are considered as E- and M-regions, respectively.
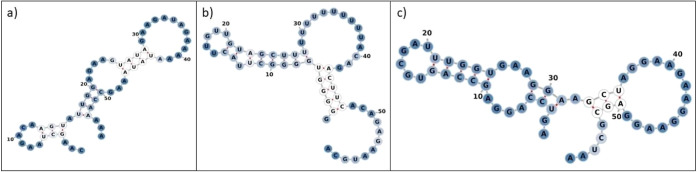
Minimum free-energy secondary structures of MALAT1 motifs (a) E-region; (b) M-region; and (c) full-length MALAT1, as obtained from RNAfold.
Afterward, the dot-bracket notations of secondary structures of the binding motifs of these regions, wherein a sequence of length n is represented by a string of equal length consisting of matching dots and brackets, were considered for tertiary structure prediction via RNAComposer50 (Figure Figure33). The overall architecture of the obtained tertiary structures of E-region and full-length region motifs was more compact, whereas that obtained for M-region motif had an unfolded/partially folded conformation at one end. These obtained structures were validated using MolProbity tool, used for the model validation of nucleic acid and protein structures. For a given RNA system, it gives a model score called clashscore based on all-atom contact, covalent geometry, and backbone conformational criteria along with sugar pucker analysis tailored for RNA. This clashscore, which is calculated based on the number of serious steric overlaps (>0.4 Å) per 1000 atoms, is measured with 100th percentile representing the best model among structures of comparable resolution and zeroth percentile as the worst model.51 For the models obtained via RNAComposer after energy minimization, MolProbity gave a clashscore of 99, 98, and 99 percentile for M-region, E-region, and full-length MALAT1 motif models, respectively, showing that the models obtained were accurate in their resolution. Visualizing all the three structures as right-handed helix with more than one helix in the motifs of M-region and full-length region through Dissecting the Spatial Structure of RNA (DSSR) structural analysis tool,52 we observed that the 3D structure of E-region motif had six non-Watson–Crick base pairs (bps) between the atoms out of a total of 16 bps, while that of the M-region motif had eight non-Watson–Crick bps out of a total of 16 bps. The 3D structure of the binding motif of the full-length region had six non-Watson–Crick bps out of a total of 15 bps.
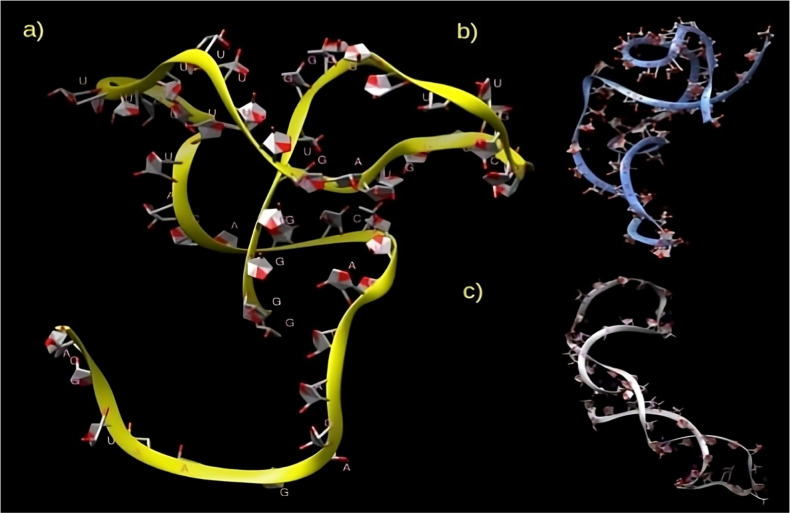
Energy minimized tertiary structures of MALAT1 region motifs predicted through RNAComposer (a) M-region motif in yellow color, (b) E-region motif in blue color, and (c) full-length region motif of MALAT1 in gray color.
To gain more confidence in our structural models, we extended these short motif regions by some nucleotides at each flanking end and used these extended structures, to include the sequence environment and in order to approach the currently available limitation of 500 nucleotides, and modeled them in RNAComposer. Superimposing the individually modeled tertiary structures to these new models, we found that the two structures matched closely for the E-region motif (Figure Figure44a). The M-region motif also aligned well (Figure Figure44b) barring a small portion at one terminal end which is not aligned well, most probably due to the effect of a long flexible loop at this terminal end as seen in Figure Figure33a, the presence/absence of neighboring residues in the full-length structure as well as sequence length on folding. This agreement, in general, provides us a degree of confidence in the correct modeling of the individual secondary and tertiary structures.
RNPS1 Structure Modeling
Human RNPS1 amino acid sequence retrieved from NCBI with accession number NP_006702 was fed to the Iterative Threading ASSEmbly Refinement (I-TASSER) web server,53 and default parameters were used. The model was generated on a eukaryotic ASAP core complex (PDB ID 4A8X, A chain) as a template with sequence identity of 99%. The ASAP complex is a complex of Acinus, RNPS1, and SAP18 subunits, involved in the transcriptional regulation, pre-mRNA splicing, and quality control.54 While the human RNPS1 protein sequence retrieved from NCBI is a longer sequence of 305 aa, that present in PDB ID 4A8X is a shorter sequence of 88 aa only, and so the longer sequence was subjected to computational modeling. Five models were generated, and the first model with high TM-score and highest sequence identity (99%) to the template was taken into account. Template modeling (TM) score represents a measure of structure accuracy when the native structure is known.55 RNPS1 model structure consisted of about eight alpha-helices and seven beta-strands along with several loops (Figure Figure55a).
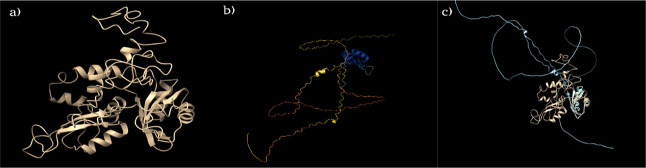
Structure of the RNPS1 protein. (a) RNPS1 structure (305 amino acids) modeled via I-TASSER using eukaryotic ASAP core complex with human RNPS1 (88 amino acids) subunit (PDB ID: 4A8X) as a template; (b) AlphaFold2-predicted RNPS1 structure (UniProt ID: Q15287) color-coded with pLDDT values showing per-residue model confidence ranging from dark blue (very high; pLDDT > 90) to light blue (high; 90 > pLDDT > 70) to yellow (low; 70 > pLDDT > 50) to orange (very low; pLDDT < 50); and (c) RNPS1 structure derived from I-TASSER (in golden color) superimposed on AlphaFold2-derived structure (in blue color) using Chimera visualization tool.
We also retrieved AlphaFold2 structure with UniProt ID Q15287 (Figure Figure55b) showing 100% sequence identity with NP_006702 (from NCBI). Generated with the neural network-based artificial intelligence technology, and MSA using homologous proteins, the RMSD upon superposition of I-TASSER-generated and AlphaFold2-generated structures was 60.472 Å across all 305 pairs! With 72 pruned atom pairs, the value was 0.780 Å (Figure Figure55c). An X-ray crystallographic structure for regions 159–244 of RNPS1 (not full-length) is available with PDB ID 4A8X, and this region was predicted with high pLDDT score by AlphaFold2. The rest of the AlphaFold2-predicted structure (not present in the PDB) was found to be highly unstructured, and per-residue model confidence in these locations showed a low to very low pLDDT score (Figure Figure55b). In these same unstructured regions, I-TASSER, which generates a model through ab initio folding based on replica-exchange Monte Carlo simulations, when homologous templates are not available, predicted a far more ordered structure, with the correct geometry as shown by Ramachandran plots.
Protein structural modeling relies on proper conformational sampling, energy scoring, and structural refinement which can be derived either from ab initio, knowledge-based, and/or hybrid approaches. AlphaFold256 predicts the structure of a protein based on multiple sequence alignments and coevolutionary information achieving near-experimental resolution of protein structure but faces the limitation or accuracy in predicting regions of proteins that have few intrachain contacts and when the median alignment depth is <30 sequences. The model predicted by AlphaFold2 for full-length RNPS1 was low-confidence in many parts (Figure Figure55b). We, therefore, employed I-TASSER, one of the most successful prediction methods in the community-wide CASP experiments, ranking number 1 as Zhang-server in CASP14 in the year 2020.53 This template-based method reassembles structural fragments from various threading templates using computational algorithm of replica exchange Monte Carlo simulations, and the solid pipeline predicts a highly reliable protein structure. We generated our RNPS1 model using this server, and the Ramachandran plot analysis of the energy-minimized predicted structure showed that the model structure was observed to display good stereochemical properties. Hence, we used the I-TASSER-generated structure for our further work.
RNPS1 Binding to the Structural Motifs of MALAT1 Uses Loops in RS/P Domain as Predominant Secondary Structures in the Interfaces
The work published by Saleembhasha and Mishra17 hypothesized the RNPS1 as a key coding gene upregulated in at least 15 types of cancer, and so, potentially involved in pan-cancer development. A separate study from the same laboratory postulated MALAT1 as a possible master regulator of genes involved in the pan-cancer multidrug resistance.16 Interestingly, RNPS1 (an RBP) was shown to influence MALAT1 localization to nuclear speckles, through an in vitro study, which showed disruption of MALAT1 localization through the depletion of RNPS1. In this same study, purified RNPS1 was also shown to bind to MALAT1 RNA, using a filter-binding assay.35 Accordingly, we were interested in understanding the structural basis of this interaction in order to gain more insights to decipher the laws of lncRNA interactions with macromolecules and understand the mechanistic aspects. Specifically, we wanted to know if RNPS1 binding affected the conformational dynamics of MALAT1, and vice versa. We also wanted to ascertain the binding sites and the contribution of intermolecular interactions to this binding. Therefore, in order to first generate a complex structure, we performed molecular docking of the 3D binding motifs of MALAT1 with RNPS1 using ClusPro57 with blind docking. We also performed blind docking with another tool, PatchDock.58 While PatchDock employs geometry-based rigid docking algorithm, ClusPro applies an energy-based rigid docking algorithm based on the fast Fourier transform correlation. This tool finds shape complementarity in molecules through docking transformations and performs root-mean-square deviation (RMSD) clustering to obtain the best scoring of the structure. We observed that the interacting sites were consistent in both the predictions, lending credence to our docking approach (Figure Figure66).
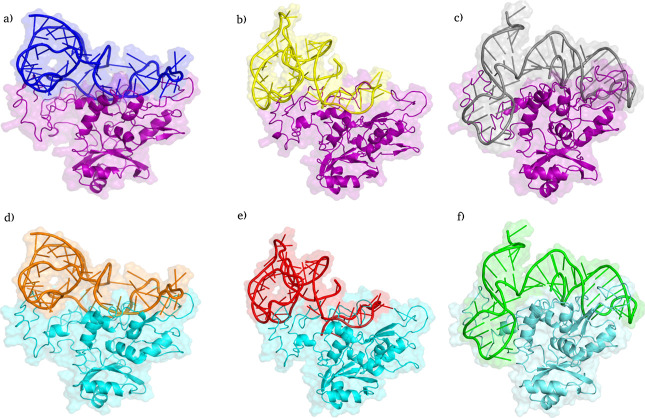
Upper panel: ClusPro-docked structures of (a) E-region motif of MALAT1 (in blue color) docked with RNPS1; (b) M-region motif of MALAT1 (in yellow color) docked with RNPS1 and; (c) full-length MALAT1 motif (in gray color) docked with RNPS1. RNPS1 protein structure is shown in purple color. Lower panel: PatchDock-docked structures of the (d) E-region motif of MALAT1 (in orange color) docked with RNPS1; (e) M-region motif of MALAT1 (in red color) docked with RNPS1 and; (f) full-length MALAT1 motif (in green color) docked with RNPS1. RNPS1 protein structure is shown in cyan color.
The modeled structures of MALAT1 motifs interacted with RNPS1 (Figure Figure66), through several hydrogen bonding and nonbonded interactions (Figure S1: E-region–RNPS1 interactions; Figure S2: M-region–RNPS1 interactions; and Figure S3: full-length-region-RNPS1 interactions). Interestingly, the binding site in RNPS1 was the arginine–serine/proline-rich (RS/P) domain, comprising residues 238–305 (UniProt ID Q15287). While the RNA-recognition motif (RRM) domain (161–240 residues) exhibits broad RNA-binding functions, the RS/P domain is involved in the protein–protein interactions and is observed to be necessary for interaction with hTra2β, nuclear localization, and exon-skipping.59 Since MALAT1 is also localized to nuclear speckles and is observed to bind to this same RS/P region in our blind docking studies, we were intrigued if the RS/P region can also bind RNA apart from proteins. Indeed, studies using UV-cross-linking60,61 show that the RS domain can potentially contact the pre-mRNA branchpoint and thus can directly bind to and interact with both RNA and protein.62 Another recent study observed RS regions of SRSF1 protein to exhibit binding preference for RNA rich in purines.63 This indicates that MALAT1 and RNPS1 may form a tight lncRNA–protein complex at the designated sites in an energetically favorable binding mode. While the most tight interaction with RNPS1 was that of the M-region motif, with a lowest energy score of −3984.2 kcal/mol, that of the lowest energy structure with the E-region motif and the motif of full-length MALAT1 were of the value of −3638.5 and −3403.5 kcal/mol, respectively, and hence, there was less tight binding than the former interaction. PatchDock scores also ranked these regions similarly. These energies from the docked structures ranged from the motifs of bound M-region (highest) to bound E-region to bound full-length MALAT1 (lowest) and fully conform to the independent experimental observations35 where the binding affinity as determined by filter-binding assay with M fragment RNA was much stronger as compared to E fragment RNA and full-length MALAT1.
Atomistic details of the docked conformations revealed a number of hydrogen bonding and nonbonded interactions between RNPS1 and MALAT1 regions. R34, K44, W263, R265, R271, R273, R278, R280, R285, R286, and R288 of RNPS1 were the common RNPS1 residues that showed hydrogen bonding with all of the MALAT1 regions studied (Figures S1–S3). Structural mapping showed that these arginines are enriched in the loop region of RNPS1. Our data also suggest that RNPS1 binding may aid in the compaction of both regions and full-length MALAT1 lncRNA, as seen from the docking energy analyses. These observations are fully in accordance with the in vitro study carried out by Miyagawa et al.35 as noted above, which our docking study corroborates well.
Intrinsic Global Dynamics of MALAT1 Regions Bound to RNPS1
In order to explore how RNPS1 binding affects MALAT1 conformation at the atomistic level and vice versa, we performed MD simulations simulating the in vivo conditions inside the living cells. MD simulations of three individual MALAT1 motif structures and the three docked complexes of these regions, respectively, were analyzed. Three plots, for free-form MALAT1 motifs, for MALAT1 motifs in complex, and for RNPS1 in complex were generated for each parameter (RMSD, RMSF, and Rg) over a simulation time of 100 ns for each system totalling 600 ns of MD production run. Considering the RMSD plots, the backbone atoms of RNPS1 (C, CA, N) and MALAT1 (P, O5′, C5′, C4′, C3′, and O3′) were taken into account. The free-form 3D motifs of MALAT1 regions showed a high RMSD value ranging from 0.5 (initial trajectories) to 2 nm, as expected (Figure Figure77a). In the docked complexes, in the case of MALAT1 RNA, while there is an initial rise in RMSD values in the full-length region, the conformations in all of the regions under study quickly stabilize over time at an average value of about 0.75 nm (M-region) to 1.5 nm (full-length region) (Figure Figure77b). Consequent upon complex formation with RNPS1, conformations of the same MALAT1 region motifs show lowered RMSD values, also as expected, as the mobility decreases when the molecules are in the bound form. Analyses using backbone atoms of RNPS1 conformations (Figure Figure77c) in the E-region–RNPS1 (red line) and full-length MALAT1-RNPS1 (green line) docked complexes showed an average RMSD of ~0.85 and ~0.75 nm, respectively. M-region–RNPS1 docked complex (black line) fluctuates between ~0.75 and ~0.95 nm during the first 25 ns simulation. After this initial period of fluctuation, the docked complex continues fluctuating with higher average values of ~1.1 and ~1.5 nm over the course of the simulation. These larger fluctuations in the M-region–RNPS1 docked complex might be attributed to the conformational dynamics of a highly dynamic region comprising amino acid residues 100–160 of this protein, as seen from the RMSF graph (Figure Figure88c), which may not interact with or is bound by the M-region of MALAT1, as is also evident from the absence of these amino acid residues in its PDBsum interaction map (Figure S2), and therefore exists as a freely flexible form.
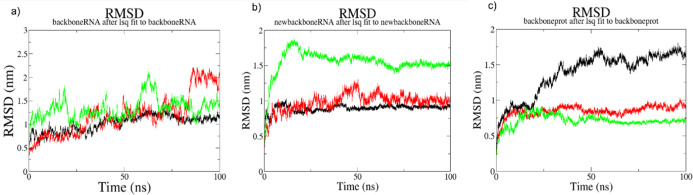
Root-mean-square deviation (RMSD) plots: (a) RMSD vs time plot of backbone atoms of only M-region (in black color) vs E-region (in red color) vs full-length region motifs (in green color) of MALAT1, present in a free form; (b) RMSD vs time plot of backbone atoms of M-region bound to RNPS1 (in black color) vs bound E-region (in red color) vs bound full-length region motifs (in green color), present in the docked complexes; (c) RMSD vs time plot of backbone atoms of RNPS1 bound to M-region (in black color) vs bound to E-region (in red color) vs bound to full-length region motifs (in green color). Superimposition of conformations at different time points was performed relative to the energy-minimized, equilibrated structure.
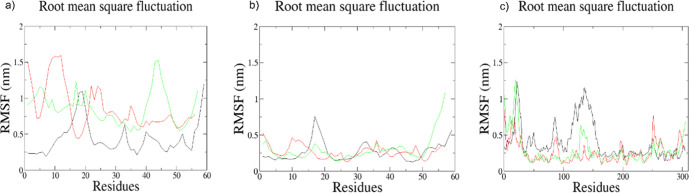
Root-mean-square fluctuation (RMSF) plots: (a) RMSF plot of only M-region (in black color) vs E-region (in red color) vs full-length region motifs (in green color) of MALAT1, present in a free form; (b) RMSF plot of bound MALAT1 M-region (in black color) vs bound E-region (in red color) vs bound full-length region motifs (in green color), present in the docked complexes; and (c) RMSF plot of RNPS1 bound to M-region (in black color) vs bound to E-region (in red color) vs bound to full-length region motifs (in green color).
To understand the degree of local fluctuations in the atomic positions in the trajectories of the bound RNA–protein complex, we calculated the root-mean-square fluctuations (RMSF) of these structures. As expected, fluctuations in the free form of MALAT1 regions are the highest (Figure Figure88a) which get lowered when in the bound form, where the M-region motif shows the highest fluctuation (Figure Figure88b). The fluctuations in the RNPS1 protein molecule in the M-region–RNPS1 docked complex are larger than those of the other two docked complexes, as is observed from the RMSF plot (Figure Figure88c). Notably, the largest increases in flexibility occur around residues 130–145 (which are present within highly fluctuating residues 100–160) in both the M-region–RNPS1 and full-length-RNPS1 complexes. There is also a high degree of local fluctuations in the initial parts of RNPS1 in both these complexes. The E-region–RNPS1 bound complex shows decreased flexibility among all, implicating the E-region to be far more ordered when bound by RNPS1. We further compared the global motions and compactness of our systems using radius of gyration (Rg) of the free form of MALAT1 (Figure Figure99a) and bound form of MALAT1 (Figure Figure99b) and RNPS1 protein (Figure Figure99c). Rg of the free form of MALAT1 appears compact across the trajectories with the E-region motif loosening a little. Rg of bound MALAT1 across these complexes showed compact conformations except for a little displacement, again in the E-region, across the trajectories. Rg of RNPS1 across the complexes was found to be ranging between 2.25 and 2.55 nm, demonstrating the compactness and the stability of the protein in the docked complexes during the entire simulation time. It is worthwhile to mention that in the E-region-bound RNPS1, the protein appears more compact than the RNA throughout the time points. Taken together, these observations show the M-region of MALAT1 to be more interactive and proactive toward complexation with RNPS1, despite its higher degree of flexibility due to the presence of an unfolded/partially folded region at one end in its tertiary structure. As the hydrogen bond interaction stabilizes the complex, hydrogen bonding plots show all three systems demonstrating a stable complex over the course of the trajectories (Figure Figure99d).
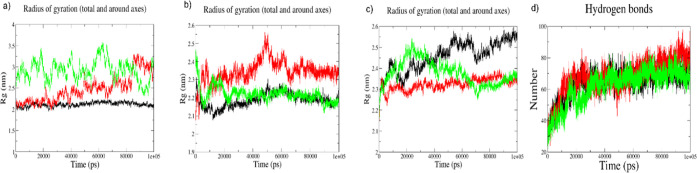
Radius of gyration (Rg) and global hydrogen bond plots: (a) for free-form MALAT1 regions, M-region is depicted in black color, E-region in red color, and full-length region motifs is shown in green color; (b) for bound MALAT1 regions, M-region is depicted in black color, E-region in red color, and full-length region motifs is shown in green color; (c) for RNPS1 bound to MALAT1 regions over time, for RNPS1 bound to M-region (in black color), to E-region (in red color), and to full-length region motifs (in green color); and (d) global hydrogen bond plots for RNPS1 bound to M-region (in black color) vs bound to E-region (in red color) vs bound to full-length region motifs (in green color).
Relative Binding Free Energies Using Generalized Born and Poisson–Boltzmann Models Reveal that Simulations Recapitulate the Preferential Binding of MALAT1 Regions In Vitro
Calculation of binding free energies was performed by the gmx_MMPBSA64 version with GROMACS files. Strikingly, binding energy calculations revealed the same ranking of M-region, followed by E-region and then full-length structure of MALAT1, toward the binding affinities, as is observed from our docking studies as well as previously published experiments35 as noted above. The average deltaG binding ± SEM from generalized Born (GB)-based calculations was found to be −361.07 ± 2.69 kcal/mol for bound M-region, −335.37 ± 2.79 kcal/mol for bound E-region, and −297.77 ± 3.04 kcal/mol for bound full-length region of MALAT1, respectively (Table 1). We also performed Poisson–Boltzmann (PB)-based calculations using the same parameter values as GB-based calculations, and the results agreed with GB-based calculations in the ranking of the bound regions. The average deltaG binding ± SEM from PB-based calculations were found to be −749.16 ± 3.58 kcal/mol for the bound M-region, −736.70 ± 2.62 kcal/mol for the bound E-region, and −673.21 ± 3.44 kcal/mol for the bound full-length region of MALAT1, respectively. These negative binding energies also show that these complexes are favorable under the solvation conditions. Notably, and as expected, major favorable contributions to this binding energy come from electrostatic contributions, ΔEEL, as observed from Table 1. As is also expected, electrostatic contributions to the solvation free energy, ΔEGB, are unfavorable across these complexes because this is the energy used to desolvate the particles present in the binding interface.
Table 1
Energy component | Average | SD (Prop.) | SD | SEM (Prop.) | SEM |
---|---|---|---|---|---|
M-region: In kcal/mol | |||||
Delta (Complex–Receptor–Ligand) | |||||
ΔBOND | 0.00 | 5.80 | 0.00 | 1.83 | 0.00 |
ΔANGLE | –0.00 | 10.07 | 0.00 | 3.18 | 0.00 |
ΔDIHED | –0.00 | 8.41 | 0.00 | 2.66 | 0.00 |
ΔVDWAALS | –256.60 | 9.53 | 8.24 | 3.01 | 2.61 |
ΔEEL | –3806.75 | 7.79 | 64.76 | 2.46 | 20.48 |
Δ1–4 VDW | –0.00 | 5.44 | 0.00 | 1.72 | 0.00 |
Δ1–4 EEL | –0.00 | 2.01 | 0.00 | 0.63 | 0.00 |
ΔEGB | 3739.38 | 7.73 | 62.08 | 2.44 | 19.63 |
ΔESURF | –37.10 | 0.31 | 0.59 | 0.10 | 0.19 |
ΔGGAS | –4063.35 | 12.31 | 65.85 | 3.89 | 20.82 |
ΔGSOLV | 3702.28 | 7.73 | 61.66 | 2.45 | 19.50 |
ΔTOTAL | –361.07 | 14.53 | 8.50 | 4.60 | 2.69 |
E-region: In kcal/mol | |||||
Delta (Complex–Receptor–Ligand) | |||||
ΔBOND | 0.00 | 12.49 | 0.00 | 3.59 | 0.00 |
ΔANGLE | 0.00 | 17.36 | 0.00 | 5.49 | 0.00 |
ΔDIHED | 0.00 | 7.65 | 0.00 | 2.42 | 0.00 |
ΔVDWAALS | –221.72 | 12.71 | 9.35 | 4.02 | 2.96 |
ΔEEL | –3979.21 | 9.23 | 24.96 | 2.92 | 7.89 |
Δ1–4 VDW | –0.00 | 7.33 | 0.00 | 2.32 | 0.00 |
Δ1–4 EEL | –0.00 | 1.20 | 0.00 | 0.38 | 0.00 |
ΔEGB | 3897.81 | 8.79 | 23.45 | 2.78 | 7.41 |
ΔESURF | –32.25 | 0.15 | 1.07 | 0.05 | 0.34 |
ΔGGAS | –4200.93 | 15.71 | 25.63 | 4.97 | 8.10 |
ΔGSOLV | 3865.56 | 8.79 | 23.07 | 2.78 | 7.30 |
ΔTOTAL | –335.37 | 18.00 | 8.84 | 5.69 | 2.79 |
Full-length: In kcal/mol | |||||
Delta (Complex–Receptor–Ligand) | |||||
ΔBOND | –0.00 | 5.94 | 0.00 | 1.88 | 0.00 |
ΔANGLE | –0.00 | 22.15 | 0.00 | 7.00 | 0.00 |
ΔDIHED | –0.00 | 4.73 | 0.00 | 1.50 | 0.00 |
ΔVDWAALS | –198.30 | 4.01 | 7.92 | 1.27 | 2.50 |
ΔEEL | –3610.34 | 12.83 | 36.94 | 4.06 | 11.68 |
Δ1–4 VDW | –0.00 | 6.88 | 0.00 | 2.18 | 0.00 |
Δ1–4 EEL | 0.00 | 1.62 | 0.00 | 0.51 | 0.00 |
ΔEGB | 3540.47 | 14.44 | 34.96 | 4.56 | 11.05 |
ΔESURF | –29.60 | 0.40 | 0.69 | 0.13 | 0.22 |
ΔGGAS | –3808.64 | 13.44 | 42.20 | 4.25 | 13.35 |
ΔGSOLV | 3510.87 | 14.44 | 34.38 | 4.57 | 10.87 |
ΔTOTAL | –297.77 | 19.73 | 9.60 | 6.24 | 3.04 |
Residue Contributions Using Energy Decomposition
To identify the major residue contributors to the BFE calculated by the MM-GBSA method, total decomposition contribution calculations using generalized Born decomposition energies for residues within 4 Å in both the receptor and the ligand showed that in the M-region-bound RNPS1, Arg273, Lys7, Lys8 (all these three in hydrogen-bonding interactions), and Arg271 in nonbonded contacts were among the topmost major ranked contributors (Figure Figure1010a). In the E-region-bound RNPS1 (Figure Figure1010b), Arg286 in nonbonded contacts contributed most to the binding energy, followed by Arg288 (in hydrogen-bonding interaction) and Arg284 (nonbonded contacts), while in the full-length MALAT1 motif-bound RNPS1, Arg273, Arg265, and Trp263, all these three residues in hydrogen-bonding interactions ranked high (Figure Figure1010c).
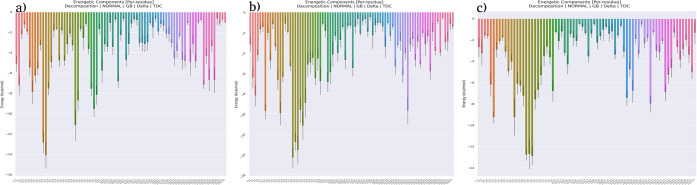
Per-residue energy decomposition plot in decomposition calculations for (a) E-region–RNPS1 complex; (b) M-region–RNPS1 complex; and (c) full-length–RNPS1 complex.
Among the nucleotides, G25, A24, and A40 nucleotides, through the interactions of their base-sugar–phosphate, sugar–phosphate, and base-phosphate moieties, respectively, in the E-region (Figure Figure1010a and Figure S1) and A50 and A48 of the M-region nucleotides, through the interactions of their base-phosphate and sugar–phosphate moieties, respectively, displayed the highest negative values toward energy contributions (Figures Figures1010b and S2). G48, G49, and A47 nucleotides in full-length MALAT1, through the interactions of their base-sugar–phosphate, sugar–phosphate, and sugar–phosphate moieties, respectively (Figures Figures1010c and S3), were the ones with the highest negative total energy values. Interestingly, all of these RNA residues with higher energy contributions are purines and are present in the internal or terminal loops, rather than that in stems, in their respective secondary structures. This also revealed that purines in MALAT1 regions interacted with RNPS1 more strongly than pyrimidines, and hence, may contribute more toward the binding free energies.
Discussion
LncRNA interactions with macromolecules such as DNA, RNA, and proteins are the driving forces in the regulation of several cellular and molecular processes, in health and in diseases.10 Messenger RNA stability, splicing, and degradation processes have been found to be regulated by lncRNA molecules, while several RBPs are bound by lncRNAs to regulate the activity of these proteins, and vice versa. Even poly(A) tail length control of bulk RNA can be accomplished in vivo by an RBP, ZC3H14,66 which is evolutionarily conserved, is observed to be localized to nuclear speckles and undergoes alternative splicing. MALAT1, a highly conserved long noncoding RNA, is a dynamic biomolecule capable of undergoing compositional and conformational changes, while playing multi-faceted roles. While several biochemical and biophysical studies on MALAT1 have been reported till date, with a 3D structure of its 3′ ENE region generated using X-ray crystallization at 3.1 Å resolution,40 presence of a well-defined full-length tertiary structure is lacking, and speculations are ongoing whether globally, MALAT1 possesses a compact, disordered, or an extended form.67 Given their large nucleotide length (MALAT1 has ~8.3k nt-long sequence), presence of multiple transcripts of the same gene, and their dynamic binding with proteins and other macromolecules, lncRNAs are difficult to be analyzed via high-resolution methods of structural determination. However, the secondary structure elucidation of a few of the known lncRNAs, including group I68 and group II introns69 as well as HOTAIR,21 has shown that these indeed contain a modular structure. Cryo-EM structures of few of the known RNA–protein complexes including spliceosomes70 and ribosomes71 have also been obtained. What remains to be fully understood is whether or not some or all of the lncRNAs exist as well-defined modular structures and, if they indeed have, how exactly do they interact and carry out their dynamic binding with proteins, their potential binding partner biomolecules. Our MD simulation results lay a step in this direction by providing structural insights into the ubiquitously expressed MALAT1 lncRNA and its interaction with an RBP, RNPS1, which has been observed experimentally.35 Our study has corroborated this interaction between MALAT1 and RNPS1, on a structural basis, besides furnishing key novel insights on the mechanism of alternative splicing in cancers.
Given the weak sequence conservation of lncRNAs, identifying homologues is challenging, especially when compared to that of the protein-coding genes. Through our novel computational approach, we report that the structural motifs of MALAT1 with no known homologues may be capable of forming both the secondary and tertiary structures. As these are observed to be flexible in nature, the structures obtained can adopt various conformations. This observation is not surprising as a study showed the structural conformational flexibility of HIV-1 RNA (47-nucleotides dimer) through both cryo-EM and MD simulations.72 The dynamic conformations of lncRNAs might confer multiple biological relevance to these molecules.
The secondary structures of both the motif regions, namely, E- and M- regions of MALAT1 appear as distinct structures with no sequence homology. These regions, further modeled as 3D structures, adopt a specific conformation and are observed to bind to the RS/P domain of RNPS1 with high affinities, albeit differing in their strength of binding. This binding categorization is in sync with the in vitro binding of fragments of MALAT1 with RNPS1,35 and its effect on the localization of MALAT1 has also been observed. Our MD simulation results reveal that RNPS1 binds to the M-region motif with the highest binding affinity as revealed by MM-GBSA binding energy calculations, followed by the E-region and then the full-length region motifs. Even though the data do not achieve full quantitative convergence because of the computationally demanding task due to a large complex system with many degrees of freedom such as this, MD simulations are capable of providing a wealth of information pertaining to RNA-protein complex structural dynamics.73
In one study, simulations of the partially folded MALAT1(45) observed a large increase in RMSD, with an initial increase between 15 and 30 Å (corresponding to 1.5 and 3 nm, respectively). The core folded form had an RMSD of 5 Å (corresponding to 0.5 nm). Our simulation studies found MALAT1 regions in the bound complex showing RMSD values between 0.75 and 1.5 nm, which remain stable throughout the trajectory period. This shows that the modeled structures are in a properly folded form in the complex, consistent with that of the core ENE triple helix of MALAT1.45 Mapping of the binding free energies across multiple complexes in our study is consistent with the docking energies from our studies as well as previously published experimental observations, as noted above. In particular, arginines are the largest contributors to this free energy of binding across complexes, as is usually expected.
In the case of the higher BFE contributors from MALAT1, purines (G and A) were observed across all of these complexes studied (Figure Figure1010). Another common theme running through all of these complexes is that these purines are almost always found in internal or terminal loops in their secondary structures (Figure Figure22), with some purines forming non-Watson–Crick base pairs. This propensity of purines to bind more efficiently is observed in several examples, such as in the binding modes of serine/arginine (SR) proteins, which are known to possess RRM. Interacting through its ΨRRM, the specific GGA region of 5′-UGAAGGAC-3′ RNA was observed to be bound by SRSF1 using isothermal titration calorimetry experiments and regulates alternative splicing of target transcripts.74 In a most recent study,63 RS region of SRSF1, apart from RRM region, was also observed to bind to G-quadruplex from ARPC2 mRNA, preferring purines over pyrimidines. It is also widely observed that the purine-rich sequences in RNAs are highly conserved, and single-stranded regions such as the terminal, internal, and junction loops are the major sites harboring these.75 SRA lncRNA, riboswitches, and eukaryotic ribosomes harbor a number of purine-rich single-stranded regions.67 Purine bases are also commonly observed to occur in the triplex structures as in the crystal structure of the SAM-II riboswitch aptamer domain.76 In one study, Drosophila RBP UNR was observed to interact most strongly with the purine-rich region of the lncRNA roX2 fragment (nucleotides 316–379), located within the stem–loop 6 secondary structure, using electrophoretic mobility shift experiments.77 Arginine was observed to interact with either the O6 or N7 atoms, but not with both simultaneously, on guanine, in a single-bond interaction type.78 Ours and these other observations indicate that there must be some general principles in the amino-acid–base contacts across all the lncRNA–protein complexes, similar to those observed in protein–DNA complexes.78 Loops appear to be the most commonly involved secondary structure regions compared to stems/helices, preferably due to their increased accessibility on a structural basis. Why are the purines more involved than pyrimidines in such contacts is a question yet to be answered completely in the context of lncRNA molecules, and it would require a larger data set to arrive at a robust conclusion. Given that ENCODE and FANTOM consortia have generated huge amounts of sequence and annotation data, a reproducible answer to this question is not far away.
The fact that MALAT1 may bind to RS/P region of RNPS1 involved in binding to hTra2β, an exonic splicing enhancer-binding protein, or may be involved in exon skipping, leads us to deduce a potential mechanism. Preferential MALAT1 binding may disrupt the protein-protein interaction of this RS/P region with hTRA2β, leading to an aberrant splicing. Overall, our near-accurate computational approach can be extended to the other lncRNA molecules, as well. We have shown that despite the longer lengths of these lncRNAs creating a challenge, their 3D structures can be determined by utilizing this unique approach encompassing the binding motifs. Furthermore, multinanosecond-long all-atom MD simulations can provide unprecedented insights into the lncRNA–protein interactions and binding energies and dispense a noteworthy piece of information for a thorough mechanistic understanding of such interactions in the regulation of gene expression.
Materials and Methods
Preparation of Molecular Structures
3D Motifs of MALAT1
The RefSeq transcript of MALAT1 was retrieved from NCBI with accession ID NR_002819.4. This long sequence was split into multiple regions,35 E-region (1961–3040 nucleotides) and M-region (6008–7011 nucleotides) of MALAT1. Structural modeling, especially that of tertiary structures, using the whole sequence length, was unfeasible as none of the in silico tools known could model the tertiary structures with more than 500-nucleotide length. We therefore took a unique approach by taking into account motifs, shorter sequences that comprise a potential protein-binding region. We employed RBPmap database (https://rbpmap.technion.ac.il/), a web server that enables accurate mapping and prediction of the binding motifs of RBP on a query RNA sequence.46 A weighted-rank score is calculated around each putative binding site. This is carried out to reflect the propensity of suboptimal motifs to cluster around significant motif.
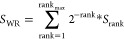
Where, according to the developers, “rankmax is the number of suboptimal sites within the 50 nts window and Srank is the match score of each ranked suboptimal site”. Thereafter, Z-scores are calculated coupled to a p-value, and the putative binding sites are reported when p-value < 0.05.
The E-region, M-region, and full-length MALAT1 sequences from the RefSeq transcript were provided in a FASTA format, and the human genome was considered for the motif search. With ~150 motifs obtained as the output, the motif having the highest p-value (p-value < 0.05) was considered. This narrowed down our search to the RBM24 motif for the M-region, UNK motif for the E-region, and RBM5 motif for full-length MALAT1. We modeled the secondary structure of these motifs of E-region, M-region, and full-length MALAT1 through RNAfold (http://rna.tbi.univie.ac.at/cgi-bin/RNAWebSuite/RNAfold.cgi), a powerful computational tool that predicts secondary structures based on the minimum free-energy conformation.47 It uses an algorithm based on loop-based energy models and dynamic programming in creating optimal subproblems, to generate MFE structures using stacking and destabilizing energies, created by Zuker and Steigler, 1981.48 The free energy F(s) of an RNA secondary structure is treated as the sum of the contributing free energies FL of loops L contained in its secondary structure. The dot-bracket notation outputs of these secondary structures were taken for tertiary structure modeling via a fully automated 3D structure prediction tool, RNAComposer version 1.0 (https://rnacomposer.cs.put.poznan.pl/). RNAComposer uses the RNA FRABASE database of RNA secondary structures and elements of tertiary structure, such as “residues, base pairs, multiplets, dinucleotide steps, stems, and single-stranded regions of loops (apical, bulge, internal, and n-way junctions)”.50 3D elements/fragments of a given sequence as an input are computed as per structural similarity in RNA FRABASE and then joined by loop modeling, followed by further refinement, to predict a 3D structure of sequences less than 500 nucleotides. This was followed by energy minimization using UCSF Chimera version 1.17.3. MolProbity version 4.5.2 (http://molprobity.biochem.duke.edu/), a tool, was used for the RNA structure validation.51 The 3D structure was analyzed using DSSR tool (http://web.x3dna.org/)52 to identify Watson-Crick and non-Watson–Crick base pairs and structure classification.
RNPS1 Structure Modeling
The sequence of human RNPS1, a 305 amino acids long protein, was retrieved from NCBI with accession ID NP_006702 and was modeled using I-TASSER web server version 5.1(https://zhanggroup.org/I-TASSER/)53 as no full-length structure exists as yet in the PDB. The protein was modeled on a eukaryotic ASAP core complex (PDB ID 4A8X, A chain) as a template with highest percent sequence identity of 99% with 88 amino acids long RNPS1 present in this complex, and was energy minimized in UCSF Chimera.79 The Ramachandran plot obtained in PROCHECK version 3.5.4 (https://saves.mbi.ucla.edu/)80 showed 2.3% residues in disallowed regions (with 72.1% residues in the most favored region). AlphaFold2-modeled structure of the human RNPS1 (UniProt ID Q15287) showing 100% sequence identity with NCBI sequence NP_006702 was also retrieved from AlphaFold2 database (https://alphafold.ebi.ac.uk/).56
RNA–Protein Molecular Docking
To obtain crucial structural insights into the complex formation, MALAT1-RNPS1 docking was performed using rigid body docking protocol of ClusPro version 2.0 (https://cluspro.bu.edu/login.php)57 considering the “balanced” model with 70,000 rotations. Besides protein–protein docking, ClusPro2 has also been developed to accept RNA as a receptor, with all the supported RNA residues and atomic data available (https://cluspro.org/rna.php). The RNA molecule (MALAT1) was treated as a receptor, and RNPS1 protein was treated as a ligand. ClusPro2 adds polar hydrogens before docking. The biggest cluster size representative, which is the topmost hit as well as with the lowest energy, is used as a starting complex for MD studies. We also corroborated this docking with another docking tool, PatchDock (https://bioinfo3d.cs.tau.ac.il/PatchDock/) with default parameters.58
PDBsum Generate (https://www.ebi.ac.uk/thornton-srv/databases/pdbsum/), a program that generates schematic representations of nucleic acids (DNA/RNA)–protein complex interactions as a nucplot, was considered for the structural interaction analysis.81
MD Simulation and Contact Analyses
MD simulations were performed on six systems, including three individual RNA systems, namely, motifs of M-region, E-region, and full-length MALAT1, and their respective docked complexes with RNPS1. GROMACS 2021 with amber99SB force field, the current state-of-the art force field,82 was used. Each structure was solvated in an octahedral water box with the TIP3P water model83 with a cutoff distance of 10 Å from the wall of the box. Sodium and chloride ions were added to the system and in order to constrain the covalent bonds involving hydrogen atoms, the LINCS algorithm was used.84 The long-range electrostatic interactions were calculated using particle mesh Ewald summation algorithm.85 For the nonbonded interactions, a cutoff of 10 Å was set. Each system was minimized for 50,000 steps using a steepest descent algorithm. After the minimization, each system was equilibrated in two phases, at 300 K using a V-rescale thermostat and 1 atm pressure using a Parinello–Rahman barostat. Finally, the nonconstrained production run of each system was conducted for 100 ns with an integration step of 2 fs. Combining all of the replicates of the six systems, trajectories of a grand total of 600 ns MD simulations were obtained. The trajectory analyses including the RMSD, clustering, RMSF, and radius of gyration (Rg) were calculated using GROMACS analysis tools, XMGRACE plotting tool https://plasma-gate.weizmann.ac.il/Grace/,86 and VMD version 1.9.3 (https://www.ks.uiuc.edu/Research/vmd/).87 The hydrogen bond (H-bond) occupancy was calculated using the GROMACS module gmx hbond.
BFE and Decomposition Analyses
BFE and decomposition analyses were carried out using gmx_MMPBSA version 1.6.3,64 which incorporates mmpbsa.py version 16 of Amber biomolecular simulations package.65 Default parameters were used, with igb8 for the GB-Neck2 model and a salt concentration set to 0.15 M. Also, a high dielectric constant of 10 was specified because of the presence of a high number of charged residues at the protein–RNA interface. PB-based calculations using the same parameter values as the GB-based calculations were also performed. BFE, DeltaGbind, is calculated as per the following equation

where Gcomplex, GRNA, and Gprotein are the free energies of complex, RNA, and protein, respectively. Implicit solvation is used, and the total solvation free energy of a molecule is a combination of electrostatics and nonelectrostatic components

For per-residue decomposition analysis, residues within 4 Å in both the receptor and ligand were taken into account. From the decomposition calculation output files, delta values were taken into account for the analysis. Plots were plotted using the gmx_MMPBSA_ana tool. Positive energy values were removed while plotting. The images were rendered through PyMol88 and Chimera visualization tools.
Acknowledgments
This work was supported by the Institution of Eminence IoE grant number UoH-IoE-RC3-21-062, which is now a finished grant. The authors gratefully acknowledge this grant and also the usage of CMSD HPC facilities at the University of Hyderabad, India.
Data Availability Statement
All data are taken from the public domain appropriately cited, and results, figures, and tables are presented in this manuscript.
Supporting Information Available
The Supporting Information is available free of charge at https://pubs.acs.org/doi/10.1021/acsomega.4c04467.
E-region–RNPS1 interactions, M-region–RNPS1 interactions, and full-length-region–RNPS1 interactions (PDF)
Notes
The authors declare no competing financial interest.
References
- Wright C. J.; Smith C. W. J.; Jiggins C. D. Alternative splicing as a source of phenotypic diversity. Nat. Rev. Genet. 2022, 23, 697–710. 10.1038/s41576-022-00514-4. [Abstract] [CrossRef] [Google Scholar]
- Borišek J.; Casalino L.; Saltalamacchia A.; Mays S. G.; Malcovati L.; Magistrato A. Atomic-Level Mechanism of Pre-mRNA Splicing in Health and Disease. Acc. Chem. Res. 2021, 54 (1), 144–154. 10.1021/acs.accounts.0c00578. [Abstract] [CrossRef] [Google Scholar]
- David C. J.; Manley J. L. The search for alternative splicing regulators: new approaches offer a path to a splicing code. Genes Dev. 2008, 22 (3), 279–285. 10.1101/gad.1643108. [Europe PMC free article] [Abstract] [CrossRef] [Google Scholar]
- Clark M. B.; Amaral P.; Schlesinger F. J.; Dinger M. E.; Taft R. J.; Rinn J. L.; Ponting C. P.; Stadler P. F.; Morris K. V.; Morillon A.; et al. The reality of pervasive transcription. PLoS Biol. 2011, 9 (7), e100062510.1371/journal.pbio.1000625. [Europe PMC free article] [Abstract] [CrossRef] [Google Scholar]
- Brown S. D. XIST and the mapping of the X chromosome inactivation centre. Bioessays 1991, 13 (11), 607–612. 10.1002/bies.950131112. [Abstract] [CrossRef] [Google Scholar]
- Brown C. J.; Hendrich B. D.; Rupert J. L.; Lafrenière R. G.; Xing Y.; Lawrence J.; Willard H. F. The human XIST gene: analysis of a 17 kb inactive X-specific RNA that contains conserved repeats and is highly localized within the nucleus. Cell 1992, 71, 527–542. 10.1016/0092-8674(92)90520-M. [Abstract] [CrossRef] [Google Scholar]
- Brannan C. I.; Dees E. C.; Ingram R. S.; Tilghman S. M. The product of the H19 gene may function as an RNA. Mol. Cell. Biol. 1990, 10 (1), 28–36. 10.1128/mcb.10.1.28. [Europe PMC free article] [Abstract] [CrossRef] [Google Scholar]
- Wutz A.; Smrzka O. W.; Schweifer N.; Schellander K.; Wagner E. F.; Barlow D. P. Imprinted expression of the Igf2r gene depends on an intronic CpG island. Nature 1997, 389 (6652), 745–749. 10.1038/39631. [Abstract] [CrossRef] [Google Scholar]
- Lakhotia S. C. Forty years of the 93D puff of Drosophila melanogaster. J. Biosci. 2011, 36 (3), 399–423. 10.1007/s12038-011-9078-1. [Abstract] [CrossRef] [Google Scholar]
- Statello L.; Guo C. J.; Chen L. L.; Huarte M. Gene regulation by long non-coding RNAs and its biological functions. Nat. Rev. Mol. Cell Biol. 2021, 22 (2), 96–118. 10.1038/s41580-020-00315-9. [Europe PMC free article] [Abstract] [CrossRef] [Google Scholar]
- Wang X.; Arai S.; Song X.; Reichart D.; Du K.; Pascual G.; Tempst P.; Rosenfeld M. G.; Glass C. K.; Kurokawa R. Induced ncRNAs allosterically modify RNA-binding proteins in cis to inhibit transcription. Nature 2008, 454 (7200), 126–130. 10.1038/nature06992. [Europe PMC free article] [Abstract] [CrossRef] [Google Scholar]
- Monfort A.; Minin D. G.; Postlmayr A.; et al. Identification of Spen as a Crucial Factor for Xist Function through Forward Genetic Screening in Haploid Embryonic Stem Cells. Cell Rep. 2015, 12 (4), 554–561. 10.1016/j.celrep.2015.06.067. [Europe PMC free article] [Abstract] [CrossRef] [Google Scholar]
- Jachowicz J. W.; Strehle M.; Banerjee A. K.; Blanco M. R.; Thai J.; Guttman M. Xist spatially amplifies SHARP/SPEN recruitment to balance chromosome-wide silencing and specificity to the X chromosome. Nat. Struct. Mol. Biol. 2022, 29 (3), 239–249. 10.1038/s41594-022-00739-1. [Europe PMC free article] [Abstract] [CrossRef] [Google Scholar]
- Cesana M.; Cacchiarelli D.; Legnini I.; Santini T.; Sthandier O.; Chinappi M.; Tramontano A.; Bozzoni I. A long noncoding RNA controls muscle differentiation by functioning as a competing endogenous RNA. Cell 2011, 147 (2), 358–369. 10.1016/j.cell.2011.09.028. [Europe PMC free article] [Abstract] [CrossRef] [Google Scholar]
- Kim D. N.; Thiel B.; Mrozowich T.; Hennelly S. P.; Hofacker I. L.; Patel T. R.; Sanbonmatsu K. Y. Zinc-finger protein CNBP alters the 3-D structure of lncRNA Braveheart in solution. Nat. Commun. 2020, 11 (1), 148.10.1038/s41467-019-13942-4. [Europe PMC free article] [Abstract] [CrossRef] [Google Scholar]
- Kumar S.; Mishra S. MALAT1 as master regulator of biomarkers predictive of pan-cancer multi-drug resistance in the context of recalcitrant NRAS signaling pathway identified using systems-oriented approach. Sci. Rep. 2022, 12 (1), 7540.10.1038/s41598-022-11214-8. [Europe PMC free article] [Abstract] [CrossRef] [Google Scholar]
- Saleembhasha A.; Mishra S. Long non-coding RNAs as pan-cancer master gene regulators of associated protein-coding genes: a systems biology approach. PeerJ 2019, 7, e638810.7717/peerj.6388. [Europe PMC free article] [Abstract] [CrossRef] [Google Scholar]
- Bradley R. K.; Anczuków O. RNA splicing dysregulation and the hallmarks of cancer. Nat. Rev. Cancer 2023, 23 (3), 135–155. 10.1038/s41568-022-00541-7. [Europe PMC free article] [Abstract] [CrossRef] [Google Scholar]
- Novikova I. V.; Hennelly S. P.; Sanbonmatsu K. Y. Structural architecture of the human long non-coding RNA, steroid receptor RNA activator. Nucleic Acids Res. 2012, 40 (11), 5034–5051. 10.1093/nar/gks071. [Europe PMC free article] [Abstract] [CrossRef] [Google Scholar]
- Hawkes E. J.; Hennelly S. P.; Novikova I. V.; Irwin J. A.; Dean C.; Sanbonmatsu K. Y. COOLAIR Antisense RNAs Form Evolutionarily Conserved Elaborate Secondary Structures. Cell Rep. 2016, 16 (12), 3087–3096. 10.1016/j.celrep.2016.08.045. [Europe PMC free article] [Abstract] [CrossRef] [Google Scholar]
- Somarowthu S.; Legiewicz M.; Chillón I.; et al. HOTAIR forms an intricate and modular secondary structure. Mol. Cell 2015, 58 (2), 353–361. 10.1016/j.molcel.2015.03.006. [Europe PMC free article] [Abstract] [CrossRef] [Google Scholar]
- Wilusz J. E.; JnBaptiste C. K.; Lu L. Y.; Kuhn C. D.; Joshua-Tor L.; Sharp P. A. A triple helix stabilizes the 3′ ends of long noncoding RNAs that lack poly (A) tails. Genes Dev. 2012, 26 (21), 2392–2407. 10.1101/gad.204438.112. [Europe PMC free article] [Abstract] [CrossRef] [Google Scholar]
- Brown J. A.; Valenstein M. L.; Yario T. A.; Tycowski K. T.; Steitz J. A. Formation of triple-helical structures by the 3′-end sequences of MALAT1 and MENβ noncoding RNAs. Proc. Natl. Acad. Sci. U.S.A. 2012, 109 (47), 19202–19207. 10.1073/pnas.1217338109. [Europe PMC free article] [Abstract] [CrossRef] [Google Scholar]
- Zhang B.; Mao Y. S.; Diermeier S. D.; Novikova I. V.; Nawrocki E. P.; Jones T. A.; Lazar Z.; Tung C. S.; Luo W.; Eddy S. R.; et al. Identification and Characterization of a Class of MALAT1-like Genomic Loci. Cell Rep. 2017, 19 (8), 1723–1738. 10.1016/j.celrep.2017.05.006. [Europe PMC free article] [Abstract] [CrossRef] [Google Scholar]
- Uroda T.; Anastasakou E.; Rossi A.; Teulon J. M.; Pellequer J. L.; Annibale P.; Pessey O.; Inga A.; Chillón I.; Marcia M. Conserved Pseudoknots in lncRNA MEG3 Are Essential for Stimulation of the p53 Pathway. Mol. Cell 2019, 75 (5), 982–995.e9. 10.1016/j.molcel.2019.07.025. [Europe PMC free article] [Abstract] [CrossRef] [Google Scholar]
- Liu F.; Somarowthu S.; Pyle A. M. Visualizing the secondary and tertiary architectural domains of lncRNA RepA. Nat. Chem. Biol. 2017, 13 (3), 282–289. 10.1038/nchembio.2272. [Europe PMC free article] [Abstract] [CrossRef] [Google Scholar]
- Ji P.; Diederichs S.; Wang W.; Böing S.; Metzger R.; Schneider P. M.; Tidow N.; Brandt B.; Buerger H.; Bulk E.; et al. MALAT-1, a novel noncoding RNA, and thymosin β4 predict metastasis and survival in early-stage non-small cell lung cancer. Oncogene 2003, 22 (39), 8031–8041. 10.1038/sj.onc.1206928. [Abstract] [CrossRef] [Google Scholar]
- Hutchinson J. N.; Ensminger A. W.; Clemson C. M.; Lynch C. R.; Lawrence J. B.; Chess A. A screen for nuclear transcripts identifies two linked noncoding RNAs associated with SC35 splicing domains. BMC Genom. 2007, 8, 39.10.1186/1471-2164-8-39. [Europe PMC free article] [Abstract] [CrossRef] [Google Scholar]
- Brown J. A.; Kinzig C. G.; DeGregorio S. J.; Steitz J. A. Methyltransferase-like protein 16 binds the 3′-terminal triple helix of MALAT1 long noncoding RNA. Proc. Natl. Acad. Sci. U.S.A. 2016, 113 (49), 14013–14018. 10.1073/pnas.1614759113. [Europe PMC free article] [Abstract] [CrossRef] [Google Scholar]
- Tripathi V.; Ellis J. D.; Shen Z.; Song D. Y.; Pan Q.; Watt A. T.; Freier S. M.; Bennett C. F.; Sharma A.; Bubulya P. A.; et al. The nuclear-retained noncoding RNA MALAT1 regulates alternative splicing by modulating SR splicing factor phosphorylation. Mol. Cell 2010, 39 (6), 925–938. 10.1016/j.molcel.2010.08.011. [Europe PMC free article] [Abstract] [CrossRef] [Google Scholar]
- Bernard D.; Prasanth K. V.; Tripathi V.; Colasse S.; Nakamura T.; Xuan Z.; Zhang M. Q.; Sedel F.; Jourdren L.; Coulpier F.; et al. A long nuclear-retained non-coding RNA regulates synaptogenesis by modulating gene expression. EMBO J. 2010, 29 (18), 3082–3093. 10.1038/emboj.2010.199. [Europe PMC free article] [Abstract] [CrossRef] [Google Scholar]
- Wilusz J. E.; Freier S. M.; Spector D. L. 3′ end processing of a long nuclear-retained non-coding RNA yields a tRNA-like cytoplasmic RNA. Cell 2008, 135 (5), 919–932. 10.1016/j.cell.2008.10.012. [Europe PMC free article] [Abstract] [CrossRef] [Google Scholar]
- Schmidt G.; Werner D. Sequence of a complete murine cDNA reflecting an S phase-prevalent transcript encoding a protein with two types of nucleic acid binding motifs. Biochim. Biophys. Acta 1993, 1216 (2), 317–320. 10.1016/0167-4781(93)90164-9. [Abstract] [CrossRef] [Google Scholar]
- Deka B.; Chandra P.; Yadav P.; Rehman A.; Kumari S.; Kunnumakkara A. B.; Singh K. K. RNPS1 functions as an oncogenic splicing factor in cervical cancer cells. IUBMB Life 2023, 75 (6), 514–529. 10.1002/iub.2686. [Abstract] [CrossRef] [Google Scholar]
- Miyagawa R.; Tano K.; Mizuno R.; Nakamura Y.; Ijiri K.; Rakwal R.; Shibato J.; Masuo Y.; Mayeda A.; Hirose T.; et al. Identification of cis- and trans-acting factors involved in the localization of MALAT-1 noncoding RNA to nuclear speckles. RNA 2012, 18 (4), 738–751. 10.1261/rna.028639.111. [Europe PMC free article] [Abstract] [CrossRef] [Google Scholar]
- Larochelle S. RNA-binding proteome redux. Nat. Methods 2019, 16 (3), 219.10.1038/s41592-019-0349-3. [Abstract] [CrossRef] [Google Scholar]
- Marcinkiewicz K. M. AFM in a split second. Nat. Methods 2019, 16, 24.10.1038/s41592-018-0289-3. [Abstract] [CrossRef] [Google Scholar]
- Simon M. D.; Wang C. I.; Kharchenko P. V.; West J. A.; Chapman B. A.; Alekseyenko A. A.; Borowsky M. L.; Kuroda M. I.; Kingston R. E. The genomic binding sites of a noncoding RNA. Proc. Natl. Acad. Sci. U.S.A. 2011, 108 (51), 20497–20502. 10.1073/pnas.1113536108. [Europe PMC free article] [Abstract] [CrossRef] [Google Scholar]
- Ule J.; Jensen K. B.; Ruggiu M.; Mele A.; Ule A.; Darnell R. B. CLIP identifies Nova-regulated RNA networks in the brain. Science 2003, 302 (5648), 1212–1215. 10.1126/science.1090095. [Abstract] [CrossRef] [Google Scholar]
- Brown J. A.; Bulkley D.; Wang J.; Valenstein M. L.; Yario T. A.; Steitz T. A.; Steitz J. A. Structural insights into the stabilization of MALAT1 noncoding RNA by a bipartite triple helix. Nat. Struct. Mol. Biol. 2014, 21 (7), 633–640. 10.1038/nsmb.2844. [Europe PMC free article] [Abstract] [CrossRef] [Google Scholar]
- Bhattacharjee R.; Devi A.; Mishra S. Molecular docking and molecular dynamics studies reveal structural basis of inhibition and selectivity of inhibitors EGCG and OSU-03012 toward glucose regulated protein-78 (GRP78) overexpressed in glioblastoma. J. Mol. Model. 2015, 21, 272.10.1007/s00894-015-2801-3. [Abstract] [CrossRef] [Google Scholar]
- Gurusinghe K. R. D. S. N. S.; Mishra A.; Mishra S. Glucose-regulated protein 78 substrate-binding domain alters its conformation upon EGCG inhibitor binding to nucleotide-binding domain: Molecular dynamics studies. Sci. Rep. 2018, 8 (1), 5487.10.1038/s41598-018-22905-6. [Europe PMC free article] [Abstract] [CrossRef] [Google Scholar]
- Saltalamacchia A.; Casalino L.; Borišek J.; Batista V. S.; Rivalta I.; Magistrato A. Decrypting the Information Exchange Pathways across the Spliceosome Machinery. J. Am. Chem. Soc. 2020, 142 (18), 8403–8411. 10.1021/jacs.0c02036. [Europe PMC free article] [Abstract] [CrossRef] [Google Scholar]
- Casalino L.; Palermo G.; Spinello A.; Rothlisberger U.; Magistrato A. All-atom simulations disentangle the functional dynamics underlying gene maturation in the intron lariat spliceosome. Proc. Natl. Acad. Sci. U.S.A. 2018, 115 (26), 6584–6589. 10.1073/pnas.1802963115. [Europe PMC free article] [Abstract] [CrossRef] [Google Scholar]
- Yonkunas M.; Baird N. J. A highly ordered, nonprotective MALAT1 ENE structure is adopted prior to triplex formation. RNA 2019, 25 (8), 975–984. 10.1261/rna.069906.118. [Europe PMC free article] [Abstract] [CrossRef] [Google Scholar]
- Paz I.; Kosti I.; Ares M. Jr.; Cline M.; Mandel-Gutfreund Y. RBPmap: a web server for mapping binding sites of RNA-binding proteins. Nucleic Acids Res. 2014, 42 (W1), W361–W367. 10.1093/nar/gku406. [Europe PMC free article] [Abstract] [CrossRef] [Google Scholar]
- Gruber A. R.; Lorenz R.; Bernhart S. H.; Neubock R.; Hofacker I. L. The Vienna RNA websuite. Nucleic Acids Res. 2008, 36 (Web Server), W70–W74. 10.1093/nar/gkn188. [Europe PMC free article] [Abstract] [CrossRef] [Google Scholar]
- Zuker M.; Stiegler P. Optimal computer folding of large RNA sequences using thermodynamics and auxiliary information. Nucleic Acids Res. 1981, 9 (1), 133–148. 10.1093/nar/9.1.133. [Europe PMC free article] [Abstract] [CrossRef] [Google Scholar]
- Mathews D. H.; Disney M. D.; Childs J. L.; Schroeder S. J.; Zuker M.; Turner D. H. Incorporating chemical modification constraints into a dynamic programming algorithm for prediction of RNA secondary structure. Proc. Natl. Acad. Sci. U.S.A. 2004, 101 (19), 7287–7292. 10.1073/pnas.0401799101. [Europe PMC free article] [Abstract] [CrossRef] [Google Scholar]
- Purzycka K. J.; Popenda M.; Szachniuk M.; Antczak M.; Lukasiak P.; Blazewicz J.; Adamiak R. Automated 3D RNA structure prediction using the RNAComposer method for riboswitches. Methods Enzymol. 2015, 553, 3–34. 10.1016/bs.mie.2014.10.050. [Abstract] [CrossRef] [Google Scholar]
- Williams C. J.; Headd J. J.; Moriarty N. W.; Prisant M. G.; Videau L. L.; Deis L. N.; Verma V.; Keedy D. A.; Hintze B. J.; Chen V. B.; et al. MolProbity: More and better reference data for improved all-atom structure validation. Protein Sci. 2018, 27 (1), 293–315. 10.1002/pro.3330. [Europe PMC free article] [Abstract] [CrossRef] [Google Scholar]
- Li S.; Olson W. K.; Lu X.-J. Web 3DNA 2.0 for the analysis, visualization, and modeling of 3D nucleic acid structures. Nucleic Acids Res. 2019, 47 (W1), W26–W34. 10.1093/nar/gkz394. [Europe PMC free article] [Abstract] [CrossRef] [Google Scholar]
- Yang J.; Zhang Y. I-TASSER server: new development for protein structure and function predictions. Nucleic Acids Res. 2015, 43 (W1), W174–W181. 10.1093/nar/gkv342. [Europe PMC free article] [Abstract] [CrossRef] [Google Scholar]
- Schwerk C.; Prasad J.; Degenhardt K.; Erdjument-Bromage H.; White E.; Tempst P.; Kidd V. J.; Manley J. L.; Lahti J. M.; Reinberg D. ASAP, a novel protein complex involved in RNA processing and apoptosis. Mol. Cell. Biol. 2003, 23 (8), 2981–2990. 10.1128/MCB.23.8.2981-2990.2003. [Europe PMC free article] [Abstract] [CrossRef] [Google Scholar]
- Zhang Y.; Skolnick J. Scoring function for automated assessment of protein structure template quality. Proteins 2004, 57 (4), 702–710. 10.1002/prot.20264. [Abstract] [CrossRef] [Google Scholar]
- Jumper J.; Evans R.; Pritzel A.; Green T.; Figurnov M.; Ronneberger O.; Tunyasuvunakool K.; Bates R.; Žídek A.; Potapenko A.; et al. Highly accurate protein structure prediction with AlphaFold. Nature 2021, 596 (7873), 583–589. 10.1038/s41586-021-03819-2. [Europe PMC free article] [Abstract] [CrossRef] [Google Scholar]
- Kozakov D.; Hall D. R.; Xia B.; Porter K. A.; Padhorny D.; Yueh C.; Beglov D.; Vajda S. The ClusPro web server for protein-protein docking. Nat. Protoc. 2017, 12 (2), 255–278. 10.1038/nprot.2016.169. [Europe PMC free article] [Abstract] [CrossRef] [Google Scholar]
- Schneidman-Duhovny D.; Inbar Y.; Nussinov R.; Wolfson H. J. PatchDock and SymmDock: servers for rigid and symmetric docking. Nucleic Acids Res. 2005, 33 (Web Server), W363–W367. 10.1093/nar/gki481. [Europe PMC free article] [Abstract] [CrossRef] [Google Scholar]
- Sakashita E.; Tatsumi S.; Werner D.; Endo H.; Mayeda A. Human RNPS1 and its associated factors: a versatile alternative pre-mRNA splicing regulator in vivo. Mol. Cell. Biol. 2004, 24 (3), 1174–1187. 10.1128/MCB.24.3.1174-1187.2004. [Europe PMC free article] [Abstract] [CrossRef] [Google Scholar]
- Shen H.; Kan J. L.; Green M. R. Arginine-serine-rich domains bound at splicing enhancers contact the branchpoint to promote prespliceosome assembly. Mol. Cell 2004, 13 (3), 367–376. 10.1016/S1097-2765(04)00025-5. [Abstract] [CrossRef] [Google Scholar]
- Valcárcel J.; Gaur R. K.; Singh R.; Green M. R. Interaction of U2AF 65 RS Region with Pre-mRNA of Branch Point and Promotion Base Pairing with U2 snRNA. Science 1996, 273, 1706–1709. 10.1126/science.273.5282.1706. [Abstract] [CrossRef] [Google Scholar]
- Graveley B. R. A protein interaction domain contacts RNA in the prespliceosome. Mol. Cell 2004, 13 (3), 302–304. 10.1016/S1097-2765(04)00055-3. [Abstract] [CrossRef] [Google Scholar]
- De Silva N.; Lehman N.; Fargason T. U.; Paul T.; Zhang Z.; Zhang J. Unearthing a novel function of SRSF1 in binding and unfolding of RNA G-quadruplexes. Nucleic Acids Res. 2024, 52, 4676–4690. 10.1093/nar/gkae213. [Europe PMC free article] [Abstract] [CrossRef] [Google Scholar]
- Valdés-Tresanco M. S.; Valdés-Tresanco M. E.; Valiente P. A.; Moreno E. gmx_MMPBSA: A New Tool to Perform End-State Free Energy Calculations with GROMACS. J. Chem. Theory Comput. 2021, 17 (10), 6281–6291. 10.1021/acs.jctc.1c00645. [Abstract] [CrossRef] [Google Scholar]
- Miller B. R. III; McGee T. D. Jr.; Swails J. M.; Homeyer N.; Gohlke H.; Roitberg A. E. MMPBSA.py: An Efficient Program for End-State Free Energy Calculations. J. Chem. Theory Comput. 2012, 8 (9), 3314–3321. 10.1021/ct300418h. [Abstract] [CrossRef] [Google Scholar]
- Rha J.; Jones S. K.; Fidler J.; Banerjee A.; Leung S. W.; Morris K. J.; Wong J. C.; Inglis G. A. S.; Shapiro L.; Deng Q.; et al. The RNA-binding protein, ZC3H14, is required for proper poly (A) tail length control, expression of synaptic proteins, and brain function in mice. Hum. Mol. Genet. 2017, 26 (19), 3663–3681. 10.1093/hmg/ddx248. [Europe PMC free article] [Abstract] [CrossRef] [Google Scholar]
- Novikova I. V.; Hennelly S. P.; Tung C. S.; Sanbonmatsu K. Y. Rise of the RNA machines: exploring the structure of long non-coding RNAs. J. Mol. Biol. 2013, 425 (19), 3731–3746. 10.1016/j.jmb.2013.02.030. [Abstract] [CrossRef] [Google Scholar]
- Adams P. L.; Stahley M. R.; Kosek A. B.; Wang J.; Strobel S. A. Crystal structure of a self-splicing group I intron with both exons. Nature 2004, 430 (6995), 45–50. 10.1038/nature02642. [Abstract] [CrossRef] [Google Scholar]
- Robart A. R.; Chan R. T.; Peters J. K.; Rajashankar K. R.; Toor N. Crystal structure of a eukaryotic group II intron lariat. Nature 2014, 514 (7521), 193–197. 10.1038/nature13790. [Europe PMC free article] [Abstract] [CrossRef] [Google Scholar]
- Zhang X.; Zhan X.; Yan C.; Zhang W.; Liu D.; Lei J.; Shi Y. Structures of the human spliceosomes before and after release of the ligated exon. Cell Res. 2019, 29 (4), 274–285. 10.1038/s41422-019-0143-x. [Europe PMC free article] [Abstract] [CrossRef] [Google Scholar]
- Fromm S. A.; O’Connor K. M.; Purdy M.; Bhatt P. R.; Loughran G.; Atkins J. F.; Jomaa A.; Mattei S. The translating bacterial ribosome at 1.55 Å resolution generated by cryo-EM imaging services. Nat. Commun. 2023, 14 (1), 1095.10.1038/s41467-023-36742-3. [Europe PMC free article] [Abstract] [CrossRef] [Google Scholar]
- Zhang K.; Keane S. C.; Su Z.; Irobalieva R. N.; Chen M.; Van V.; Sciandra C. A.; Marchant J.; Heng X.; Schmid M. F.; et al. Structure of the 30 kDa HIV-1 RNA Dimerization Signal by a Hybrid Cryo-EM, NMR, and Molecular Dynamics Approach. Structure 2018, 26 (3), 490–498.e3. 10.1016/j.str.2018.01.001. [Europe PMC free article] [Abstract] [CrossRef] [Google Scholar]
- Šponer J.; Bussi G.; Krepl M.; Banáš P.; Bottaro S.; Cunha R. A.; Gil-Ley A.; Pinamonti G.; Poblete S.; Jurečka P.; et al. RNA Structural Dynamics As Captured by Molecular Simulations: A Comprehensive Overview. Chem. Rev. 2018, 118 (8), 4177–4338. 10.1021/acs.chemrev.7b00427. [Europe PMC free article] [Abstract] [CrossRef] [Google Scholar]
- Cléry A.; Sinha R.; Anczuków O.; Corrionero A.; Moursy A.; Daubner G. M.; Valcárcel J.; Krainer A. R.; Allain F. H. T. Isolated pseudo-RNA-recognition motifs of SR proteins can regulate splicing using a noncanonical mode of RNA recognition. Proc. Natl. Acad. Sci. U.S.A. 2013, 110 (30), E2802–1110.1073/pnas.1303445110. [Europe PMC free article] [Abstract] [CrossRef] [Google Scholar]
- Novikova I. V.; Hennelly S. P.; Sanbonmatsu K. Y. Sizing up long non-coding RNAs: do lncRNAs have secondary and tertiary structure?. Bioarchitecture 2012, 2 (6), 189–199. 10.4161/bioa.22592. [Europe PMC free article] [Abstract] [CrossRef] [Google Scholar]
- Gilbert S. D.; Rambo R. P.; Van Tyne D.; Batey R. T. Structure of the SAM-II riboswitch bound to S-adenosylmethionine. Nat. Struct. Mol. Biol. 2008, 15 (2), 177–182. 10.1038/nsmb.1371. [Abstract] [CrossRef] [Google Scholar]
- Militti C.; Maenner S.; Becker P. B.; Gebauer F. UNR facilitates the interaction of MLE with the lncRNA roX2 during Drosophila dosage compensation. Nat. Commun. 2014, 5, 4762.10.1038/ncomms5762. [Abstract] [CrossRef] [Google Scholar]
- Luscombe N. M.; Laskowski R. A.; Thornton J. M. Amino acid-base interactions: a three-dimensional analysis of protein-DNA interactions at an atomic level. Nucleic Acids Res. 2001, 29 (13), 2860–2874. 10.1093/nar/29.13.2860. [Europe PMC free article] [Abstract] [CrossRef] [Google Scholar]
- Pettersen E. F.; Goddard T. D.; Huang C. C.; Meng E. C.; Couch G. S.; Croll T. I.; Morris J. H.; Ferrin T. E. UCSF ChimeraX: Structure visualization for researchers, educators, and developers. Protein Sci. 2021, 30 (1), 70–82. 10.1002/pro.3943. [Europe PMC free article] [Abstract] [CrossRef] [Google Scholar]
- Laskowski R. A.; Rullmann J.; MacArthur M. W.; Kaptein R.; Thornton J. AQUA and PROCHECK-NMR: programs for checking the quality of protein structures solved by NMR. J. Biomol. NMR 1996, 8 (4), 477–486. 10.1007/bf00228148. [Abstract] [CrossRef] [Google Scholar]
- Laskowski R. A.; Jabłońska J.; Pravda L.; et al. PDBsum: Structural summaries of PDB entries. Protein Sci. 2018, 27 (1), 129–134. 10.1002/pro.3289. [Europe PMC free article] [Abstract] [CrossRef] [Google Scholar]
- Lindorff-Larsen K.; Piana S.; Palmo K.; Maragakis P.; Klepeis J. L.; Dror R. O.; Shaw D. E. Improved side-chain torsion potentials for the Amber ff99SB protein force field. Proteins 2010, 78 (8), 1950–1958. 10.1002/prot.22711. [Europe PMC free article] [Abstract] [CrossRef] [Google Scholar]
- Jorgensen W. L.; Jayaraman C.; Jeffry D.; Madura; et al. Comparison of simple potential functions for simulating liquid water. J. Chem. Phys. 1983, 79, 926–935. 10.1063/1.445869. [CrossRef] [Google Scholar]
- Hess B.; Bekker H.; Berendsen H. J. C.; Fraaije J. G. E. M. LINCS: a linear constraint solver for molecular simulations. J. Comput. Chem. 1997, 18, 1463–1472. 10.1002/(SICI)1096-987X(199709)18:12<1463::AID-JCC4>3.0.CO;2-H. [CrossRef] [Google Scholar]
- Darden T.; York D.; Pedersen L. Particle mesh Ewald: an N.log (N) method for Ewald sums in large systems. J. Chem. Phys. 1993, 98, 10089–10092. 10.1063/1.464397. [CrossRef] [Google Scholar]
- Turner P. J.XMGRACE. Version 5.1.19; Center for Coastal and Land-Margin Research, Oregon Graduate Institute of Science and Technology: Beaverton, OR, 2005.
- Humphrey W.; Dalke A.; Schulten K. VMD: visual molecular dynamics. J. Mol. Graph. 1996, 14 (1), 33–38. 10.1016/0263-7855(96)00018-5. [Abstract] [CrossRef] [Google Scholar]
- The PyMOL Molecular Graphics System, Version 3.0 Schrödinger, LLC, 2010.
Articles from ACS Omega are provided here courtesy of American Chemical Society
Citations & impact
This article has not been cited yet.
Impact metrics
Alternative metrics

Discover the attention surrounding your research
https://www.altmetric.com/details/168932377
Data
Data behind the article
This data has been text mined from the article, or deposited into data resources.
Genes & Proteins
- (4 citations) UniProt - Q15287
Protein structures in PDBe (2)
-
(5 citations)
PDBe - 4A8XView structure
-
(2 citations)
PDBe - 4PLXView structure
RefSeq - NCBI Reference Sequence Database
- (2 citations) RefSeq - NR_002819.4
Similar Articles
To arrive at the top five similar articles we use a word-weighted algorithm to compare words from the Title and Abstract of each citation.
Human RNPS1 and its associated factors: a versatile alternative pre-mRNA splicing regulator in vivo.
Mol Cell Biol, 24(3):1174-1187, 01 Feb 2004
Cited by: 74 articles | PMID: 14729963 | PMCID: PMC321435
Serine-rich domain of RNPS1 functions in activation of alternative splicing.
Genes Cells, 28(8):615-623, 19 May 2023
Cited by: 0 articles | PMID: 37204171
RNPS1 functions as an oncogenic splicing factor in cervical cancer cells.
IUBMB Life, 75(6):514-529, 04 Nov 2022
Cited by: 5 articles | PMID: 36300671
MALAT1 -- a paradigm for long noncoding RNA function in cancer.
J Mol Med (Berl), 91(7):791-801, 26 Mar 2013
Cited by: 459 articles | PMID: 23529762
Review